- 1Department of Veterinary and Animal Sciences, Faculty of Health and Medical Sciences, University of Copenhagen, Frederiksberg, Denmark
- 2Centre for Epidemiology and Planetary Health (CEPH), SRUC School of Veterinary Medicine, Inverness, United Kingdom
The sustainability of the salmon farming industry is being challenged by increased mortality rates. Scotland’s open-source salmon production data provides the possibility of developing an industry-wide mortality monitoring model, valuable for identifying and addressing unexpected increases in mortality without needing data sharing agreements across different companies. This study aimed to utilize these data to develop a hierarchical dynamic linear model (DLM) for monitoring monthly mortality of maricultured Atlantic salmon in Scotland. We evaluated whether considering the hierarchical structure present in the data (country, region, and site) would improve mortality predictions when compared to the production cycle level DLMs developed in a previous study. Our findings demonstrated that the hierarchical DLM outperformed the production cycle level DLMs, confirming the value of this more complex modelling approach. Nevertheless, the hierarchical model, like the production cycle level DLMs, exhibited some uncertainty in the mortality predictions. When mortality is higher than expected, site level warnings are generated, which can encourage producers and inspectors to further investigate the cause. Between 2015 and 2020, approximately 25% of the production cycles and 50% of the sites encountered at least one warning, with most warnings happening in the summer and autumn months. Additionally, the hierarchical model enabled monitoring mortality at multiple levels. This information is useful for various stakeholders as part of a monitoring system, offering insights into mortality trends at national, regional, and sites levels that may benefit from strategic resource management. Recommendations for model improvements include utilizing shorter data aggregation periods, such as weekly, which are not currently available as open-source data.
1 Introduction
Farmed salmon are a significant contributor to the aquaculture trade, playing an increasingly important role in providing food and nourishment worldwide (FAO, 2022). Scotland stands as the third-largest producer of farmed salmon globally (Iversen et al., 2020). Within the UK, farmed salmon is one of the top food exports (Department for Environment, Food & Rural Affairs, 2023). Despite these achievements, the industry faces sustainability concerns particularly with mortality rates exceeding 20% of the production in the last years in Scotland (Munro, 2023), a trend also observed in Norway (Sommerset et al., 2024). This mortality translates into economic losses and suboptimal fish welfare and is one of the main challenges to the industry’s sustainable growth (Noble et al., 2018).
Salmon aquaculture is a highly technologically advanced industry (FAO, 2022), which collects an increasing amount of data. In Scotland, the government systematically collects and collates monthly mortality data from all producers, making it publicly accessible in a standardized format across all sites. This continuously updated resource holds the potential for the development of an industry-wide mortality monitoring model, eliminating the need for complex data sharing agreements.
In a previous study, these Scottish open-source mortality data were used to create dynamic linear models (DLMs) at production cycle level (Merca et al., 2024). A univariate production cycle level DLM was created using exclusively mortality data. Additionally, several multivariate production cycle level DLMs were developed using mortality data with different combinations of environmental variables. The best multivariate model was the one that incorporated mortality and salinity related variables. Despite the presence of uncertainty in mortality predictions, the Scottish open-source mortality data enabled monitoring salmon mortality. Indeed, it was possible to trigger warnings when mortality was higher than expected, enabling further investigation of the cases if implemented in real-time. Nevertheless, the authors hypothesized that a hierarchical framework, where multiple units (in this case: sites, regions, and country) organized in a stratified structure are monitored simultaneously, could potentially improve the monitoring of salmon mortality. For instance, the hierarchical model can take into account that the sites within the same region are more closely correlated than sites in different regions (Dohoo et al., 2014). Besides, hierarchical modelling would enable monitoring mortality at multiple levels. Such information can be valuable for different stakeholders, by providing insights about mortality trends at regional and national levels, and by allowing comparisons of mortality across different regions.
In animal production, hierarchical DLMs are proven effective in capturing complex relationships and dynamics across multiple organizational levels, facilitating informed decision-making. For instance, in pig production, a hierarchical DLM was successfully employed to analyze drinking patterns across pen, section, and herd levels (Dominiak et al., 2019b, a). In another study in pig herds, conducted by Bono et al. (2012), DLMs were employed for monitoring litter sizes at both herd and sow levels. In dairy cows, DLMs have been implemented to estimate the effects of interventions at herd level, while also considering the cow effect (Stygar et al., 2017; Skjølstrup et al., 2022; Rustas et al., 2024). For aquatic animals, fewer examples of hierarchical modelling exist. In trout, hierarchical modelling has been used to study trout growth in locations such as Lake Superior, North America (Stebbins et al., 2024), and in Neste d’Oueil, Pyrénéss, France (Lecomte and Laplanche, 2012). For salmon, examples include Hubley and Gibson (2011) who developed a Bayesian hierarchical model to estimate the annual mortality of wild Atlantic salmon in Nova Scotia, Canada. Another example is Scheuerell et al. (2015), who used a hierarchical time-series model to investigate the effects of large-scale hatchery supplementation on the density of wild Pacific salmon adults.
The purpose of this study was to use the same open-source mortality data as in Merca et al. (2024) to develop a hierarchical DLM for monitoring monthly mortality of maricultured Atlantic salmon in Scotland. We assessed whether this more complex modelling approach that takes the hierarchical structure of the data into account improved the predictions of mortality when compared to the production cycle level DLMs previously developed. Besides, the hierarchical model can generate estimates for salmon mortality at site, region, and country levels, allowing stakeholders to monitor mortality at different levels. Additionally, the hierarchical DLM created was designed to trigger warnings when the observed mortality exceeds the expected levels. These warnings can inform producers, veterinarians, and inspectors, alerting them to further investigate. More specifically, we had four objectives: (1) to create a hierarchical DLM using mortality data from salmon sites in Scotland; (2) to compare the production cycle level DLMs with the hierarchical model and select the best model for monitoring salmon mortality; (3) to monitor salmon mortality at multiple levels (4) to create warnings at site level when observed mortality exceeded the expected levels.
2 Materials and methods
The present study involved data cleaning, manipulation, and modelling utilizing the statistical programming environment R (R Core Team, 2022) and RStudio (Posit team, 2022). The time-series analysis workflow is freely available (https://doi.org/10.5281/zenodo.13881599).
2.1 Data source
The data used in this study consisted of the same production data previously utilized in Merca et al. (2024), obtained from the Scotland’s Aquaculture website (http://aquaculture.scotland.gov.uk/; last accessed 9 February 2023). The data were extracted from a dataset called “Fish Farm Monthly Biomass and Treatments”, which consists of monthly data submitted by all active producers of all fish species produced in Scotland. Specifically, this study focuses on production data of seawater Atlantic salmon (Salmo salar L.), covering the period from 2002 to 2020.
2.2 Data cleaning and data manipulation
The data cleaning and manipulation procedures conducted in this study followed the framework outlined in Merca et al. (2024), as the same mortality data were utilized in both studies. For a more extensive description of these procedures, refer to Merca et al. (2024).
All marine salmon sites operating in Scotland from 2002 to 2020 were included in the original dataset, totaling 402 sites (open sea farms) and 2138 production cycles (period between stocking and harvesting). Those sites were spread across the six regions of Scotland: Highland, Argyll & Bute, Shetland Islands, Eilean Siar, Orkney Islands, and North Ayrshire (see Merca et al., 2024, Figure 1). Since North Ayrshire region only has one site, it was grouped with the nearest region, i.e. Argyll & Bute. Only the resulting five regions were considered.
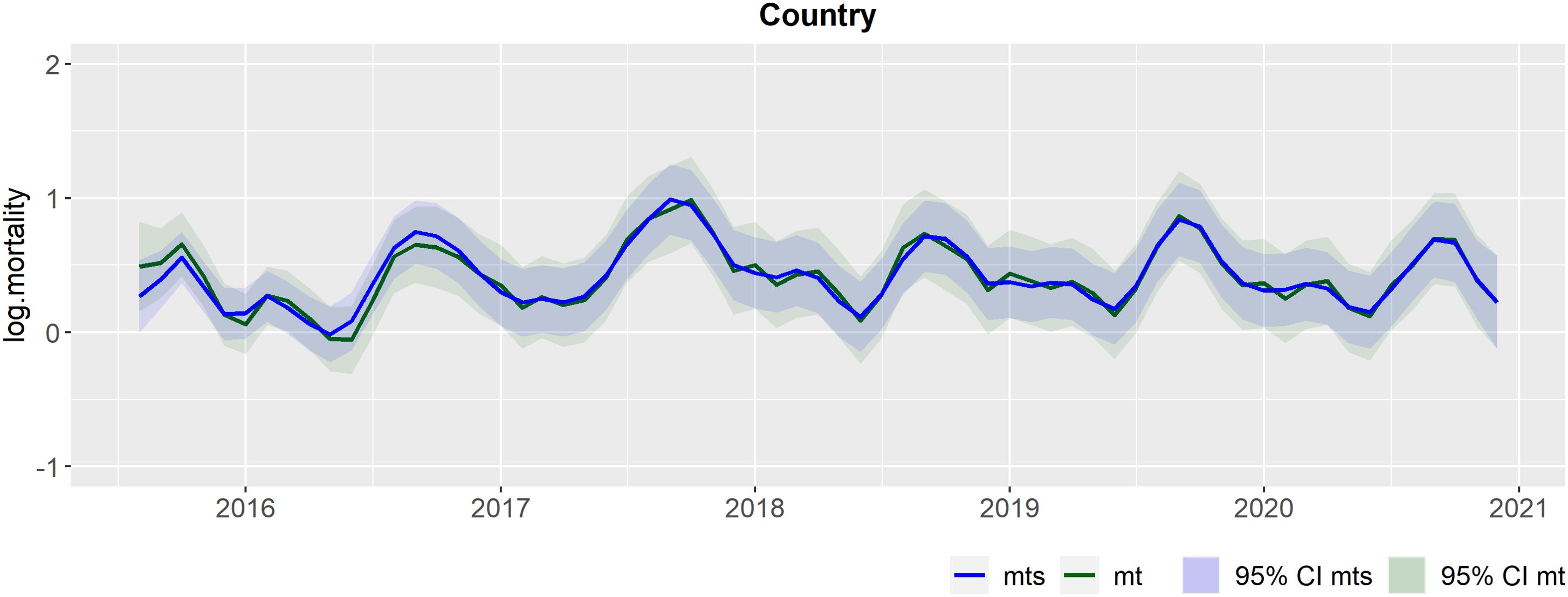
Figure 1. Outcomes from the hierarchical DLM at country level. In green: Filtered mean for the country level (mt) and the respective 95% credible interval (CI); In blue: Smoothed mean for the country level (mts) and the respective 95% credible interval (CI).
The dataset used contained monthly mortality data reported as kilograms of dead salmon, per month and per site. In order to account for variations in production size, we converted mortality into proportions by utilizing the biomass data also available:
Upon analyzing the mortality proportions, we identified some as unrealistic, such as instances of negative mortality proportions. The unrealistic values are potentially due to fish movements between sites during the month, for which timing and quantities were unavailable. The reported biomasses represent a snapshot of the biomasses at the end of the month, while mortality accounts for cumulative mortalities throughout the month. Therefore, when fish are moved from one location to another within a given month, the site from where the fish left will report biomasses at the end of the month that are lower relative to the mortalities recorded within that same month. On the other hand, when fish are introduced to a site during the month, the reported biomasses are higher compared to the mortalities observed. To mitigate this error, we tried to capture these movements by defining biomasses considered lower than realistic as
and biomasses higher than realistic as:
where corresponds to the current month and to the previous month. For both cases we established limits of 20% deviation from expected biomasses, and any biomasses falling below or above were deemed abnormal (Equations 2 and 3). Additionally in Equation 3, we relied on feed intake reports (available in the dataset) to foresee how much the salmon where expected to grow in each month. We used a reported feed conversion ratio (FCR) of 1.3 for salmon in the United Kingdom (Torrissen et al., 2011), meaning that 77% of feed intake is transformed into weight gain. Equations 2 and 3 were applied to all reported biomasses in the dataset, and any considered lower or higher than realistic were replaced with missing values. The remaining realistic biomasses were then used to calculate mortality according to Equation 1.
Additionally as part of the data cleaning process, some sites and production cycles were excluded from the study. This was done for various reasons such as missing data and records that did not meet the standard production cycle for commercial purposes, as detailed in Merca et al. (2024).
After data cleaning and manipulation, our study population was reduced to 293 seawater Scottish salmon sites, corresponding to 1610 production cycles distributed in five regions of Scotland.
2.2.1 Transformation and standardization
According to West and Harrison (1997), the residuals of a DLM are expected to follow a normal distribution, as long as the system does not deviate from the expected behavior. In order to achieve this, mortality values underwent a logarithmic transformation: . As some of these mortality values were zero, we added a small constant before applying the logarithmic transformation. The data were then standardized by subtracting the sample mean from all observations and dividing them by the sample standard deviation.
2.3 Learning and test sets
The available data (293 sites and 1610 production cycles) were utilized to create the learning and test sets, employing the same methodology as described in Merca et al. (2024). By using the same learning and test sets in both studies, a direct and comprehensive comparison of the results can be achieved. The learning set consisted of 145 sites and 784 production cycles, which were utilized to estimate the parameters for the DLM. The test set comprised the same 145 sites and 353 additional production cycles used to validate the models.
2.4 Hierarchical dynamic linear model
Dynamic linear models (DLMs) represent a specific type of time series models. DLMs rely on a Bayesian framework to infer the underlying parameter vector from observed data, while incorporating any relevant prior information available before the observations are made. Each time step involves forecasting values accommodating measurement errors and allowing systematic fluctuations (West and Harrison, 1997).
Hierarchical models (also referred to as multi-level models) are convenient when dealing with data that have an inherent stratified structure. In this case, a dataset comprising a country with different regions, each containing various sites, is an example of a dataset that might benefit from hierarchical modelling. This approach is likely to be advantageous because different geographical locations likely result in greater similarity within the same region, such as similar water temperatures or currents. Hierarchical modelling captures those correlations between different units, thereby potentially explaining more variation than would be explained without taking the structure into account. Moreover, it enabled simultaneous monitoring of mortality at multiple levels.
An extensive framework for constructing production cycle level DLMs (non-hierarchical) using the same mortality data is described in Merca et al. (2024). Hierarchical DLMs are defined within the usual framework of a DLM with an observation equation and a system equation. The hierarchical architecture of the model is only reflected in the structure of the matrices present in those equations.
In this study, we created a hierarchical DLM using site level monthly mortality data (logarithmically transformed). The model created had all three levels (country, region, and site) directly represented. Consider as the observed value (mortality) in month for site within region. A straightforward approach of modelling the hierarchy (country/region/site) is through the following model:
where is a dynamic country level, is a dynamic region level, is a dynamic site level, and is a random observation error. Therefore, each component contributes to explaining a specific aspect of the observed mortality. This framework allows for changes over time across all levels – country, region, and site.
2.4.1 Harmonic waves and trend factors
After running the simplest type of a hierarchical DLM where no time trends were assumed, we noticed a seasonal pattern in the country level mortality estimates. Thus, we incorporated that seasonality into our model by using the Fourier form representation (West and Harrison, 1997), which involves a linear combination of trigonometric functions (sine and cosine, also known as harmonic waves). We tested four approaches: including solely one harmonic wave, the sum of two harmonic waves, the sum of three harmonic waves, and not including any harmonic wave. To compare the performance of these four approaches, we calculated the Root Mean Squared forecast Error (RMSE) for the forecast errors across all sites. The forecast errors consist of the difference between the observed mortality and the forecasted mortality values. Smaller RMSE values indicate greater model precision. We found that the sum of two harmonic waves provided the best fit for the data.
Each harmonic wave is represented by two parameters, in addition to a mean level element. Using the sum of two harmonic waves, 5 parameters were needed: . Thus, the country level was defined as follows:
where corresponds to the intercept, and represent the parameters of the first harmonic wave and and represent the parameters of the second harmonic wave.
For the regional levels, we did not consider any time trend. However, we assumed a trend for the site levels, as they may change according to the stage of the production cycle (i.e. the age of the fish at the site). To model this trend, we use a spline function created using the smooth.spline function available in stats R package (R Core Team, 2022). One spline function was developed for each site, which provided the desired shape to reflect the mortality of the corresponding site’s production cycles. The magnitude of the trend was dynamically estimated as a trend factor .
2.4.2 Observation equation
The observation equation (Equation 5) can be written in matrix notation as
and describes how the values of an observation vector depend on underlying (unobservable) parameters , through a transposed design matrix . Observation errors are also considered . Here, corresponds to a vector with the observed mortalities of all sites in month . The parameter vector was defined as
where indexes , , , , and represent the five regions, denotes the trend factor for site in region at month and corresponds to the total number of sites in region . The parameter vector consisted of 300 elements.
The transposed design matrix is where the structure of the model is represented, allowing the integration of information from all three levels. The number of rows in is equal to the number of sites and the number of columns corresponds to the size of. Thus, when no observations were missing in month , it corresponded to a matrix:
Each row corresponded to a site (here five sites are represented, each belonging to a different region). The first five columns corresponded to the country level, followed by other five columns representing the regions levels. The remaining 290 columns corresponded to the sites levels and trend factors. When there was a missing observation for some site in month , the row corresponding to that site on the matrix was excluded.
This model already takes the hierarchy into account by seeing each observation as a result of effects at all three levels. Furthermore, it may be reasonable to assume that the error terms within each level are correlated with each other. This can be accomplished if the individual error term is seen as the sum of three underlying independent errors at the country , region , and site levels
Thus, the variance-covariance matrix of , when no observations were missing in month , corresponded to a matrix, represented as:
considering that the sites represented in the first two columns (and rows) are situated within the same region (region ), while the sites represented in the last two columns (and rows) are both part of a common region which is different from the initial one (region E).
2.4.3 System equation
The system equation (Equation 6) is represented as
where is called the system matrix and serves to update the parameter vector, and represents the system errors. The system matrix is a quadratic matrix with the same size as :
where , (being the expected log-transformed mortality at times and for site in region , given by the spline function), and corresponds to the total number of sites in region . The two harmonic waves for the country level are represented in the first five rows and columns. The expected rate of change in log-transformed mortality are shown in the corresponding rows and columns of each site, being utilized to update the site levels.
The systematic variance-covariance matrix of describes how much each element of the system is likely to randomly change from one time step to the next, and was calculated using a component discounting approach described by West and Harrison (1997). A discount factor is a numeric value that falls within the range of 0 to 1. Discount factors that are closer to 0 reflect large system variance, while those closer to 1 indicate a small variance. This approach is referred to as component discounting, as it allows each level (component) to have its own discount factor. Therefore, the model can change at a different rate for each level, offering more modelling flexibility. In this case, three discount factors were included: one for country, one for region , and one for site . The resulting variance-covariance matrix is a block-diagonal matrix with a dimension of .
2.4.4 Initialization
In order to fully describe a DLM, it is necessary to provide the initial distribution of the parameter vector , before any observations are made . We achieved this my defining an initial mean and the initial variance-covariance matrix .
The initial mean followed the same structure of , first having the country elements, then the regions, followed by the sites. For the season-dependent country level two harmonic waves were introduced, representing 5 elements. We created a linear regression to determine the relationship between the observations of mortality (on the learning set) and a trigonometric function representing a sum of two harmonic waves, for a period of 12 months. The resulting coefficients estimates were used in . The coefficient estimates corresponded to the intercept and one sine and cosine wave for each harmonic wave used, resulting in 5 elements. For the regions’ levels, we calculated the average of mortality for each region using the learning set. We then subtracted the five elements calculated for the country level, and added the results to . Finally, for the sites’ elements, each site had a level and a trend factor. For all sites, the level and the trend factor were set to 0 and 1, respectively. A trend factor of 1 indicates that, prior to any observations, we expected the system to evolve according to the estimated spline function.
The initial variance-covariance matrix corresponded to a quadratic matrix with the same size as , also having the country, regions, and sites elements. For the country part, we applied the vcov function available on stats R package (R Core Team, 2022) to the linear regression previously created for . This function returned a variance-covariance matrix of the main parameters of the regression model. For the regions’ elements, we used the learning set to calculate the average of mortality in each region, for the first 7 months of the production cycles. Therefore, for each region we had 7 values, one for each of the 7 months. From each of those values, we subtracted the five elements calculated for the country level in . Then, we computed the covariance of those subsequent 7 elements, resulting in one value per region. For the sites, we also calculated the average of mortality in each site, for the first 7 months of the production cycles. To each of those 7 elements, we subtracted the corresponding region mean and the five elements calculated for the country level in . Then, on the 7 resulting values, we calculated the differences between each two consecutive elements. Finally, we computed the covariance between the differences and the 7 mortality averages already discounting the country and region parts. The final variance-covariance matrix was accomplished by diagonally combining the country individual variance-covariance matrix, the regions variances and the sites variance-covariance matrices.
It is worth mentioning that the initial values of and were of minor importance. As soon as the DLM was applied to the data, it automatically adapted over time.
2.4.4.1 When a new production cycle starts
Every time a new production cycle started, the site needed to be reinitialized to ensure it did not incorporate information from previous production cycles when predicting the new one.
The system equation (Equation 6) updates the parameter vector from to . Whenever a new production cycle started, we wanted to reset the corresponding site to its initial state. Therefore, we changed the level and the trend factor for the specific site in to 0 and 1, respectively (as in ). Moreover, in , we inserted zeros in the rows and columns of the site that had a new production cycle starting in order to “break the connection” between cycles. Finally, we added some additional variance to the elements of for the specific site that was initiating a new production cycle. Hence, we increased the adaptability of the model.
2.4.5 Optimizing DLM variance components
For a full specification of the variance components of this hierarchical model, six parameters were needed: three observational variances (used in ) and three discount factors (used in ).
These six parameters were estimated from the learning set, through numerical optimization. The values selected were the ones that minimized the Root Mean Squared forecast Error (RMSE). This estimation was performed using the optim function in R (R Core Team, 2022), with the Nelder-Mead optimization algorithm. The resulting observational variances were , and , and the discount factors were , and .
2.4.6 Filtering and smoothing
The DLM incorporated the Kalman filter technique, which produces estimates updated according to new observations (West and Harrison, 1997). Given the information available at time (), the conditional probability of the parameter vector is denoted as , where is the filtered mean and the variance-covariance matrix. The Kalman filter allowed us to obtain monthly expected values (filtered mean and variance) and forecasts for mortality at site level. Additionally, it gave us expected mortality values for the country as a whole and for each of the five regions.
The parameter vectors are autocorrelated. The Kalman filter estimates the based on previous information only. However, due to this autocorrelation, the future observations also contain valuable information for estimating . Therefore, a retrospective analysis called smoothing can be employed (West and Harrison, 1997). This method takes into account all the available observations, including past and future ones, providing the best possible estimates.
2.5 Monitoring at country and region levels
As stated in Equation 4, the observed mortalities at a site consisted of the sum of the dynamic country, region, and site levels. However, obtaining mortality estimates at the country and regional levels required additional calculations.
To obtain the mortality estimates at regional level, we defined a vector that could extract the regional estimates from the estimated means (filtered and smoothed). For each region and at each time step, a different vector was created. For example, the vector for region at time was defined as
where corresponds to the number of sites belonging to region with observations at time . This vector had the same structure as , where the first five elements correspond to the country level, the following five values represent the different regions (with the number 1 in the position of region ), and the remaining elements correspond to the sites levels and trends. In the positions of the sites levels belonging to region with observations at time , we assigned to equally capture each site’s influence on the region. The same logic was employed to create the vectors for all five regions. Then, to extract the mortality estimates at regional level, we multiplied the previously described vector by the filtered mean (). To extract the mortality estimates at regional level estimated by the retrospective smoothening, the same methodology was used: the same previously explained vector was multiplied by the smoothed mean.
For the country level, we also created a vector to extract the mortality estimates:
where the , , , , and correspond to the number of sites belonging to regions , , , , and , respectively, with observations at time . The logic behind the structure of this vector was the same as that used in creating the vectors for the regions: the first five elements represent the country level, the next five values correspond to the regions, and the following represent the sites levels and trends. In all region positions, we assigned , where 5 represents the number of regions, to equally reflect the impact for each of the five regions on the country. The same rationale was employed for the site levels, where we divided 1 by the product of 5 (number of regions) and the number of sites with observations at time within each region. Positions corresponding to sites where no observations existed at time were set as 0. Subsequently, to extract the mortality estimates at country level, both filtered and smoothed, we multiplied the country vector by the filtered mean () and by the smoothed counterpart, respectively.
Finally, we also needed to extract the variance of the smoothed and filtered levels for region and for country . The same previously defined vectors were again used. At regional level the variance was extract as
where and at country level
where the corresponds to the variances of the filtered or smoothed levels, depending on which variance we are extracting, at time .
2.6 Generating warnings
Warnings were generated at site level. A warning was defined as any observation of mortality falling above the 95% credible intervals (CI). The 95% CI were determined using the forecasted values ft (and their respective variance Qt) produced by the Kalman filter, as:
where . The warnings were generated using the same methodology as in the previous study (Merca et al., 2024).
3 Results
The results presented were achieved by applying the hierarchical DLM to the test set using initial specifications derived from the learning set.
3.1 Comparison of hierarchical and production cycle level DLMs
After developing the hierarchical DLM (objective 1), the second objective was to compare the performances of the univariate production cycle level DLM (which used only mortality data) and the best multivariate production cycle level DLM (which incorporated both mortality and salinity related variables) created in a previous study (Merca et al., 2024), with the newly developed hierarchical DLM. These comparisons involved assessing the discrepancies between the mortality observations and the models predictions, represented as forecast errors. The RMSEs were calculated for the collective set of mortality forecast errors across all production cycles for the production cycle level DLMs and across all sites for the hierarchical DLM.
The RMSEs of the three models are shown in Table 1. The smaller RMSE for the hierarchical DLM suggest better precision. To determine the statistical significance of these differences, a paired t-test was performed on the squared forecast errors of the models being compared. T-tests are recognized for their utility in studies characterized by large sample sizes and are noted for their robustness, even when dealing with skewed data (Fagerland, 2012). Since we aimed to compare variances, squaring the forecast errors was appropriate. The p-value computed between the univariate production cycle level DLM and the hierarchical DLM was , and between the multivariate production cycle level DLM and the hierarchical DLM. Thus, we can conclude that the hierarchical DLM performed significantly better than both production cycle level DLMs.
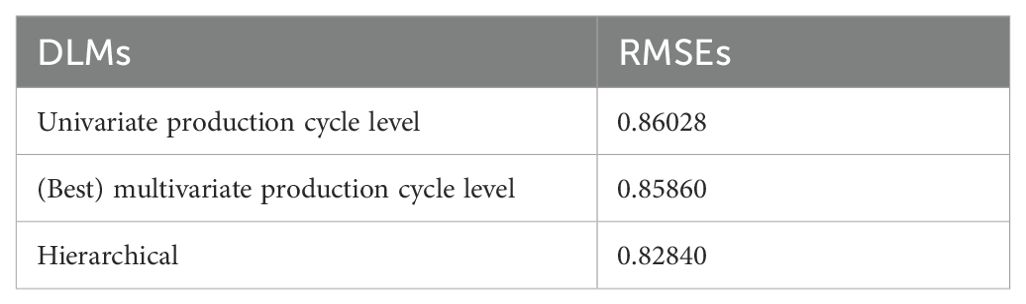
Table 1. Root mean squared errors (RMSEs) for the univariate and best multivariate production cycle level DLMs (Merca et al., 2024), and the hierarchical DLM.
3.2 Monitoring mortality at multiple levels
The hierarchical DLM provided estimates of mortality at country, region, and site levels (objective 3). At country level (Figure 1), we can see the filtered mean estimated by the prospective Kalman filter (in green) and the smoothed mean (in blue) estimated by the retrospective smoothening. Both estimates are accompanied by their corresponding 95% credible intervals (CI), derived from their respective variance components. The filtered mean (in green) provides the most accurate estimates of the true underlying mortality level at each time step, incorporating all prior information. Meanwhile, the smoothed mean (in blue) offers the best possible estimates of mortality at country level by considering all available information both before and after each time step. At country level, mortality exhibits a consistent pattern with clear seasonal variations, showing higher mortality in the summer months (Figure 1).
At region level (Figure 2), the filtered mean (in green) and the smoothed mean (in blue) are illustrated for each of the five regions included in this study. At the region levels it is also possible to see the seasonality in the estimates, with increased mortality during the summer. Each region displayed distinct mortality trends that can be analyzed individually. The region Highland experienced an upward trajectory in mortality from 2016 to 2018, while being stable in subsequent years. Argyll & Bute showed a notable decrease in mortality in 2018 compared to the other years. The Shetland Islands exhibited a decrease in mortality in the last months of 2020. In the Orkney Islands it is possible to see a declining trend in mortality over the years. Eilean Siar showed an increase in mortality in the last six months of 2020.
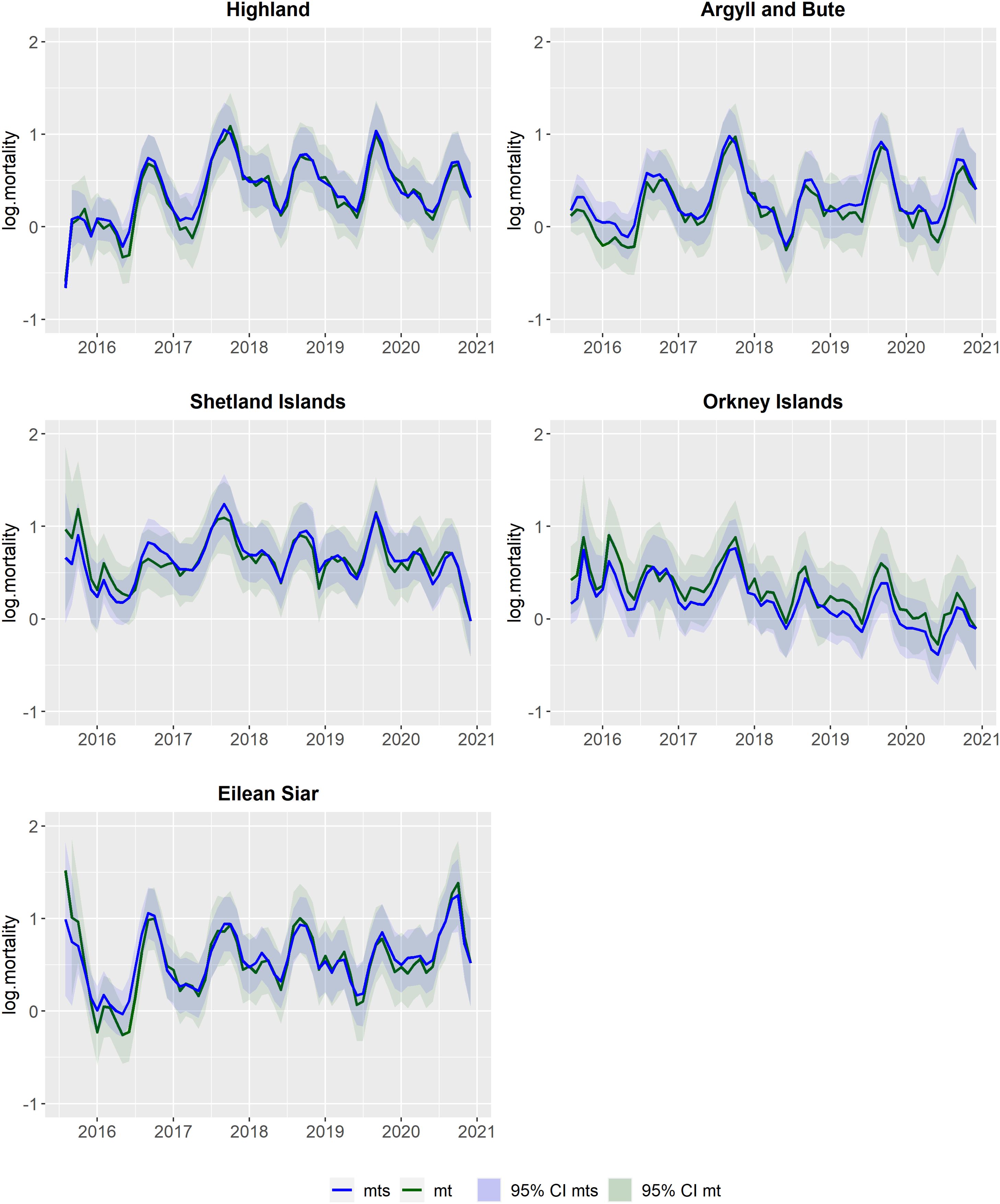
Figure 2. Outcomes from the hierarchical DLM at region level, for each of the five regions. In green: Filtered means for the regional levels (mt) and the respective 95% credible intervals (CI); In blue: Smoothed means for the regional levels (mts) and the respective 95% credible intervals (CI).
Here, we explain the site level using two sites as examples: Site X (Figures 3A, B) and Site Y (Figures 4A, B). Starting with Site X, the DLM provided estimates (filtered and smoothed means - Figure 3A) but also the forecasted values produced at each time step in the Kalman filter (Figure 3B). The two darker grey shaded areas represent the two production cycles present in Site X during the analyzed timeframe, interrupted by a fallow period in which no fish were present in the site. Figure 3A illustrates that the means (filtered and smoothed) demonstrated a consistent alignment with the observations. It is worth noticing that the DLM continued to provide estimates even in the absence of observations, based on information from other sites (for instance in the last months of 2017 and beginning of 2018). Additionally, it is evident that during the periods without observations (between the production cycles), the 95% confidence intervals widened, reflecting increased uncertainty about the true mortality values due to the lack of mortality data. In Figure 3B, the forecasts are depicted along with the corresponding 95% CI. The 95% CI are wide, implying a degree of uncertainty in the forecasts. In Site X the mortality is considered to be as expected, since the observations do not exceed the 95% CI.
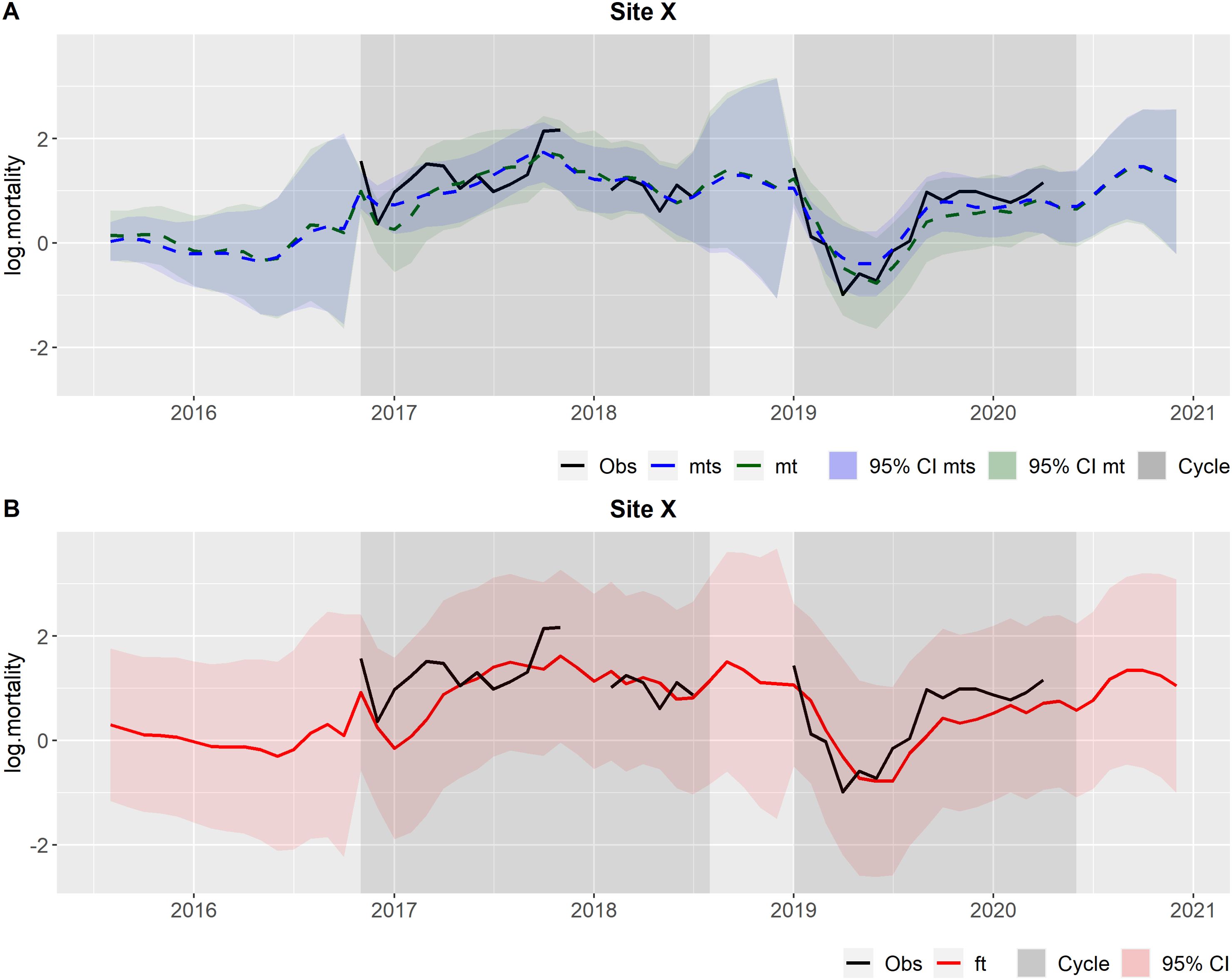
Figure 3. Outcomes from the hierarchical DLM at site level, for Site X. Observations (obs) in black and the production cycles are represented by the grey shaded areas. (A) In green: Filtered mean (mt) and the respective 95% credible interval (CI); In blue: Smoothed mean (mts) and the respective 95% credible interval (CI). (B) In red: Forecasts (ft) and the respective 95% credible interval (CI).
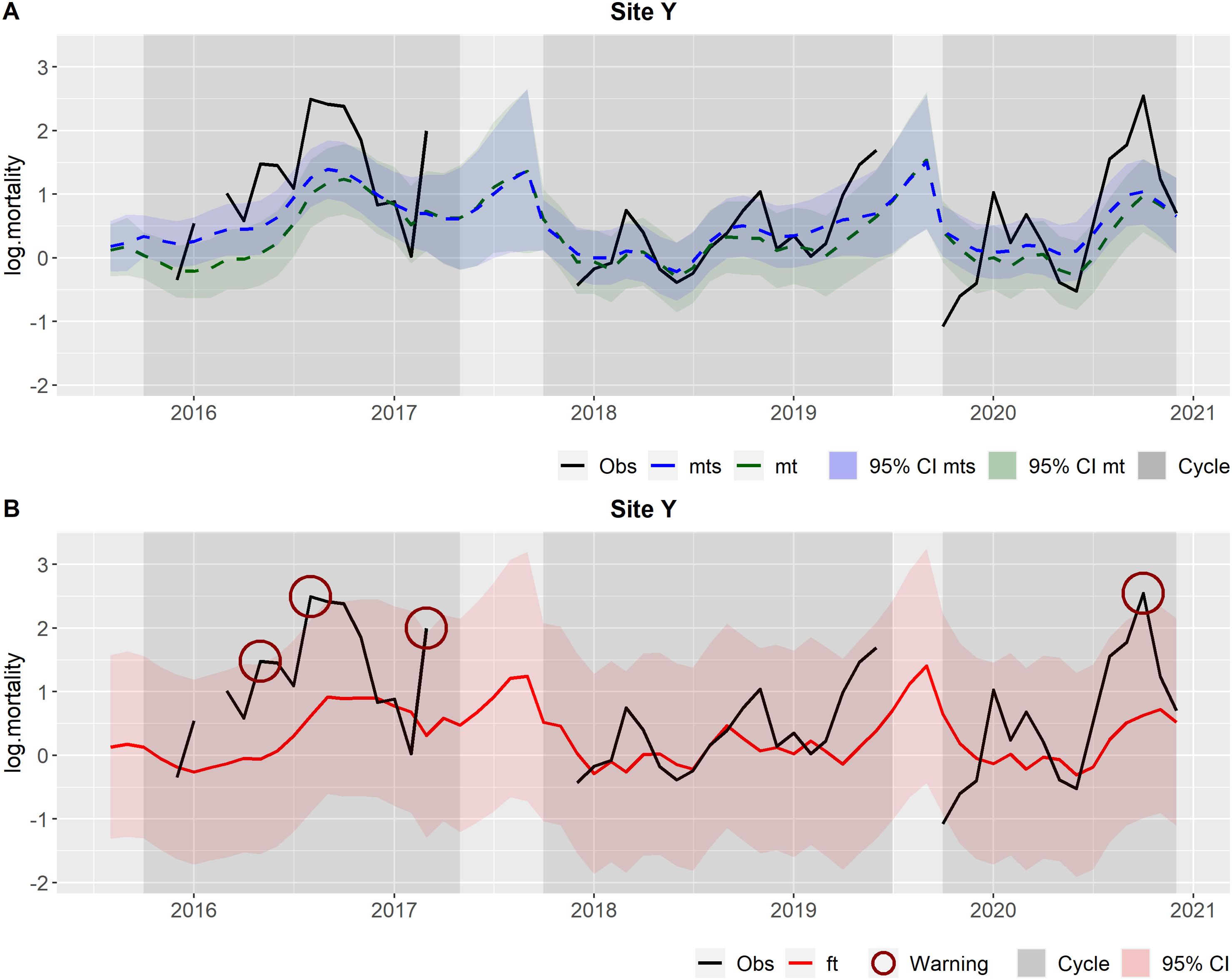
Figure 4. Outcomes from the hierarchical DLM at site level, for Site Y. Observations (obs) in black and the production cycles are represented by the grey shaded areas. (A) In green: Filtered mean (mt) and the respective 95% credible interval (CI); In blue: Smoothed mean (mts) and the respective 95% credible interval (CI). (B) In red: Forecasts (ft) and the respective 95% credible interval (CI); Circles: warnings.
Figure 4 illustrates the filtered and smoothed means (Figure 4A) and the forecasted values (Figure 4B), for Site Y. The observations exceeded the forecasts 95% CI in May and August 2016, March 2017 and October 2020, therefore these observations gave rise to warnings (Figure 4B).
At all levels - country, region and site - the estimates at the initial time steps should be interpreted with caution, given that the DLM was still adapting to the data, and the accuracy of these estimates may be limited during this initial phase.
3.3 Warnings
The fourth and last objective of this study was to generate warnings at site level when the observed mortality exceeded the expected levels. To ensure comparability with the warnings generated using the non-hierarchical models created in Merca et al. (2024), the warnings here were also assessed per production cycle. However, it is important to clarify that in this study the warnings were generated at site level. We could then identify the corresponding production cycle for each warning generated.
Between 2015 and 2020, 84 of the 353 production cycles experienced at least one warning. Of these 84 cycles, 70% had only one warning, 21% encountered two warnings, and 9% experienced three.
Of the 145 sites, 71 exhibited warnings, spanning all five regions. The region of Argyll and Bute had the highest occurrence, with 19 of 59 production cycles (32%) experiencing at least one warning, with a total of 28 warnings (Table 2). This was followed by the Highland region with 29% and Eilean Siar with 27%. The Orkney Islands region had the lowest number of warnings, with 5 production cycles each recording one warning out of 55 production cycles (9%), resulting in 5 total warnings, as shown in Table 2.
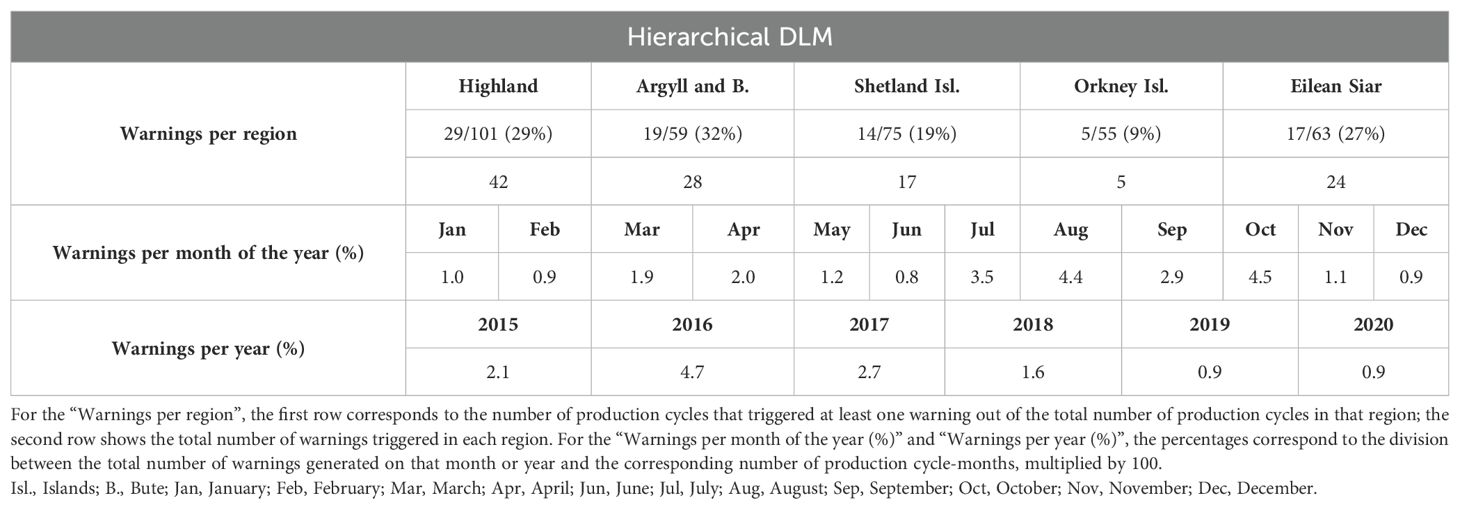
Table 2. Warnings identified in the hierarchical DLM between 2015 and 2020: per region, month of the year and year.
Regarding the months of the year (Table 2), there was an increase in March with 9 warnings out of 462 production cycle-months (1.9%). Similarly, April had a 2.0% occurrence rate. The months with the highest warning occurrences were July (3.5%), August (4.4%), September (2.9%), and October (4.5%).
Concerning the years (Table 2), 2016 had the highest rate with 42 warnings over 903 production cycle-months (4.7%). This was followed by 2017 with a 2.7% warning rate, 2015 with 2.1%, and 2018 with 1.6%. However, in 2015 only three months of data were available, making direct comparisons inapplicable.
4 Discussion
A hierarchical DLM was developed to monitor salmon mortality using open-source Scottish salmon data. The main goal was to evaluate whether this more complex modelling approach, which leverages the hierarchical data structure, offers better model precision compared to the simpler production cycle level DLMs (non-hierarchical) described in a previous study (Merca et al., 2024). Indeed, the hierarchical model outperformed the production cycle level DLMs, demonstrating that the additional modelling effort of accounting for the structure of the data confers a significant advantage. Similarly to the production cycle level models, the hierarchical DLM also exhibited a degree of uncertainty in the mortality predictions. Nevertheless, it was possible to trigger warnings at site level when the observed mortality exceeded the expected levels. An additional benefit of the hierarchical model was its ability to monitor mortality at country, region, and site levels. This information provided by the hierarchical model can be particularly valuable for various stakeholders as part of a monitoring system, offering insights into mortality trends at multiple levels, while also providing warnings at site level when mortality is higher than expected.
Using open-source data offers numerous advantages, particularly in the context of hierarchical modelling. Monitoring production at higher levels, such as a country or region, typically involves aggregating information from various companies operating in different locations. Openly available datasets simplify the creation of comprehensive models by facilitating data integration across these entities. Open-source datasets eliminate the need for complicated and time-consuming data sharing agreements between companies, thereby reducing administrative complexities and fostering a more efficient process. This accessibility not only accelerates innovation but also ensures transparency and reproducibility of the methods.
The idea behind creating a hierarchical DLM aimed to reflect the inherent hierarchical structure present in the data. It is typically anticipated that a relationship among the responses of observations within a group exists, as the shared feature tends to make the outcomes more similar than they would be otherwise (Dohoo et al., 2014). In a previous study, we developed production cycle level DLMs (Merca et al., 2024), which modelled each production cycle individually, without considering correlations between production cycles within the same site or region. In the current study, we incorporated the data hierarchy, encompassing a country level, grouped into five different regions, with each region containing several sites. Accounting for this hierarchy has proven beneficial in monitoring salmon mortality, justifying the development of this more complex modelling approach.
The hierarchical DLM provided valuable outcomes: (1) it enabled the monitoring of mortality at various levels, and (2) it triggered site level warnings when mortality exceeded the expected levels. Regarding the first outcome, we were able to calculate estimates for country, region, and site levels over time (using data collected at site level) offering critical insights into mortality trends. This information can be valuable for various stakeholders, including health authorities, in understanding trends that may benefit from strategic resource management practices at regional or national levels. Two types of estimates were generated at country, regional, and site levels: filtered and smoothed values. It should be noted that the smoothed values, obtained through retrospective analysis, are not suitable for real-time monitoring and are therefore only relevant for retrospective assessments. In turn, filtered values are best suited for real-time analysis, and could be implemented as an industry-wide mortality monitoring model. Concerning the second outcome, triggering warnings when observed mortality significantly exceeds the expected levels is an important feature of monitoring systems. These warnings can alert stakeholders, such as salmon producers or authorities, to investigate the causes of excessive mortality. The models are based on monthly mortality that is collated by a government body. Therefore, the outputs are at least one or two months behind real-time observations, and thus results of our model are too late to be of use for immediate mitigative actions. However, increasing warnings, or unexplained warnings, can point at a need for health management issues or emerging diseases that may have been otherwise left unnoticed for longer. This could save the industry time, money and resources, and improve its sustainability.
Warnings were generated at site level when the observed mortalities exceeded the 95% CI of the forecasts, calculated as shown in Equation 7. A warning indicates that mortality on that site was significantly higher than expected for that particular time step. The application of the hierarchical model revealed that approximately 25% of the production cycles and 50% of the sites encountered at least one warning between 2015 and 2020. When compared to the production cycle level DLMs (Merca et al., 2024), the hierarchical DLM produced fewer warnings overall but the warnings were coherent across models. This can be beneficial because stakeholders in the agricultural sector emphasize the necessity for reliability and robustness to justify the utilization of the models and mitigate distrust in technology (Kaler and Ruston, 2019; Kopler et al., 2023).
Geographically, the region of Argyll and Bute experienced the highest warning rate (32%), followed by Highland (29%), Eilean Siar (27%), Shetland Islands (19%) and Orkney Islands (9%). In both univariate and multivariate production cycle level DLMs developed in the previous study, the region of Orkney Islands also had the lowest warning rate (11% on both cases), but Eilean Siar had the highest (44% and 40%, respectively). This difference might be due to the consideration of the region each site belongs to, which influenced the expected mortality, and therefore the warnings generated. By accounting for the region associated with each site, the hierarchical DLM can better estimate the expected mortalities, reflecting the regional variations.
In terms of seasonality, most warnings triggered by the hierarchical DLM occurred during the summer and autumn months. This is in accordance with what was found in the production cycle level DLMs. During summer, rising water temperatures increase salmon metabolic rates, escalating oxygen demand. Simultaneously, warmer water temperatures lead to diminishing dissolved oxygen levels in water (Noble et al., 2018). Additionally, warm water fosters the proliferation of most of the currently relevant infectious agents and parasites such as amoebic gill disease and sea lice (Oldham et al., 2016; Brooker et al., 2018; Murray et al., 2022), and non-infectious harmful agents such as micro jellyfish and phytoplankton (Boerlage et al., 2020). No open-source data about the cause(s) of excessive mortalities were available during the full study period, limiting our ability to incorporate these factors into the models. During the summer, the model already expects higher mortalities due to the incorporation of a seasonal pattern at country level within the hierarchical framework, which prevents the generation of warnings for these already expected increases. However, the model indicates that the instances of higher-than-expected mortalities, which do trigger warnings, are also more frequent during this period.
The distribution of warnings from 2015 to 2020 was also similar between the hierarchical DLM and the production cycle level models. For all models (since the same mortality data were used) the year 2015 only had three months of data available, making direct comparisons not applicable. The year 2016 continued as the one with the highest warning rate, followed by a declining trend until 2020. The reasons for the declining trend are unclear; however, it is plausible that they may be attributed to potentially less precise mortality estimates during the initial time steps of the modelling process, leading to overestimations of warnings in 2016. Such an effect would not occur in later years when the model has been running for longer.
In all three models here compared (hierarchical, univariate production cycle level, and multivariate production cycle level DLMs), the warnings were defined as any observation exceeding the 95% credible interval. The method chosen was applied to all three types of DLMs to ensure comparability of warnings across different models. Alternative methods for generating warnings from DLM outputs could have been used, as discussed in Merca et al. (2024).
The residuals of a DLM should follow a normal distribution, as long as the system remains as expected (West and Harrison, 1997). The original mortality data resulted in non-normally distributed residuals. Thus, we applied a logarithmic transformation to meet normality assumptions. The results can still be interpreted on the original data scale. The decision of using DLMs was previously discussed in Merca et al. (2024). To ensure methodological consistency, all models compared utilized the same logarithmically transformed mortality data.
Similarly to the production cycle level DLMs developed in the previous study (Merca et al., 2024), the mortality forecasts derived from the hierarchical model also exhibited wide 95% credible intervals. This may be attributed to the absence of health, management, and fish movement data. Ideally, models should be trained with data representing “normal” mortality to establish baseline patterns, as conducted by Jensen et al. (2016). However, the absence of health and management related information in the mortality dataset made it impossible to distinguish normal from abnormal mortality. Salmon producers collect these data internally, but they only report it to government and to the salmon producers’ organization (Salmon Scotland) when mortality reaches a specific threshold. These data have only become openly available recently, and therefore could not be incorporated in the entire timeframe of our study. Additionally, the movement of fish among sites, a common practice among producers, may have not been entirely captured in our analysis, despite our attempts detailed in section 2.2. For a more detailed exploration of potential sources of uncertainty, refer to Merca et al. (2024).
To address these uncertainties, future models could incorporate data on the reasons for mortality and salmon movements. However, salmon movements’ data are not openly available. Additionally, including mortality data over shorter time periods, such as weekly intervals, would likely improve the monitoring process.
5 Conclusion
Accounting for the hierarchical structure of the data (country, region, and site) is beneficial in monitoring salmon mortality, outperforming the production cycle level DLMs developed in a previous study. This hierarchical approach enabled us to monitor mortality at country, region, and site levels, providing additional valuable insights into the mortality trends. Moreover, we could provide warnings at site level when the observed mortality exceeded the expected levels, which can contribute to the detection of management issues or emerging diseases. However, some uncertainty was found in the mortality predictions. Our exclusive reliance on open-source data has enabled the development of an industry-wide mortality monitoring model, providing additional value to already existing data, and eliminating the need for complex data sharing agreements.
Data availability statement
Publicly available datasets were analyzed in this study. This data can be found here: https://aquaculture.scotland.gov.uk/.
Author contributions
CM: Writing – original draft, Visualization, Software, Methodology, Investigation, Formal analysis, Data curation, Conceptualization. AB: Writing – review & editing, Supervision, Resources, Methodology, Conceptualization. AK: Writing – review & editing, Supervision, Software, Resources, Methodology, Funding acquisition, Formal analysis, Conceptualization. DJ: Writing – review & editing, Supervision, Software, Resources, Methodology, Funding acquisition, Formal analysis, Conceptualization.
Funding
The author(s) declare financial support was received for the research, authorship, and/or publication of this article. This work has received funding from the European Union’s Horizon 2020 research and innovation programme under grant agreement No. 101000494 (DECIDE).
Conflict of interest
The authors declare that the research was conducted in the absence of any commercial or financial relationships that could be construed as a potential conflict of interest.
The author(s) declared that they were an editorial board member of Frontiers, at the time of submission. This had no impact on the peer review process and the final decision.
Publisher’s note
All claims expressed in this article are solely those of the authors and do not necessarily represent those of their affiliated organizations, or those of the publisher, the editors and the reviewers. Any product that may be evaluated in this article, or claim that may be made by its manufacturer, is not guaranteed or endorsed by the publisher.
Author disclaimer
This document reflects only the author’s view and the Research Executive Agency (REA) and the European Commission cannot be held responsible for any use that may be made of the information it contains.
References
Boerlage A. S., Ashby A., Herrero A., Reeves A., Gunn G. J., Rodger H. D. (2020). Epidemiology of marine gill diseases in Atlantic salmon (Salmo salar) aquaculture: a review. Rev. Aquac. 12, 2140–2159. doi: 10.1111/raq.12426
Bono C., Cornou C., Kristensen A. R. (2012). Dynamic production monitoring in pig herds I: Modeling and monitoring litter size at herd and sow level. Livestock Sci. 149, 289–300. doi: 10.1016/j.livsci.2012.07.023
Brooker A. J., Skern-Mauritzen R., Bron J. E. (2018). Production, mortality, and infectivity of planktonic larval sea lice, Lepeophtheirus salmonis (Kroye): Current knowledge and implications for epidemiological modelling. ICES J. Mar. Sci. 75, 1214–1234. doi: 10.1093/icesjms/fsy015
Department for Environment, Food & Rural Affairs (2023).Chapter 13: Overseas trade. In: National statistics: Agriculture in the United Kingdom 2022. Available online at: https://www.gov.uk/government/statistics/agriculture-in-the-united-kingdom-2022/chapter-13-overseas-trade (Accessed March 1, 2024).
Dohoo I., Martin W., Stryhn H. (2014). Veterinary Epidemiologic Research. 2nd Edn (Charlottetown, Prince Edward Island, Canada: VER). Available at: www.upei.ca/ver.
Dominiak K. N., Hindsborg J., Pedersen L. J., Kristensen A. R. (2019a). Spatial modeling of pigs’ drinking patterns as an alarm reducing method II. Application of a multivariate dynamic linear model. Comput. Electron. Agric. 161, 92–103. doi: 10.1016/j.compag.2018.10.037
Dominiak K. N., Pedersen L. J., Kristensen A. R. (2019b). Spatial modeling of pigs’ drinking patterns as an alarm reducing method I. Developing a multivariate dynamic linear model. Comput. Electron. Agric. 161, 79–91. doi: 10.1016/j.compag.2018.06.032
Fagerland M. W. (2012). t-tests, non-parametric tests, and large studies-a paradox of statistical practice? BMC Med. Res. Methodol. 12:78. doi: 10.1186/1471-2288-12-78
FAO (2022). The State of World Fisheries and Aquaculture 2022. Towards Blue Transformation (Rome: FAO). doi: 10.4060/cc0461en
Hubley P. B., Gibson A. J. F. (2011). A model for estimating mortality of Atlantic salmon, Salmo salar, between spawning events. Can. J. Fish. Aquat. Sci. 68, 1635–1650. doi: 10.1139/f2011-074
Iversen A., Asche F., Hermansen Ø., Nystøyl R. (2020). Production cost and competitiveness in major salmon farming countries 2003–2018. Aquaculture 522, 735089. doi: 10.1016/j.aquaculture.2020.735089
Jensen D. B., Hogeveen H., De Vries A. (2016). Bayesian integration of sensor information and a multivariate dynamic linear model for prediction of dairy cow mastitis. J. Dairy Sci. 99, 7344–7361. doi: 10.3168/jds.2015-10060
Kaler J., Ruston A. (2019). Technology adoption on farms: Using Normalisation Process Theory to understand sheep farmers’ attitudes and behaviours in relation to using precision technology in flock management. Prev. Vet. Med. 170, 104715. doi: 10.1016/j.prevetmed.2019.104715
Kopler I., Marchaim U., Tikász I. E., Opaliński S., Kokin E., Mallinger K., et al. (2023). Farmers’ Perspectives of the benefits and risks in precision livestock farming in the EU pig and poultry sectors. Animals 13, 2868. doi: 10.3390/ani13182868
Lecomte J. B., Laplanche C. (2012). A length-based hierarchical model of brown trout (Salmo trutta fario) growth and production. Biom. J. 54, 108–126. doi: 10.1002/bimj.201100083
Merca C., Boerlage A. S., Kristensen A. R., Jensen D. B. (2024). Monitoring monthly mortality of maricultured Atlantic salmon (Salmo salar L.) in Scotland I. Dynamic linear models at production cycle level. Front. Mar. Sci. 11. doi: 10.3389/fmars.2024.1436755
Munro L. A. (2023). Scottish fish farm production survey 2022 (Edinburgh: Scottish Government). Available online at: https://www.gov.scot/publications/scottish-fish-farm-production-survey-2022/pages/5/ (Accessed February 5, 2024).
Murray A., Falconer L., Clarke D., Kennerley A. (2022). Climate change impacts on marine aquaculture relevant to the UK and Ireland. MCCIP Sci. Rev. 2022, 18. doi: 10.14465/2022.reu01.aqu
Noble C., Gismervik K., Iversen M. H., Kolarevic J., Nilsson J., Stien L. H., et al. (2018). Welfare Indicators for farmed Atlantic salmon: tools for assessing fish welfare. Available online at: www.nofima.no/fishwell/english (Accessed February 24, 2023).
Oldham T., Rodger H., Nowak B. F. (2016). Incidence and distribution of amoebic gill disease (AGD) - An epidemiological review. Aquaculture 457, 35–42. doi: 10.1016/j.aquaculture.2016.02.013
Posit team (2022). RStudio: Integrated Development for R (Boston, MA: RStudio, PBC). Available at: http://www.rstudio.com/.
R Core Team (2022). R: A language and environment for statistical computing (Vienna, Austria: R Foundation for Statistical Computing). Available at: https://www.R-project.org/.
Rustas B. O., Persson Y., Ternman E., Kristensen A. R., Stygar A. H., Emanuelson U. (2024). The evolutionary operation framework as a tool for herd-specific control of mastitis in dairy cows. Livestock Sci. 279, 105390. doi: 10.1016/j.livsci.2023.105390
Scheuerell M. D., Buhle E. R., Semmens B. X., Ford M. J., Cooney T., Carmichael R. W. (2015). Analyzing large-scale conservation interventions with Bayesian hierarchical models: A case study of supplementing threatened Pacific salmon. Ecol. Evol. 5, 2115–2125. doi: 10.1002/ece3.1509
Skjølstrup N. K., Lastein D. B., de Knegt L. V., Kristensen A. R. (2022). Using state space models to monitor and estimate the effects of interventions on treatment risk and milk yield in dairy farms. J. Dairy Sci. 105, 5870–5892. doi: 10.3168/jds.2021-21408
Sommerset I., Wiik-Nielsen J., Moldal T., Henrique Silva de Oliveira V., Christine Svendsen J., Haukaas A., et al. (2024). Fiskehelserapporten 2023. Available online at: https://www.vetinst.no/rapporter-og-publikasjoner/rapporter/2024/fiskehelserapporten-2023 (Accessed May 27, 2024). Veterinærinstituttet rapportserie nr 8a/2024.
Stebbins E., Bence J. R., Brenden T. O., Hansen M. J. (2024). A hierarchical model of persistent and transient growth variation applied to Lake Superior lake trout. Fish. Res. 278, 107081. doi: 10.1016/j.fishres.2024.107081
Stygar A. H., Krogh M. A., Kristensen T., Østergaard S., Kristensen A. R. (2017). Multivariate dynamic linear models for estimating the effect of experimental interventions in an evolutionary operations setup in dairy herds. J. Dairy Sci. 100, 5758–5773. doi: 10.3168/jds.2016-12251
Torrissen O., Olsen R. E., Toresen R., Hemre G. I., Tacon A. G. J., Asche F., et al. (2011). Atlantic salmon (Salmo salar): the “Super-chicken” of the sea? Rev. Fisheries Sci. 19, 257–278. doi: 10.1080/10641262.2011.597890
Keywords: salmon, mortality, multi-level model, hierarchical model, dynamic linear models, aquaculture, open-source data, warnings
Citation: Merca C, Boerlage AS, Kristensen AR and Jensen DB (2024) Monitoring monthly mortality of maricultured Atlantic salmon (Salmo salar L.) in Scotland II. A hierarchical dynamic linear model. Front. Mar. Sci. 11:1483796. doi: 10.3389/fmars.2024.1483796
Received: 20 August 2024; Accepted: 27 September 2024;
Published: 30 October 2024.
Edited by:
Yngvar Olsen, NTNU, NorwayReviewed by:
Ioannis A. Giantsis, Aristotle University of Thessaloniki, GreeceShengjie Ren, Queensland University of Technology, Australia
Copyright © 2024 Merca, Boerlage, Kristensen and Jensen. This is an open-access article distributed under the terms of the Creative Commons Attribution License (CC BY). The use, distribution or reproduction in other forums is permitted, provided the original author(s) and the copyright owner(s) are credited and that the original publication in this journal is cited, in accordance with accepted academic practice. No use, distribution or reproduction is permitted which does not comply with these terms.
*Correspondence: Carolina Merca, Y21nbUBzdW5kLmt1LmRr