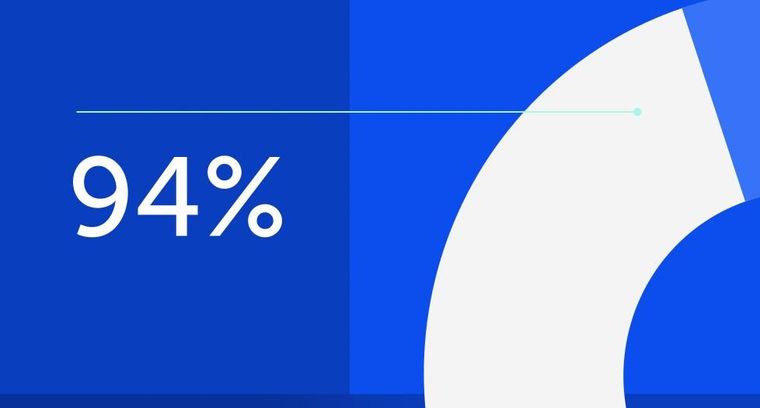
94% of researchers rate our articles as excellent or good
Learn more about the work of our research integrity team to safeguard the quality of each article we publish.
Find out more
ORIGINAL RESEARCH article
Front. Mar. Sci., 11 March 2025
Sec. Ocean Observation
Volume 12 - 2025 | https://doi.org/10.3389/fmars.2025.1522160
Underwater images segmentation is essential for tasks such as underwater exploration, marine environmental monitoring, and resource development. Nevertheless, given the complexity and variability of the underwater environment, improving model accuracy remains a key challenge in underwater image segmentation tasks. To address these issues, this study presents a high-performance semantic segmentation approach for underwater images based on the standard SegFormer model. First, the Mix Transformer backbone in SegFormer is replaced with a Swin Transformer to enhance feature extraction and facilitate efficient acquisition of global context information. Next, the Efficient Multi-scale Attention (EMA) mechanism is introduced in the backbone’s downsampling stages and the decoder to better capture multi-scale features, further improving segmentation accuracy. Furthermore, a Feature Pyramid Network (FPN) structure is incorporated into the decoder to combine feature maps at multiple resolutions, allowing the model to integrate contextual information effectively, enhancing robustness in complex underwater environments. Testing on the SUIM underwater image dataset shows that the proposed model achieves high performance across multiple metrics: mean Intersection over Union (MIoU) of 77.00%, mean Recall (mRecall) of 85.04%, mean Precision (mPrecision) of 89.03%, and mean F1score (mF1score) of 86.63%. Compared to the standard SegFormer, it demonstrates improvements of 3.73% in MIoU, 1.98% in mRecall, 3.38% in mPrecision, and 2.44% in mF1score, with an increase of 9.89M parameters. The results demonstrate that the proposed method achieves superior segmentation accuracy with minimal additional computation, showcasing high performance in underwater image segmentation.
Oceans, often called the “sixth continent”, represent Earth’s largest ecosystem, covering approximately 71% of the planet’s surface. Oceans are rich in resources such as oil, natural gas, sea salt, and marine life, all of which are crucial for sustainable development and play pivotal roles in science, economy, and ecology (Chen et al., 2022). Ocean exploration is essential for the sustainable development of marine resources and advancing the marine sciences. However, the complexities and unknown hazards of the underwater environment significantly limit human exploration, resulting in a heavy reliance on Autonomous Underwater Vehicles (AUVs). Equipped with a range of sensors and visual systems, AUVs autonomously capture underwater images and data, assisting humans in conducting underwater exploration tasks (Bogue, 2015).
Image segmentation is essential for AUV underwater exploration, as it precisely distinguishes objects and regions in images, enabling AUVs to more effectively identify marine life, seabed features, and man-made objects (such as shipwrecks and pipelines), thereby supporting underwater exploration, resource development, environmental protection, and military applications (Abdullah et al., 2023). Nonetheless, underwater image segmentation faces numerous challenges. Unlike land images, underwater images often suffer from lower quality due to factors such as scattering of light in seawater, uneven lighting, color distortion, and water turbidity (Islam et al., 2020b). Additionally, current underwater image datasets are limited in scope, often containing few classes, such as fish and background, resulting in a scarcity of multi-class underwater image datasets. These factors cause traditional image segmentation methods to perform poorly in underwater scenes, with insufficient accuracy and stability to meet real-world demands. Consequently, improving image segmentation performance in underwater environments has become a crucial research focus in both academia and industry.
In recent years, the rapid growth of deep learning has accelerated the application of image segmentation across diverse domains, fostering the development of foundational models. In 2015, Shelhamer et al. introduced the Fully Convolutional Network (FCN) (Long et al., 2015), originally leveraging the VGG model (Simonyan and Zisserman, 2014) as a backbone, with later iterations incorporating other convolutional networks such as ResNet (He et al., 2016). This model facilitated end-to-end training by supporting pixel-level classification, significantly advancing semantic image segmentation. Subsequently, Chen et al. developed the DeepLab series (v1, v2, v3) (Chen et al., 2014, 2017a, 2017b), achieving substantial progress in segmentation accuracy by employing techniques such as dilated convolution (Yu and Koltun, 2015) and Conditional Random Fields. However, these models have some limitations, such as the fixed receptive field of FCN and the high computational cost of the DeepLab series restrict their generalization capabilities. U-Net (Ronneberger et al., 2015), a classic encoder-decoder structured model, uses skip connections to enhance segmentation performance, and is widely applied in areas like image analysis. However, U-Net’s performance in underwater image segmentation remains suboptimal, particularly in its limited feature extraction ability within complex backgrounds. Subsequent models, such as SegNet (Badrinarayanan et al., 2017) and DeepLabv3+ (Chen et al., 2018), improved performance in specific applications but still faced limitations in receptive field size, which can lead to neglect of important features and affect overall performance. In recent years, the proposal of the Transformer (Vaswani et al., 2017) model has injected new vitality into image segmentation techniques. In 2020, Dosovitskiy et al. introduced the Vision Transformer (Dosovitskiy et al., 2020), which divides images into patches, divides images into patches. In 2021, Liu et al. proposed the Swin Transformer model (Liu et al., 2021), specifically designed for computer vision tasks. By leveraging shifted windows and self-attention, it addressed the unique challenges of applying Transformers to vision tasks, making it a general backbone model, especially successful in image classification and object detection. SegFormer (Xie et al., 2021) represents a new generation of Transformer-based segmentation models, striking an effective balance between accuracy and efficiency with its Mix Transformer backbone and lightweight decoder. However, there is still room for improvement in segmentation performance for underwater tasks due to challenges posed by complex environments and image quality issues.
With the advancement of underwater target research and the rapid progress of deep learning across various fields, underwater image segmentation has increasingly garnered widespread attention (Ma et al., 2021; Wang et al., 2022). In recent years, numerous researchers have proposed semantic segmentation models and image enhancement methods tailored for underwater environments. For instance, Wang et al. developed a discriminative underwater image enhancement method empowered by large foundation model technology (Wang et al., 2025), which represents the pioneering application of large foundation model technology to empower underwater image enhancement. Khekare et al. used a machine learning technique that combines histogram equalization and manual white balance for underwater image enhancement (Khekare et al., 2024). Islam et al. noted that underwater images contain visual content significantly different from land images, and that, at the time, publicly available underwater datasets for training and evaluating semantic segmentation models were scarce. Consequently, they manually annotated and released the SUIM dataset for multi-class underwater semantic segmentation, and proposed two versions of the SUIM-Net model (Islam et al., 2020a). Based on the SUIM dataset, their model demonstrated effective semantic segmentation performance, but it lacked in structural optimization. The first version of SUIM-Net exhibited limited feature representation in complex scenes, which could lead to accuracy decline. Although the second version adopted VGG-16 for feature extraction, its computational cost increased significantly with high-resolution images, leading to slower inference speeds. Zhang et al. proposed the WaterBiSeg-Net model (Zhang et al., 2024) to improve the performance of the marine debris segmentation tasks by introducing a multi-scale information enhancement module and a boundary information extraction method, but the model is poorly stabilized in scenarios with high background interference. Liu et al. improved the segmentation accuracy of underwater images by introducing an unsupervised color correction module into the DeepLab v3+ model, but the method is deficient in feature fusion, which makes it difficult to make full use of multi-scale features (Liu and Fang, 2020). Particularly when dealing with irregularly shaped or low-contrast targets, the segmentation results remain suboptimal. Kim et al. enhanced the localization function by adding an attention mechanism to a parallel semantic segmentation network (Kim and Park, 2022), which improved the performance of the model, but still lacked deep structural optimization, resulting in limited effectiveness when dealing with highly diverse and complex environments.
In conclusion, the ocean plays an essential role in human society, and deep exploration of the ocean remains a significant objective for the future. Underwater image segmentation is vital for exploring underwater environments and targets, motivating researchers to investigate effective segmentation methods that advance national marine exploration efforts. While current image segmentation models achieve good results in various scenarios on land, they encounter multiple challenges in underwater environments. This research focuses on the inadequacy of feature extraction capabilities in existing underwater segmentation models when dealing with complex backgrounds and multi-scale targets (Kerai and Khekare, 2024), which fail to effectively capture the details and contextual semantic information of the targets. To address these challenges, this study has implemented targeted improvements on the standard SegFormer model. In particular, uneven lighting, complex shapes of targets, and background disturbances in underwater environments often make it difficult for existing models to extract complete and distinguishable features, and the absence of contextual information also restricts the segmentation accuracy of the models. Therefore, we introduce a more powerful feature extraction backbone network and attention mechanisms (Fu et al., 2018; Li et al., 2018; Zhong et al., 2019) to better capture multi-scale information and detailed features in complex environments, and by optimizing the model’s feature fusion strategy, we enable effective dissemination and integration of contextual information across different scales, thereby improving the model’s segmentation performance in intricate underwater scenes. The segmentation performance of this model has been enhanced compared to the baseline model. The primary contributions of this paper are:
1. Replacing the Mix Transformer backbone network in SegFormer with the Swin Transformer further enhances the model’s feature extraction capabilities in complex environments.
2. An efficient multi-scale attention (EMA) mechanism (Ouyang et al., 2023) is incorporated into the downsampling phase of the backbone network and the decoder to more effectively capture multi-scale features, thereby enhancing the model’s segmentation accuracy.
3. The model structure is optimized by implementing a feature pyramid network (FPN) (Lin et al., 2016) in the decoder, which improves the model’s accuracy and robustness in processing complex scenes through multi-level feature fusion.
The remainder of this paper is organized as follows. Section 2 discusses the methods proposed in this study, Section 3 presents and analyzes the experimental results, and Section 4 summarizes the research findings.
The dataset utilized in this paper is sourced from the publicly available underwater image dataset SUIM, accessible on the IRVLab laboratory’s official website (https://irvlab.cs.umn.edu/resources/suim-dataset). This dataset was meticulously gathered during marine exploration and human-robot cooperation experiments, comprising 1,635 pixel-level annotated images across eight categories: fish (vertebrates), coral reefs (invertebrates), aquatic plants, shipwrecks (ruins), human divers, robots, and the seafloor. This encompasses not only the primary objectives of underwater exploration and measurement (Bingham et al., 2010; Shkurti et al., 2012; Girdhar et al., 2014), but also other underwater entities. The SUIM dataset is the first large-scale multi-class dataset dedicated to underwater image semantic segmentation, featuring natural images and authentic semantic labels, as illustrated in Figure 1. In the experiments, based on the official division of the SUIM dataset, 1,525 images were used for training and 110 images for validation and testing. Given the varied image collection methods and inconsistent sizes within the dataset, we standardized the images by resizing them to pixels to enhance the model’s training efficiency. Furthermore, to improve data diversity during the experiments, we implemented five data augmentation techniques, which included random resizing, random cropping, random alterations in lighting and color, random rotations, and random horizontal flips. These strategies significantly enhance data diversity and help mitigate the model’s overfitting to specific sample characteristics.
Figure 1. SUIM dataset of natural underwater images and real semantic labels, and corresponding color tables for each class.
In this section, we will outline the overall network architecture of the model introduced in this research. In designing any model, it is crucial to consider both its performance and computational efficiency. The standard SegFormer model strikes a good balance in this aspect, as its authors have avoided the complex designs of many traditional methods, and fully considering the efficiency of the model. Nevertheless, this simplified structure compromises performance to some extent. Consequently, this study introduces the Swin Transformer, EMA attention mechanism, and FPN structure on the basis of the standard SegFormer model to further improve the segmentation capability of the model, while ensuring that the computational efficiency of the model does not significantly decline. Compared to the standard SegFormer model, the parameter count has increased slightly by 9.89M, reaching 91.86M. The complete architecture of the model is illustrated in Figure 2.
The model comprises a Swin Transformer encoder and a SegFormer decoder. The input image is initially divided into non-overlapping patches by the Patch Partition module, and then it proceeds through four stages of the backbone network for feature extraction. In Stage 1, there are Linear Embedding module, Swin Transformer Blocks module, and EMA module. As the hierarchy progresses, stages 2, 3, and 4 utilize Patch Merging instead of Linear Embedding, to acquire feature maps at various scales through the iterative processes of patch merging and feature transformation.
The features obtained from the encoder are then transmitted to the decoder. The standard SegFormer model is outfitted with a lightweight multilayer perceptron (MLP) decoder (Tolstikhin et al., 2021), which aggregates feature maps from various layers of the encoder, integrating local and global semantic information to deliver robust performance. However, this feature aggregation approach is somewhat limited and does not fully leverage the information available in the feature maps. Consequently, we incorporated the FPN structure and the EMA attention mechanism. The FPN structure facilitates gradual feature fusion via successive upsampling and lateral connections, whereas the EMA attention mechanism performs cross-spatial learning through parallel substructures to utilize more contextual information between features. The integration of these two modules allows for the comprehensive fusion of feature maps across various levels, thereby further improving the segmentation performance of the model.
In this research, the backbone network primarily comprises the Patch Partition, Linear Embedding, Swin Transformer Blocks, EMA module, and Patch Merging, for effective feature extraction of input images. Firstly, the 3-dimensional RGB input image is divided into non-overlapping patches through the Patch Partition module, with a patch size of used in this study. Each patch is then flattened and mapped to a feature space of C dimension via a linear embedding layer. Next, the image undergoes feature extraction using Swin Transformer Blocks. The image resolution is gradually reduced while ensuring computational efficiency, in the first stage, it is reduced to . Following the blocks, we add an EMA module to enhance the extracted feature representations at each layer. The output feature map size at the first stage is . As the network deepens, the Patch Merging module reduces the patch count by merging adjacent patch features, followed by Swin Transformer Blocks and EMA module to perform feature transformations. The image resolution is downsampled to , with the feature dimension expanding to , resulting in a feature map size of at the second stage output. This process repeats in the subsequent stages twice, producing output feature map sizes of and , respectively, ultimately generating multi-level feature maps across various scales that offer rich multi-scale features to the decoder.
The key mechanism of the Swin Transformer Block is based on shifted windows self-attention, replacing the multi-head self-attention (MSA) module in the standard Transformer Block with a shifted windows module. This design incorporates Window Multi-head Self-Attention (W-MSA) and Shifted Window Multi-head Self-Attention (SW-MSA) to address the limitations of the standard Transformer Block in dense prediction tasks and high-resolution visual tasks. W-MSA computes self-attention within non-overlapping windows, uniformly dividing the image, however, its modeling capacity is limited due to a lack of inter-window connectivity. Consequently, the introduction of SW-MSA enables information exchange across windows, thereby enhancing the model’s capacity to capture relationships. W-MSA and SW-MSA are alternated within successive Swin Transformer Blocks, as illustrated in Figure 3, comprising two Swin Transformer Blocks. The input feature is first processed through a LayerNorm layer, then passed through a (S)W-MSA module, with the resulting output added to via residual connection to yield the intermediate feature . Subsequently, the intermediate feature is processed through an LayerNorm layer and an MLP layer, with the final output added to the intermediate feature via residual connection, resulting in the output feature . This design maintains feature stability and information flow, markedly improving the model’s perception of multi-scale features, thereby boosting visual task performance.
The EMA module employs a parallel processing strategy, enabling the network to avoid the constraints of sequential processing and reducing the need for greater network depth; its overall structure is illustrated in Figure 4. For the input feature map, the module divides its channel dimension into G sub-feature groups. Subsequently, the module employs three parallel paths to extract attention weight descriptors from the grouped feature maps, with two paths featuring a convolution branch and one featuring a convolution branch. In the convolution branch, two global average pooling operations encode channels along both spatial directions, after which the two channel-level attention mappings are concatenated. In the convolution branch, a convolution operation is used to capture feature representations. For the output of the convolution branch, the EMA module employs two non-linear Sigmoid functions to fit the 2D distribution over the linear convolution. The cross-space learning component encodes global spatial information from the convolution branch output through 2D global average pooling, aligning the output with the target dimensions. A softmax function then approximates a linear transformation over the 2D global average pooling output with a 2D Gaussian mapping. The parallel processing output is multiplied by the matrix dot-product operation, producing the first spatial attention map. Additionally, the same procedure is applied to the output from the convolution branch, resulting in a second spatial attention map that preserves precise spatial location information. Ultimately, the two spatial attention maps are combined, and the final output is produced through a Sigmoid function.
The EMA module divides the channel dimension into several sub-features, encoding global information to calibrate channel weights across branches, effectively learning multi-scale spatial semantics and strengthening feature capture capability. In this study, the EMA module is integrated into the downsampling stages of the backbone network and decoder to enhance model’s segmentation performance.
In semantic segmentation, the integration of contextual semantic information is crucial. In semantic information processing, low-level features primarily consist of details such as edges, while high-level features offer an expression of the overall structure of the image. The SegFormer decoder uses a simple MLP layer for feature fusion during the integration process, which leads to inadequate feature merging and may affect the model’s overall segmentation performance. Thus, this study introduces the FPN structure to enhance feature fusion.
FPN is a top-down structure built on feature image pyramids that includes lateral connections, used to generate semantic feature maps at all scales, as shown in Figure 5. The bottom-up pathway of the backbone network extracts features from the image, generating feature maps at different resolutions: , , and . Each feature map is adjusted to the channel dimension of C via a convolution, standardizing channels for effective merging. Next, the top-down path of the structure upsamples the low-resolution but semantically rich feature maps by a factor of two, merging them with the adjusted channel dimension higher-resolution feature maps through lateral connections. By repeating this process, we obtain merged maps for each layer. To reduce the aliasing effects that may occur during upsampling, the feature maps after fusion at each layer are processed through a convolution, resulting in the final feature maps with dimensions of , , and , uniformly denoted as , where i denotes the ith stage of the backbone network. The feature maps outputted at each layer integrate both high-level and low-level features of the image, providing richer semantic and spatial information, thereby effectively improving the segmentation performance of the network.
The decoder also features a lightweight MLP structure, whose purpose is to merge multi-scale features to generate the final semantic segmentation map. The design of the decoder circumvents complex and computationally heavy modules, using simple UpSample layers and MLP layers to accomplish feature fusion and prediction. Firstly, the feature maps at multiple scales processed by the FPN module already possess the same channel dimension. After going through the EMA module, the feature maps with unified channel numbers are upsampled to a common resolution and concatenated, aligning them spatially to enable effective fusion. Next, a single MLP layer processes the merged feature maps to further combine features from different scales, and ultimately, another MLP layer converts the fused feature maps into the final semantic segmentation map.
In this research, we employ the cross-entropy loss function (Zhang and Sabuncu, 2018), widely used in classification tasks to measure the disparity between the predicted distribution and the true distribution, assessing the degree of correspondence between the model’s output probability distribution and the actual labels. Specifically, let the true probability distribution be and the predicted probability distribution be . The loss function is computed using the Equation 1:
Transfer learning is a technique in machine learning designed to apply knowledge gained from one task to another related task, particularly in scenarios where data for the target task is scarce. The fundamental concept is that features learned by a model in one domain can aid in learning in other similar domains, thus minimizing the requirement for extensive data and training time. In this research, given the limited size of the underwater image dataset, transfer learning was employed to enhance the model’s training performance. We initially pre-trained the Swin Transformer model on the ADE20K (Zhou et al., 2017) dataset, then fine-tuned the model with the weights obtained from the pre-trained model and applied it to the underwater image dataset. This approach enabled the model to achieve improved performance by utilizing it as the backbone network for feature extraction in this study.
In this research, to ensure the objectivity and reliability of the experimental results, all experiments were carried out under consistent conditions. The experiments were conducted on Ubuntu 20.04, utilizing an Intel(R) Xeon(R) Platinum 8352V CPU @ 2.10GHz, equipped with an NVIDIA GTX 4090 GPU and 24GB of VRAM. The programming language employed was Python 3.8.10, and the deep learning framework used was PyTorch 1.11.0 along with CUDA 11.3. The experimental training batch size was configured to be 24, with the number of epochs set at 400. The optimizer employed was the weight-decay adaptive moment estimation (Kingma and Ba, 2014), with an initial learning rate of 0.00006 and a learning rate decay factor of 0.01.
In this study, to fully assess the segmentation performance of the network, commonly used evaluation metrics in image segmentation were utilized, including Mean Intersection over Union (MIoU), Recall, Precision, and F1score, which are computed as shown in Equations 2–5:
In this context, True Positive (TP) refers to the positive samples correctly predicted by the model, True Negative (TN) refers to the negative samples correctly predicted by the model, False Positive (FP) indicates the negative samples incorrectly predicted as positive, and False Negative (FN) refers to the positive samples incorrectly predicted as negative. MIoU is a widely used metric in semantic segmentation that computes the average intersection over union for each class in the dataset to assess the accuracy of the model when segmenting pixels of different categories in the image. A higher MIoU value signifies superior model performance in differentiating between various objects. Recall is defined as the ratio of actual positive samples that the model correctly identifies as positive, and it serves as a crucial metric for assessing classification models. Precision refers to the ratio of correctly predicted positive samples by the model that are truly positive; higher precision indicates greater accuracy in predicting positive samples, thus showcasing better model performance. However, within the performance metrics, higher Recall suggests that the model can detect more positive samples, but it may lead to decrease in Precision, resulting in more negative samples being misclassified as positive. Conversely, higher Precision may cause decline in Recall, meaning the model may be too conservative, only predicting those positive samples it is highly confident about, thereby potentially overlooking some actual positives. Consequently, the F1score is introduced as a holistic evaluation metric that considers both Precision and Recall, acting as a balanced indicator for both.
In the experiments, we conducted ablation studies to evaluate the impact of various modules on the performance of the network. Starting with the standard SegFormer model, referred to as Model1, we incrementally introduced different modules for the ablation experiments, naming them Model2, Model3, and Model4. The results of the experiments are presented in Table 1. The “√” in the table signifies that the module was included. As shown in Table 1, Model1 attained an MIoU of 73.27%, mRecall of 83.06%, mPrecision of 85.65%, and mF1score of 84.19% on the SUIM underwater image dataset. Model2 initially incorporated the Swin Transformer to replace the original backbone network, thereby enhancing the model’s feature extraction capability. On the SUIM dataset, it recorded an MIoU of 75.27%, mRecall of 84.85%, mPrecision of 86.51%, and an mF1score of 85.54%. Compared to Model1, despite an increase of 5.53M in parameter count, the MIoU, mRecall, mPrecision, and mF1score rose by 2.0%, 1.79%, 0.86%, and 1.35%, respectively. Subsequently, Model3 incorporated the EMA mechanism into the down-sampling phase and the decoder to capture multi-scale features. Building on Model2, it improved the MIoU by 0.93%, the mRecall by 0.35%, the mPrecision by 0.88%, and the mF1score by 0.63%, with only 0.23M increase in parameters, achieving an MIoU of 76.20%, mRecall of 85.20%, mPrecision of 87.39%, and an mF1score of 86.17% on the SUIM dataset. Lastly, Model4 added the FPN structure to the decoder, merging multi-level features. In the end, Model4 attained an MIoU of 77.00%, mRecall of 85.04%, mPrecision of 89.03%, and an mF1score of 86.63%. Compared to Model3, the MIoU rose by 0.80%, mRecall dropped by 0.16%, mPrecision increased by 1.64%, and the mF1score improved by 0.46%, with a 4.13M increase in parameters. Finally, compared to Model1, Model4, which integrates the three modules, improved the MIoU by 3.73%, increased mRecall by 1.98%, mPrecision by 3.38%, and mF1score by 2.44%, with a slight increase in parameters of 9.89M, FLOPs increased by 14.07G.
We adopt Swin Transformer to replace the original MixTransformer backbone network, which significantly improves the feature extraction capability of the model.Swin Transformer adopts a hierarchical local-global feature modeling approach, which enables the model to be more fine-grained in capturing the detailed information in complex environments, especially for the challenges such as blurring and illumination unevenness and scale changes in underwater images. challenges such as blurring, uneven illumination and scale changes, Swin Transformer demonstrates a strong feature representation capability. The EMA attention mechanism aims to enhance the model’s ability to capture multi-scale features. In the underwater image segmentation task, due to the large scale difference of target objects, the EMA mechanism can better retain the feature information of different scales, thus effectively improving the segmentation accuracy of small objects and long-distance targets. The FPN structure is introduced into the decoder to enhance the segmentation performance of the model through multi-level feature fusion. The FPN structure can effectively integrate the features of different scales, so that the contextual information is better fused between different levels. Especially for images with complex backgrounds, FPN can effectively improve the model’s ability to capture details and further enhance the segmentation accuracy. The experimental results indicate that this study successfully improved the standard SegFormer model by introducing a more powerful feature extraction backbone network, the Swin Transformer, and the EMA attention mechanism. This effectively captured multi-scale information and detail features in complex environments. Additionally, by optimizing the model’s feature fusion strategy and introducing the FPN structure, contextual information was effectively integrated across different scales, in the current environment of abundant computational resources, ensuring model efficiency while significantly enhancing its semantic segmentation performance.
To thoroughly assess the segmentation performance of the model developed in this study, we further conducted comparative experiments in the same experimental environment with existing popular models, such as UNet, FCN, DeepLabv3+, ISANet (Huang et al., 2019), PSPNet (Zhao et al., 2016), KNet (Zhang et al., 2021), MaskFormer (Cheng et al., 2021), Mask2Former (Cheng et al., 2022), UperNet (Xiao et al., 2018) and SegFormer. The results of these comparative experiments are presented in Table 2. As shown in Table 2, for the SUIM underwater image dataset, the model developed in this study achieved an MIoU of 77.00%, mRecall of 85.04%, mPrecision of 89.03%, and an mF1score of 86.63%, which surpasses other models across these evaluation metrics. Compared to PSPNet, the number of parameters of this model has risen, but MIoU, mRecall, mPrecision, and mFscore have improved by 7.69%, 7.18%, 2.71%, and 7.58%, respectively, and FLOPs have decreased by 11.56G, which proves that the computational efficiency of this model is better. Compared to the classical model KNet, the number of parameters rises a bit, but MIoU improves by 6.46%, mRecall by 5.75%, mPrecision by 2.99%, mFscore by 5.31%, and FLOPs by 11.32G. Comparing Maskformer and Mask2former, our model’s evaluation metrics are all better than their evaluation metrics, representing that our model has good segmentation performance and computational efficiency. In comparison to UperNet, which demonstrates the best segmentation performance among other models, our model achieved increases of 4.3% in MIoU, 2.84% in mRecall, 2.88% in mPrecision, and 2.86% in mF1score, that reduces 31.02M and 17.97G in the number of parameters and FLOPs, respectively. Compared to the standard SegFormer model, our model showed improvements of 3.73% in MIoU, 1.98% in mRecall, 3.38% in mPrecision, and 2.44% in mF1score, the number of parameters increased by 9.89M and FLOPs increased by 14.07G, which ensures a certain efficiency of the model and at the same time makes its semantic segmentation performance well improved. The analysis of the above results indicates that our model has better evaluation metrics compared to other popular models in the underwater image segmentation task, which reflects its superior segmentation performance.
To visually compare the model proposed in this study with existing popular models, we created a graph depicting the MIoU metrics for all models used during the training process on the validation set, as illustrated in Figure 6. As observed in Figure 6, with an increase in the number of training epochs, the training performance of each model gradually converges. The model proposed in this research clearly outperformed other models in terms of MIoU, showcasing superior segmentation performance.
Furthermore, this study validated the model using test images by selecting four images that encompass all categories in the dataset, with performance evaluations conducted on each model, as illustrated in Figure 7. UNet, as a classic image segmentation model, performs poorly in the segmentation task of underwater multi-classified objects, and when multiple objects rely on each other, the recognition of UNet produces incorrect predictions, which proves that the model needs better multi-scale feature extraction and fusion capabilities in the complex underwater environment. The performance of FCN, DeeplabV3+, ISANet and PSPNet is closer to each other overall, but there are problems in many details that cannot be segmented accurately, such as divers, water plants and some other objects close to the background color, which also indirectly proves that these models have deficiencies in detail feature extraction capabilities. performance is closer, but there are problems in many details that cannot be segmented accurately, such as divers, water plants and some other objects that are close to the background color, which also indirectly proves that these models have deficiencies in the detailed feature extraction ability of the objects, resulting in the poor performance of the models. KNet, Maskformer, Mask2former and standard SegFormer have the same problem in the water plant segmentation suffer from the same problem of incorrect segmentation recognition when there are different classes of objects with close color proximity, but their performance on fish segmentation is much improved. UperNet and our model perform close to each other on water grass and fish segmentation, but our model performs better on details such as both small tentacles and small object boundaries of divers and fish. Our model better captures multi-scale information and detailed features in complex environments, so that contextual information can be effectively integrated between different scales, and successfully solves the problems of insufficient feature extraction ability and inadequate integration of contextual semantic information in complex backgrounds, which results in a better performance of the model.
The results indicate that the model developed in this study excelled in segmenting categories such as aquatic vegetation and fish, with its segmentation results being closer to the actual labels, in terms of model inference speed, excluding the standard SegFormer model, the FLOPs of all the remaining models are higher than our model, and their performance is still lower than our model, thus, both in terms of performance and inference speed, our model shows very good segmentation performance. In conclusion, taking into account Table 2, Figures 6, 7, and the result analysis, the model proposed in this study attained commendable metrics in the underwater image segmentation task, showcasing strong segmentation performance.
In Figure 7, it can be found that our model has incorrect prediction in the segmentation of very small categories, and will incorrectly predict small fishes, small aquatic grasses, and tiny branches of submersibles, etc. into other categories, the main reason is that when the small objects are highly overlapped with other similarly-colored objects, due to the lack of underwater light and other reasons, the model’s ability to segment small objects or long-distance targets will be fully tested, and there is still room for improvement in the segmentation accuracy of small objects and long-distance targets. There is still room for improvement in the segmentation accuracy of small objects and long-distance targets. In the future research, we will study the better attention mechanism and feature fusion strategy to enhance the segmentation performance of the model. We also need to consider excellent image preprocessing methods to enhance the visual quality of the image and help the model extract features better, so as to overcome the image quality problems caused by the complex underwater environment and further improve the segmentation performance of the model.
This study introduces an enhanced underwater image semantic segmentation model based on the SegFormer framework, capable of effectively and accurately segmenting underwater targets while achieving satisfactory segmentation performance. Initially, we substituted the backbone of the standard SegFormer model with the Swin Transformer to improve the model’s ability to extract features, enabling it to more effectively acquire the semantic information of the images. Additionally, we incorporated the EMA mechanism in both the downsampling stage and the decoder to more effectively capture multi-scale features, thereby improving segmentation precision. Lastly, we integrated the FPN architecture into the decoder to merge multi-level feature maps, thereby improving the model’s accuracy and robustness in complex environments. This model demonstrates a significant enhancement in segmentation performance while preserving computational efficiency. The experimental results show that this model attained MIoU of 77.00%, mRecall of 85.04%, mPrecision of 89.03%, and mF1score of 86.63% on the SUIM underwater image dataset. In comparison to the UperNet, which is the top-performing model among other popular models, our model demonstrated improvements of 4.3%, 2.84%, 2.88%, and 2.86% in MIoU, mRecall, mPrecision, and mF1score, respectively. When compared to the standard SegFormer model, our model achieved 3.73% increase in MIoU, 1.98% increase in mRecall, 3.38% increase in mPrecision, and 2.44% increase in mF1score, with only a modest rise of 9.89M in parameters. Overall, the model developed in this research demonstrates outstanding segmentation performance, effectively resolving the challenges of insufficient feature extraction and inadequate fusion of contextual semantic information in complex backgrounds.
Historically, underwater image semantic segmentation models have primarily focused on improving either feature extraction ability or feature fusion capability, without successfully integrating both approaches. This research enhances the baseline model by integrating both approaches and introducing attention mechanisms along with feature pyramid networks, which more effectively capture multi-scale information and detailed features in complex environments while efficiently acquiring global information from images, leading to strong performance in underwater image segmentation tasks. The experimental findings indicate that the model proposed in this study achieved the highest MIoU on the SUIM dataset, confirming its superior performance in underwater image segmentation. Thus, this research offers an effective approach to semantic segmentation of underwater images.
Nevertheless, our model requires further optimization regarding computational efficiency. Given the real-time demands of AUVs during underwater operations, deeper exploration of the model’s computational efficiency remains essential. Thus, researching a lightweight and high-performance semantic segmentation model for underwater images is a significant future direction. Furthermore, the design of the attention mechanism and feature fusion strategy in this model is somewhat simplistic. The future introduction of more effective attention mechanisms and feature fusion strategies could hold considerable research significance in improving model performance. Finally, in contrast to the outstanding performance of popular models on terrestrial datasets, the complexity of underwater environments results in lower quality datasets, causing many models to underperform on underwater images. This represents one of the primary challenges encountered in the domain of underwater image processing. Additionally, the significant shortage of underwater image datasets poses another critical issue that requires immediate attention. Thus, in our future research, we intend to explore two main avenues. In terms of datasets, we will utilize data augmentation techniques and leverage advanced methods like Generative Adversarial Networks to generate high-quality underwater images, thereby enhancing the dataset’s diversity. Concurrently, we aspire to collaborate with marine research organizations to share data resources and perform field collection, thereby ensuring the dataset’s authenticity and effectiveness. From an algorithmic perspective, we will investigate lightweight model research to fulfill the demands for both model efficiency and high performance. Moreover, during the preprocessing phase, we intend to incorporate advanced and efficient image processing techniques to enhance the visual quality of images, thereby aiding the model in better feature extraction. We will also emphasize the enhancement of underwater image processing algorithms to address image quality issues resulting from complex underwater conditions, further improving the model’s segmentation performance. By pursuing these two avenues, we aim to propel the advancement of underwater image processing technologies, thereby offering more accurate technical support for marine ecological monitoring and biological studies.
Publicly available datasets were analyzed in this study. This data can be found here: https://irvlab.cs.umn.edu/resources/suim-dataset.
Ethical review and approval was not required for the animal study because in our study, we used an open database of SUIM dataset which can be freely used for academic purpose according to the instructions of database provider. Therefore, ethical review and approval was not required for this study.
BC: Funding acquisition, Methodology, Supervision, Writing – review & editing. WZ: Data curation, Methodology, Software, Writing – original draft, Writing – review & editing. QZ: Investigation, Writing – review & editing. ML: Conceptualization, Writing – review & editing. MQ: Supervision, Writing – review & editing. YT: Resources, Supervision, Validation, Writing – review & editing.
The author(s) declare that financial support was received for the research, authorship, and/or publication of this article. This work was supported by the Science and Technology Development Plan Project of Jilin Province, No.20240302074GX. This work was supported by the Smart Agricultural Engineering Research Center of Jilin Province Foundation, No.119122022005.
The authors declare that the research was conducted in the absence of any commercial or financial relationships that could be construed as a potential conflict of interest.
The author(s) declare that no Generative AI was used in the creation of this manuscript.
All claims expressed in this article are solely those of the authors and do not necessarily represent those of their affiliated organizations, or those of the publisher, the editors and the reviewers. Any product that may be evaluated in this article, or claim that may be made by its manufacturer, is not guaranteed or endorsed by the publisher.
Abdullah A., Barua T., Tibbetts R., Chen Z., Islam M. J., Rekleitis I. (2023). CaveSeg: deep semantic segmentation and scene parsing for autonomous underwater cave exploration. IEEE International Conference Robotics Automation (ICRA). 25, 3781–3788. doi: 10.1109/ICRA57147.2024.10611543
Badrinarayanan V., Kendall A., Cipolla R. (2017). SegNet: A deep convolutional encoder-decoder architecture for image segmentation. IEEE Trans. Pattern Anal. Mach. Intell. 39, 2481–2495. doi: 10.1109/TPAMI.2016.2644615
Bingham B., Foley B., Singh H., Camilli R., Delaporta K., Eustice R. M., et al. (2010). Robotic tools for deep water archaeology: Surveying an ancient shipwreck with an autonomous underwater vehicle. J. Field Robotics 27, 702–717. doi: 10.1002/rob.20350
Bogue R. (2015). Underwater robots: a review of technologies and applications. Ind. Robot: Int. J. 42, 186–191. doi: 10.1108/IR-01-2015-0010
Chen L. C., Papandreou G., Kokkinos I., Murphy K. P., Yuille A. L. (2014). Semantic image segmentation with deep convolutional nets and fully connected CRFs. CoRR abs/1412.7062. doi: 10.48550/arXiv.1412.7062
Chen L. C., Papandreou G., Kokkinos I., Murphy K., Yuille A. L. (2017a). Deeplab: Semantic image segmentation with deep convolutional nets, atrous convolution, and fully connected crfs. IEEE Trans. Pattern Anal. Mach. Intell. 40, 834–848. doi: 10.1109/TPAMI.2017.2699184
Chen L. C., Papandreou G., Schroff F., Adam H. (2017b). Rethinking atrous convolution for semantic image segmentation. ArXiv abs/1706.05587. doi: 10.48550/arXiv.1706.05587
Chen J., Tang J., Lin S., Liang W., Su B., Yan J., et al. (2022). RMP-Net: A structural reparameterization and subpixel super-resolution-based marine scene segmentation network. Front. Mar. Sci. 9, 1032287. doi: 10.3389/fmars.2022.1032287
Chen L. C., Zhu Y., Papandreou G., Schroff F., Adam H. (2018). “Encoder-Decoder with Atrous Separable Convolution for Semantic Image Segmentation.,” Lecture Notes in Computer Science (Springer International Publishing), 833–851. doi: 10.1007/978-3-030-01234-2_49
Cheng B., Misra I., Schwing A. G., Kirillov A., Girdhar R. (2022). “Masked-attention mask transformer for universal image segmentation,” in Proceedings of the IEEE/CVF conference on computer vision and pattern recognition. 1290–1299.
Cheng B., Schwing A., Kirillov A. (2021). Per-pixel classification is not all you need for semantic segmentation. Adv. Neural Inf. Process. Syst. 34, 17864–17875.
Dosovitskiy A., Beyer L., Kolesnikov A., Weissenborn D., Zhai X., Unterthiner T., et al. (2020). An image is worth 16x16 words: transformers for image recognition at scale. ArXiv abs/2010.11929. doi: 10.48550/arXiv.2010.11929
Fu J., Liu J., Tian H., Fang Z., Lu H. (2018). “Dual attention network for scene segmentation,” in 2019 IEEE/CVF Conference on Computer Vision and Pattern Recognition (CVPR). 3141–3149.
Girdhar Y. A., Giguère P., Dudek G. (2014). Autonomous adaptive exploration using realtime online spatiotemporal topic modeling. Int. J. Robotics Res. 33, 645–657. doi: 10.1177/0278364913507325
He K., Zhang X., Ren S., Sun J. (2016). “Deep residual learning for image recognition,” in Proceedings of the IEEE conference on computer vision and pattern recognition. 770–778.
Huang L., Yuan Y., Guo J., Zhang C., Chen X., Wang J. (2019). Interlaced sparse self-attention for semantic segmentation. ArXiv abs/1907.12273. doi: 10.48550/arXiv.1907.12273
Islam M. J., Edge C., Xiao Y., Luo P., Mehtaz M., Morse C., et al. (2020a). “Semantic segmentation of underwater imagery: dataset and benchmark,” in 2020 IEEE/RSJ International Conference on Intelligent Robots and Systems (IROS). 1769–1776.
Islam M. J., Xia Y., Sattar J. (2020b). Fast underwater image enhancement for improved visual perception. IEEE Robotics Automation Lett. 5, 3227–3234. doi: 10.1109/LSP.2016.
Kerai S., Khekare G. (2024). Contextual embedding generation of underwater images using deep learning techniques. IAES Int. J. Artif. Intell. (IJ-AI) 13, 3111–3118. doi: 10.11591/ijai.v13.i3.pp3111-3118
Khekare G., Kerai S., Turukmane A. V., Khekare U., Sharma R., Agrawal R. (2024). Enhancing underwater imagery using multicriteria decision-making with. Multi-Criteria Decision-Making Optimum Design Mach. Learning. doi: 10.1201/9781032635170-9
Kim Y. H., Park K. R. (2022). PSS-net: Parallel semantic segmentation network for detecting marine animals in underwater scene. Front. Mar. Sci. 9, 1003568. doi: 10.3389/fmars.2022.1003568
Kingma D. P., Ba J. (2014). Adam: A method for stochastic optimization. CoRR abs/1412.6980. doi: 10.48550/arXiv.1412.6980
Li H., Xiong P., An J., Wang L. (2018). Pyramid attention network for semantic segmentation. ArXiv abs/1805.10180. doi: 10.48550/arXiv.1805.10180
Lin T.-Y., Dollár P., Girshick R. B., He K., Hariharan B., Belongie S. J. (2016). “Feature pyramid networks for object detection,” in 2017 IEEE Conference on Computer Vision and Pattern Recognition (CVPR). 936–944.
Liu F., Fang M. (2020). Semantic segmentation of underwater images based on improved Deeplab. J. Mar. Sci. Eng. 8, 188. doi: 10.3390/jmse8030188
Liu Z., Lin Y., Cao Y., Hu H., Wei Y., Zhang Z., et al. (2021). “Swin transformer: hierarchical vision transformer using shifted windows,” in 2021 IEEE/CVF International Conference on Computer Vision (ICCV). 9992–10002.
Long J., Shelhamer E., Darrell T. (2015). “Fully convolutional networks for semantic segmentation,” in Proceedings of the IEEE conference on computer vision and pattern recognition. 3431–3440.
Ma Z., Li H., Wang Z., Yu D., Wang T., Gu Y., et al. (2021). An underwater image semantic segmentation method focusing on boundaries and a real underwater scene semantic segmentation dataset. doi: 10.48550/arXiv.2108.11727
Ouyang D., He S., Zhan J., Guo H., Huang Z., Luo M. L., et al. (2023). “Efficient multi-scale attention module with cross-spatial learning,” in ICASSP 2023 - 2023 IEEE International Conference on Acoustics, Speech and Signal Processing (ICASSP). 1–5.
Ronneberger O., Fischer P., Brox T. (2015). “U-net: Convolutional networks for biomedical image segmentation,” in Medical image computing and computer-assisted intervention–MICCAI 2015: 18th international conference, Munich, Germany, October 5-9, 2015, proceedings, part III 18: Springer. 234–241.
Shkurti F., Xu A., Meghjani M., Higuera J. C. G., Girdhar Y. A., Giguère P., et al. (2012). “Multi-domain monitoring of marine environments using a heterogeneous robot team,” in 2012 IEEE/RSJ International Conference on Intelligent Robots and Systems. 1747–1753.
Simonyan K., Zisserman A. (2014). Very deep convolutional networks for large-scale image recognition. arXiv preprint arXiv:1409.1556. doi: 10.48550/arXiv.1409.1556
Tolstikhin I. O., Houlsby N., Kolesnikov A., Beyer L., Zhai X., Unterthiner T., et al. (2021). Mlp-mixer: An all-mlp architecture for vision. Adv. Neural Inf. Process. Syst. 34, 24261–24272. doi: 10.48550/arXiv.2105.01601
Vaswani A., Shazeer N. M., Parmar N., Uszkoreit J., Jones L., Gomez A. N., et al. (2017). “Attention is all you need,” in Neural Information Processing Systems.
Wang J., He X., Shao F., Lu G., Hu R., Jiang Q. (2022). Semantic segmentation method of underwater images based on encoder-decoder architecture - PubMed. PloS One 17. doi: 10.1371/journal.pone.0272666
Wang H., Köser K., Ren P. (2025). Large foundation model empowered discriminative underwater image enhancement. IEEE Journals Magazine | IEEE Xplore. 63, 1–17. doi: 10.1109/TGRS.2025.3525962
Xiao T., Liu Y., Zhou B., Jiang Y., Sun J. (2018). “Unified perceptual parsing for scene understanding,” in Proceedings of the European conference on computer vision (ECCV). 418–434.
Xie E., Wang W., Yu Z., Anandkumar A., Alvarez J. M., Luo P. (2021). SegFormer: Simple and efficient design for semantic segmentation with transformers. Adv. Neural Inf. Process. Syst. 34, 12077–12090. doi: 10.48550/arXiv.2105.15203
Yu F., Koltun V. (2015). Multi-scale context aggregation by dilated convolutions. CoRR abs/1511.07122. doi: 10.48550/arXiv.1511.07122
Zhang W., Pang J., Chen K., Loy C. C. (2021). K-net: Towards unified image segmentation. Adv. Neural Inf. Process. Syst. 34, 10326–10338. doi: 10.48550/arXiv.2106.14855
Zhang Z., Sabuncu M. (2018). Generalized cross entropy loss for training deep neural networks with noisy labels. Adv. Neural Inf. Process. Syst. 31, 8792–8802.
Zhang W., Wei B., Li Y., Li H., Song T. (2024). WaterBiSeg-Net: An underwater bilateral segmentation network for marine debris segmentation. Mar. pollut. Bull. 205, 116644. doi: 10.1016/j.marpolbul.2024.116644
Zhao H., Shi J., Qi X., Wang X., Jia J. (2016). “Pyramid scene parsing network,” in 2017 IEEE Conference on Computer Vision and Pattern Recognition (CVPR). 6230–6239.
Zhong Z., Lin Z. Q., Bidart R., Hu X., Daya I. B., Li J., et al. (2019). “Squeeze-and-attention networks for semantic segmentation,” in 2020 IEEE/CVF Conference on Computer Vision and Pattern Recognition (CVPR). 13062–13071.
Keywords: underwater images, semantic segmentation, attention mechanism, feature fusion, SegFormer
Citation: Chen B, Zhao W, Zhang Q, Li M, Qi M and Tang Y (2025) Semantic segmentation of underwater images based on the improved SegFormer. Front. Mar. Sci. 12:1522160. doi: 10.3389/fmars.2025.1522160
Received: 04 November 2024; Accepted: 21 February 2025;
Published: 11 March 2025.
Edited by:
David Alberto Salas Salas De León, National Autonomous University of Mexico, MexicoReviewed by:
Khaled Ahmed Nagaty, British University in Egypt, EgyptCopyright © 2025 Chen, Zhao, Zhang, Li, Qi and Tang. This is an open-access article distributed under the terms of the Creative Commons Attribution License (CC BY). The use, distribution or reproduction in other forums is permitted, provided the original author(s) and the copyright owner(s) are credited and that the original publication in this journal is cited, in accordance with accepted academic practice. No use, distribution or reproduction is permitted which does not comply with these terms.
*Correspondence: Mingyang Qi, cWltaW5neWFuZ0BqbG5rdS5lZHUuY24=; You Tang, dGFuZ3lvdTkwMDBAMTYzLmNvbQ==
†These authors have contributed equally to this work and share first authorship
Disclaimer: All claims expressed in this article are solely those of the authors and do not necessarily represent those of their affiliated organizations, or those of the publisher, the editors and the reviewers. Any product that may be evaluated in this article or claim that may be made by its manufacturer is not guaranteed or endorsed by the publisher.
Research integrity at Frontiers
Learn more about the work of our research integrity team to safeguard the quality of each article we publish.