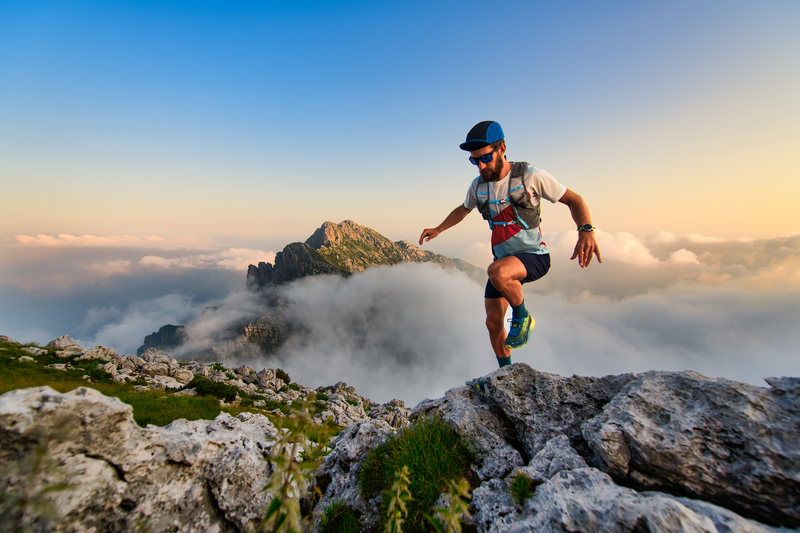
95% of researchers rate our articles as excellent or good
Learn more about the work of our research integrity team to safeguard the quality of each article we publish.
Find out more
ORIGINAL RESEARCH article
Front. Mar. Sci. , 20 March 2025
Sec. Ocean Observation
Volume 12 - 2025 | https://doi.org/10.3389/fmars.2025.1523729
Underwater image restoration confronts three major challenges: color distortion, contrast degradation, and detail blurring caused by light absorption and scattering. Current methods face difficulties in effectively balancing local detail preservation with global information integration. This study proposes SwinCNet, an innovative deep learning architecture that incorporates an enhanced Swin Transformer V2 following primary convolutional layers to achieve synergistic processing of local details and global dependencies. The architecture introduces two novel components: a dual-path feature extraction strategy and an adaptive feature fusion mechanism. These components work in tandem to preserve local structural information while strengthening cross-regional feature correlations during the encoding phase and enable precise multi-scale feature integration during decoding. Experimental results on the EUVP dataset demonstrate that SwinCNet achieves PSNR values of 24.1075 dB and 28.1944 dB on the EUVP-UI and EUVP-UD subsets, respectively. Furthermore, the model demonstrates competitive performance in reference-free evaluation metrics compared to existing methods while processing 512×512 resolution images in merely 30.32 ms—a significant efficiency improvement over conventional approaches, confirming its practical applicability in real-world underwater scenarios.
With the advancement of marine exploration, underwater imaging technology has become increasingly critical for scientific research and resource exploration. However, captured images frequently suffer from quality degradation caused by three primary factors: light attenuation, water scattering, and color distortion. These degradation effects significantly impair image analysis effectiveness, necessitating robust underwater image enhancement techniques to restore color fidelity and structural clarity.
Recent developments in deep learning have revolutionized underwater image restoration. While traditional convolutional neural networks [CNNs (Lecun et al., 1998)] have achieved notable success in image processing tasks, their limited receptive fields constrain their ability to capture long-range dependencies. In contrast, Transformer-based architectures (Vaswani et al., 2017) like the Swin Transformer (Liu et al., 2021) leverage advanced attention mechanisms to effectively model global relationships. This complementary capability motivates our integration of Swin Transformer’s global processing strengths with CNN-based local feature extraction.
The Swin Transformer (Liu et al., 2021) demonstrates superior performance in modeling global dependencies but exhibits limitations in capturing intricate local patterns. Conversely, conventional CNNs excel at local feature extraction but struggle with long-range contextual relationships. These mutually exclusive limitations lead to suboptimal restoration quality when either architecture operates independently. Our proposed SwinCNet framework addresses this fundamental challenge through strategic integration of both architectures, enabling synergistic processing of local details and global context.
SwinCNet implements a novel cascaded structure where enhanced Swin Transformer V2 modules (Liu et al., 2022b) follow primary convolutional layers, enabling progressive refinement from local features to global context.
The architecture develops complementary processing paths-convolutional streams for spatial detail preservation and transformer pathways for long-range dependency modeling.
A hierarchical feature integration framework dynamically balances local and global information across multiple scales through learnable attention weights.
Experimental validation on the EUVP (Islam et al., 2020b) and LSUI (Peng et al., 2023) datasets demonstrates SwinCNet’s superior performance in underwater image restoration. The framework achieves significant improvements in both quantitative metrics and visual quality while maintaining computational efficiency critical for real-world applications.
Early approaches to underwater image enhancement predominantly employed conventional image processing methods. Fundamental techniques including histogram equalization (Zuiderveld, 1994) and white balance adjustment formed the basis of initial solutions. Subsequent developments introduced Retinex-based variational models, such as the Bayesian Retinex framework (Zhuang et al., 2021) and edge-preserving filtering variants (Zhuang and Ding, 2020), which demonstrated improved handling of color distortion and non-uniform illumination. Recent advancements in this area include the Bayesian Retinex approach (Zhuang et al., 2021) and edge-preserving filtering Retinex algorithm (Zhuang and Ding, 2020), further enhancing the robustness of these methods. While effective in controlled scenarios, these methods exhibit limited adaptability to complex underwater conditions characterized by severe scattering and chromatic aberration.
The advent of deep learning has revolutionized underwater image processing. Convolutional Neural Networks (CNNs) have shown remarkable success in tasks ranging from denoising to color correction, leveraging their hierarchical feature extraction capabilities (Islam et al., 2020b; Li et al., 2021; Islam et al., 2020a). However, the intrinsic locality of convolutional operations constrains their ability to model long-range dependencies—a critical requirement for addressing widespread illumination variations and scattering effects in underwater environments.
Inspired by breakthroughs in natural language processing, Vision Transformers have emerged as powerful alternatives for image restoration tasks. The Swin Transformer (Liu et al., 2021), with its hierarchical attention mechanism and shifted window strategy, has demonstrated particular efficacy in capturing global contextual relationships. Recent applications in image super-resolution (Chen et al., 2021) and semantic segmentation (Long et al., 2015) validate its potential, though direct adoption for underwater image restoration remains underexplored due to the domain’s unique challenges.
Recent studies attempt to bridge the complementary strengths of CNNs and Transformers. Notable examples include dual-stream networks for medical imaging (Dai and Gao, 2021) and attention-guided fusion mechanisms in remote sensing (Qingyun et al., 2022). In underwater image processing, proposed a CNN-Transformer cascade for turbidity removal, while developed an adaptive feature mixing module for color correction. These hybrid approaches motivate our investigation into more sophisticated integration strategies that preserve local details while maintaining global coherence.
Parallel developments in marine target detection demonstrate CNN’s versatility in underwater applications. Innovations include dualistic cascade architectures for PolSAR ship detection (Gao et al., 2023), few-shot learning frameworks for SAR classification (Gao et al., 2025b), and multimodal fusion techniques for intelligent target recognition (Gao et al., 2025a). These advancements inform our network design through insights into multi-scale feature processing and domain adaptation.
The proposed SwinCNet model in this study is a deep learning framework designed to address key challenges in underwater image restoration tasks, such as color distortion, reduced contrast, and blurred details. The model employs an innovative architecture that combines Convolutional Neural Networks (CNNs) and Swin Transformer V2 (Liu et al., 2022b) to leverage CNN’s capabilities in feature extraction and Swin Transformer’s advantages in handling long-distance dependencies. The framework diagram of the model we constructed, Figure 1, is shown below.
Figure 1. SwinCNet model. The framework diagram of the model we constructed, Figure 1, is shown below.
The model receives a three-channel RGB underwater image as input and initially processes it through a convolution layer (Conv1). This layer uses 32 filters of size 3x3, with a stride of 1 and padding of 1, followed by batch normalization and ReLU (Nair and Hinton, 2010) activation function. This is aimed at extracting preliminary image features while maintaining the original image dimensions.
In parallel to the primary convolution processing, the model uses Swin Transformer V2 (Liu et al., 2022b) to capture global contextual information from the output of Conv1. Configured to handle 32-channel feature maps, the Swin Transformer (Liu et al., 2021) has an embedding dimension of 96 and a window size of 4x4. This setup allows the model to begin addressing the image’s long-distance dependencies early on, which is crucial for subsequent feature fusion.
The model further extracts features through a series of deep convolution layers (Conv2 to Conv6). Before each convolution layer, 2x2 max pooling (LeCun et al., 1989a) is applied to halve the dimensions of the feature map, while the number of output channels progressively increases across layers: 64, 128, 256, 512, 1024, and 2048. Each layer uses 3x3 filters, with a stride of 1 and padding of 1, and is followed by batch normalization and ReLU (Nair and Hinton, 2010) activation function to enhance the model’s nonlinear representation capabilities and stability.
After deep feature extraction, the feature maps are concatenated with the outputs from Swin Transformer V2 (Liu et al., 2022b), and then progressively restored to their spatial dimensions through a series of upsampling and convolution layers (upconv1 to upconv5). Each upsampling stage is accompanied by a feature fusion with corresponding downsampling layers (using skip connections) to restore image details and local structure. These upsampling layers (Shelhamer et al., 2015) utilize bilinear interpolation for resizing, and the convolution layers adjust the number of channels, ultimately restoring the dimensions back to that of the original input. The bilinear interpolation process can be expressed as follows (see Equation 1):
Here, represents the interpolated pixel values, and are the original image pixels.
Upsampling and Feature Fusion Diagram (see Equation 2):
UpConv represents the upsampling convolution layer. Concat represents feature fusion.
Finally, the model maps the fused feature map back to a three-channel RGB image through two convolution layers (Final Conv0 and Final Conv1). Final Conv0 uses a 1x1 convolution kernel to adjust channel numbers and employs a ReLU (Nair and Hinton, 2010) activation function to enhance nonlinearity. Final Conv1 is a 1x1 convolution operation designed to produce the final image output. The output image is processed through a Tanh (LeCun et al., 1989b) activation function to ensure pixel values are within the [0, 1] range, suitable for image display.
Output Mapping (see Equation 3):
The final mapping ensures pixel values are within the [0, 1] range, making them suitable for display, preserving image integrity and visual quality.
In Figure 2, we perform visualization of feature maps from key layers to gain deeper insights into the network’s internal representations and information flow. By analyzing feature maps from multiple levels within the model, we can reveal the roles each layer plays in image processing and their contributions to the generation of the final output image.
The SwinCNet model integrates Convolutional Neural Networks [CNN (Lecun et al., 1998)] and Swin Transformer V2 (Liu et al., 2022b) to process and enhance underwater images. The model’s design aims to extract local features through convolution operations and capture global contextual information using Swin Transformer V2 (Liu et al., 2022b). The overall architecture includes multiple convolution layers, Swin Transformer modules (Liu et al., 2021), and upsampling and feature fusion layers. Specifically, the model initially processes the input image through an initial convolution layer (conv1) and simultaneously feeds the result into subsequent convolution networks and the Swin Transformer (Liu et al., 2021).
The feature maps from the conv1 layer display basic edge and texture information of the input image, which are low-level features progressively combined and expanded in subsequent layers. Extracting and visualizing conv1 layer feature maps allow observation of how the network initially extracts useful features from raw data.
The feature maps from the conv6 layer show more abstract and complex patterns, such as advanced features of specific shapes or objects. These feature maps reflect the network’s deep understanding of the input image and demonstrate the effectiveness of feature extraction and combination through layers.
The feature maps from the Swin Transformer module (Liu et al., 2021) represent global contextual information. Through its self-attention mechanism, it is evident how the network captures long-range dependencies and fuses them with local features. Visualizing these feature maps helps understand the role of the Transformer in the model and its grasp of global image information.
The feature maps resulting from the concatenation of conv6 and Swin Transformer (Liu et al., 2021) outputs showcase the fusion effects of local and global information. This fusion enhances the model’s overall understanding of the input image and improves the details and accuracy of the final output generated.
The feature maps from the upconv5 layer illustrate how the network uses previously extracted local and global information to reconstruct image details as spatial dimensions are progressively restored. These feature maps provide a visual representation of the model’s mechanism during the image restoration process.
The feature maps from the final output layer display the ultimate synthesis results of all processing stages. After upsampling, feature fusion, and convolution operations, these feature maps provide a high-resolution three-channel output, reflecting the model’s global understanding and reconstruction capabilities of the input image.
To deeply analyze and understand the feature extraction and processing mechanisms at different levels in the SwinCNet model, this study conducted extraction and visualization of feature maps from several key layers.
During the forward propagation, we utilized the PyTorch deep learning framework and registered hooks on each layer of interest. These hooks are used to capture the output activations following each convolution operation, ensuring that feature maps are obtained from various levels of the model. Specifically, for an input sample x, the activations at layer l, where l denotes the layer index, are recorded. The formula is expressed as follows (see Equation 4):
Here, represents the activations from the previous layer, and denotes the operation at layer lll, resulting in a high-dimensional tensor as the extracted feature map. To ensure the consistency of feature map visualization, we performed min-max normalization on each feature map, scaling its values to the range [0,1] for improved visualization display. The normalization is conducted across the spatial dimensions, specifically as follows (see Equation 5):
Here, and represent the minimum and maximum values of the feature map at layer lll, respectively.
In practical applications, we save the feature maps as.npy files for subsequent analysis. After each feature map is converted into a NumPy array, it is saved to disk via a specified path. This method allows us to efficiently manage and analyze feature maps from different layers. The process of saving feature maps is as follows (see Equation 6):
Folders are created for different layers, and feature maps for each layer are saved within these folders. To ensure robustness in processing, the feature map saving process includes clearing the cache of previous feature maps before model prediction, saving output images, and finally saving the feature maps. For feature map visualization, we use tools like Matplotlib to generate two-dimensional heatmaps, which can intuitively display the feature patterns extracted at different levels of the network. The training procedure of the SwinCNet framework for underwater image restoration is detailed in Algorithm 1.
Algorithm 1. Training procedure of the SwinCNet framework for underwater image restoration.
To optimize the performance of the model, we defined a composite loss function that combines three different loss metrics: Mean Squared Error (MSE) (Hastie et al., 2001a), L1 loss (Hastie et al., 2001b), and Structural Similarity Index (SSIM) (Wang et al., 2004). The composite loss function LLL is defined as follows (see Equation 7):
Here, and represent the mean squared error and L1 distance between the predicted image and the target image, respectively, while measures the structural similarity between the two. The parameters α, β, and γ are weights used to adjust the relative importance of the components of the loss function. In our experiments, these parameters are set to 0.3, 0.5, and 0.2 respectively.
The model is trained using the Adam optimizer with an initial learning rate set at 0.001. The reason for choosing Adam is that it combines the benefits of momentum and adaptive learning rate adjustments, which helps in achieving faster convergence in complex loss landscapes.
The model training involves iteratively performing forward and backward propagation on the training dataset. Each batch contains 4 images, and after each iteration, model weights are updated using the backpropagation algorithm to minimize the loss function. The training process is conducted over 100 epochs, with the model’s performance evaluated on an independent validation set after each epoch. Performance is monitored by calculating the composite loss on the validation set, and the best model weights are saved based on the lowest validation loss.
We conducted experiments to evaluate underwater image restoration performance. The LSUI dataset (Peng et al., 2023) was divided into two subsets: LSUI-A (3,979 training images) and LSUI-B (300 test images). Additional validation was performed on two EUVP (Islam et al., 2020b) subsets: EUVP-UI (3,700 images) and EUVP-UD (5,550 images).
Our SwinCNet architecture combines a deep convolutional network with Swin Transformer V2 (Liu et al., 2022b). The model was optimized using the Adam optimizer with a learning rate of 0.001 and a composite loss function combining Mean Squared Error (MSE) (Hastie et al., 2001a), L1 loss (Hastie et al., 2001b), and Structural Similarity Index (SSIM) (Wang et al., 2004). Training lasted 300 epochs, with model weights saved based on minimum validation loss. All images were resized to 256×256 pixels using PyTorch’s transforms module, and experiments were conducted on an NVIDIA GeForce RTX 4070 GPU.
Figure 3 compares SSIM (Wang et al., 2004) and PSNR (Gonzalez, 2009) values across different models. SwinCNet achieves the best performance with SSIM of 0.8767 and PSNR of 25.4054, indicating superior structural preservation and noise suppression. The model demonstrates clearer fish-scale details and rock texture preservation compared to DA-hyper (Zhuang et al., 2022), UWCNN (Anwar et al., 2018), and other baseline methods. Notably, SwinCNet effectively reduces green color bias while avoiding oversaturation issues observed in Dahaze (Wang et al., 2022) and Ucolor (Li et al., 2021).
Figure 4 shows local comparisons with doubled pixel values. Traditional methods [UIR (Huang et al., 2023), MLLE (Zhang et al., 2022)] exhibit amplified noise artifacts, while learning-based approaches [UWCNN (Anwar et al., 2018), Twin-UIE (Liu et al., 2022a)] display color distortion. Although U-Transformer (Peng et al., 2023) maintains low noise levels, it retains residual green hues in background regions. In contrast, SwinCNet successfully removes unwanted green tones while preserving fine details in fish eye structures.
Table 1 compares nine methods across three datasets. SwinCNet consistently outperforms competitors, achieving 28.19 dB PSNR on EUVP-UD and 0.893 SSIM on LSUI-B. The model shows strong generalization capabilities with minimal performance variance across different datasets compared to other methods.
Table 1. Comparison of PSNR and SSIM values across different models on three datasets, with the best in red and the second best in blue.
In this study, we evaluated several mainstream underwater image enhancement models on three different datasets: EUVP-UI, EUVP-UD, and LSUI-B, to assess their effectiveness in underwater image processing applications. These models include traditional methods (such as DCP, MLLE, and DA-hyper) and deep learning-based methods (such as Ucolor, UWCNN, U-Transformer, Twin-UIE, and Semi-UIR). From Table 2, it is evident that our model achieves the best values on the EUVP-UI and EUVP-UD datasets, and it shows the best SSIM and second-best PSNR on the LSUI-B dataset, demonstrating superior performance and strong generalization capabilities. Especially on the EUVP-UD dataset, SwinCNet reaches a PSNR of 28.1944 dB and an SSIM of 0.9538, significantly outperforming other models. This indicates SwinCNet’s clear advantages in maintaining image structure and quality.
Table 2. Comparison of non-reference metrics (UCIQE, UIQM, CCF) across different methods and datasets.
Table 2 presents the comparison of non-reference metrics [UCIQE (Yang and Sowmya, 2015), UIQM (Panetta et al., 2016) and CCF (Drews et al., 2013)] across different models on three datasets. Higher values of UCIQE and UIQM indicate better image quality, while lower CCF values represent better color fidelity. Although SwinCNet shows moderate performance in UCIQE, it achieves impressive results in UIQM, particularly scoring 3.1139 on the EUVP-UI dataset, which is second only to U-Transformer (3.121) and Twin-UIE (3.117). Notably, in terms of color fidelity measured by CCF, SwinCNet demonstrates superior performance across all datasets, achieving optimal results of 0.1699, 0.2015, and 0.1804 on the EUVP-UI, EUVP-UD, and LSUI-B datasets, respectively, significantly outperforming other comparative methods.
The visual comparison analysis in Figure 5 shows that SwinCNet excels in overall color restoration, particularly in the red-boxed regions where it successfully removes blue-green color casts caused by underwater scattering, presenting clear and natural colors. In contrast, while U-Transformerr (Peng et al., 2023), Ucolor (Li et al., 2021), and Twin-UIE (Liu et al., 2022a) show excellent UIQM scores, they retain green residuals in actual image processing and fail to fully correct color casts. Meanwhile, UIR (Huang et al., 2023), DA-hyper (Zhuang et al., 2022), and MLLE (Zhang et al., 2022) introduce excessive contrast in image restoration, affecting detail presentation. UWCNNUWCNN (Anwar et al., 2018) and DCP (He et al., 2009), in pursuit of higher UCIQE scores, result in over-restored colors, causing images to deviate from their natural state.
Figure 6 displays the RGB channel pixel intensity variation curves across different models. In this experiment, we compared the pixel intensity variations in the Red, Green, and Blue (RGB) channels across different underwater image processing methods to assess their performance in color restoration and detail enhancement. The graph shows the pixel intensity variation curves for the input image (Input), the ground truth image (Truth), and the outputs of eight different underwater image algorithms. The X-axis represents pixel positions, ranging from [0, 256], corresponding to the horizontal axis of the image, while the Y-axis represents the pixel intensity values of each channel, ranging from [0, 255].
The comparison between the input image and the ground truth image reveals the challenges of underwater image processing. The RGB channel intensity curves of the input image are close together with overall low intensity, reflecting the typical problems of low contrast and color distortion in underwater images. In contrast, the RGB channel curves of the ground truth image show significant differences, especially with the red channel being noticeably higher than the blue and green channels, displaying the true color distribution characteristics of real-world scenes. This contrast highlights that the goal of underwater image processing methods is to restore color distribution and contrast as close as possible to that of the ground truth image.
Among the results of different algorithms, our proposed method, SwinCNet (Ours), performs exceptionally well. It successfully restores the RGB channel color distribution, particularly in the red channel, where its curve approaches that of the ground truth image, indicating strong capabilities in color restoration. In contrast, methods like U-Transformer and UIR also perform well in restoring the red channel but still show slight differences compared to the ground truth image, suggesting room for improvement in handling certain details. Other methods such as Ucolor and DA-hyper perf.
To evaluate the computational performance and complexity of SwinCNet, we analyzed several key metrics, including FLOPS, the number of model parameters, and runtime across different image sizes. The results are summarized in Tables 3, 4.
Table 3 highlights the floating-point operations (FLOPS) and the total number of parameters for SwinCNet and other comparative models. SwinCNet’s FLOPS reach 207.99 G, which is moderate among the models considered. It is higher than UWCNN (5.68 G) and U-Transformer (26.11 G) but far lower than Ucolor’s 14,025.22 G. This demonstrates that SwinCNet is capable of executing complex image processing tasks without requiring extremely high computational resources.
In terms of model parameters, SwinCNet has 57.24 million parameters, significantly exceeding most comparison models, such as UWCNN (0.04 M) and Semi-UIR (1.68 M). Only U-Transformer (31.6 M) and Twin-UIE (11.4 M) come relatively close. The higher number of parameters indicates that SwinCNet incorporates a more complex network structure, likely with additional layers and intricate connections, which enhances its ability to process and learn from underwater images effectively.4.6.2 Runtime Performance.
Table 4 presents the runtime (in milliseconds) of SwinCNet and other methods for processing single images at various resolutions (128×128, 256×256, 512×512, and 1024×1024). SwinCNet demonstrates competitive runtime performance, particularly on larger images. For instance, SwinCNet requires only 88.2 ms to process a 1024×1024 image, which is significantly faster than traditional methods like DCP (30,819.93 ms) and Ucolor (134,832 ms). Among deep learning methods, SwinCNet’s runtime is also highly competitive, outperforming UWCNN (3,656.88 ms) and U-Transformer (769.36 ms) at the same resolution.
For smaller image sizes, SwinCNet maintains excellent efficiency, requiring only 11.83 ms for 128×128 images and 18.73 ms for 256×256 images. This balance between computational efficiency and restoration quality makes SwinCNet suitable for real-time applications.
The SwinCNet model proposed in this study demonstrates superior performance in underwater image restoration tasks. By integrating Convolutional Neural Networks [CNN (Lecun et al., 1998)] with Swin Transformer V2 (Liu et al., 2022b) architecture, the model effectively addresses typical underwater image degradation issues including color distortion, contrast reduction, and detail blurring. SwinCNet’s innovative feature fusion architecture enhances both local detail preservation and long-range dependency modeling.
Comprehensive evaluations across multiple datasets reveal SwinCNet’s significant advantages in color correction and detail restoration compared to existing methods. The model achieves state-of-the-art performance in key metrics [PSNR (Gonzalez, 2009) and SSIM (Wang et al., 2004)], confirming its exceptional image restoration quality. Furthermore, the carefully balanced computational efficiency and parameter size demonstrate the model’s rational design, making SwinCNet suitable for both high-performance computing environments and resource-constrained applications.
Through rigorous experimental validation, SwinCNet not only improves underwater image visual quality but also enhances their analytical utility. These advancements underscore SwinCNet’s practical value and potential applications in contemporary underwater image processing. The proposed model therefore provides an effective solution for advancing underwater image restoration technology, exemplifying the transformative potential of deep learning in complex image processing challenges.
While achieving promising results, SwinCNet presents certain limitations. The model’s computational complexity, particularly in terms of floating-point operations (FLOPS) and parameter count, may constrain deployment in resource-limited scenarios. Future research directions include model efficiency optimization through pruning and quantization techniques, as well as integration with real-time underwater imaging systems to enhance practical applicability.
The raw data supporting the conclusions of this article will be made available by the authors, without undue reservation.
CY: Writing – review & editing, Methodology, Project administration, Writing – original draft. LS: Writing – review & editing, Funding acquisition, Supervision. YD: Investigation, Writing – review & editing, Software, Validation. JW: Writing – review & editing, Funding acquisition, Validation. HZ: Data curation, Software, Writing – review & editing.
The author(s) declare that no financial support was received for the research and/or publication of this article.
The authors declare that the research was conducted in the absence of any commercial or financial relationships that could be construed as a potential conflict of interest.
The author(s) declare that no Generative AI was used in the creation of this manuscript.
All claims expressed in this article are solely those of the authors and do not necessarily represent those of their affiliated organizations, or those of the publisher, the editors and the reviewers. Any product that may be evaluated in this article, or claim that may be made by its manufacturer, is not guaranteed or endorsed by the publisher.
Anwar S., Li C., Porikli F. (2018). Deep underwater image enhancement. arXiv Preprint arXiv, 1807.03528. Available online at: https://arxiv.org/abs/1807.03528.
Chen H., Wang Y., Guo T., Xu C., Deng Y., Liu Z., et al. (2021). Pre-trained image processing transformer. In Proceedings of the IEEE/CVF Conference on Computer Vision and Pattern Recognition (pp. 12299–12310).
Dai Y., Gao Y. (2021). Transmed: Transformers advance multi-modal medical image classification. 11 (8), 1384. doi: 10.3390/diagnostics11081384
Drews P. Jr, do Nascimento E., Moraes F., Botelho S., Campos M. (2013). “Transmission estimation in underwater single images,” in 2013 IEEE International Conference on Computer Vision Workshops, Sydney, NSW, Australia, 825–830. doi: 10.1109/ICCVW.2013.113
Gao G., Bai Q., Zhang C., Zhang L., Yao L. (2023). Dualistic cascade convolutional neural network dedicated to fully PolSAR image ship detection. ISPRS J. Photogrammetry Remote Sens. 202, 663–681. doi: 10.1016/j.isprsjprs.2023.07.006
Gao G., Wang M., Zhang X., Li G. (2025a). Den: A new method for sar and optical image fusion and intelligent classification. IEEE Trans. Geosci. Remote Sens. 63, 1–18. doi: 10.1109/TGRS.2024.3500036
Gao G., Wang M., Zhou P., Yao L., Zhang X., Li H., et al. (2025b). A multibranch embedding network with bi-classifier for few-shot ship classification of sar images. IEEE Trans. Geosci. Remote Sens. 63, 1–15. doi: 10.1109/TGRS.2024.3500034
Guzmán-Cabrera R., Guzmán-Sepúlveda J. R., Torres-Cisneros M. (2013). Processing Technique for Breast Cancer Detection. Int J Thermophys 34, 1519–1531. doi: 10.1007/s10765-012-1328-4
Hastie T., Friedman J., Tibshirani R. (2001a). The elements of statistical learning. Springer series in statistics (New York, NY: Springer New York). doi: 10.1007/978-0-387-21606-5
Hastie T., Friedman J., Tibshirani R. (2001b). The elements of statistical learning. Springer series in statistics (New York, NY: Springer New York). doi: 10.1007/978-0-387-21606-5
He K., Sun J., Tang X. (2009). “Single image haze removal using dark channel prior,” in 2009 IEEE conference on computer vision and pattern recognition, 1956–1963. doi: 10.1109/CVPR.2009.5206515
Huang S., Wang K., Liu H., Chen J., Li Y. (2023). “Contrastive semi-supervised learning for underwater image restoration via reliable bank,” in Proceedings of the IEEE/CVF conference on computer vision and pattern recognition, (Piscataway, NJ, USA: IEEE) 18145–18155.
Islam M. J., Edge C., Xiao Y., Luo P., Mehtaz M., Morse C., et al. (2020a). “Semantic segmentation of underwater imagery: Dataset and benchmark,” in 2020 IEEE/RSJ international conference on intelligent robots and systems (IROS) (Piscataway, NJ, USA: IEEE), 1769–1776. doi: 10.1109/IROS45743.2020.9340821
Islam M. J., Xia Y., Sattar J. (2020b). Fast underwater image enhancement for improved visual perception. IEEE Robotics Automation Lett. 5, 3227–3234. doi: 10.1109/LRA.2020.2974710
LeCun Y., Boser B., Denker J., Henderson D., Howard R., Hubbard W., et al. (1989a). “Handwritten digit recognition with a back-propagation network,” in Advances in neural information processing systems, vol. 2. (San Francisco, CA, USA: Morgan-Kaufmann).
LeCun Y., Boser B., Denker J. S., Henderson D., Howard R. E., Hubbard W., et al. (1989b). Backpropagation applied to handwritten zip code recognition. Neural Comput. 1, 541–551. doi: 10.1162/neco.1989.1.4.541
Lecun Y., Bottou L., Bengio Y., Haffner P. (1998). Gradient-based learning applied to document recognition. Proc. IEEE 86, 2278–2324. doi: 10.1109/5.726791
Li C., Anwar S., Hou J., Cong R., Guo C., Ren W. (2021). Underwater image enhancement via medium transmission-guided multi-color space embedding. IEEE Trans. Image Process. 30, 4985–5000. doi: 10.1109/TIP.2021.3076367
Liu Z., Hu H., Lin Y., Yao Z., Xie Z., Wei Y., et al. (2022b). “Swin transformer v2: Scaling up capacity and resolution,” in Proceedings of the IEEE/CVF conference on computer vision and pattern recognition (CVPR), (Piscataway, NJ, USA: IEEE) 12009–12019.
Liu R., Jiang Z., Yang S., Fan X. (2022a). Twin adversarial contrastive learning for underwater image enhancement and beyond. IEEE Trans. Image Process. 31, 4922–4936. doi: 10.1109/TIP.2022.3190209
Liu Z., Lin Y., Cao Y., Hu H., Wei Y., Zhang Z., et al. (2021). “Swin transformer: Hierarchical vision transformer using shifted windows,” in Proceedings of the IEEE/CVF international conference on computer vision (ICCV), (Piscataway, NJ, USA: IEEE) 10012–10022.
Long J., Shelhamer E., Darrell T. (2015). Fully convolutional networks for semantic segmentation. In Proceedings of the IEEE Conference on Computer Vision and Pattern Recognition (pp. 3431–3440).
Nair V., Hinton G. E. (2010). “Rectified linear units improve restricted boltzmann machines,” in Proceedings of the 27th international conference on machine learning (ICML-10), (Madison, WI, USA: Omnipress) 807–814.
Panetta K., Gao C., Agaian S. (2016). Human-visual-system-inspired underwater image quality measures. IEEE J. Oceanic Eng. 41, 541–551. doi: 10.1109/JOE.2015.2469915
Peng L., Zhu C., Bian L. (2023). U-shape transformer for underwater image enhancement. IEEE Trans. Image Process. 32, 3066–3079. doi: 10.1109/TIP.2023.3276332
Qingyun F., Dapeng H., Zhaokui W. (2022). Cross-modality fusion transformer for multispectral object detection. arXiv preprint arXiv:2111.00273.
Shelhamer E., Long J., Darrell T. (2015). “Fully Convolutional Networks for Semantic Segmentation,” in IEEE Transactions on Pattern Analysis and Machine Intelligence, 39 (4), 640–651. doi: 10.1109/TPAMI.2016.2572683
Vaswani A., Shazeer N., Parmar N., Uszkoreit J., Jones L., Gomez A. N., et al. (2017). “Attention is all you need,” in Advances in neural information processing systems, vol. 30. (Red Hook, NY, USA: Curran Associates, Inc).
Wang Z., Bovik A. C., Sheikh H. R., Simoncelli E. P. (2004). Image quality assessment: from error visibility to structural similarity. IEEE Trans. image Process. 13, 600–612. doi: 10.1109/TIP.2003.819861
Wang Y., Yan X., Wang F. L., Xie H., Yang W., Wei M., et al. (2022). UCL-dehaze: Towards real-world image dehazing via unsupervised contrastive learning. IEEE Transactions on Image Processing 33, 1361–1374.
Yang M., Sowmya A. (2015). An underwater color image quality evaluation metric. IEEE Trans. Image Process. 24, 6062–6071. doi: 10.1109/TIP.2015.2491020
Zhang W., Zhuang P., Sun H.-H., Li G., Kwong S., Li C. (2022). Underwater image enhancement via minimal color loss and locally adaptive contrast enhancement. IEEE Trans. Image Process. 31, 3997–4010. doi: 10.1109/TIP.2022.3177129
Zhuang P., Ding X. (2020). Underwater image enhancement using an edge-preserving filtering retinex algorithm. Multimedia Tools Appl. 79, 17257–17277. doi: 10.1007/s11042-019-08404-4
Zhuang P., Li C., Wu J. (2021). Bayesian retinex underwater image enhancement. Eng. Appl. Artif. Intell. 101, 104171. doi: 10.1016/j.engappai.2021.104171
Zhuang P., Wu J., Porikli F., Li C. (2022). Underwater image enhancement with hyper-laplacian reflectance priors. IEEE Trans. Image Process. 31, 5442–5455. doi: 10.1109/TIP.2022.3196546
Keywords: Swin Transformer V2, CNN, underwater image restoration, precise color correction, deep learning
Citation: Yang C, Shao L, Deng Y, Wang J and Zhai H (2025) SwinCNet leveraging Swin Transformer V2 and CNN for precise color correction and detail enhancement in underwater image restoration. Front. Mar. Sci. 12:1523729. doi: 10.3389/fmars.2025.1523729
Received: 06 November 2024; Accepted: 20 February 2025;
Published: 20 March 2025.
Edited by:
Zhibin Yu, Ocean University of China, ChinaCopyright © 2025 Yang, Shao, Deng, Wang and Zhai. This is an open-access article distributed under the terms of the Creative Commons Attribution License (CC BY). The use, distribution or reproduction in other forums is permitted, provided the original author(s) and the copyright owner(s) are credited and that the original publication in this journal is cited, in accordance with accepted academic practice. No use, distribution or reproduction is permitted which does not comply with these terms.
*Correspondence: Liwei Shao, c2hhb2x3QGJpdC5lZHUuY24=
†ORCID: Jiahang Wang, orcid.org/0009-0008-2216-0984
Hexiang Zhai, orcid.org/0009-0005-1069-7256
Disclaimer: All claims expressed in this article are solely those of the authors and do not necessarily represent those of their affiliated organizations, or those of the publisher, the editors and the reviewers. Any product that may be evaluated in this article or claim that may be made by its manufacturer is not guaranteed or endorsed by the publisher.
Research integrity at Frontiers
Learn more about the work of our research integrity team to safeguard the quality of each article we publish.