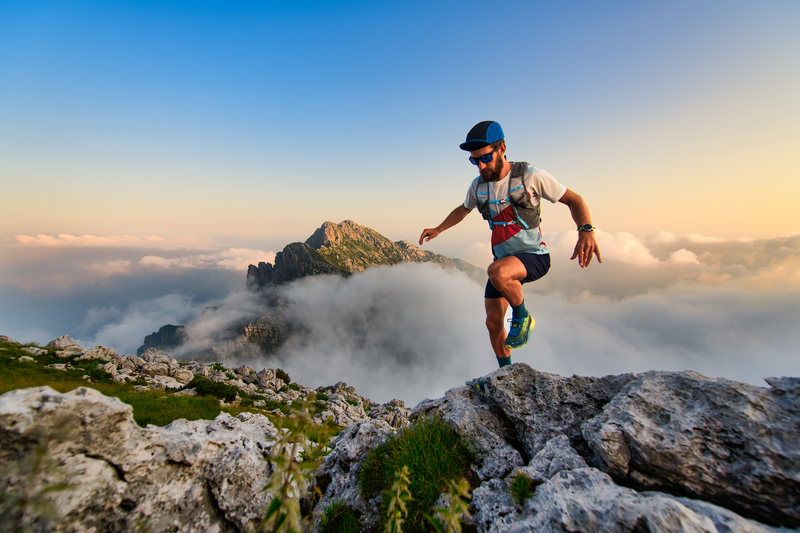
95% of researchers rate our articles as excellent or good
Learn more about the work of our research integrity team to safeguard the quality of each article we publish.
Find out more
ORIGINAL RESEARCH article
Front. Mar. Sci. , 02 April 2025
Sec. Marine Fisheries, Aquaculture and Living Resources
Volume 12 - 2025 | https://doi.org/10.3389/fmars.2025.1528586
Effective fisheries management is crucial for the sustainable use of fishery resources, increasing relying on stock assessments. The Megalaspis cordyla an economically important fish species in Pakistan, require an accurate assessment of its current biomass to take effective management strategies. This study utilized stock assessment techniques, including the Catch-Based Monte Carlo Maximum Sustainable Yield (CMSY), length-based Bayesian Biomass (LBB), Just Another Bayesian Biomass Assessment (JABBA), and ARIMA models. While CMSY, BSM, JABBA, ARIMA rely on annual catch-effort data, while LBB analyzes length-frequency data along with resilience inputs. An analysis of 15 years of catch data (2007-2021), and 1,442 length-frequency data from Pakistani waters revealed that the fishery is overfished both in terms of exploitation and biomass (LBB at F/M = 1.6, B/BMSY = 0.76 and B/B0 = 0.27). The CMSY method estimated biological reference points as r = 0.53, k = 231, and a maximum sustainable yield (MSY) of 3.06. In comparison, the BSM provide values of r =0.03, k =271, MSY = 2.56. The JABBA model estimated MSY of 3.637, with a biomass to MSY2021 (B2021/BMSY) of 0.68 and F2021/FMSY of 1.56, indicating excessive exploitation. The projected biomass ratio (B2021/BMSY) of 0.798, is<1, confirms overexploitation. Additionally, the ARIMA (2, 0, 1) model, demonstrated the lowest mean square error, predicts a significant upward trend in fish catches in the near future. The findings across all models consistently indicate that the M. cordyla fishery is overfished, with current catches exceeding sustainable limits. Biological reference points from CMSY, JABBA, and LBB models, all below 1.0, underscore the unsustainable of the fishery. If current trend continues, the fishery faces a substantial risk of collapse. To mitigate this, immediate management measures should be implemented to promote the sustainable utilization of this critical fishery resource in Pakistan.
Fisheries resources play a vital role in achieving sustainable development goals, supporting global food and security, and maintaining the health of marine ecosystems. These resources are not only essential for national economies but also for the livelihoods and cultural heritage of coastal communities (FAO, 2023). In Pakistan, the marine fisheries sector significantly contributes to the national economy by providing employment, ensuring food security, and boosting exports. This sector encompasses a diverse range of species and relies on both artisanal and industrial fishing practices. Pakistan coast spans 1,001 km, stretching from the Indian to the Iranian borders, with a continental shelf up to 350 nautical miles (UN, 2015; Pakistan Navy, 2016). The coastline is divided into two distinct regions: the Sindh and Pakistan coasts. The Sindh coast features sandy and muddy seabed enriched by freshwater inflows from the Indus River, which fosters fertile mangrove ecosystems that serve as critical breeding and nursery grounds for various fishery resources. In contrast, the Balochistan coast comprises a rocky, uneven continental shelf (FAO, 2009). Pakistan marine waters are rich in resources, including large and small pelagic fish, demersal fish, shrimp, crabs, lobsters, and cephalopods (FAO, 2009). The marine fisheries industry in Pakistan accounts for approximately 57% of the country’s total fish and fishery products, contributing about 160.9 million US dollars to the gross domestic production (FAO, 2009). The sector supports the livelihoods of approximately 400,000 fishermen and their families. However, Pakistan’s fisheries have been severely impacted by overfishing in recent decades. Numerous studies highlight concerns about global fish stock depletion due to overexploitation (Hilborn et al., 2020). Nevertheless, research suggests that fish stocks can recover if fishing efforts are reduced to sustainable levels via effective fishery management (Worm et al., 2009).
In recent years, national and regional fisheries regulations have increasingly shifted toward science-based management approaches to ensure the sustainability of both commercial and non-commercial fish stocks (MSA, 2007; CFP, 2013). Several factors contribute to the depletion of fisheries resources, including climate change, habitat destruction, water pollution, and disease outbreak. However, overfishing remains the primary threat (Jackson et al., 2001), exacerbated by the rise of industrial fishing since the last century (McIntyre, 1991). Overfishing has significantly disrupted species interactions within the marine ecosystems (Jackson et al., 2001). Pakistan’s fisheries resources operate under open-access conditions, and recent surveys reveal significant stock depletion (Fanning et al., 2011). Achieving balance in natural ecosystems requires accurately quantifying the status of fisheries, not only for sustainable harvests but also for sustainability of marine ecosystems. However, stock assessments are often hindered by limited data availability. Traditional tools often fail to evaluate sustainability goals for key fish stocks due to inadequate data (Costello et al., 2012). Advanced stock evaluation models require extensive datasets, including fishing effort, time-series catch data, and species life history information (Costello et al., 2012; Methot and Wetzel, 2013). Such datasets are often unavailable in developing countries like Pakistan. As a result, researchers frequently use catch-and length-based models (Froese et al., 2017, 2018, 2019). Data limitations in Pakistan are a significant challenge, with over 90% of global fisheries facing similar constraints (Geromont and Butterworth, 2015). While the fisheries department collects annual data on catches and fishing vessels, this data is insufficient for comprehensive stock assessments. To address this gap, more tailored models that utilize limited datasets are needed to calculate biological reference points (BRPs) for maintaining fish stocks. Several methods have been developed to overcome data deficiencies. The Catch-Based Maximum Sustainable Yield (CMSY) and Bayesian Schaefer Model (BSM) offer promising solutions by using time-series catch and efforts data to estimate biomass, MSY, fishing rate (F/Fmsy) relative biomass size (B/Bmsy) and other related fisheries reference points for given stock (Martell and Froese, 2013; Froese et al., 2017). Just Another Bayesian Biomass Assessment (JABBA) models also requires catch and catch per unit effort (CPUE) data (Froese et al., 2017). These models provide reliable insights into fisheries status using incomplete abundance data, aligning analyses with specific biomass conditions (Wang et al., 2020). Similarly, the LBB technique relies on the ratio of natural mortality to somatic growth (M/K) and fishing mortality to somatic growth (F/K) to analyze the proportion of exploited to stable biomass (B/B0), stock levels needed for maximum sustainable yield (MSY) (B/BMSY) (Yue et al., 2021). This length-based approach is particularly effective for estimating species growth throughout their lifespans (Froese et al., 2019). By integrating these methods, fisheries management in data-limited context can be significantly improved. Employing innovative tools such as CMSY, BSM, JABBA, and LBB can provide actionable insights to ensure the sustainability of Pakistan marine fisheries.
In fisheries sciences, a wide range of mathematical and statistical techniques are employed to analyze and interpret the dynamics of commercially important fish populations (Haddon, 2011). Among these, autoregressive integrated moving average (ARIMA) models, introduced by Box and Jenkins (1976), are commonly used for time series analysis. ARIMA models assume a linear relationship within the time series data, where each observation is expressed as a linear function of preceding values, with an added error term. This univariate approach, which focuses on linear associations among variables, offers both strength and limitations, depending on the complexity of the data. ARIMA models share foundational principles with other time-series models, such as moving-average (MA) and autoregressive (AR) models, and are capable of capturing seasonal and cyclical patterns. These features contribute to their broad application across scientific and engineering fields, including fisheries science. Their utility in fisheries sciences is particularly significant for analyzing datasets that are constrained by limitations in size or complexity, such as those from the northern Arabian Sea (Sathianandan and Srinath, 1995; Czerwinski et al., 2007; Prista et al., 2011).
Torpedo scad (Megalaspis cordyla), a small pelagic fish from the Perciformes order, inhabits reef-associated areas at depths of 20-100 m (Al-Sakaff and Esseen, 1999). This species is widely distributed across the Indo-Pacific region, including Pakistan, and is commonly found in schools (Kuiter and Tonozuka, 2001). While it can grow up to 80 cm in length, individuals typically reach around 45 cm. The maximum recorded weight of this species is 4.0 kg, and it has a lifespan of 3-5 years. Sexual maturity is reached at approximately 22 cm, marking a crucial point in its life cycle (Smith-Vaniz, 1984; Smith-Veniz, 2003; Hu et al., 2015; Qamar et al., 2018a). It is commercially significant species, primarily exported in frozen form, contributing substantially to Pakistan economy. Due to its high commercial value, it is classified with the higher price category with high price category (Sumaila et al., 2007). Additionally, it is also highly vulnerable to climate change and fishing pressure (Jones and Cheung, 2017; Qamar and Panhwar, 2018b). Along the coastal regions, it is also widely consumed due to its convenient size. Although the biological aspects and ecological importance of M. cordyla have been well-documented (Zafar et al., 2000; Qamar et al., 2016), there has been limited research on its stock assessment in Pakistani waters (Qamar and Panhwar, 2018b; Razaaq et al., 2019). Given its commercial importance, assessing the current biomass of this fishery is essential. Various modeling approaches, including CMSY, BSM, LBB, JABBA, and ARIMA used in this assessment. CMSY is particularly beneficial in data-limited context, relying solely on catch data to estimate stock status and sustainable yield. BSM and JABBA require both catch and CPUE data, providing a more comprehensive view of population dynamics through Bayesian modeling techniques. LBB, using length-frequency data, offers insights into stock status and growth parameters. ARIMA models, on the other hand, use historical time-series data to forecast future catch trends, making them invaluable for developing predictive management strategies. In Pakistan, where detailed data on fishing effort and biological parameters are often lacking, these models provide complementary information on stock status and future projections. For instance, CMSY can deliver preliminary assessment using only catch data, while LBB offers additional reliability by analyzing size-based data. Comparison across models can strengthens confidence in results, particularly in data-poor context. Understanding the current status of M. cordyla stocks through these methodologies is crucial for informed decision-making and sustainable fisheries management in Pakistan. By integrating these approaches, fisheries managers can develop strategies to ensure the long-term viability of this economically significant species, addressing challenges posed by limited data availability.
Yearly catch data for M. cordyla were sourced from the FAO (UN) global marine fish catch database (https://www.fao.org/fishery/statistics-query/en/capture/capture_quantity) for the period 2007-2021, a reputable and globally recognized fishery database. While the data on fishing boats were obtained from annual fisheries statistical book published by the Marine Fisheries Department, Pakistan (MFD, 2017, 2021). Over the 15-year period, total annual catches (in metric tons, MT) and fishing effort (number of fishing boats) were analyzed. The highest recorded catch occurred in 2008, with a total of 5,924 MT. However, catches consistently declined in subsequent years, with the lowest recorded catch of 3,321 MT in 2010. The average catch over the 15 years was 4,259 MT, with an average fishing effort of 17,509 boats. Despite the declining catches, fishing efforts showed an increasing trend over the same time period (Figure 1). Length-frequency distribution data were collected along the Pakistani coast in 2021. A total of 1,442 specimens were measured, with fork lengths ranging from 170 mm to 450 mm (± 85.15 mm). These length-frequency data, along with the catch and effort information (number of fishing boats) and catch per unit effort (CPUE), were analyzed using R statistical software with the LBB (version 33a.R), CMSY (version 2019f.R), and JABBA (JABBAv1.1.R) models developed by Froese et al. (2018, 2019), and Winker et al. (2018).
Figure 1. Annual catch (MT) and effort data (number of fishing boats) of Torpedo scad fishery from Pakistani waters during 2007-2021.
Froese et al. (2019) introduced the LBB model to analyze length-based data, providing insights into the exploitation rate for fishery resources. This method is particularly effective for species with determinate growth throughout their lifespan and for invertebrates. The model outputs key parameters such as the asymptotic length (Linf), length at capture (Lc), natural mortality (M), and fishing mortality (F) (Froese et al., 2018, 2019). Using these parameters, standard fishery equations estimate the reduction in stock biomass compared to its unexploited state (B/B0). Additionally, the LBB model provides proxies for the biomass required to achieve BMSY/B0 and Lc (length at capture), and Lc opt (optimal length at capture), which helps optimize both stock sustainability and catch efficiency.
The Von-Bertalanffy (1938) growth function is used in this analysis and is expressed by following Equation 1:
t is age, L∞ is the asymptotic size, K denote growth coefficient (yr-1), to is hypothetical age of fish when length is zero. the VBGF incorporates factors such as growth, selectivity, and mortality enabling an analysis of catch trends relative to stock status (Froese et al., 2018). Mortality (F/M) was estimated as F/M = (F/K)/(M/K) in LBB parameters. The determination of the optimum length (Lopt) for maximum yield and optimum length at first catch (Lc_opt) is taken from Equations 2 and 3 (Holt, 1958; Froese et al., 2017).
The yield er recruit (Y’/R) (Beverton and Holt, 1966) was estimated using (Equation 4)
Index of catch per unit effort per recruit (CPUE’/R) estimated by Beverton and Holt (1966) Equation 5:
The relative stock per recruit during population exploitation phase, excluded catching activities (Froese et al., 2018) by Equation 6:
This equation content, (B’0 >Lc/R) signifies an exploitation proportion (>Lc) of stable biomass (B0). Relative biomass (B/B0) is determined by the Equation 7, as specified for the exploitation population (Beverton and Holt, 1966).
The capability of production MSY (BMSY/B0, where B0 is expected at 0.5) was estimated by re-running Equations 4–8 (Froese et al., 2018), and it serves as replacement for the relative biomass.
This study divided the fish species it looked at into three categories: Strongly overfished (B/Bmsy<0.2-0.5), grossly overfished (B/Bmsy<0.5), overfished (B/Bmsy 0.5 - 1), and healthy (B/Bmsy >1) (Palomares et al., 2018).
The comprehensive stock evaluation, including a detailed description and analysis, is provided in Froese et al. (2018, 2019). For the LBB method, length-frequency data (1,442) were used, and basis prior information was sources from FishBase (Table 1), which includes essential parameters such as species resilience and growth rates. This prior information is integral to the LBB method and helps to inform the model assessment of the stock status.
Table 1. Prior and basic input information needed for the LBB model of Torpedo scad fishery in Pakistan.
The CMSY method, which incorporates the BSM model, was utilized used to evaluate the status of fish stocks (Froese et al., 2017). key statistics are in Table 1, while the relative priors are in Table 2. Parameters such as B/BMSY, exploitation rate (F/FMSY), carrying capacity (k), and intrinsic growth rate of the population (r) were estimated using annual catch data, CPUE, and resilience parameters (Froese et al., 2017). Biomass for the subsequent year was estimated using the following Equations 9, 10.
Table 2. Suggested prior biomass ranges of the biomass under assessment from supplementary input (Froese et al., 2017).
Bt+1 is harvested stock (t+1) year, Bt is the present stock, and Ct, catch in year t., biomass falls k:
The resilience of Torpedo scad is classified as “medium” according to FishBase. Table 2 presents the prior ranges for the CMSY parameters, with, r ranging from 0.3 to 0.8. Biomass priors are also provided in Table 2 (Froese and Pauly, 2021). (q) is catchability coefficient; calculated by following equation:
CPUE in year t, Bt is annual stock and q is catch coefficient (Equation 11). The CMSY and BSM parameters stock biomass is in Table 2 (Froese and Pauly, 2015).
The JABBA model is a Bayesian State-Space surplus production model (SPM) designed to utilize CPUE time-series data. It employs a generalized three-parameter SPM developed by Pella and Tomlinson (1969) expressed as follows. These models do not require any age-structure data (Winker et al., 2018).
Where, k, r, B, are intrinsic population rate, carrying capacity and biomass at time t, and m is the parameters of B/K ration of surplus production
Equations from 13, 14 and 15 BMSY and FMSY are fishing mortality at MSY
C=FB, fishing mortality, C is the annual catch, therefore MSY can be calculated Equation 17.
Together Equations 14 and 16, it is possible to show r in Equation 18 as
From Equations 14 and 18 shows the possibility of converting MSY/BMSY and BMSY/K estimates into r and m.
From Equations 12–18, where, BMSY and FMSY and fishing mortality calculation, C= FB, fishing mortality, C is the annual catch,
Equation 19, represents the recruitment potential, which specify biomass levels ranging from 0.25-0.5, commonly used as recruitment overfishing limits (Palomares et al., 2018). This equation is a composite model for the Pella-Tomlinson model Plim. Equation 19. This model helps in assessing the potential overfishing limits based on recruitment dynamics, a crucial component for evaluating sustainable fishing practices.
The ARIMA model uses past observations and error terms to predict future values in a time series (Brockwell and Davis, 2016). An AR model of order p is denoted by AR(p), while MA model clarifies Yt, as a role of an independent term and errors in the past terms and in represented by MA(q). ARIMA model can be stated as:
Yt = value of the time series at time t, ϕ = AR coefficients, θ = MA coefficients, ϵt = white noise error team, p = number of AR terms, d = degree of differencing, q = number of MA terms.
For, future predicted values, the forecast can be derived from:
Where, h is forecast horizon.
A statistical method can be used to examine the presence of stationarity, commonly referred to as the unit-root hypothesis test. Widely used approach for this purpose is the Augmented Dicky-Fuller (ADF) test, which assess stationarity (Dickey and Fuller, 1979). The Akaike Information Criterion (AIC) and Bayesian Information Criterion (BIC) values for ARIMA model were calculated using the following formulas, where T’ defines the observations used for estimation of parameters.
Estimation of accuracy metrics
Mean error (ME);
Fi = forecasted values, Ai = actual values, n= number of observations
Mean Absolute Error (MAE):
Root Mean Squared Error (RMSE):
Mean Absolute Scaled Error (MASE):
The ARIMA model provides framework for forecasting time series data by leveraging past observations and error terms. The described metrics (ME, MAE, RMSE, and MASE) help assess the accuracy of the forecasts, enabling better decision-making based on model performance.
The length-frequency data (1,442) were used to estimate the stock biomass using the LBB model. The calculated parameters, including F/M = 1.6, B/BMSY = 0.76 and B/B0 = 0.27, indicate that the stock is overexploited, and the biomass is severely depleted (Table 3). Additionally, the size at first capture is below the optimal length, indicating that smaller fish are being harvested, which exacerbates the overfishing issue. The estimated asymptotic length (L∞) for this species 46.6 FL-cm (Figure 2). This combination of overfishing and the capture of juvenile fish is contributing significantly to the depletion of the stock.
Figure 2. The aggregated length frequency (total of 1,442 specimens) and stock estimation using length-based biomass method for Torpedo scad fishery from Pakistani waters during 2021. Lc defines the size of first capture (green line) and indicating the catch of small size of fish, Linf indicate species length limit and Lopt shows the length at maximum sustainable catch.
The annual catch was higher during the early years (2007-2009) followed by a consistent decline over time (Figure 3A). Both the CMSY and BSM models provided reliable evaluations for assessing sustainability of the fishery. The CMSY model estimated biological reference points with r = 0.53, k = 231, and MSY = 3.06 (Table 4A), while the BSM model estimated r = 0.03, k = 271, and MSY = 2.56 for Pakistani waters (Table 4B). The estimated B2021/BMSY biomass ratio was 0.798, which is below 1, indicating that the stock is overexploited (Figures 3B–D; Table 4B). Additionally, the fishing mortality (F2021/FMSY = 1.56) exceeded the sustainable catch limit of 1 (Figure 3E) and no values were recorded below the curve (Figure 3F). The Kobe plot further highlights that F2021/FMSY is greater than 1 and B2021/BMSY is less than 1, confirming the conclusion that the stock is overfished. The change in biomass over time is shown as a black line, with initial years falling in the green zone (square marker), but over time the biomass shifted into the red zone (triangle marker), signifying that 95.5% of the depletion is attributed to fishing activities (Figure 4). Fishing mortality and MSY biomass exceeded sustainable catch limits (Figure 5), further confirming overfishing in Pakistani waters. The JABBA model contributed a more refined analysis, with key parameters presented in Table 4B and Figures 6 and 7. The estimated MSY was 3.637, with B2021/BMSY = 0.68 and FMSY = 1.56. The Kobe plot (Figure 7A) illustrated catch levels over time, starting in the green zone (square markers), but shifting to red zone (triangle markers), indicating the high fishing mortality. The surplus production and biomass model (Figure 7B) also shows that catches have exceeded the surplus production, which further confirms stock overexploitation. These findings are consistent with the BSM model results, where BMSY and FMSY values have surpassed sustainable catch limits. Collectively, the JABBA outputs further emphasize that that the stock is under significant stress and in an overexploited condition.
Figure 3. Torpedo scad fish stock estimation of CMSY and BSM model. (A) Black line indicates changes in fish stock, while the blue line represents the average, with red dots showing the catch. (B, C) These panels depict the r-k variables from the CMSY (blue) and BSM model (red) models, along with 95% confidence intervals. (D) Relative biomass during the study period. (E) F2021/FMSY values across the years, with good model performance indicated by the proximity of the blue and red curves. Dotted values above the parabola in the phase plot suggest a declining stock, while those below the parabola indicate potential stock recovery. (F) Catch/MSY in relation to relative biomass, with CMSY (blue) and BSM (red) curves. Points above parabola suggest future biomass shrinkage, while points below the parabola indicate potential stock recovery.
Table 4A. Estimated outcomes for fisheries reference points of Torpedo scad stocks derived from CMSY method.
Table 4B. Estimated outcomes for fisheries reference points of M.s cordyla fishery stocks derived from BSM, and JABBA method outputs.
Figure 4. Kobe plot of Torpedo scad fishery during 2007-2021 from Pakistani waters. The change in F/FMSY and B/B/BMSY during the time. The black line shows the stock variation with initial (2007), and last year (2021) status in (square and triangle). Most of the catch shows in red quadrate which clearly defines the overfishing of the stock from Pakistani waters.
Figure 5. Management information of BSM of Torpedo scad fishery in Pakistani waters defines the annual catch, total biomass and exploitation rate in relation with biomass, Stock size with exploitation rate defines in black line with indicate the stock variation and light grey, grey and dark grey indicating the 50%, 80% and 95% confidence interval respectively.
Figure 6. The estimated outputs from the JABBA model for the Torpedo scad fishery in Pakistan include observed and expected indexes, as well as prior and posterior distribution, along with the estimated biomass trajectories.
Figure 7. The Kobe plot (A) and surplus production (B) illustrate the relationship between biomass and fishing efforts, as well as catches and surplus trajectories during the study period (2007-2021).
Catch data from 2007 to 2021 was analyzed using an ARIMA model to predict and forecast future trends and assess the statistical characteristics of both historical and projected values. The stationarity of the time series was confirmed using the ADF test, which yielded a p-value of 7.21e-06, significantly below the 0.05 threshold, allowing us to reject the null hypothesis and conclude that the data is stationary. Based on the Autocorrelation Function (ACF) and Partial Autocorrelation Function (PACF) patterns (Figure 8), ARIMA (1, 0, 0) was identified as the most suitable starting model. To ensure the optimality of the selected model, additional ARIMA models, such as ARIMA (2, 0, 1) and ARIMA (0, 0, 2), were tested and evaluated in Python based on the criterion of minimum AIC and BIC values. Among these, ARIMA (2, 0, 1) emerged as the best-fit model, with the lowest AIC (243.44) and BIC (246.98) values. The slightly higher BIC reflects a stronger penalty for model complexity, but both metrics suggest the ARIMA model achieved a good balance. The model performance was evaluated using statistical metrics to assess its accuracy and reliability. The ME was calculated at -89.93, indicating a slight underestimation of actual catch values. On average, the predicted catch is slightly lower than the actual catch, though the deviation is minor. The RMSE, which measures the standard deviation of residuals or predicted errors, was of 674.44, reflecting moderate variability between predicted and observed values. A lower RMSE would signify better model precision, indicating room for improvement. The MAE was recorded at 479.82, represents the average magnitude of prediction errors, regardless to direction. This suggests that, on average, the model predictions deviate from actual catch values by approximately 480 MT. The MASE was 1.12, indicating that the ARIMA model performed comparably to simpler baseline forecasts.
Figure 8. Autocorrelation function (ACF) and partial autocorrelation function (PACF) of Torpedo scad fishery time series.
Historical catch data shows significant year-to-year variability, with notable fluctuations. For instance, the catch dropped dramatically in 2010 to 3,321 MT, one of the lowest values in the datasets (Figures 1, 9). While, the model attempts to replicate these variations, some discrepancies are evident. In 2010, the model overestimated the catch by approximately 54%, indicating challenges in capturing the severity of the decline that year. Conversely, in 2016, when the catch reached one of its highest values (4,666 MT), the model underestimated the catch by around 17%, highlighting the need for further calibration to improve predictions during extreme fluctuations. Forecasted values from 2022 to 2026 suggest a gradual and steady increase in catches (Figure 9). In 2022, the model predicts a catch of 3,996 MT, rising to 4,300 MT by 2026. This projected trend aligns with the historical patterns, which indicate slow recovery after periods of lower catch rates in the early 2010s.
Figure 9. Time series catch analysis using ARIMA (2, 0, 1) model for actual, model fitted and five-year forecasted values.
Fisheries managers rely on biological reference points, stock assessments, and other key metrics, as essential tools for the sustainable management and optimal utilization of fishery resources. These tools, along with approaches like maximum economic yield and ecosystem-based fisheries management, help ensure ecological balance and socio-economic benefits while reducing risks like overexploitation. However, in Pakistan, many key fishery resources lack such estimates due to data limitations and the absence of reliable methods. The country faces significant challenges in acquiring complex datasets, such as research surveys and time-series age structure data, which complicates the establishment of sustainable fishing practices. Machine learning techniques, including ARIMA, LBB, CMSY, and JABBA models, offer reliable results even with limited datasets. Catch and effort data are available for many fish stocks and can be effectively utilized for sustainable fishery management.
In this study, CMSY, BSM, JABBA, and LBB models were applied to estimate the current status of Torpedo scad, while the ARIMA (2, 0, 1) model was used to predict the future catch trends and assess stock size in Pakistani waters. Biological reference points (B/BMSY) derived from the LBB, CMSY, and JABBA models were all below 1.0 (LBB 0.76, CMSY 0.798, JABBA 0.68), indicating overfishing as suggested by Froese et al. (2018), and Palomares et al. (2018). Additionally, the estimated values of F/FMSY were higher than sustainable levels, further confirming overexploitation. Stock evaluations based on frameworks of Froese et al. (2017); Martell and Froese (2013), and Palomares et al. (2018), reinforce these findings (Tables 3, 4a, b). The current fishing mortality (F > FMSY) exceeds standard thresholds by Froese et al. (2018), and the catch of small-sized individuals, as indicated by Lmean/Lopt and Lc/Lc_opt rations, further exacerbates the issue. The length recorded in this study (17 cm) (Table 1; Figure 2) is smaller than the optical length indicating the harvesting occurred before fish have reached full maturity at the size of 22 cm (Smith-Veniz, 2003; Hu et al., 2015). Similar findings have been reported for other fish species from Pakistani waters indicating the use of smaller trawl mesh sizes (Raza et al., 2022). This could be one of the factors contributing to stock depletion in Pakistani waters. The LBB model also highlighted that F/M values exceed 1, F/K values exceed 2, and Z/K values are high, all indicative of overfishing (Table 3). Similarly, the LBB model offered detailed stock information, while CMSY and JABBA models provided insights for setting sustainable total allowable catch (TAC) limits. These surplus production models (SPMs), such as CMSY and BSM, are widely recognized for their simplicity and effectiveness in assessing biomass removal from fish populations (Ren and Liu, 2020).
In present study, the ARIMA (2, 0, 1) model was identified as the most appropriate model for forecasting catch data, demonstrating superior predictive performance. These findings underscore the importance of selecting optimal model parameters to enhance forecasting accuracy. Previous studies have reported varying ARIMA model configurations for fishery forecasting, reflecting differences in data characteristics and regional trends. For instance, Paul and Das (2010) applied the ARIMA (1, 2, 1) model for inland fish production in India, while Yadav et al. (2020) found the ARIMA (1, 1, 0) model to be most effective for predicting fish production in Assam. Additionally, Pradeep et al. (2021) identified ARIMA (2, 2, 1) and ARIMA (3, 2, 0) as optimal models for forecasting inland and total fish production in India. The ARIMA (0, 2, 1) model has also been successfully applied in fisheries research (Mahalingaraya et al., 2018; Boruah et al., 2020; Rajani et al., 2024), while Selvaraja et al. (2020) reported that ARIMA (5, 1, 5) was suitable for forecasting for seer fish production and ARIMA (2, 2, 1) for mullet fish production. Koutroumanidis et al. (2006) applied various models to different fish species, using ARIMA (1, 1, 1) and (3, 1, 3) for anchovy, ARIMA (1, 0, 1) and (1, 1, 0) for hake, and ARIMA (1, 0, 0) and (0, 1, 1) for Atlantic bonito landing. The identification of the ARIMA (2, 0, 1) model in this study align with previous research that emphasizes the importance of customizing model parameters based on dataset characteristics. This result highlights the need for careful model selection to improve forecasting prevision in fisheries research. The ARIMA model, employed to forecast landing trends, demonstrated low mean square errors, making it particularly useful for data-poor fisheries characterized by limited datasets (Prista et al., 2011). This model can predict future conditions, highlighting potential risks for catch rates under current trajectories (Scandol, 2003; Mesnil and Petitgas, 2009). However, these predictions suggest that the fisheries may face increasing pressure, underscoring the urgent need for effective management strategies. These analyses provide critical insights into historical trends, projected changes, and the challenges confronting fisheries, providing a solid foundation for data-driven decision-making aimed at ensuring sustainable management.
Numerous studies have been conducted on the biological aspects of Torpedo scad in Pakistani waters (Qamar et al., 2018a, b), stock assessment utilizing traditional methods remain limited (e.g., Qamar et al., 2016; Razzaq et al., 2019). Previous assessments often used outdated catch data, but the present study provides an updated evaluation using modern techniques. Studies on various fish stocks in Pakistan have employed CMSY and LBB models to improve fisheries management and conservation (e.g., Raza et al., 2023; Kalhoro et al., 2024a, b). LBB models have been applied to several species to assess their status and suggest management measures for their conservation (Raza et al., 2022). Previously various surplus production models were used to assess different fish species in Pakistani waters to suggest management measures for sustaining the fish biomass (Afzaal et al., 2016, 2018; Kalhoro et al., 2013, 2014a, b, 2015a, b, 2017, 2018; Nadeem et al., 2017). These studies were conducted using the catch and effort data analysis and a stock production model incorporating covariates packages. Razaaq et al. (2019) indicated overexploitation of the fishery using outdated catch data and traditional methods. However, recent, advances have seen the CMSY and LBB models becoming more prevalent in Asian waters, including Pakistan, to suggest the stock status for better fishery management (Zhu et al., 2020, 2021; Liang et al., 2020; Zhang et al., 2022a, b, Al-Mamun et al., 2022; Raza et al., 2022; Khatun et al., 2023; Barua et al., 2023). Multiple modeling approaches were employed to cross-check and validate the results, ensuring robustness and reliability in the stock assessment. By utilizing various models, the study aims to account for different assumptions and methodological variations, enhancing the consistency and support for the findings. The results across the different models reveal minimal discrepancies in the overall outcomes, particularly with regard to the exploitation status of the stock. Despite the variations in the modeling approaches, all models consistently indicate that fishing mortality and exploitation rates exceed the recommended reference points suggested by Froese et al. (2018), and Palomares et al. (2018). This multi-model approach strengths the credibility of the conclusions, providing a comprehensive understanding of the fishery status and offering a solid basis for making informed management decisions to guide sustainable fisheries practices. Global fisheries assessments indicate a decline in fishery resources, driven by overexploitation and inadequate management practices (FAO, 2018; Palomares et al., 2020). Results from multi-model approach confirm that the stock is overfished, with confidence intervals indicating depletion across various exploitation scenario (Table 4). FAO fisheries data may introduce uncertainty due to potential under-reporting, particularly in small-scale fisheries (Zeller et al., 2016; Pauly and Zeller, 2016). However, CMSY, BSM, and JABBA incorporate prior knowledge and Bayesian approaches, which help adjust for incomplete data and maintain predictive reliability (Froese et al., 2017, 2019; Winker et al., 2018). Comparative analysis confirms their robustness, even in data-limited contexts. Despite uncertainties in catch data, the consistency of results across models strengthens confidence in these findings.
Depletion of inshore resources has forced fishing activities into offshore waters. Major fish stocks, including mackerel, tuna, croakers, and pomfrets, face severe threats (Razaaq et al., 2019; Baloch et al., 2020; Raza et al., 2022, 2023). Similar patterns of decline in fisheries resources is evident in the Indian Ocean and adjacent waters including Pakistan using similar methodologies (Nisar et al., 2021; Kalhoro et al., 2024a, b). Studies conducted in Bangladesh waters using JABBA model also provide robust information for better fishery management (Barua et al., 2023). These declines significantly impacted marine ecosystems and local economics, forcing many fishermen to seek alternative livelihood. This study represents the first comprehensive assessment of Torpedo scad stock and future catch predictions using machine learning methods. All models indicate that the stock is currently overexploited. Future catch predictions indicated that without immediate management intervention, the fishery may face significant risk of collapse. It may be advised to reduce fishing efforts in order to alleviate the fishing pressure on marine resources and promote long-term sustainability. To ensure sustainability, it is crucial to implement management measures such as catch limits, regular monitoring, and the development of tools to monitor small-scale fisheries that have limited data records. The ARIMA model, in particular, offers reliable forecasting technique for limited time-series data, aiding resource managers in effective planning.
Biological reference points are essential for achieving sustainable fisheries management, especially in data-limited context. Techniques like CMSY, LBB, JABBA, and ARIMA offer valuable tool for managing such fisheries, though they are limited by not accounting for environmental interactions between stocks. Integrating these methods with ecosystem-based approaches could provide a more comprehensive assessment. The results of this study showed that the M. cordyla fishery is overexploited, with LBB, CMSY, BSM, and JABBA models consistently indicating overfishing. The ARIMA (2, 0, 1) model predicts that if current catch trends continue, this fishery may face collapse. These findings highlight the effectiveness of using catch-only data for management in the absence of more complex datasets. Pakistani marine waters are open-access, which exacerbates the risk of overexploitation. Multi-model approaches could effectively support sustainable fisheries management to inform strategic decisions. To ensure sustainability, measures such as implementing a fishing ban during the breeding season, increasing trawl mesh sizes, and establishing marine protected areas (MPAs) are essential. Additionally, enforcing vessel monitoring and implementing stricter regulations are necessary to combat the illegal fishing activities. Scientific-based TAC limits should be established for each fishery. The alternative approaches, including aquaculture, could be considered to support recovery of wild stocks while ensuring food security. Collaboration between fishery managers and the scientific community is essential to ensure the sustainable utilization of fisheries resources.
The original contributions presented in the study are included in the article/supplementary material. Further inquiries can be directed to the corresponding author.
Ethical approval was not required for the study involving animals in accordance with the local legislation and institutional requirements because Commercial fish catch was used, obtained from FAO fisheries data.
MK: Data curation, Formal Analysis, Investigation, Methodology, Software, Visualization, Writing – original draft. JS: Formal Analysis, Funding acquisition, Software, Visualization, Writing – review & editing. LZ: Formal Analysis, Investigation, Methodology, Visualization, Writing – review & editing. ZL: Formal Analysis, Funding acquisition, Project administration, Resources, Supervision, Writing – review & editing. CL: Data curation, Formal Analysis, Investigation, Methodology, Visualization, Writing – review & editing. HR: Formal Analysis, Investigation, Methodology, Visualization, Writing – review & editing.
The author(s) declare that financial support was received for the research and/or publication of this article. Present work is supported by the Shandong University, Marine College, Weihai (202367800206), also supported by Guangzhou Bojin Information Technology Co. Ltd, Guangzhou (NO: BJ-2022-RD03); and funded by corresponding author. Guangzhou Bojin Information Technology Co. Ltd was not involved in the study design, collection, analysis, interpretation of data, the writing of this article, or the decision to submit it for publication.
Authors acknowledge Mr. Asmatullah, Dr. Asad (Balochistan Fisheries Department) and Abdul Salam Maheri (Marine Fisheries Department, Government of Pakistan) for providing fisheries data.
Author JS was employed by the company Guangzhou Bojin Information Technology Co. Ltd.
The remaining authors declare that the research was conducted in the absence of any commercial or financial relationships that could be construed as a potential conflict of interest.
The author(s) declare that no Generative AI was used in the creation of this manuscript.
All claims expressed in this article are solely those of the authors and do not necessarily represent those of their affiliated organizations, or those of the publisher, the editors and the reviewers. Any product that may be evaluated in this article, or claim that may be made by its manufacturer, is not guaranteed or endorsed by the publisher.
Afzaal Z., Kalhoro M. A., Buzdar M. A., Nadeem A., Saeed F., Haroon A., et al. (2016). Stock assessment of blue swimming crab Portunus pelagicus (Linnaeus 1758) from Pakistani waters (northern arabian sea). Pak J. Zool. 48, 1531–1541. doi: 0030-9923/2016/0005-1531
Afzaal Z., Kalhoro M. A., Buzdar M. A., Tariq S., Shafi M., Nadeem A., et al. (2018). Carapace length-weight and carapace length-width relationship of blue swimming crab from Pakistani waters. Indian J. Geo-Mari. Sci. 47, 890–896.
Al-Mamun M. A., Shamsuzzaman M. M., Schneider P., Mozumder M. M. H., Liu Q. (2022). Estimation of stock status using the LBB and CMSY methods for the Indian salmon leptomelanosoma indicum (Shaw 1804) in the bay of bengal, Bangladesh. J. Mar. Sci. Eng. 10, 1–9. doi: 10.3390/jmse10030366
Al-Sakaff H., Esseen M. (1999). Occurrence and distribution of fish species off Yemen (Gulf of Aden and Arabian Sea). Naga ICLARM Quart. 22 (1), 43–47. Available online at: https://hdl.handle.net/20.500.12348/2467.
Baloch A., Zhang K., Kalhoro M. A., Memon A. M., Kalhoro M. T., Buzdar M. A., et al. (2020). Preliminary investigations on stock analysis of Tigertooth Croaker (Otolithes ruber) from Balochistan coast, Pakistan. J. Anim. Plant Sci. 30, 1309–1318. doi: 10.36899/JAPS.2020.5.0149
Barua S., Liu Q., Alam M. S., Schneider P., Chowdhury S. K., Mozumder M. M. H. (2023). Assessment of three major shrimp stocks in Bangladesh marine waters using both length based and catch-based approaches. Sustainability 15, 12835. doi: 10.3390/su151712835
Beverton R. J. H., Holt S. J. (1966). “Manual of methods for fish stock assessment, part II—Tables of yield functions,” in FAO Fisheries Technical Paper No. 38 (Rev. 1) (FAO, Rome, Italy), 10.
Boruah B. B., Pinky R., Anupam D., Bidyutt B. H. (2020). Forecasting marine and total fish production in India using ARIMA models. Indian J. Econom. Business 19 (1), 161–174. Available online at: https://www.ashwinanokha.com/resources/fish%20p.edited.pdf.
Box G. E. P., Jenkins G. M. (1976). Time series analysis: Forecasting and control (Boca Raton, Florida: Holden-Day).
Brockwell P. J., Davis R. A. (2016). Introduction to time series and forecasting, third ed (Springer Nature Switzerland AG: Springer) pp. XIV, 425. doi: 10.1007/978-3-319-29854-2
CFP. (2013). Regulation (EU) No.1380/2013 of the European Parliament and of the Council of 11 December 2013 on the Common Fisheries Policy, amending Council Regulations (EC) No 1954/2003 and (EC) No 1224/2009 and repealing Council Regulations (EC) No 2371/2002 and (EC) No 639/2004 and Council Decision 2004/585/EC. Off. J. Eur. Union L354, 22–61. Available online at: http://data.europa.eu/eli/reg/2013/1380/oj.
Costello C., Ovando D., Hilborn R., Gaines S. D., Deschenes O., Lester S. E. (2012). Status and solutions for the world’s unassessed fisheries. Science 338, 517–520. doi: 10.1126/science.1223389
Czerwinski I. A., Gutiérrez-Estrada J. C., Hernando-Casal J. A. (2007). Short-term forecasting of halibut CPUE: Linear and non-linear univariate approaches. Fish. Res. 86, 120–128. doi: 10.1016/j.fishres.2007.05.006
Dickey D. A., Fuller W. A. (1979). Distribution of the Estimators for Autoregressive Time Series with a Unit Root. J. American Statistical Association 74 (366a), 427–431. doi: 10.1016/j.fishres.2007.05.006
Fanning L. P., Khan M. W., Kidwai S., Macauley G. J. (2011). Surveys of the offshore fisheries resources of Pakistan-2010. FAO Fish. Aqua. Circ. I, 12–22. Available online at: https://openknowledge.fao.org/handle/20.500.14283/i2279e.
FAO (2018). The state of World Fisheries and Aquaculture 2018 (SOFIA): Meeting the Sustainable Development Goals (Rome: Food and Agriculture Organization), xiii + 210.
FAO (2023). Fisheries and Aquaculture Statistics 2023: Yearbook 2020 (Rome: FAO, Yearbook of fishery and Aquaculture Statistics), 220, ISBN: 978-92-5-138107-6. doi: 10.4060/cc7493en
Froese R., Demirel N., Coro G., Kleisner K. M., Winker H. (2017). Estimating fisheries reference points from catch and resilience. Fish. Fish. 18, 506–526. doi: 10.1111/faf.12190
Froese R., Demirel N., Gianpaolo C., Winker H. (2019). A Simple User Guide for CMSY+ and BSM (CMSY_2019_9f.R): 2019. Available online at: https://oceanrep.geomar.de/33076/ (Accessed June 23, 2021).
Froese R., Pauly D. (Eds.) (2015). FishBase (2015: World Wide Web electronic publication). Available at: www.fishbase.org. accessed at www.fishbase.org in November/December 2015 .
Froese R., Pauly D. (2021). FishBase (Philippines: World Wide Web electronic publication 2021). Fishbase.com.
Froese R., Winker H., Coro G., Demirel N., Tsikliras A. C., Dimarchopoulou D. (2018). A new approach for estimating stock status from length frequency data. ICES J. Mar. Sci. 75, 2004–2015. doi: 10.1093/icesjms/fsy078
Geromont H. F., Butterworth D. S. (2015). Complex assessments or simple management procedures for efficient fisheries management: a comparative study. ICES J. Mar. Sci. 72, 262–274. doi: 10.1093/icesjms/fst232
Haddon M. (2011). Modelling and Quantitative Methods in Fisheries. 2nd ed (New York: Chapman and Hall/CRC). doi: 10.1201/9781439894170
Hilborn R., Amoroso R. O., Anderson C. M., Baum J. K., Branch T. A., Costello C., et al. (2020). Effective fisheries management instrumental in improving fish stock status. Proc. Natl. Acad. Sci. 117, 2218–2224. doi: 10.1073/pnas.1909726116
Holt S. J. (1958). The evaluation of fisheries resources by the dynamic analysis of stocks, and notes on the time factors involved. In Some Problems for Biological Fishery Survey and Techniques for their Solution (a symposium held at Biarritz, France, March 1-10, 1956). ICNAF Spec. Publ. 1, 77–95.
Hu W., Ye G., Lu Z., Du J., Chen M., Chou L. M., et al. (2015). Study on fish life history traits and variation in the Taiwan Strait and its adjacent waters. Acta Oceanol. Sin. 34, 45–54. doi: 10.1007/s13131-015-0625-8
Jackson J. B., Kirby M. X., Berger W. H., Bjorndal K. A., Botsford L. W., Bourque B. J., et al. (2001). Historical overfishing and the recent collapse of coastal ecosystems. Science 293, 629–637. doi: 10.1126/science.1059199
Jones M. C., Cheung W. W. L. (2017). Using fuzzy logic to determine the vulnerability of marine species to climate change. Glob. Change Biol. 2018, 719–731. doi: 10.1111/gcb.13869
Kalhoro M. A., Liu Q., Memon K. H., Chnag M. S., Jatt A. N. (2013). Estimation of maximum sustainable yield of Bombay duck, Harpodan nehersus fishery in Pakistan using the CEDA and ASPIC packages. Pak. J. Zool. 45, 1757–1764.
Kalhoro M. A., Liu Q., Memon K. H., Waryani B., Soomro S. H. (2015a). Maximum sustainable yield of greater lizardfish Saurida tumbil fishery in Pakistan using CEDA and ASPIC packages. Acta Oceanol. Sin. 34, 68–73. doi: 10.1007/s13131-014-0463-0
Kalhoro M. A., Liu Q., Valinassab T., Waryani B., Memon K. H., Abbasi A. R. (2015b). Population dynamics of Greater lizardfish Saurida tumbil from Pakistani waters. Pakistan J. Zool. 47, 47–55. doi: 0030-9923/2015/0004-0921
Kalhoro M. A., Liu Q., Waryani B., Memon K. H., Chang M. S., Zhang K. (2014a). Population dynamics of Japanese threadfin bream Nemipterus japonicas from Pakistani waters. Acta Oceanol. Sin. 33, 1–9. doi: 10.1007/s13131-014-0401-1
Kalhoro M. A., Liu Q., Waryani B., Panhwar S. K., Memon K. H. (2014b). Growth and mortality of Brushtooth lizardfish Saurida undosquamis from Pakistani waters. Pak. J. Zool. 46, 139–151. doi: 0030-9923/2014/0001-0139
Kalhoro M. A., Liu Q., Zhu L., Zhaoyang J., Liang Z. (2024a). Assessing fishing capacity of two tuna fish species using different time-series data in Pakistan, northern arabian sea. Estuar. Coast. Shelf. Sci. 299, 108692. doi: 10.1016/j.ecss.2024.108692
Kalhoro M. A., Tang D., Ye H. J., Morozov E., Liu Q., Memon K. H., et al. (2017). Population dynamics of Randall’s threadfin bream Nemipterus randalli from Pakistani waters, northern Arabian Sea. Indian J. Mar. Sci. 46 (3), 551–561.
Kalhoro M. A., Tang D., Ye H. J., Morozov E., Wang S., Buzdar M. A. (2018). Fishery appraisal of family Portunidae Portunus spp. using different surplus production models from Pakistani waters, northern Arabian Sea. Pak. J. Zool., 50, 135–141. doi: 10.17582/journal.pjz/2018.50.1.135.141
Kalhoro M. A., Zhu L., Kim J. H., Liu X., Liu C., Liang Z. (2024b). Small in scale big in contribution: evaluating the stock status of Indian Mackerel (Rastrelliger kanagurta) fishery using CMSY and LBB approaches from Pakistan, northern Arabian Sea. J. Coast. Conserv. 28, 70. doi: 10.1007/s11852-024-01068-9
Khatun M., Zahangir M. M., Akhter B., Parvej M. R., Liu Q. (2023). Clupeids in the Kaptai reservoir, a blessing or a curse: estimation of fisheries reference points. Heliyon 9, e13818. doi: 10.1016/j.heliyon.2023.e13818
Koutroumanidis T., Iliadis L., Sylaios G. K. (2006). Time-series modeling of fishery landings using ARIMA models and Fuzzy Expected Intervals software. Environ. Model. Softw. 21, 1711–1721. doi: 10.1016/j.envsoft.2005.09.001
Kuiter R. H., Tonozuka T. (2001). Pictorial guide to Indonesian reef fishes. Part 2. Fusiliers - Dragonets, Caesionidae - Callionymidae (Australia: Zoonetics), 304–622.
Liang C., Xian W., Pauly D. (2020). Assessments of 15 exploited fish stocks in Chinese, South Korean and Japanese waters using the CMSY and BSM methods. Front. Mar. Sci. 7. doi: 10.3389/fmars.2020.00623
Mahalingaraya, Santosha R., Kanchan S., Shekhawat R. S., Shashikala C. (2018). Statistical modeling and forecasting of total fish production of India: A time series perspective. Int. J. Curr. Microbiol. Appl. Sci. 7, 1698–1707. doi: 10.20546/ijcmas.2018.703.201
Martell S., Froese R. (2013). A simple method for estimating MSY from catch and resilience. Fish. Fish. 14, 504–514. doi: 10.1111/j.1467-2979.2012.00485.x
Mesnil B., Petitgas (2009). Detection of changes in time-series of indicators using CUSUM control charts. Aquat. Living Resour. 22, 187–192. doi: 10.1051/alr/2008058
Methot R. D., Wetzel C. R. (2013). Stock synthesis: A biological and statistical frame work for fish stock assessment and fishery management. Fish. Res. 142, 86–99. doi: 10.1016/j.fishres.2012.10.012
MFD (2017). Marine fisheries department 2017: Handbook of Fisheries Statistics of Pakistan 1995-2017, Government of Pakistan. Pakistan Publishing House, Karachi, Pakistan.
MFD (2021). Marine fisheries department 2021: Handbook of Fisheries Statistics of Pakistan 2017-2021, Government of Pakistan. Pakistan Publishing House, Karachi, Pakistan.
MSA (2007).Magnuson-stevens fishery conservation and management act 2007: public law 94–265. In: As amended by the Magnuson-Stevens Fishery Conservation and Management Reauthorization Act (P.L. 109-479). Available online at: http://www.nmfs.noaa.gov/msa2005/docs/MSA_amended_msa%20_20070112_FINAL.pdf (Accessed 19 December 2014).
Nadeem A., Kalhoro M. A., Buzdar M. A., Tariq S., Shafi M., Afzaal Z., et al. (2017). ). Growth, mortality and exploitation rate of Sardinella longiceps (Valenciennes 1847) from Pakistani waters based on length frequency distribution data. Indian J. Geo-Mar. Sci. 46, 1693–1703.
Nisar U., Ali R., Mu Y., Sun Y. (2021). Assessing five major exploited tuna species in India (Eastern and western Indian ocean) using the monte carlo method (CMSY) and the bayesian schaefer model (BSM). Sustainability 13, 8868. doi: 10.3390/su13168868
Pakistan Navy (2016). Notification, calculation of Pakistan coastline 2016: Hydrography Department, Navel Headquarters, 11, Liaquat Barrackers, Karachi, Notification, No. HD/0628/11/938/12 April 2016.
Palomares M. L. D., Froese R., Derrick B., Meeuwig J. J., Nöel S. L., Tsui G., et al. (2020). Fishery biomass trends of exploited fish populations in marine ecoregions, climatic zones and ocean basins. Estuarine Coast. Shelf. Sci. 243, 106896. doi: 10.1016/j.ecss.2020.106896
Palomares M. L. D., Froese R., Derrick B., Nöel S. L., Tsui G., Woroniak J., et al. (2018). “A preliminary global assessment of the status of exploited marine fish and invertebrate populations,” in A Report Prepared by the Sea around Us for OCEANA (The University of British Columbia, Vancouver, BC, Canada), 60.
Paul R. K., Das M. K. (2010). Statistical modelling of in land fish production in India. J. Inland Fish. Soc India. 42 (2), 1–7. doi: 10.47780/jifsi.42.2.2010.121301
Pauly D., Zeller D. (2016). Global Atlas of Marine Fisheries: A Critical Appraisal of Catches and Ecosystem Impacts (Washington, D.C: Island Press), xvii + 486.
Pella J. J., Tomlinson P. K. (1969). A generalized stock production model. InterAmerican Trop. Tuna Commun. Bull. 13, 421–458. Available online at: https://books.google.com/books/about/A_Generalized_Stock_Production_Model.html?id=5nToGwAACAAJ.
Pradeep M., Ray S., Tiwari S., Badr A., Balloo R. (2021). Estimation of fish production in India using ARIMA, holt’s linear, BATS and TBATS models. Indian J. Ecol. 48, 1254–1261.
Prista N., Diawara N., Costa M. J., Jones C. (2011). Use of SARIMA models to assess data-poor fisheries: a case study with sciaenid fishery of Portugal. Fish. Bull. 109 (2), 170–182. Available online at: https://digitalcommons.odu.edu/oeas_fac_pubs/40.
Qamar N., Panhwar S. K. (2018b). Assessment of maturity, reproduction and reproductive potentials of torpedo scad, megalaspis cordyla (Linnaeus 1758) from northern arabian sea coast of Pakistan. Russ. J. Mar. Biol. 44, 42–50. doi: 10.1134/S1063074018010078
Qamar N., Panhwar S. K., Brouwer S. (2016). Population characteristics and biological reference point estimates for Two carangid fishes, Megalaspis cordyla and Scomberoides tol, in the northern Arabian Sea coast of Pakistan. Pakistan J. Zool. 48 (3), 869–874. doi: 0030-9923/2016/0003-869
Qamar N., Panhwar S. K., Riede R. (2018a). Feeding patterns of two commercially important fish species Scomberoides commersonnianus and S. tol in the Northern Arabian Sea. Pakistan J. Zool. 50, 941–949. doi: 10.17582/journalpjz/2018.50.3.941.949
Rajani M., Balasubramanian A., Sudhakar O. (2024). Forecasting of fish production of India using ARIMA models. Eco. Env. Cons. 30, S62–S69. doi: 10.53550/EEC.2024.v30i04s.011
Raza H., Liu Q., Alam M. S., Han Y. (2022). Length based stock assessment of five fish species from the marine water of Pakistan. Sustainability 14, 1587. doi: 10.3390/su14031587
Raza H., Liu Q., Hanif M. T., Han Y. (2023). Stock evaluation of the data-limited fisheries: A case study of five major commercially important fishes from the Western Indian Ocean, Pakistan. Pak. J. Zool. 53, 1099–1108. doi: 10.17582/journal.pjz/20211108201136
Razzaq S., Kalhoro M. A., Memon A. M., Buzdar M. A., Shafi M., Buzdar F. (2019). Fishery stock assessment of Megalaspis cordyla by using non equilibrium surplus production models in the Arabian Sea coast of Pakistan. J. Anim. Plant Sci. 29, 132–140.
Ren Q., Liu M. (2020). Assessing northwest pacific fishery stocks using two new methods: The Monte Carlo Catch-MSY (CMSY) and the Bayesian Schaefer Model (BSM). Front. Mar. Sci. 7. doi: 10.3389/fmars.2020.00430
Sathianandan T. V., Srinath M. (1995). Time series analysis of marine fish landings in India. Mar. Biol. Assoc. 37, 171–178. Available online at: http://eprints.cmfri.org.in/id/eprint/646
Scandol J. P. (2003). Use of cumulative sum (CUSUM) control charts of landed catch in the management of fisheries. Fish. Res. 64, 19–36. doi: 10.1016/S0165-7836(03)00104-8
Selvaraja J. J., Arunachalamb V., Coronado-Francoa K. V., Romero-Orjuelab L. V., Ramírez-Yara Y. N. (2020). Time-series modeling of fishery landings in the Colombian Pacific Ocean using an ARIM Amodel. Regional Stud. Mar. Sci. 39, 101477. doi: 10.1016/j.rsma.2020.1014772352
Smith-Vaniz W. F. (1984). “Carangidae,” in Fischer W., Bianchi G. (eds.) FAO species identification sheets for fishery purposes. Western Indian Ocean fishing area 51. Vol. 1. [pag. var.]. FAO, Rome. Fishery Resources and Environment Division FAO Fisheries Department Rome, Italy. Available at: http://www.fao.org/docrep/009/ad468e/ad468e00.HTM.
Smith-Veniz W. F. (2003). “Carangidae,” in The living marine resources of the western central Atlantic, vol. 3 . Ed. Carpenter K. E.FAO species identification guide for fishery purposes (FAO, Rome), 1426–1468.
Sumaila U. R., Marsden A. D., Watson R., Pauly D. (2007). A global ex-vessel fish price database: construction and applications. J. Bioeconom. 9, 39–51. doi: 10.1007/s10818-007-9015-4
UN (2015).United Nations; Accepts Pakistan’s demand for extending sea limit to 350 nautical miles. March 21, 2015. Case submitted by National Institute of Oceanography, Government of Pakistan. MZN (Maritime Zone Notifications) Released on 08/09/2016. Circular Communications from the Division for Ocean Affairs and The Law of the Sea Office of Legal Affairs. Available online at: https://niopk.gov.pk/docs/6.pdf (Accessed March 21, 2015).
Von-Bertalanffy L. (1938). A quantitative theory of organic growth (inquiries on growth laws. ii). Hum. Bio. 10, 181–213.
Wang Y., Wang Y., Liu S., Liang C., Zhang H., Xian W. (2020). Stock assessment using LBB method for eight fish species from the Bohai and yellow seas. Front. Mar. Sci. 7, 164. doi: 10.3389/fmars.2020.00164
Winker H., Carvalho F., Kapur M. (2018). JABBA: just another bayesian biomass assessment. Fish. Res. 204, 275–288. doi: 10.1016/j.fishres.2018.03.010
Worm B., Hilborn R., Baum J. K., Branch T. A., Collie J. S., Costello C., et al. (2009). Rebuilding global fisheries. Science 325, 578–585. doi: 10.1126/science.1173146
Yadav A. K., Kishore K. D., Pronob D., Rohan K. R., Jeetendra K., Basanta K. B. (2020). Growth trends and forecasting of fish production in Assam, India using ARIMA model. J. Appl. Natural Sci. 12, 415–421. doi: 10.31018/jans.v12i3.2353
Yue L., Wang Y., Zhang H., Xian W. (2021). Stock assessment using the LBB method for Portunus trituberculatus collected from the Yangtze Estuary in China. Appl. Sci. 11, 342. doi: 10.3390/app11010342
Zafar M., Mustafa M. G., Haque M. A. (2000). Population dynamics of Megalaspis cordyla (Linneaus 1758) from north-eastern part of the Bay of Bengal, Bangladesh. Indian J. Fish. 47, 163–168. Available online at: https://epubs.icar.org.in/index.php/IJF/article/view/8526.
Zeller D., Palomares M. L. D., Tavakolie A., Ang M., Belhabib D., Cheung W. W. L., et al. (2016). Still catching attention: sea Around Us reconstructed global catch data, their spatial expression and public accessibility. Mar. Pol. 70, 145–152. doi: 10.1016/j.marpol.2016.04.046
Zhang K., Geng P., Li J., Xu Y., Kalhoro M. A., Sun M., et al. (2022a). Influences of fisheries management measures on biological characteristics of Threadfin Bream (Nemipterus virgatus) in the Beibu Gulf, South China Sea. Acta Oceanol. Sin. 41, 24–33. doi: 10.1007/s13131-021-1925-9
Zhang K., Li M., Li J. J., Sun M. S., Xu Y. W., Cai Y. C., et al. (2022b). Climate-induced small pelagic fish blooms in an overexploited marine ecosystem of the South China Sea. Ecol. Indic. 145, 109598. doi: 10.1016/j.ecolind.2022.109598
Zhu L., Ge C., Jiang Z., Liu C., Hou G., Liang Z. (2021). Stock assessment of small yellow croaker (Larimichthys polyactis) off the coast of China using per-recruit analysis based on bayesian inference. Front. Mar. Sci. 8. doi: 10.3389/fmars.2021.652293
Keywords: fisheries management, ARIMA model, CMSY, illegal fishing, northern Arabian Sea
Citation: Kalhoro MA, Sun J, Zhu L, Liang Z, Liu C and Raza H (2025) Assessing the stock status of Megalaspis cordyla in the northern Arabian Sea: a multi-model approach for sustainable fishery management. Front. Mar. Sci. 12:1528586. doi: 10.3389/fmars.2025.1528586
Received: 15 November 2024; Accepted: 17 March 2025;
Published: 02 April 2025.
Edited by:
Morten Omholt Alver, Norwegian University of Science and Technology, NorwayReviewed by:
Brett W. Molony, Oceans and Atmosphere (CSIRO), AustraliaCopyright © 2025 Kalhoro, Sun, Zhu, Liang, Liu and Raza. This is an open-access article distributed under the terms of the Creative Commons Attribution License (CC BY). The use, distribution or reproduction in other forums is permitted, provided the original author(s) and the copyright owner(s) are credited and that the original publication in this journal is cited, in accordance with accepted academic practice. No use, distribution or reproduction is permitted which does not comply with these terms.
*Correspondence: Jing Sun, c3VuamluZ3JzQDEyNi5jb20=
Disclaimer: All claims expressed in this article are solely those of the authors and do not necessarily represent those of their affiliated organizations, or those of the publisher, the editors and the reviewers. Any product that may be evaluated in this article or claim that may be made by its manufacturer is not guaranteed or endorsed by the publisher.
Research integrity at Frontiers
Learn more about the work of our research integrity team to safeguard the quality of each article we publish.