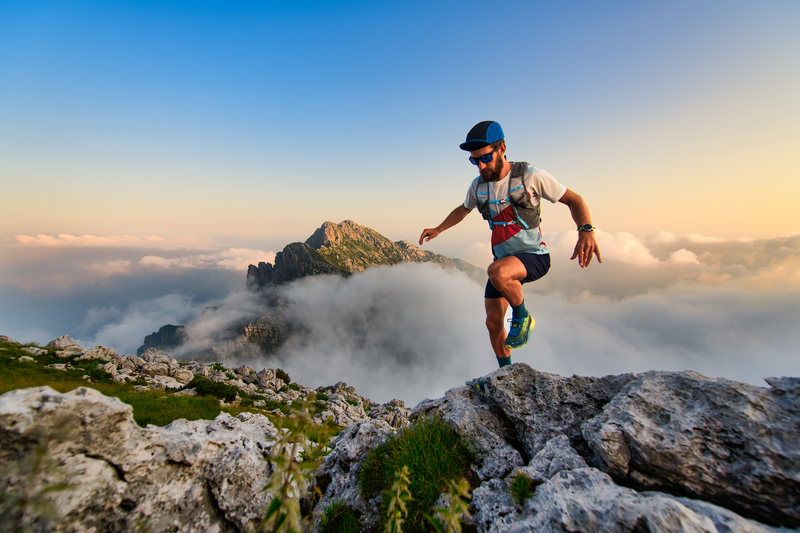
95% of researchers rate our articles as excellent or good
Learn more about the work of our research integrity team to safeguard the quality of each article we publish.
Find out more
REVIEW article
Front. Mar. Sci. , 25 October 2024
Sec. Ocean Observation
Volume 11 - 2024 | https://doi.org/10.3389/fmars.2024.1458036
This article is part of the Research Topic Demonstrating Observation Impacts for the Ocean and Coupled Prediction View all 18 articles
Ocean observing systems in coastal, shelf and marginal seas collect diverse oceanographic information supporting a wide range of socioeconomic needs, but observations are necessarily sparse in space and/or time due to practical limitations. Ocean analysis and forecast systems capitalize on such observations, producing data-constrained, four-dimensional oceanographic fields. Here we review efforts to quantify the impact of ocean observations, observing platforms, and networks of platforms on model products of the physical ocean state in coastal regions. Quantitative assessment must consider a variety of issues including observation operators that sample models, error of representativeness, and correlated uncertainty in observations. Observing System Experiments, Observing System Simulation Experiments, representer functions and array modes, observation impacts, and algorithms based on artificial intelligence all offer methods to evaluate data-based model performance improvements according to metrics that characterize oceanographic features of local interest. Applications from globally distributed coastal ocean modeling systems document broad adoption of quantitative methods, generally meaningful reductions in model-data discrepancies from observation assimilation, and support for assimilation of complementary data sets, including subsurface in situ observation platforms, across diverse coastal environments.
At the interface between the land margin and open ocean, coastal waters support a wide variety of societal benefits, including economic, recreational, and ecological services. Local physical and biogeochemical dynamics result in their sizable contributions to the Earth System including climate relative to their spatial area. To support local societal benefits and broader scientific understanding, regional and coastal ocean observing systems have developed around the globe, generally consisting of a suite of continuously operating in situ and remotely sensed ocean observations. Dynamical and statistical models have been developed to capitalize on these data streams to provide historical, near real-time, and forecast information to local and national communities, resource managers, and scientists. Because specific coastal priorities and financial constraints vary with region, the type and density of observing platforms as well as details of ocean model and configuration are diverse.
Platforms collecting remotely sensed data for physical variables (temperature, salinity, velocity, and sea level) that are routinely assimilated in the coastal and regional ocean forecast systems include satellite observations of sea surface temperature (SST) and sea surface height (SSH), as well as high frequency radar (HFR) estimates of surface currents. In addition, satellite observations of sea surface salinity (SSS) have recently become available. In situ assets include coastal tide gauges, Argo floats and gliders equipped with temperature and salinity sensors, as well as moorings that also may include velocity observations. Unique observational challenges limit spatial coverage in coastal environments (for example, with land corruption of the radar reflection used for satellite sea level anomaly calculations and shallow bathymetry for autonomous in situ platforms), particularly close to the coast where spatial and temporal scales decrease.
The challenge of effectively observing coastal waters given the scales of oceanic variability combined with the large expense of in situ assets motivates analyses to assess the value of observations, instrument platforms, and the overall observation system. Routine monitoring offers direct benefit when particular observations provide actionable data to stakeholders (e.g., with wave information). Additional, broader benefits derive from models that yield full four-dimensional representations of the ocean state whose output can be analyzed for multiple stakeholder needs.
While ocean observations deliver sparse coverage of ocean conditions relative to spatial scales of natural variability, dynamical state-of-the-art, coastal models are generally constructed at O(1-10 km) grid spacing that resolves mesoscale and a portion of submesoscale motions. Despite limited coverage, ocean observations provide invaluable information, critical for initial tuning of the model configuration and ongoing evaluation. In addition, such observations formally constrain data assimilative (DA) models that adjust model control parameters (e.g., state variables and forcing fields) to produce more accurate estimates of the overall ocean state than occur with free running models (Edwards et al., 2015). Improved, DA-derived ocean state estimates supply initial conditions for skillful forecasts, often of greater interest to key stakeholders.
Methods to quantify the impact of observations on model solutions have been developed, and it is the subject of this paper to review these efforts with examples from coastal, shelf, and marginal sea systems. Following Robinson et al. (2004) and Kourafalou et al. (2015), we adopt a broad view of these environments, defined as domains influenced by nearshore, shelf, or shelf-break processes as well as, potentially, open ocean forcing. This contribution updates previous accounts (Oke et al., 2015; De Mey-Frémaux et al., 2019) as numerous investigations have emerged as well as novel methods. We emphasize projects that are linked to the Coastal and Shelf Seas Task Team, part of OceanPredict, an “international network and science programme that facilitates knowledge exchange between scientists and experts of operational oceanography from around the world to accelerate, strengthen and increase the impact of ocean prediction” 1. Although the ocean state broadly consists of physical and other information (e.g., related to biogeochemistry, water quality, fisheries), we focus here on efforts in ocean circulation physics as many benefits derive exclusively from these fields, and these systems are generally prerequisites for multi-disciplinary systems. We begin in Section 2 with a discussion of general issues that arise with quantitative observation impact studies, recognizing that they must be considered in practice, regardless of model domain (open ocean or smaller). Section 3 then offers specific examples of observation impact studies spanning a range of complementary methods, primarily from regional, coastal, and shelf sea modeling systems. Section 4 concludes with a brief discussion of commonalities, recommendations, and future opportunities.
In this section, we introduce notation and issues that naturally arise when quantitatively comparing ocean model output to observations. We note that these issues are general, applying equally well to open ocean and coastal environments.
Data assimilation involves comparing observations measured by a variety of diverse sensors with their model counterparts. In most modern data assimilation schemes, such comparison is performed in observation space, meaning that a function, potentially non-linear, must be formulated to project the model state variables onto the observation space. Such a function is commonly referred to as an “observation operator”, and it is indicated by H(x), where x represents the ocean state vector. Projecting the ocean state vector into observation space is typically much simpler than the reverse, allowing optimal inversion algorithms (e.g., Lorenc, 1986). Observation operators are formulated according to our knowledge of the relationship between the ocean state and the observable measurement.
The formulation of the operators H(x) for each observation type is a common requirement of both sequential (extended and ensemble Kalman filters and all its variants) and variational methods, the latter requiring, additionally, the derivation of the tangent-linear and adjoint versions of H(x), denoted usually by H and HT respectively (see e.g. Ide et al., 1997), which are required for efficient minimization in the quadratic cost-function framework (Talagrand and Courtier, 1987). These operators are derived from Taylor expansions truncated at the first order, where the derivative of H(x) is evaluated around the background (prior) ocean state (Errico, 1997), usually a forecast initialized from a previous assimilation cycle.
The observation operator can be as simple as a linear interpolation operator, for instance when the observed variable is also a model state variable. In other cases, it can be quite complex, for instance including a radiative transfer model in the case of satellite observations of sea-ice concentration from passive microwave sensors in brightness temperature space (Scott et al., 2012), which relates the oceanic and atmospheric states to the reflected radiance measured by the satellite. Another notable example concerns underwater acoustic measurements, which in turn require an underlying acoustic propagation model - with variable complexity - to provide a relationship between the ocean state and the observations through the effects that underwater sound speed (and currents) have on the acoustic propagation itself (Ngodock et al., 2017; Storto et al., 2020).
During the last few years, with the rise of deep learning algorithms in geoscientific applications, observation operators have benefited from these data-driven formulations. Radiative transfer models (Liang et al., 2023), acoustic observations (Storto et al., 2021), and, in general, observations that cannot be formulated analytically from the ocean state, are examples where deep learning algorithms can improve observation operators and, in turn, data assimilation.
In the Bayesian approach to data assimilation, the process of model-data comparison and model update necessitates knowledge or assumptions about the error characteristics or uncertainty properties of the observed value and prior estimate. One challenge encountered is that the discrete geophysical model cannot capture all spatial and temporal scales of the observed geophysical state. Consequently, the prior estimate may differ significantly from the observed value, even in the absence of any measurement or instrument error, resulting in a perceived error that must be taken into account to update the prior estimate accurately. For instance, a perfect (error free measurement) observation of the temperature gradient across an oceanic front may be much higher than the forecast value from a numerical prediction model, resulting in a misfit that must be estimated somehow. The discrepancy between the modeled representation of a quantity and its actual observation has traditionally been addressed by incorporating what is commonly referred to as representation, representativity, or representativeness errors in scholarly works (Janjić et al., 2018). Consequently, the observation error generally comprises two distinct elements, the measurement error and the representation error.
In variational schemes that rely on the tangent-linear approximation, an additional component of the representation error derives from the tangent-linear assumption: the more non-linear the H(x) function, the larger the error associated with its tangent-linearization (Errico et al., 1993). While there are several possible remedies to reduce the impact of the tangent-linear assumption – for instance increasing the number of outer loops in variational schemes, and thus linearizing H(x) around an increasingly improved approximation of the analysis – the tangent-linear error cannot be eliminated, unless the observation operator is already linear (e.g., in the case of linear interpolation schemes).
Representation errors are in general dominant in the observation error budget (Oke and Sakov, 2008), and, beyond possible spatial representativeness issues discussed earlier, may be relevant when the numerical ocean model is inadequate in representing certain processes. For example, if data includes high frequency information such as tidal processes or inertial oscillations that are not represented in the model, low-pass filtering can be applied prior to model-data comparison. Oke et al. (2002) apply a 40-hour half-amplitude filter to observations and model output for quantitative comparison in a model off the Oregon coast without tides. Yu et al. (2012), Pasmans et al. (2020) and Hernandez-Lasheras et al. (2021) assimilated daily mean HFR surface current estimates into coastal ocean circulation models to focus improvements on subtidal circulation features.
Given that high-resolution, in-situ measurements are expensive to deploy and maintain, the best use of remotely sensed data is crucial (Le Traon, 2011; Oke et al., 2015). Several methods aimed at enhancing how sea surface measurements from satellite-borne sensors correct and constrain the sub-surface ocean model solution have been developed. A significant example is the assimilation of daytime sea surface temperature (SST) retrievals at high temporal frequency, which has the potential to correct possible mixed layer depth biases (Miyazawa et al., 2017; Liu and Fu, 2018), with obvious benefits for several oceanographic applications (Liu and Fu, 2018). However, several SST observational products from infrared or microwave sensors have not been widely used in operational contexts because surface layer diurnal variability in OGCMs is difficult to model, with additional difficulties, in turn, defining H and the observational error. For instance, infrared sensors (the Advanced Very High Resolution Radiometer, AVHRR, or the Spinning Enhanced Visible and Infrared Imager, SEVIRI) measure the skin temperature valid at a depth of approximately 10 μm. On the other hand, microwave sensors (the Advanced Microwave Scanning Radiometer 2, AMSR-2) measure the sub-skin temperature at a depth of around 1 mm.
In contrast, SST analyses such as the Operational Sea Surface Temperature and Ice Analysis (OSTIA) (Donlon et al., 2012) or NOAA OIv2 (Optimum Interpolation, version 2, Reynolds et al., 2002) provide the foundation SST, which is nominally at a depth of 10 m. The 10 m depth is considered as a reference to ensure that the temperature is not influenced by the diurnal cycle signal, thus significantly simplifying the formulation of the observation operator. Between 0.2 m and 1 m depth, where the first level of most OGCMs is located, the diurnal cycle of SST is damped compared to that of the skin or sub-skin SST and this discrepancy has the potential to introduce systematic errors in the analyses. Common remedies to this discrepancy rely on i) bias-correcting the skin-SST through the use of a set of bias predictors (Petrenko et al., 2016; Storto and Oddo, 2019); ii) using statistical tools, such as canonical correlations, to infer the cool skin and warm layer amplitudes (e.g., Jansen et al., 2019); or iii) modeling analytically the skin SST variations through parameterizing their difference with respect to the foundation SST (While et al., 2017; Pimentel et al., 2019). Recently, de Toma et al. (2024) successfully reduced skin temperature biases in a regional model of the Mediterranean Sea by spatially and temporally varying the depth of the warm layer, deduced from chlorophyll concentration data.
For over twenty years, satellite altimetry has significantly advanced our understanding of ocean dynamics by consistently providing information on both mesoscale and fine-scale dynamics (Fu et al., 2010; Morrow et al., 2017) as well as the overall circulation at basin scales (Stammer, 1997). As a result, global (Verrier et al., 2017) and regional (Pujol et al., 2010) operational analysis and forecast systems are profoundly impacted by altimetry observations and serve as a vital complement to in situ observation profiles (Storto et al., 2013). However, assimilating altimetry observations presents several challenges mostly related to the multivariate balances used to project the altimetry innovations in the subsurface, the spatial scales and dynamical regime of the study region, and technical considerations such as the computational costs of the assimilation scheme. Furthermore, as discussed above, characterizing observation errors is generally difficult due to the need to assess not only instrumental errors but also representation errors and those associated with geophysical corrections (e.g., Storto et al., 2011).
To facilitate the formulation and minimization of the cost function, most variational data assimilation systems assume that there is no correlation between errors in pairs of observations. This assumption allows the observation-error covariance matrix to be defined as diagonal, simplifying the implementation of the variational algorithm and the preconditioning step, needed to improve the speed of convergence in the minimization process. However, the validity of this assumption may be questioned when considering high-resolution satellite observations. In fact, satellite altimetry data undergo various geophysical corrections, such as tropospheric, ionospheric, and tidal corrections (Chelton et al., 2001). These corrections, along with instrumental errors, can support relaxing the assumption of uncorrelated altimetry observational errors. High-resolution regional scale analysis systems may be more affected by this assumption compared to coarse-resolution systems, especially when their resolution exceeds that of the satellite data. To address this issue, common approaches include combining satellite data into so-called super observations (e.g., Oke et al., 2008), data thinning (Cummings, 2005), and adjusting or inflating observational errors (Liu and Rabier, 2003; Rainwater et al., 2015).
Previous attempts at incorporating altimetry error correlations have shown promise. Brankart et al. (2009, 2010) demonstrated that by applying a linear transformation to the observation vector and augmenting it with gradient observations, error correlations can be introduced, resulting in improved ocean circulation in regional prediction systems. Le Hénaff et al. (2008), Ruggiero et al. (2016) and Yaremchuk et al. (2018) explored different approaches to investigate the optimal assimilation of Surface Water and Ocean Topography (SWOT) simulated altimetry data including observational error correlation. Furthermore, Storto et al. (2019) used co-located glider trajectories and altimetry tracks to estimate the altimetry correlation length scale and used it in the definition of the off-diagonal elements of the altimetry error covariance matrix, resulting in significant improvements in forecast skill scores compared to the case when the matrix is diagonal. In addition to confirming the analysis improvement deriving from the introduction of spatially correlated observational errors, the latest study also strengthens the relevance of properly designed observational networks.
How observations impact ocean model estimates must be defined in terms of metrics that quantify one or several aspects of the ocean state. Any one specific measure is necessarily subjective, reflecting ocean qualities that practitioners or, perhaps, stakeholders deem critical. Sometimes, metrics represent model-data misfit against independent observations not included in the assimilation system. In these cases, misfit reduction resulting from inclusion of particular observations or observation platforms establishes the positive impact the observations have on model fidelity. In other cases, metrics represent unobserved features of value in a particular oceanographic context. In these circumstances, impact is represented by the magnitude of metric change resulting from included observations. Examples of metrics used include average eddy kinetic energy (EKE; Röhrs et al., 2018; Gwyther et al., 2023b), average sea surface temperature (Moore et al., 2017), measures of stratification such as the depth of an isopycnal surface (Moore et al., 2017; Röhrs et al., 2018) or thermocline, available potential energy, upper ocean heat content (Halliwell et al., 2015; Gwyther et al., 2023a), and volume transport (Kerry et al., 2018; Siripatana et al., 2020; Christensen et al., 2018; Levin et al., 2020). Additionally region specific metrics can be used to ensure important dynamics are well represented, for example the separation latitude of a western boundary current (Gwyther et al., 2022). When used by multiple, independent, analysis systems in a particular oceanographic context, any reduction in metric spread by ensemble members when assimilating particular observations suggests convergence in data-constrained state estimates and a positive impact of those observations on ocean analyses (Storto et al., 2013).
We note that observation impact can depend on user choices in the assimilation system. Liu and Hirose (2022) demonstrate using model twin experiments in a regional domain of southwest Japan that while adjustment of surface forcing through assimilation of satellite altimetry is effective in shallow regions, its impact does not extend to deep regions off the shelf. They find improvement in estimates of Kuroshio Current properties result instead by allowing assimilation-induced updates to the lateral boundary conditions.
A first demonstration of the impact of particular observations on an ocean state estimate focuses on the Norwegian shelf, set up by the Norwegian Meteorological Institute (MET Norway) (Röhrs et al., 2018). The model domain includes the Skagerrak in the southeast, the northern parts of the North Sea, the Shelf sea off western Norway including the shelf slope, and parts of the Barents Sea in the north. The NorShelf model is based on the Regional Ocean Modeling System (ROMS; Shchepetkin and McWilliams, 2005; Haidvogel et al., 2008) with a physical space 4D Variational (4D-Var) DA scheme. A horizontal model resolution of 2.4 km was chosen to suit the scale of the available observations, and to satisfy competing needs to resolve high resolution eddy dynamics while confining nonlinearities that limit the 4D-Var DA capabilities. The model is used as a forecasting tool for ocean circulation and hydrography beyond the coastal area, including the entire shelf sea and the dynamics of the North Atlantic current at the shelf slope. Some model cycles were found to benefit locally from dense observation campaigns (e.g., when CTD sections are taken from research vessels or during glider campaigns). These types of observation have significant impact on the model trajectory, with a stronger response in deeper layers compared to periods when only SST fields are assimilated. All model state variables (i.e., temperature, salinity, velocity, and the free surface) are adjusted, and typically an increase in eddy kinetic energy is seen in the mixed layer, along with modifications to vorticity that suggests a repositioning of mesoscale eddies.
The impact of observations toward improved estimates of the ocean state may be evaluated through straightforward experiments that compare DA runs with specific observations removed/denied. Such Observing System Experiments (OSEs) can range in configuration from withholding all observations, and thus evaluating the impact of the overall observing system relative to a non-DA approach, to withholding only particular sets of observations or observation platforms, or for comparing DA schemes themselves. Here we present several examples to illustrate their application in coastal and shelf seas.
One example illustrates the application of OSEs toward parts of observational programs. The Korean National Institute of Fisheries Science (NIFS) operates a regular ocean observation system which has observed temperature and salinity profiles at standard depths down to 500 m in the marginal seas around Korea bimonthly since 1961 (Figure 1). Chang et al. (2023) assimilated these in-situ temperature profiles in the marginal seas around Korea, resulting in improved accuracy of subsurface temperature and salinity in the East/Japan Sea (EJS) as well as in the Kuroshio and Kuroshio Extension regions and illustrating how regional observation networks can improve ocean prediction accuracy nonlocally, for example in adjacent open oceans.
Figure 1. (A) Distribution of in-situ temperature and salinity (T/S) profiles used in data assimilation in February 2019. Dots indicate T/S profiles provided by the Korean NIFS observation system (yellow) and other observation programs (red) including the Global Temperature and Salinity Profile Programme (GTSPP). Color shading indicates the bottom depth in meters. (B) Distribution of T/S profiles, in the eastern Yellow Sea (YS), northeastern East China Sea (ECS), Korea Strait (KS), and Ulleung Basin (UB) in the East/Japan Sea, provided by the Korean NIFS bimonthly since 1961. (C–F) RMSE for temperature and salinity profiles at the eastern Yellow Sea, and northeastern East China Sea. Freerun, CTRL, and NO NIFS represent numerical simulations without assimilation, with assimilation of all observed data, and with assimilation of observed data excluding NIFS data, respectively. ‘nobs’ represents the number of independent observation data used for evaluation.
An OSE can assess the impact of the Korean NIFS observation system specifically on predicted hydrography in local shelf seas. For this experiment, the ocean prediction system was based on ROMS with a horizontal grid spacing of 5 km and 30 vertical layers. SST data from the Operational Sea Surface Temperature and Ice Analysis (OSTIA), temperature and salinity profiles from the NIFS observation system and the Global Temperature and Salinity Profile Programme (GTSPP), and surface geostrophic currents estimated from gridded satellite altimeter data were assimilated daily in 2019 using the Ensemble Optimal Interpolation (EnOI) method with 30 ensemble members. Independent (i.e., unassimilated) T/S profiles were used for quantitative assessment. A simulation with no data assimilation (Freerun) was compared to analyses assimilating all observations (CTRL) and assimilating all data excluding NIFS observations (NO NIFS). Free run RMSEs from the upper 100 m are generally larger than those with data assimilation, and the CTRL run typically exhibits meaningfully lower RMSEs compared to the NO NIFS experiment. Though variations occurred between regions, with only slight changes particularly in the Ulleung Basin, NIFS data enhanced the accuracy of subsurface temperature and salinity overall by approximately 18% and 37%, respectively, in the coastal shelf seas (two examples given in Figure 1), making the NIFS observation system a valuable asset for the coastal operational ocean prediction system.
A second example highlights collections of observation types. In the East Australian Current (EAC) region, OSEs have been used to compare the impact of assimilating only relatively traditional near real time observations, such as satellite derived observations and vertical hydrographic profiles from Argo and eXpendable BathyThermographs (XBTs) (the TRAD experiment), versus also including newer observation types such as from HF radar (HFR, to be described in more detail below), glider and deep and shallow moorings (the FULL experiment) (Siripatana et al., 2020; Kerry et al., 2020, 2024). Results showed that while assimilating traditional data sets alone improved surface and subsurface properties, including velocities, relative to a free run, newer coastal mooring, radar and glider observations further improved estimates of the overall ocean state. Mooring observations along the continental shelf improved estimates of velocity and temperature inshore of the EAC, and gliders observations were key to constraining estimates of subsurface structure on the continental shelf and the offshore EAC eddies. HF radar observations covering the continental shelf and slope region at 30S were key to representing the cyclonic vorticity inshore of the EAC, resulting in increased cyclonic vorticity both up and downstream of the HF radar location and increased vorticity variance (Siripatana et al., 2020). However, after 5-day forecast windows the predictive skill for shelf velocities was equivalent to that of the TRAD experiment (Siripatana et al., 2020; Kerry et al., 2024a). For these same experiments, Kerry et al. (2020) showed that downscaling to a higher resolution (1km) coastal/shelf model was more effective at maintaining the vorticity gradient in the 5-day forecasts; however, correctly predicting the timing and location of fine-scale features (specifically cyclonic eddies that form inshore of the EAC) remains a challenge.
In cases, OSEs can help identify issues with insufficient data coverage and/or noise, possibly resulting in erroneous variability associated with over-fitting. An example here shows results from OSE tests of the West Coast Operational Forecast System (WCOFS; Xu et al., 2022), specifically related to single or multiple satellite platforms. Model dynamics are based on ROMS at 4-km horizontal resolution in a domain centered on the United States west coast stretching from Mexico to British Columbia, Canada. DA includes HFR surface current vectors, satellite along-track altimetry, and SST. Specifically, Level 3 Visible Infrared Imaging Radiometer Suite (VIIRS) SST from one or two satellites (NPP-Suomi and NOAA-20) were utilized. In these data, individual swaths are mapped to a regular 2-km grid, but gaps due to clouds are not filled. The surface current variability in the forecasts constrained by DA is more energetic in the broad range of geostrophic scales (20-200 km) than in the no-DA case (Figures 2A, B), and quantitatively evident in the 1-dimensional velocity wavenumber spectra (Figure 2C). In early stages of testing, Level 3 VIIRS SST from just one satellite, NPP, was utilized. The velocity amplitudes in the geostrophic range from this estimate (red line) are 2-3 times higher than the no-DA case (blue). Adding SST data from another satellite increases coverage and data redundancy leading to reduced posterior model error. The resulting estimate (green) reduces the spectral amplitude gain due to the DA by about half, indicating that some part of the surface eddy variability in the DA-NPP case is in error, and likely the result of fitting sparse noise. Newly available observational sets, such as SWOT altimetry, will provide new opportunities to assess the level of EKE in the coastal transition zone.
Figure 2. (A, B) Surface daily averaged current amplitude (m/s) from the 4-km resolution WCOFS, 15 June 2020, (A) no DA, (B) DA, including SST from NPP and NOAA-20. (C) The amplitude wavenumber spectrum of the surface velocity component across the white line shown in (A, B) The spectra for each day, 1-30 June 2020, are obtained by applying the Hann window, then Fast Fourier Transform; the plot shows the RMS amplitudes, with averaging over these dates: (blue) no DA, (red) assimilation includes SST from just one satellite, NPP, (blue) adding the second satellite set (NOAA-20), which results in a smoother (lower eddy kinetic energy) estimate.
The impact of a single particular observation platform can also be investigated through OSEs. HFR are land-based coastal observing platforms providing accurate and high-resolution monitoring (around 1 km in space and hourly in time) of surface currents from the near-shore to several tens of km offshore. These measurements are generally accessible in near real-time, providing a unique dataset to constrain surface ocean currents in coastal forecasting systems. However, the assimilation of HFR measurements in numerical models also poses specific challenges. Several OSEs performed over the last two decades have been insightful, providing an assessment of the impact of these surface current observations when assimilated in coastal models by means of different approaches in different regions of the world.
HFR OSEs have used moored current meters and Acoustic Doppler Profilers (Oke et al., 2002; Paduan and Shulman, 2004; Shulman and Paduan, 2009; Ren et al., 2016; Wilkin and Hunter, 2013), surface drifter trajectories (Hernandez-Lasheras et al., 2021; Bendoni et al., 2023) or satellite observations (Yu et al., 2012; Couvelard et al., 2021) as independent validation datasets. Overall, the model velocity error reduction achieved by assimilating HFR observations was found to be between 10 and 50% (Barth et al., 2008; Gopalakrishnan and Blumberg, 2012; Hernandez-Lasheras et al., 2021; Couvelard et al., 2021; Bendoni et al., 2023), with an increase of the correlation with independent observations up to 85% (Oke et al., 2002).
In addition, more specific issues have been investigated. When assimilating HFR data, one must choose whether to assimilate raw radial velocities (i.e., velocity projections directed toward or away from individual antennae) or reconstructed “total” currents (i.e., velocity vectors representing both the meridional and zonal components in areas covered by two or more antennae). While total currents generally provide smoother data than radials and are somewhat easier to compare with model velocities, creating them discards information provided by radial velocities in areas covered by only one antenna. Shulman and Paduan (2009) and Hernandez-Lasheras et al. (2021) compared the assimilation of both radial and total velocities, reaching the conclusion that the relative performance was also dependent on other parameters like the direction of the flow with respect to the radials or the application of an initialization step after analysis which helps to preserve the model dynamical balance. In the EAC region, Kerry et al. (2020) showed that assimilation of radial velocities over the core of Australia’s western boundary current (the East Australian Current) at 30S produced increased cyclonic vorticity along the current’s inshore edge in the vicinity of the HF radar array as well as up and downstream.
A challenge with HFR relates to the highly variable and energetic characteristic of surface currents, which is generally associated with relatively large model error variances. Some OSEs have shown that assimilating these measurements might also have negative side effects on other model variables, on the correction of model fields at depth, or outside the HFR observation area (Zhang et al., 2010; Bendoni et al., 2023).
OSEs have been used also to evaluate the potential of HFR data assimilation to correct the surface and boundary forcing of the model rather than correcting the ocean state itself (Barth et al., 2010, 2011; Marmain et al., 2014; Ren et al., 2016). This approach is particularly useful to minimize short transients appearing during model re-initialization after analysis in sequential data assimilation schemes, which is especially critical when dealing with high-frequency measurements and short assimilation cycles. Assimilating HFR data in the East Australian Current region, Kerry et al. (2016, 2020) allow the DA system to adjust the initial conditions, boundaries and surface forcing. They find that, while the initial condition adjustments can introduce or enhance cyclonic features inshore of the current at the beginning of the analysis window, the features are maintained over the 5-day windows by adjustments to the wind stress forcing.
Finally, the good performance of HFR data assimilation highlighted by these OSEs have also allowed validation of temporal filtering applied on HFR surface currents, for example when the focus is on the sub-inertial ocean variability (Oke et al., 2002; Barth et al., 2008; Shulman and Paduan, 2009; Kerry et al., 2018; Hernandez-Lasheras et al., 2021). Alternatively, when representing high-frequency processes, specific OSEs have demonstrated the potential of HFR data to correct tides and inertial oscillations in the coastal zone (Barth et al., 2010; Gopalakrishnan and Blumberg, 2012; Vandenbulcke et al., 2017).
A second major platform providing critical observations in coastal waters is an underwater glider, and OSEs have been used to evaluate the impact of these observations on coastal modeling systems. Gliders are highly valuable autonomous observing platforms providing high-resolution, subsurface sampling of coastal environments and transition zones toward the open ocean with horizontal scales of the order of 1 km. Jones et al. (2012) showed that the assimilation of mooring and glider data significantly reduced sea surface temperature errors in a coastal model of south-west of Tasmania. In the New York Bight, Zhang et al. (2010) found the impact was more pronounced on salinity than temperature. OSEs have also highlighted the importance of having complementary surface observations when assimilating glider temperature and salinity profiles to avoid spurious velocities (Pasmans et al., 2019), and they have demonstrated the value of glider observations for assessing the representation of modeled ocean fronts (Pascual et al., 2017). Assimilating glider data from deep ocean eddies was shown to significantly improve the subsurface structure of the water column (Kerry et al., 2018). The impact of measurements from fleets of gliders in coastal zones was also assessed in several studies (Shulman et al., 2009; Pan et al., 2014; Gangopadhyay et al., 2013; Mourre and Chiggiato, 2014; Hernandez-Lasheras and Mourre, 2018) leading to valuable quantifications of the model field improvements both at the surface and in the pycnocline, and providing comparisons with more conventional observing strategies. Finally, Mourre and Alvarez (2012) also applied this approach to evaluate the benefits of piloting a glider through a real-time operational adaptive sampling procedure.
Lastly, OSEs have been used to compare the utility and benefit of different data assimilation schemes themselves. Kerry et al. (2024a) used OSEs to compare the time-dependent 4-Dimensional Variational (4D-Var) data assimilation system with the more computationally-efficient, time-independent Ensemble Optimal Interpolation (EnOI) system, across a common modeling and observational framework. They showed that although the 4D-Var system is more computationally expensive, it outperforms the EnOI system against both assimilated and independent observations at the surface and subsurface. The time-dependent DA method gave a more continuous ocean state, with smaller discontinuities between subsequent analyses, and improved forecast skill (after 5 days) for assimilated and independent observations. This was highlighted to be important for coastal and shelf regions with highly intermittent flows. In the Ligurian Sea, Mourre and Chiggiato (2014) compared the performance of the 3D super-ensemble multi-model fusion approach with that of a more conventional Ensemble Kalman Filter (EnKF), highlighting the better skills of the EnKF outside of the area spanned by the assimilated measurements.
An alternative to OSEs for evaluating the efficacy of an observing system is the Observing System Simulation Experiment (OSSE), in which synthetic observations are extracted from a numerical model simulation, referred to as a “nature run” (NR) or “truth” (Figure 3). OSSEs have been widely employed in meteorology (Arnold and Dey, 1986; Atlas, 1997) and more recently in oceanography. OSSEs provide a relatively straightforward methodology to assess the impact of new observation types (e.g., Kerry et al., 2024b), different observing scenarios (e.g., Gwyther et al., 2022, 2023a, 2023b, Barceló-Llull and Pascual, 2023; Alvarez and Mourre, 2014) and/or satellite constellations and future projects (e.g., Mourre et al., 2006), the inclusion of tides on mesoscale predictability (Kerry and Powell, 2022), or even different observation operators and processing chains of observations. OSSEs can also be used to inter-compare different data assimilation schemes (e.g., Storto et al., 2020; Moore et al., 2020). As such, OSSEs have been and continue to be a valuable method for guiding choices about extending the capabilities of the ocean observing network and/or improving existing assimilation strategies.
Figure 3. An outline of the steps taken in (top) a realistic prediction system and (bottom) an Observing System Simulation Experiment. (A) In a realistic prediction system, a forecast simulation over an assimilation cycle of length l provides the prior estimate also called the model background state, with associated background error estimates (blue). Ocean observations, along with error estimates, are collected using instruments deployed in the natural system (black). The background and observations are combined using data assimilation to provide an improved posterior estimate, also called the analysis, along with associated model error (green). The analysis provides data constrained initial conditions for the subsequent assimilation cycle. The analysis and forecasts are evaluated against assimilated or withheld observations. (B) In an OSSE, a model simulation represents the Nature Run or model Reference State (red), from which synthetic observations, perturbed by characteristic observation errors, are drawn (black). A model simulation that is distinct or perturbed from the Reference State serves as the background or Baseline simulation. As in the realistic system, data assimilation combines observations and the background to yield an analysis whose final state provides an initial condition for the subsequent assimilation cycle background. The analysis and forecasts are evaluated against the Nature Run. This figure is adapted from Kerry et al., 2024b.
However, a well-known deficiency of OSSE exercises is that their outcomes depend, to some extent, on the specificities of the analysis and forecast system used within the assessment. Consequently, care must be taken in generalizing the results. The way the NR is chosen and, accordingly, the way synthetic observations are drawn are crucial elements of an OSSE and generally relies on the use of an alternative model (e.g., Errico et al., 2013) or the same numerical model but with different configurations (e.g., Halliwell et al., 2014). It is therefore desirable that OSSE exercises are performed using multiple analysis systems, in order to achieve consensus about the impact of a certain observation type and turn the assessment results onto probabilistic metrics (e.g., percent of systems benefiting by at least a certain threshold from the assimilation of a group of observations, and so on). This approach is, for instance, being followed within the SynObs project of the United Nations Decade of Ocean Science (more information available at https://oceanpredict.org/synobs/), after the encouraging experience in the AtlantOS project (Gasparin et al., 2019). Alternative methodologies may rely on quantifying the change in ensemble properties (e.g., ensemble variance) in forecasting systems when certain synthetic observation types are assimilated, namely, the decrease in ensemble dispersion quantifies the impact of the new observing scenario (Storto et al., 2013).
A prototype fraternal twin Observing System Simulation Experiment (OSSE) system was developed for the Gulf of Mexico based on the HYCOM ocean model (Halliwell et al., 2014). This regional application of the OSSE system was rigorously evaluated by first demonstrating the realism of the unconstrained NR. Second, it was determined that realistic differences (errors) existed between an unconstrained simulation by the data assimilative Forecast Model (FM) and the NR that closely resembled errors between the NR and the true ocean. The final evaluation step compared OSEs that evaluated impacts of existing observing system components to identical OSSEs that evaluated impacts of synthetic versions of the same observing system components. Similar impact assessments were obtained, demonstrating that the OSSE results are realistic. The OSSE system was then used in Halliwell et al. (2015) to evaluate the impact of airborne expendable profiler surveys, which have been used for improving ocean model initialization for coupled hurricane prediction (e.g., Shay and Uhlhorn, 2008) and for using ocean models to predict the dispersal of the Deepwater Horizon oil spill (e.g., Shay et al., 2011). An example impact assessment is presented in Figure 4 using a Taylor (2001) diagram to quantify error reduction. This assessment focuses on error reduction in Ocean Heat Content (OHC) above the 26°C isotherm, an index of the thermal energy available for hurricane intensification. An unconstrained experiment and an experiment assimilating existing ocean observing systems were compared to experiments that also assimilated synthetic Airborne Expendable Conductivity Temperature Depth Probe (AXCTD) profiles sampled at the locations shown in Figure 4 at 1.0- and 0.5-degree horizontal resolutions. Results clearly illustrate the significant error reduction achieved by assimilating existing observing systems, along with the additional error reduction achieved by also assimilating the airborne observations at progressively higher horizontal resolution.
Figure 4. OSSE evaluation of the impact of airborne AXCTD surveys on initializing Ocean Heat Content (OHC) in ocean models. “Surveys” were conducted by deploying synthetic AXCTDs simulated from the NR into the FM at locations shown in the upper left at two horizontal resolutions: 0.5 and 1.0 degrees. Error analysis with respect to the NR (the “truth”) is presented in the Taylor diagram for four experiments. The largest errors occurred in the unconstrained experiment as expected. Large reductions in RMS differences and large increases in correlation coefficients were realized sequentially in the remaining three experiments: assimilating all observations except the airborne profiles, and then additionally assimilating the profiles at 1.0 and 0.5 degree resolution.
A different OSSE conducted in the EuroSea Project evaluated various configurations of in situ experiments aimed to reconstruct fine-scale (~20 km) ocean currents in the context of the Surface Water and Ocean Topography (SWOT) satellite validation (Barceló-Llull et al., 2023). In this work, the impact of different sampling strategies on the reconstruction of fine-scale ocean currents in Mediterranean and Atlantic study regions were identified, with recommendations for the design of in situ experiments (Barceló-Llull and Pascual, 2023). This analysis was carried out with an advanced version of a spatial optimal interpolation algorithm applied in field experiments. Two additional reconstruction methods in the Mediterranean were tested, and a pilot technique based on machine learning showed a slight improvement with respect to the spatial optimal interpolation. Another method based on model data assimilation showed that incorporating CTD simulated-observations in the experiments yielded better reconstructions of temperature and salinity compared to scenarios with no data assimilation or those assimilating only satellite simulated-observations. Lastly, the newly developed Multiscale Inversion for Ocean Surface Topography (MIOST) variational tool for mapping nadir altimetry using current observations obtained from drifters was tested. Maps generated through this method demonstrated improvements in energetic regions, such as the Algerian current, making it a valuable technique for SWOT validation.
In the East Australian Current System a series of OSSEs have been used to understand how assimilating different configurations of temperature data from the surface (satellite) and below the surface (e.g., vertical temperature profiles from XBTs) contribute to estimates of upper ocean heat content, mixed layer depth, and the sub-surface structure of mesoscale eddies (Gwyther et al., 2022, 2023a, 2023b). By exploring the ocean variability spectrum Gwyther et al. (2023b) showed the potential for aliasing in a region of high mesoscale variability if sub-surface observations are not of sufficient spatial or temporal resolution. Additionally, they identified that systematic errors can be introduced by the data assimilation system that hinder the ability of the model to produce more accurate subsurface representation with fortnightly or monthly subsurface XBT observations (compared to weekly). These errors can only be mitigated through improvements to the data assimilation system.
Kerry et al. (2024b) conducted a series of OSSEs for the New Zealand region to assess the impact of subsurface temperature observations collected from fishing vessels (Jakoboski et al., 2024), primarily in coastal and shelf regions. The experiments identified a challenge of assimilating dense coastal and shelf observations into a model that represented both coastal/shelf dynamics and the deep oceanic region around New Zealand. The experiments show that assimilation of the subsurface temperature observations in concert with surface observations results in significant improvements in bottom temperature and heat content estimates in coastal and shelf regions. However, careful specification of the prior observation and model background uncertainties, which influence the way in which the observations are projected onto the model estimates, was required to avoid overfitting to dense coastal observations. The improvement in ocean heat content estimates were particularly sensitive to these prior choices (compared to bottom temperature) as heat content represents an integration through the water column. Significantly, shorter horizontal decorrelation length scales specified for temperature in the 4D-Var background error covariance formulation resulted in improved ocean state estimates away from the dense coastal observations.
Finally, in the Philippine Sea, a region of strong internal tides and energetic mesoscale ocean circulation, a twin experiment revealed that including tides improves subtidal prediction (Kerry and Powell, 2022). The OSSE methodology allowed the authors to identify that the mechanisms were two-fold: firstly, tidal dynamics influence the subtidal circulation, and secondly, higher prior errors must be prescribed for the observations if the model does not resolve the internal tide signal. Over the shallow shelf region of the South China Sea, tidal dynamics were crucial to represent tidal mixing, which modulates the temperature of the SCS and Kuroshio waters, while in the Philippine Sea deep basin the role of tides in improving subtidal predictions related to reducing uncertainty resulting from the inertial tide signal.
The approaches in sections 3.1 and 3.2 provide global information on the actual or potential contribution of observational datasets to the estimation of the ocean state by data assimilation. As a complement, it is often useful to consider the potential contribution of individual key observations in isolation from the others. This contribution is not only local, and not only for the observed variable: as the model’s prior errors are non-local (correlated in space and in time) and span across variables, the actual influence of observations will also be non-local. This information can be accessed through representers, or influence functions: they can be calculated as the prior error covariances taken between observation points and model grid points, to within a multiplier coefficient.
Array modes provide similar information, but on the scale of a complete observational array, and in hierarchized form: among other uses, the theory can help determine which dominant prior error patterns (in space-time and multivariate) are detectable by an observational array within the limits of its observational errors and can therefore be corrected by assimilation.
Using the notation for the observation operator introduced in section 2.1, the best linear unbiased posterior state estimate (aka the analysis) resulting from DA can expressed as where xb is the prior estimate (aka the background), and y is the vector of observations. Uncertainties in xb and y are described by the background error covariance matrix B and the observation error covariance matrix R (see section 2), respectively. The model equivalent of the observations is H(xb) and H and HTrepresent a linearization of H and its transpose (aka the adjoint) respectively. Thus, the analysis represents the background corrected by the weighted sum of the departures of the model from the observations. The weight matrix is referred to as the Kalman gain matrix. The covariance matrix , the so-called stabilized representer matrix, plays a central role in assessing the efficacy and impact of the observing system. The columns of P are called representers and quantify the covariance of the total error between the space-time observation locations. The eigenvectors of P represent the EOFs of the total error variance, and when mapped back to state-space by BHT are called array modes (Bennett, 1985) which provide information about the field-of-view of the observing system. The corresponding eigenvalues provide information about the degrees of freedom of the signal (DFS). More specifically, the number of eigenvalues of the scaled representer matrix that are greater than 2 provides an estimate of the number of DFS that can be distinguished from observation errors. Moore et al. (2018, 2021) have employed these ideas to estimate the effective DFS of the observing array for the California Current System (CCS) and Mid-Atlantic Bight (MAB) and retune the data assimilation system to prevent overfitting to scales that are not resolved by the observing system. Le Hénaff et al. (2009); Charria et al. (2016) and Lamouroux et al. (2016) have applied similar ideas to explore the information provided by the observing system in the Bay of Biscay.
Monte Carlo methods such as the Ensemble Kalman Filter (EnKF) and its variants can also be used to calculate representers, as first done for example by Evensen (1994) and many others since then. Following Echevin et al. (2000), the representer in covariance form for observation k is the line vector
where hk is the observation operator for that observation (a vector projecting the three-dimensional, multivariate state space onto the individual space of this observation), and an ensemble approach can be used to derive an estimate of BHT. Besides the covariance term (Equation 1), representers can also be presented in correlation form as illustrated above in the case of and below, or in model correction form, the latter assuming an observation error estimate, an innovation value, and an analysis scheme, e.g. an EnKF analysis step as done in Echevin et al. (2000), also illustrated below.
The Bay of Biscay (BoB) physical-biogeochemical model and the ensemble generation approach are described in Vervatis et al. (2021). The configuration is a high-resolution (1/36°) subset of the Iberia–Biscay–Ireland (IBI) domain (Sotillo et al., 2015), based on the NEMOv3.6-PISCESv2 platform (Madec and the NEMO team, 2016; Aumont et al., 2015), using a stochastic model of first-order autoregressive processes for the production of ensembles. Three simulation experiments of 40-member ensembles were designed to estimate ocean model errors: Ens-1 perturbing only the physics, Ens-2 perturbing only the biogeochemistry, and Ens-3 perturbing both simultaneously.
We showcase the use of model ensemble anomalies (departures from the Ensemble mean) in the BoB, as a proxy of model uncertainties, to calculate multivariate representers of single observations, with the objective of assessing the potential impact of observations onto unobserved variables, such as other data types, or subsurface variables (not shown here).
Figure 5 shows examples of multivariate, zero-lag representers of single SST (sea-surface temperature), SSH (sea-surface height) and Chl (Chlorophyll a at the surface) observations, onto (A,E,I) SST, (B,F,J) SSS (sea-surface salinity), (C,G,K) SSH and (D,H,L) Chl, at three different BoB locations on April 30 and May 7, 2012: the Abyssal plain, the Armorican Shelf (AS), and the English Channel (EC). The representers are in correlation form. Convolution with a localization function of 3° is applied to constrain spurious long-distance correlations resulting from the small size of the ensembles (40 members).
Figure 5. (A–D) Correlations between OSTIA SST observations at 3 locations and surface variables on April 30, 2012. Ensemble covariances are calculated from Ens-1 40 members. Ens-1 contains physics-only perturbations. The single observation representers are calculated for three different locations (i.e. cor(SST,SST)~1) as shown. A localization function of 3° is applied to constrain distant spurious correlations. A line marks the 200 m isobath. (E–H) same for SSH. (I–L) same for Chl on May 07, 2012. Ensemble covariances are calculated from Ens-3 40 members. Ens-3 contains physics and biogeochemistry perturbations.
The structures of the representers reveal marked differences between the abyssal and coastal areas, as well as between variables:
● On the shelves (AS, EC), the filament-shaped structures for SST, SSS and Chl (resp. panels (A,E,I), (B,F,J), (D,H,L)) were likely linked to specific fine-scale uncertainty processes such as wind-influenced river discharges (Loire river plume; AS), mid-shelf thermal fronts (AS), and tidal fronts (EC). Due to the mixing conditions on the inner shelf at that time of year, the SSH response there (panels (C,G)) was found to be relatively large-scale as it is associated with barotropic processes at the scale of the external Rossby radius. This confirms the classic notion that coastal/shelf processes, and associated uncertainty processes, are multiscale.
● In the Abyssal plain, scales associated with mesoscale and submesoscale features could often be detected (e.g., panels (E,F,J,K)), while SST and SSH appeared as decorrelated (panel (C)) due to large-scale atmospheric forcings directly influencing SST in the spring season while SSH is largely influenced by low-frequency mesoscale variability. Observations of both variables therefore appear as very complementary in the abyssal plain.
● In the Abyssal plain and AS, the end of the spring bloom can be detected by the negative correlation (panels (D,I)) between SST (e.g., heating up) and Chl (plankton depletion following a bloom). In a similar way, the correlation between SSH and Chl appears mostly negative (panels (H,K)). This shows the need for regime-/time-dependent error covariances between variables on shelves.
Full details of what follows are contained in the original article (Nguyen-Duy et al., 2023) and only a few elements will be given here. The primitive-equation numerical model SYMPHONIE (Marsaleix et al., 2006; 2008) has been set up in the Gulf of Tonkin (GoT), which is a shallow (shelf) sea. It has a fine horizontal resolution of 300 m near the Red River mouths and a coarser resolution of 4.5 km near the open boundary. The vertical discretization consists of 20 sigma levels, and tides are included. Two 50-member ensembles are generated by perturbing the ECMWF wind forcing using pseudorandom combinations of bivariate wind EOFs – here, we illustrate results obtained by the authors’ ENS_COAST ensemble2, which is tuned toward the representation of coastal processes. The perturbations of the wind fields are meant to represent uncertainties in the ECMWF wind analyses.
In order to explore the impact of HFR observations to constrain the model if they were assimilated, the authors calculate representers in correction form, as in Echevin et al. (2000) (Figure 6). They set an observed velocity innovation value of +15 cm/s (the direction depends on the case) – this value is based on comparisons between the simulation and the HFR data, as explained in the original article. The observational uncertainty standard deviation is assumed to be 10 cm/s (also explained in the original article). The HFR observation is located at 19°N, 106°E within the coastal current (when the current is present). July 10 corresponds to a period of large spread of the current (6-8 cm/s) and with a southward coastal current at that location, with an amplitude larger than 20 cm/s (not shown). The representers are not localized, in order to show the trends in long-distance covariances, but bearing in mind that these trends may be artifacts of statistical calculations.
Figure 6. (A) Correction (in cm/s) on the meridional velocity v in response to a 15cm/s innovation in zonal velocity u at 19°N, 106°E (blue point). (B) Correction on v in response to an innovation in v (15cm/s) at the same point. (C, D) Corrections on τx, τy, respectively, in response to an innovation in v (15cm/s) at the same point. Adapted from Nguyen-Duy et al. (2023).
The impact of a meridional (alongshore) velocity observation on the meridional velocity (Figure 6B) is clear in the coastal current, showing a broad meridional extension and a narrow zonal extension. In contrast, the meridional impact of a zonal (cross-shore) velocity observation (Figure 6A) is weaker but still apparent. Both results are interesting for the deployment of a HFR site, since the azimuth of the radar beams will have to be optimized for alongshore velocity observation.
As noted by the authors, previous results (e.g. Lamouroux, 2006; Barth et al., 2011) have suggested that while high-frequency dynamics dominate on the shelf, a correction of the ocean state only may not last beyond the inertial time scale, and a correction of the surface atmospheric forcings could be needed as a complement. Figures 6C, D show the corrections to the zonal and meridional components of wind stress that would result from assimilating a northward surface current observation in a data assimilation system capable of correcting for wind errors. The authors observe an increase in the northerly component of the wind stress across the basin (Figure 6D), in agreement with the ensemble variance of the wind stress (shown in the original paper). The zonal wind stress correction is weaker (Figure 6C). Of course, one should keep in mind that the representers are not localized, and some long-distance covariances might be untrustworthy, but in general it is found that on the open sea the spatial correlation scales of atmospheric errors are larger than the scales of oceanic error processes.
The authors conclude that the impact of HFR measurements is clear on the surface coastal current and possibly the wind stress within their experimental protocol.
Array modes can also be calculated using ensemble methods. In the ensemble-based category, a full stochastic implementation of array mode analysis, as in Section 3.2 of Lamouroux et al. (2016), adopting the nondimensional array-mode definition of Le Hénaff et al. (2009), seems the most practical. In order to project information from such array modes onto model variables (state space), one can use modal representers, as e.g. in Charria et al. (2016). Also, several techniques can be used to enrich the number of DFS explained by an ensemble in order to calculate array modes, such as the so-called chaos polynomials in Oke et al. (2015). Also, it should be noted that array mode analysis can be conducted with full covariance matrices, both for the ensemble covariances and the observational error covariances.
We show an example of array mode analysis in the most southeasterly area in the Bay of Biscay, on either side of the French-Spanish border. There, the JERICO-Next European project (2015-2019) studied the deployment of a third HFR radar site on the French Landes coast, in addition to two existing sites on the Spanish Basque coast (Figure 7A), as part of a wide-reaching endeavor of expanding a European capacity of coastal observatories (JERICO = Joint European Research Infrastructure Network for Coastal Observatories). Several model ensembles were available to investigators. The – as yet unpublished – results shown here used a 500m-resolution 50-member ensemble with the SYMPHONIE model, itself downscaled from a larger-scale NEMO ensemble (details in Ghantous et al., 2020). All analyses were carried out over the period from 15 Jan – 15 Feb, considering radial velocities at antenna sites. The observational error on radial velocities was set to a constant 0.03m/s; correlated observation errors were also considered, and their impact studied, as shown below.
Figure 7. (A) Radial velocity measurement points for 3 HFR sites in the most southeasterly area in the Bay of Biscay including two existing systems (Matxitxako – blue and Higer – red) and a future system to be deployed (Land1 – green). (B) Array mode spectra for radial velocities from the HFR sites, and combinations thereof on 15 January 2012, using a downscaled ensemble from Ghantous et al. (2020). Colors as on left panel, with in addition: combination of both existing sites – pink, and combination of all sites – black. Solid lines: uncorrelated radial velocity error. Dotted lines: correlated radial velocity error (correlation distance=100km). 49 modes could be calculated from the 50 members of the ensemble. All 49 modes are detectable above the observational error, set at 0.03m/s, which translates as 1 = 100 in our nondimensional array-space representation.
The array mode analysis allows characterizing and visualizing the model error structures which are detectable by the observations and which are potentially controllable through data assimilation. This can be done by means of array mode spectra (Figure 7B, solid lines) and modal representers in state space (not shown). As can be seen, every single radar is able to detect the 49 degrees of freedom spanned by the ensemble above observational noise (represented as 1 since with our definition of array modes here spectra are nondimensional). The spectra almost follow the same slope. However, more uncertainty variance is explained when we consider more radars, and some radars catch DFS better than some others. Indeed, adding radars improves the detection of model errors by increasing the quantity and location of observations that lead to efficient sampling of model error structures. In particular, the third projected radar site would bring additional detail at sampling surface velocity errors in the model, particularly for the zonal component because of its location (detailed result not shown, but the figure shows this in synthetic form).
Additionally (Figure 7B, dashed lines), we studied the impact of correlated measurement errors on the array mode analysis. To that end, we introduced a correlation radius of 100 km on the ensemble-based uncertainties in data space (radial components of the radars). As can be seen, correlated observation errors tend to lower most of the array mode spectrum except for the leading eigenvalues. If/when observation error is even higher (not the case for this example), this could have the effect of reducing the dimension of the detectable subspace (in array mode jargon: bringing eigenvalues below threshold). However, under our hypotheses here, our previous conclusions regarding the existing array performance and the positive impact of a third site were not significantly jeopardized by such correlated noise contamination.
Complementing the discussion of Section 3.3, observation impact studies represent an additional method to quantify the influence of each assimilated datum on the estimated ocean state. In this context, observation impact refers to the difference in a chosen circulation metric that is calculated using the analysis (posterior) versus that using the background (prior). Because the assimilation increment (difference between the analysis and background) equals the product of the Kalman gain matrix (introduced in Section 3.3) and the so-called innovation vector (the vector of differences between observations and the observation operator’s estimate from the background),
K provides direct quantitative information about the influence of the observations on the analysis and ensuing forecast. Specifically, KT yields the impact of each observation on a given analysis metric or metric of forecast error (Langland and Baker, 2004), while quantifies the sensitivity of such metrics to a change in the observations or observing array (Trémolet, 2008). Stated differently, changes in a metric resulting from assimilation can be expressed as a sum of terms, each dependent on a single observation, and therefore, the impact of each individual observation on changes to a chosen metric can be calculated directly. Impacts from subsets of observations can be summed usefully in various ways (e.g., by observation platform to ascertain its overall utility or by geographical region to assess complementary, co-located influences).
Moore et al. (2011, 2017) have used these methods to quantify the impact of individual components of the observing system on ROMS ocean state estimates of the CCS. Satellite remote sensing data make up the lion’s share of available observations and in aggregate have the largest influence on ocean analyses. However, when considering a single observation from any particular platform, the impact of in situ hydrographic observations can be an order of magnitude greater than a single satellite measurement. The substantial impact of assimilating in situ hydrographic information has also been recognized in global ocean data assimilation systems (e.g., Turpin et al., 2016). The transfer of information from the observations to various space-time locations of the state estimate is controlled, in part, by the underlying ocean dynamics. Within the CCS, the influence of advection by the circulation and eddies as well as the alongshore propagation of coastally-trapped waves is very evident in the spatial distribution of the observation impacts. Fiechter et al. (2011) have used a similar approach to quantify the impact of different elements of the observing system on eddy kinetic energy and primary production in ROMS configured for the coastal Gulf of Alaska.
In a multiple nested configuration reaching approximately 700 m resolution on the New England shelf observed intensively by the U.S. National Science Foundation’s Ocean Observatories Initiative Pioneer array, Levin et al. (2020, 2021a, b) performed observation impact calculations to DA analyses of the MAB. Circulation metrics quantifying cross-isobath mass, heat and salt fluxes, revealed that in situ temperature and salinity observations offered 2-3 times the impact of remotely sensed SSH and SST observations, and in situ velocity observations had greater impact on higher than lower resolution grids as representation of transient, vigorous, geostrophically unbalanced submesoscale features increased. These results are in agreement with Kerry et al. (2018) who used a similar approach to show that hydrographic profiles from autonomous ocean gliders, while sparse in space and time, have a disproportionately large impact, as they provide information on subsurface structure. Powell (2017) showed that glider observations have a large impact on the representation of the Hawaiian Lee Counter Current transport as they constrain the isopycnal tilt across the transport section. Finally, Christensen et al. (2018) calculated that, though relatively few in number, in situ temperature and salinity observations deriving from multiple sources dominated satellite and HFR in terms of impact on the estimated transport of the Norwegian Coastal Current when calculated on a per datum basis
Both sequential and variational schemes have been successfully used for HFR impact assessment. In particular, the capability of the 4D-Var approach to evaluate the contribution of individual observations to specific index increments, where the index is a specific measure of interest of the ocean circulation has been exploited in several studies (Kerry et al., 2018; Levin et al., 2020; Bendoni et al., 2023).
In the meteorological community, the impact of observations on forecast skill based on KT is referred to as Forecast Sensitivity to Observation Impacts (FSOI). FSOI was applied by Moore et al. (2011) and Drake et al. (2023) to quantify the impact of different observing platforms on forecast skill in the CCS. Drake et al. (2023) found that in general only ~50% of all observations lead to an improvement in forecast skill. The remaining observations degrade the forecast or have little or no impact on forecast skill. This finding is in line with experience in operational numerical weather prediction. In this case also, a single in situ observation of temperature or salinity is generally an order of magnitude more impactful than a single measurement from a remote sensing platform.
Though small in geographical area, coastal, shelf, and marginal sea environments reside adjacent to population centers and experience heightened relevance due to regional societal, industrial, and other management interests. In response, many coastal ocean observing systems have developed across the globe, including the U.S. Integrated Ocean Observing System, Australia’s Integrated Marine Observing System, the Mediterranean Ocean Observing System for the Environment, and the Korea Ocean Observing Network, to name a few. Coastal, shelf, and marginal seas are challenging to comprehensively observe and accurately model due to their broad range of time and space scales from submesoscale and super-inertial motions of plumes and filaments to more slowly evolving mesoscale eddies and, in places, basin-scale features like western boundary currents. Data assimilation offers a valuable approach to interpolate and extrapolate sparse observations using ocean dynamics to produce four-dimensional estimates of the physical ocean state with improved fidelity.
This review highlights an extensive and expanding collection of studies rigorously assessing the impact of ocean observations on improving coastal ocean state estimates through data assimilation. Multiple approaches (OSE, array modes, and observation impacts) have repeatedly demonstrated positive impacts on important physical properties (e.g., transport, heat content, bottom temperature, and eddy kinetic energy) in analysis or forecast fields constrained by satellite, HFR, glider, Argo, drifter, and shipboard data platforms in terms of reduced model error against assimilated or independent data. Such outcomes support a recommendation to maintain existing, diverse observation systems. Results have also shown that limited data sets can yield erroneous circulation features, and thus we encourage analysis and forecast systems to assimilate varied, complementary data sets. Several studies emphasized the outsized contribution of subsurface hydrographic observations on data assimilative systems, supporting routine deployments of gliders and floats offshore of coastal environments and newer observation types such as widespread fishing vessel based observations of temperature in shelf seas (e.g., Jakoboski et al., 2024). The assimilation of localized subsurface observations, such as those collected from fishing vessels that can be concentrated on coastal regions, requires careful specification of prior observation and background error covariances to optimize the way in which the observations inform the numerical model (e.g., Kerry et al., 2024b). OSSEs provide cost-effective guidance toward efficient sampling strategies and data assimilation configurations to constrain desired features in model analyses, offering roadmaps toward observing system design expansion.
Accompanying these real advances, opportunities exist for continued improvement at both technical and scientific levels. Proper specification of prior and observational errors and covariances, including error of representativeness, are critical and challenging elements of effective data assimilation systems (e.g., Kerry et al., 2024b). Checks of posterior errors can ensure consistency with prior error assumptions (e.g., Desroziers et al., 2005). Computational costs of current operational 4D-Var systems remain high; future efficiency gains may occur through application of multi-precision, multi-resolution, and saddle-point algorithms (Moore et al., 2023) or using high-accuracy emulators of full-physics models. While local observations improve regional ocean state estimates, these data are not necessarily assimilated by global systems, leaving room to improve representation of coastal circulations in global analyses. The impact of directly assimilating these observations in global models or upscaling by providing more accurate fluxes from high resolution regional analysis systems to global systems could be investigated. Finally, new observational platforms, such as the Surface Water and Ocean Topography (SWOT) mission that resolves instantaneous sea level at 20 km (Fu et al., 2024), offer promising resources to further constrain coastal models in the future, though issues described above such as error correlations must be considered carefully.
In addition, the rise in popularity of data-driven techniques for regression and classification problems in complex dynamical systems, such as machine and deep learning, has led to an increasing diversity of algorithms that can be tailored to address specific challenges in DA. For instance, notable initial attempts involve the utilization of neural networks to rectify biases in dust observations within an analysis system (Jin et al., 2019), as well as the application of relevance vector machines to correct biases in sea surface temperature data (Storto and Oddo, 2019). Furthermore, machine learning algorithms have been employed to explore the subsampling and quality control of Earth observations (Lary et al., 2016, 2018). Lastly, in certain applications, the intricate coding of observation operators can be replaced by data-driven algorithms (e.g., Xue and Forman, 2017; Fang and Li, 2019; Kwon et al., 2019). Artificial intelligence algorithms can be used also to build observational operators in cases where the exact physics relating observables and model state variables is still unknown and/or its strong nonlinearity limits its applicability. Storto et al. (2021) used a neural network (NN) built observation operator to project and assimilate acoustic transmission loss data into temperature profiles. Results from the authors highlight two crucial aspects of the data-driven operator: i) expand the network of observables that can be used in data assimilation exercises; ii) improve the accuracy of the linear physics/statistics-based observation operator. These advancements have the potential to optimize the assimilation of high-resolution observing networks and significantly facilitate the integration of novel observation types into regional assimilation systems.
This review has focused on observations of physical properties and their impact improving ocean circulation models because accurate estimates of the physical ocean state are generally a valuable precursor for coupled model systems and because physical data assimilation approaches and observation platforms are quite mature. Yet many needs in coastal, shelf, and marginal seas stretch beyond ocean physics, including for example estimates of primary production, phytoplankton community structure, ocean pH, probability of harmful algal blooms or hypoxia, and air-sea carbon fluxes, as well as estimates to help manage fished marine species or reducing bycatch. Some approaches involve data assimilation into coupled physical/biogeochemical dynamical models that is analogous to the systems discussed above (e.g., Song et al., 2016; Ciavatta et al., 2018), whereas others apply statistical models given physical ocean properties (Carter et al., 2021; Brodie et al., 2018). Though not usually calculated explicitly, and exceptions occur (e.g., Raghukumar et al., 2015), improvements to physical properties through data assimilation generally extend benefits to subsequent, non-physical predictions. Thus, the extensive, positive impact of ocean observations on model solutions described above reaches well beyond the physical metrics alone. We encourage efforts to quantify these impacts in multidisciplinary modeling systems.
CE: Conceptualization, Writing – original draft, Writing – review & editing. PM-F: Conceptualization, Writing – original draft, Writing – review & editing. BB-L: Writing – original draft, Writing – review & editing. GC: Writing – original draft, Writing – review & editing. B-JC: Writing – original draft, Writing – review & editing. GH: Writing – original draft, Writing – review & editing. LH: Writing – original draft, Writing – review & editing. CK: Writing – original draft, Writing – review & editing. VK: Writing – original draft, Writing – review & editing. AK: Writing – original draft, Writing – review & editing. AM: Writing – original draft, Writing – review & editing. BM: Writing – original draft, Writing – review & editing. PO: Writing – original draft, Writing – review & editing. AP: Writing – original draft, Writing – review & editing. MR: Writing – original draft, Writing – review & editing. CS: Writing – original draft, Writing – review & editing. AS: Writing – original draft, Writing – review & editing. VV: Writing – original draft, Writing – review & editing. JW: Writing – original draft, Writing – review & editing.
The author(s) declare financial support was received for the research, authorship, and/or publication of this article. We gratefully acknowledge many sources of support for this work. CE acknowledges support by the Simons Foundation through the Simons Collaboration on Computational Biogeochemical Modeling of Marine Ecosystems (CBIOMES) grant ID 549949. Parts of this work were originally supported by two European Union (EU)-funded initiatives: (1) the Copernicus Marine Service and their Service Evolution program; (2) the JERICO-Next project (Joint European Research Infrastructure Network for Coastal Observatories) funded by the EU’s Horizon 2020 research and innovation program (2015-2019). The contribution of PD is supported by Centre National de la Recherche Scientifique (CNRS). The contribution of VV is supported by the Copernicus Marine Service Evolution projects SCRUM/2 and MULTICAST. The contribution of GC is supported by Institut Français de Recherche pour l’Exploitation de la Mer (Ifremer). The contribution of CS is supported by the JERICO-Next project. MR and CK acknowledge support of the Australian Research Council for model development through grants DP140102337, LP170100498, LP220100515. BB-L and AP acknowledge fundings from the EuroSea project, funded by the European Union’s Horizon 2020 research and innovation programme under grant agreement No 862626. GH acknowledges support by the National Oceanographic and Atmospheric Administration Atlantic Oceanographic and Meteorological Laboratory. BB-L is supported by the Balearic Islands Government Vicenç Mut program, grant PD/008/2022. B-JC is supported by the Basic Science Research Program through the National Research Foundation of Korea (NRF), the Ministry of Education (2020R1A2C1014678).
We thank Nadia K. Ayoub (CNRS, LEGOS, Toulouse, France), Thanh Ngo-Duc and Tung Nguyen-Duy (both at University of Science and Technology of Hanoi, Hanoi, Viet Nam), and Elsevier Publishing for allowing us to include an edited figure from their published work (Nguyen-Duy et al., 2023), and for discussion and rereading. We acknowledge the use of European Centre for Medium-range Weather Forecasts (ECMWF) computing and archiving facilities in this research.
Author CS was employed by company NOVELTIS.
The remaining authors declare that the research was conducted in the absence of any commercial or financial relationships that could be construed as a potential conflict of interest.
All claims expressed in this article are solely those of the authors and do not necessarily represent those of their affiliated organizations, or those of the publisher, the editors and the reviewers. Any product that may be evaluated in this article, or claim that may be made by its manufacturer, is not guaranteed or endorsed by the publisher.
Alvarez A., Mourre B. (2014). Cooperation or coordination of underwater glider networks? An assessment from observing system simulation experiments in the ligurian sea. J. Atmospheric Oceanic Technol. 31, 2268–2277. doi: 10.1175/JTECH-D-13-00214.1
Arnold C. P., Dey C. H. (1986). Observing-systems simulation experiments: past, present, and future. Bull. Amer. Meteor. Soc 67, 687–695. doi: 10.1175/1520-0477(1986)067<0687:OSSEPP>2.0.CO;2
Atlas R. (1997). Atmospheric observations and experiments to assess their usefulness in data assimilation (Special issue data assimilation in meteorology and oceanography: theory and practice). J. Meteorological Soc. Japan 75, 111–130. doi: 10.2151/jmsj1965.75.1B_111
Aumont O., Ethé C., Tagliabue A., Bopp L., Gehlen M. (2015). PISCES-v2: an ocean biogeochemical model for carbon and ecosystem studies. Geosci. Model. Dev. 8, 2465–2513. doi: 10.5194/gmd-8-2465-2015
Barceló-Llull B., Pascual A. (2023). Recommendations for the design of in situ sampling strategies to reconstruct fine-scale ocean currents in the context of SWOT satellite mission. Front. Mar. Sci. 10. doi: 10.3389/fmars.2023.1082978
Barceló-Llull B., Pascual A., Albert A., Beauchamp M., Fablet R., Guinehut S., et al. (2023). Analysis of the OSSEs with multi-platform in situ data and impact on fine-scale structures - Revised version. EuroSea. doi: 10.3289/eurosea_d2.3_v2
Barth A., Alvera-Azcárate A., Beckers J.-M., Staneva J., Stanev E. V., Schulz-Stellenfleth J. (2011). Correcting surface winds by assimilating high-frequency radar surface currents in the German Bight. Ocean Dynamics 61, 599–610. doi: 10.1007/s10236-010-0369-0
Barth A., Alvera-Azcárate A., Gurgel K.-W., Staneva J., Port A., Beckers J.-M., et al. (2010). Ensemble perturbation smoother for optimizing tidal boundary conditions by assimilation of High-Frequency radar surface currents – application to the German Bight. Ocean Sci. 6, 161–178. doi: 10.5194/os-6-161-2010
Barth A., Alvera-Azcárate A., Weisberg R. H. (2008). Assimilation of high-frequency radar currents in a nested model of the West Florida Shelf. J. Geophys. Res. 113, 2007JC004585. doi: 10.1029/2007JC004585
Bendoni M., Moore A. M., Molcard A., Magaldi M. G., Fattorini M., Brandini C. (2023). 4D-Var data assimilation and observation impact on surface transport of HF-Radar derived surface currents in the North-Western Mediterranean Sea. Ocean Model. 184, 102236. doi: 10.1016/j.ocemod.2023.102236
Bennett A. F. (1985). Array design by inverse methods. Prog. Oceanography 15, 129–156. doi: 10.1016/0079-6611(85)90033-3
Brankart J.-M., Cosme E., Testut C.-E., Brasseur P., Verron J. (2010). Efficient adaptive error parameterizations for square root or ensemble kalman filters: application to the control of ocean mesoscale signals. Monthly Weather Rev. 138, 932–950. doi: 10.1175/2009MWR3085.1
Brankart J.-M., Ubelmann C., Testut C.-E., Cosme E., Brasseur P., Verron J. (2009). Efficient parameterization of the observation error covariance matrix for square root or ensemble kalman filters: application to ocean altimetry. Monthly Weather Rev. 137, 1908–1927. doi: 10.1175/2008MWR2693.1
Brodie S., Jacox M. G., Bograd S. J., Welch H., Dewar H., Scales K. L., et al. (2018). Integrating dynamic subsurface habitat metrics into species distribution models. Front. Mar. Sci. 5. doi: 10.3389/fmars.2018.00219
Carter B. R., Bittig H. C., Fassbender A. J., Sharp J. D., Takeshita Y., Xu Y., et al. (2021). New and updated global empirical seawater property estimation routines. Limnology Ocean Methods 19, 785–809. doi: 10.1002/lom3.10461
Chang I., Kim Y. H., Jin H., Park Y.-G., Pak G., Chang Y.-S. (2023). Impact of satellite and regional in-situ profile data assimilation on a high-resolution ocean prediction system in the Northwest Pacific. Front. Mar. Sci. 10. doi: 10.3389/fmars.2023.1085542
Charria G., Lamouroux J., De Mey P. (2016). Optimizing observational networks combining gliders, moored buoys and FerryBox in the Bay of Biscay and English Channel. J. Mar. Syst. 162, 112–125. doi: 10.1016/j.jmarsys.2016.04.003
Chelton D. B., Ries J. C., Haines B. J., Fu L.-L., Callahan P. S. (2001). “Chapter 1 satellite altimetry,” in International geophysics (Elsevier), 1–ii. doi: 10.1016/S0074-6142(01)80146-7
Christensen K. H., Sperrevik A. K., Broström G. (2018). On the variability in the onset of the norwegian coastal current. J. Phys. Oceanography 48, 723–738. doi: 10.1175/JPO-D-17-0117.1
Ciavatta S., Brewin R. J. W., Skákala J., Polimene L., De Mora L., Artioli Y., et al. (2018). Assimilation of ocean-color plankton functional types to improve marine ecosystem simulations. JGR Oceans 123, 834–854. doi: 10.1002/2017JC013490
Couvelard X., Messager C., Penven P., Smet S., Lattes P. (2021). Benefits of radar-derived surface current assimilation for South of Africa ocean circulation. Geosci. Lett. 8, 5. doi: 10.1186/s40562-021-00174-y
Cummings J. A. (2005). Operational multivariate ocean data assimilation. Quart J. R. Meteoro Soc. 131, 3583–3604. doi: 10.1256/qj.05.105
De Mey-Frémaux P., Ayoub N., Barth A., Brewin R., Charria G., Campuzano F., et al. (2019). Model-observations synergy in the coastal ocean. Front. Mar. Sci. 6. doi: 10.3389/fmars.2019.00436
Desroziers G., Berre L., Chapnik B., Poli P. (2005). Diagnosis of observation, background and analysis-error statistics in observation space. Quart J. R. Meteoro Soc. 131, 3385–3396. doi: 10.1256/qj.05.108
de Toma V., Ciani D., Hesham Essa Y., Yang C., Artale V., Pisano A., et al. (2024). Skin sea surface temperature schemes in coupled ocean–atmosphere modelling: the impact of chlorophyll-interactive e-folding depth. Geosci. Model. Dev. 17, 5145–5165. doi: 10.5194/gmd-17-5145-2024
Donlon C. J., Martin M., Stark J., Roberts-Jones J., Fiedler E., Wimmer W. (2012). The operational sea surface temperature and sea ice analysis (OSTIA) system. Remote Sens. Environ. 116, 140–158. doi: 10.1016/j.rse.2010.10.017
Drake P., Edwards C. A., Arango H. G., Wilkin J., TajalliBakhsh T., Powell B., et al. (2023). Forecast Sensitivity-based Observation Impact (FSOI) in an analysis–forecast system of the California Current Circulation. Ocean Model. 182, 102159. doi: 10.1016/j.ocemod.2022.102159
Echevin V., De Mey P., Evensen G. (2000). Horizontal and vertical structure of the representer functions for sea surface measurements in a coastal circulation model. J. Phys. Oceanogr. 30, 2627–2635. doi: 10.1175/1520-0485(2000)030<2627:HAVSOT>2.0.CO;2
Edwards C. A., Moore A. M., Hoteit I., Cornuelle B. D. (2015). Regional ocean data assimilation. Annu. Rev. Mar. Sci. 7, 21–42. doi: 10.1146/annurev-marine-010814-015821
Errico R. M. (1997). What is an adjoint model? Bull. Amer. Meteor. Soc 78, 2577–2591. doi: 10.1175/1520-0477(1997)078<2577:WIAAM>2.0.CO;2
Errico R. M., Vukićević T., Raeder K. (1993). Examination of the accuracy of a tangent linear model. Tellus A: Dynamic Meteorology Oceanography 45, 462. doi: 10.3402/tellusa.v45i5.15046
Errico R. M., Yang R., Privé N. C., Tai K., Todling R., Sienkiewicz M. E., et al. (2013). Development and validation of observing-system simulation experiments at NASA’s Global Modeling and Assimilation Office. Quart J. R. Meteoro Soc. 139, 1162–1178. doi: 10.1002/qj.2027
Evensen G. (1994). Sequential data assimilation with a nonlinear quasi-geostrophic model using Monte Carlo methods to forecast error statistics. J. Geophys. Res. 99, 10143–10162. doi: 10.1029/94JC00572
Fang M., Li X. (2019). An artificial neural networks-based tree ring width proxy system model for paleoclimate data assimilation. J. Adv. Model. Earth Syst. 11, 892–904. doi: 10.1029/2018MS001525
Fiechter J., Broquet G., Moore A. M., Arango H. G. (2011). A data assimilative, coupled physical–biological model for the Coastal Gulf of Alaska. Dynamics Atmospheres Oceans 52, 95–118. doi: 10.1016/j.dynatmoce.2011.01.002
Fu L.-L., Chelton D., Le Traon P.-Y., Morrow R. (2010). Eddy dynamics from satellite altimetry. Oceanog 23, 14–25. doi: 10.5670/oceanog.2010.02
Fu L., Pavelsky T., Cretaux J., Morrow R., Farrar J. T., Vaze P., et al. (2024). The surface water and ocean topography mission: A breakthrough in radar remote sensing of the ocean and land surface water. Geophysical Res. Lett. 51, e2023GL107652. doi: 10.1029/2023GL107652
Gangopadhyay A., Schmidt A., Agel L., Schofield O., Clark J. (2013). Multiscale forecasting in the western North Atlantic: Sensitivity of model forecast skill to glider data assimilation. Continental Shelf Res. 63, S159–S176. doi: 10.1016/j.csr.2012.09.013
Gasparin F., Guinehut S., Mao C., Mirouze I., Rémy E., King R. R., et al. (2019). Requirements for an integrated in situ atlantic ocean observing system from coordinated observing system simulation experiments. Front. Mar. Sci. 6. doi: 10.3389/fmars.2019.00083
Ghantous M., Ayoub N., De Mey-Frémaux P., Vervatis V., Marsaleix P. (2020). Ensemble downscaling of a regional ocean model. Ocean Model. 145, 101511. doi: 10.1016/j.ocemod.2019.101511
Gopalakrishnan G., Blumberg A. F. (2012). Assimilation of HF radar-derived surface currents on tidal-timescales. J. Operational Oceanography 5, 75–87. doi: 10.1080/1755876X.2012.11020133
Gwyther D. E., Keating S. R., Kerry C., Roughan M. (2023a). How does 4DVar data assimilation affect the vertical representation of mesoscale eddies? A case study with observing system simulation experiments (OSSEs) using ROMS v3.9. Geosci. Model. Dev. 16, 157–178. doi: 10.5194/gmd-16-157-2023
Gwyther D. E., Kerry C., Roughan M., Keating S. R. (2022). Observing system simulation experiments reveal that subsurface temperature observations improve estimates of circulation and heat content in a dynamic western boundary current. Geosci. Model. Dev. 15, 6541–6565. doi: 10.5194/gmd-15-6541-2022
Gwyther D. E., Roughan M., Kerry C., Keating S. R. (2023b). Impact of assimilating repeated subsurface temperature transects on state estimates of a western boundary current. Front. Mar. Sci. 9. doi: 10.3389/fmars.2022.1084784
Haidvogel D. B., Arango H., Budgell W. P., Cornuelle B. D., Curchitser E., Di Lorenzo E., et al. (2008). Ocean forecasting in terrain-following coordinates: Formulation and skill assessment of the Regional Ocean Modeling System. J. Comput. Phys. 227, 3595–3624. doi: 10.1016/j.jcp.2007.06.016
Halliwell G. R., Kourafalou V., Le Hénaff M., Shay L. K., Atlas R. (2015). OSSE impact analysis of airborne ocean surveys for improving upper-ocean dynamical and thermodynamical forecasts in the Gulf of Mexico. Prog. Oceanography 130, 32–46. doi: 10.1016/j.pocean.2014.09.004
Halliwell G. R., Srinivasan A., Kourafalou V., Yang H., Willey D., Le Hénaff M., et al. (2014). Rigorous evaluation of a fraternal twin ocean OSSE system for the open gulf of Mexico. J. Atmospheric Oceanic Technol. 31, 105–130. doi: 10.1175/JTECH-D-13-00011.1
Hernandez-Lasheras J., Mourre B. (2018). Dense CTD survey versus glider fleet sampling: comparing data assimilation performance in a regional ocean model west of Sardinia. Ocean Sci. 14, 1069–1084. doi: 10.5194/os-14-1069-2018
Hernandez-Lasheras J., Mourre B., Orfila A., Santana A., Reyes E., Tintoré J. (2021). Evaluating high-frequency radar data assimilation impact in coastal ocean operational modelling. Ocean Sci. 17, 1157–1175. doi: 10.5194/os-17-1157-2021
Ide K., Courtier P., Ghil M., Lorenc A. C. (1997). Unified notation for data assimilation : operational, sequential and variational (gtSpecial issueltData assimilation in meteology and oceanography: theory and practice). J. Meteorological Soc. Japan 75, 181–189. doi: 10.2151/jmsj1965.75.1B_181
Jakoboski J., Roughan M., Radford J., De Souza J. M. A. C., Felsing M., Smith R., et al. (2024). Partnering with the commercial fishing sector and Aotearoa New Zealand’s ocean community to develop a nationwide subsurface temperature monitoring program. Prog. Oceanography 225, 103278. doi: 10.1016/j.pocean.2024.103278
Janjić T., Bormann N., Bocquet M., Carton J. A., Cohn S. E., Dance S. L., et al. (2018). On the representation error in data assimilation. Quart J. R. Meteoro Soc. 144, 1257–1278. doi: 10.1002/qj.3130
Jansen E., Pimentel S., Tse W.-H., Denaxa D., Korres G., Mirouze I., et al. (2019). Using canonical correlation analysis to produce dynamically based and highly efficient statistical observation operators. Ocean Sci. 15, 1023–1032. doi: 10.5194/os-15-1023-2019
Jin J., Lin H. X., Segers A., Xie Y., Heemink A. (2019). Machine learning for observation bias correction with application to dust storm data assimilation. Atmos. Chem. Phys. 19, 10009–10026. doi: 10.5194/acp-19-10009-2019
Jones E. M., Oke P. R., Rizwi F., Murray L. M. (2012). Assimilation of glider and mooring data into a coastal ocean model. Ocean Model. 47, 1–13. doi: 10.1016/j.ocemod.2011.12.009
Kerry C. G., Powell B. S. (2022). Including tides improves subtidal prediction in a region of strong surface and internal tides and energetic mesoscale circulation. J Geophys Res Oceans. 127, e2021JC018314. doi: 10.1029/2021JC018314
Kerry C., Powell B., Roughan M., Oke P. (2016). Development and evaluation of a high-resolution reanalysis of the East Australian Current region using the Regional Ocean Modelling System (ROMS 3.4) and Incremental Strong-Constraint 4-Dimensional Variational (IS4D-Var) data assimilation. Geosci. Model. Dev. 9, 3779–3801. doi: 10.5194/gmd-9-3779-2016
Kerry C., Roughan M., Azevedo Correia De Souza J. M. (2024b). Assessing the impact of subsurface temperature observations from fishing vessels on temperature and heat content estimates in shelf seas: a New Zealand case study using Observing System Simulation Experiments. Front. Mar. Sci. 11. doi: 10.3389/fmars.2024.1358193
Kerry C. G., Roughan M., Keating S., Gwyther D., Brassington G., Siripatana A., et al. (2024a). Comparison of 4-dimensional variational and ensemble optimal interpolation data assimilation systems using a Regional Ocean Modeling System (v3.4) configuration of the eddy-dominated East Australian Current system. Geosci. Model. Dev. 17, 2359–2386. doi: 10.5194/gmd-17-2359-2024
Kerry C., Roughan M., Powell B. (2018). Observation impact in a regional reanalysis of the east Australian current system. JGR Oceans 123, 7511–7528. doi: 10.1029/2017JC013685
Kerry C., Roughan M., Powell B. (2020). Predicting the submesoscale circulation inshore of the East Australian Current. J. Mar. Syst. 204, 103286. doi: 10.1016/j.jmarsys.2019.103286
Kourafalou V. H., De Mey P., Le Hénaff M., Charria G., Edwards C. A., He R., et al. (2015). Coastal Ocean Forecasting: system integration and evaluation. J. Operational Oceanography 8, s127–s146. doi: 10.1080/1755876X.2015.1022336
Kwon Y., Forman B. A., Ahmad J. A., Kumar S. V., Yoon Y. (2019). Exploring the utility of machine learning-based passive microwave brightness temperature data assimilation over terrestrial snow in high mountain asia. Remote Sens. 11, 2265. doi: 10.3390/rs11192265
Lamouroux J. (2006). Erreurs de prévision d’un modèle océanique barotrope du Golfe de Gascogne en réponse aux incertitudes sur les forçages atmosphériques : caractérisation et utilisation dans un schéma d’assimilation de données à ordre réduit (Theses). Toulouse France: Université Paul Sabatier - Toulouse III.
Lamouroux J., Charria G., De Mey P., Raynaud S., Heyraud C., Craneguy P., et al. (2016). Objective assessment of the contribution of the RECOPESCA network to the monitoring of 3D coastal ocean variables in the Bay of Biscay and the English Channel. Ocean Dynamics 66, 567–588. doi: 10.1007/s10236-016-0938-y
Langland R. H., Baker N. L. (2004). Estimation of observation impact using the NRL atmospheric variational data assimilation adjoint system. Tellus A: Dynamic Meteorology Oceanography 56, 189. doi: 10.3402/tellusa.v56i3.14413
Lary D. J., Alavi A. H., Gandomi A. H., Walker A. L. (2016). Machine learning in geosciences and remote sensing. Geosci. Front. 7, 3–10. doi: 10.1016/j.gsf.2015.07.003
Lary D. J., Zewdie G. K., Liu X., Wu D., Levetin E., Allee R. J., et al. (2018). “Machine learning applications for earth observation,” in Earth observation open science and innovation. Eds. Mathieu P.-P., Aubrecht C. (Springer International Publishing, Cham), 165–218. doi: 10.1007/978-3-319-65633-5_8
Le Hénaff M., De Mey P., Marsaleix P. (2009). Assessment of observational networks with the Representer Matrix Spectra method—application to a 3D coastal model of the Bay of Biscay. Ocean Dynamics 59, 3–20. doi: 10.1007/s10236-008-0144-7
Le Hénaff M., De Mey P., Mourre B., Le Traon P.-Y. (2008). Contribution of a wide-swath altimeter in a shelf seas assimilation system: impact of the satellite roll errors. J. Atmospheric Oceanic Technol. 25, 2133–2144. doi: 10.1175/2008JTECHO576.1
Le Traon P.-Y. (2011). “Satellites and operational oceanography,” in Operational oceanography in the 21st century. Eds. Schiller A., Brassington G. B. (Springer Netherlands, Dordrecht), 29–54. doi: 10.1007/978-94-007-0332-2_2
Levin J., Arango H. G., Laughlin B., Hunter E., Wilkin J., Moore A. M. (2020). Observation impacts on the Mid-Atlantic Bight front and cross-shelf transport in 4D-Var ocean state estimates: Part I — Multiplatform analysis. Ocean Model. 156, 101721. doi: 10.1016/j.ocemod.2020.101721
Levin J., Arango H. G., Laughlin B., Hunter E., Wilkin J., Moore A. M. (2021a). Observation impacts on the Mid-Atlantic Bight front and cross-shelf transport in 4D-Var ocean state estimates: Part II — The Pioneer Array. Ocean Model. 157, 101731. doi: 10.1016/j.ocemod.2020.101731
Levin J., Arango H. G., Laughlin B., Wilkin J., Moore A. M. (2021b). The impact of remote sensing observations on cross-shelf transport estimates from 4D-Var analyses of the Mid-Atlantic Bight. Adv. Space Res. 68, 553–570. doi: 10.1016/j.asr.2019.09.012
Liang J., Terasaki K., Miyoshi T. (2023). A machine learning approach to the observation operator for satellite radiance data assimilation. J. Meteorological Soc. Japan 101, 79–95. doi: 10.2151/jmsj.2023-005
Liu Y., Fu W. (2018). Assimilating high-resolution sea surface temperature data improves the ocean forecast potential in the Baltic Sea. Ocean Sci. 14, 525–541. doi: 10.5194/os-14-525-2018
Liu T., Hirose N. (2022). Comparison of surface and lateral boundary conditions controlled by pseudo-altimeter data assimilation for a regional Kuroshio model. J. Oceanogr 78, 73–88. doi: 10.1007/s10872-021-00629-y
Liu Z.-Q., Rabier F. (2003). The potential of high-density observations for numerical weather prediction: A study with simulated observations. Quart J. R. Meteoro Soc. 129, 3013–3035. doi: 10.1256/qj.02.170
Lorenc A. C. (1986). Analysis methods for numerical weather prediction. Quart J. R. Meteoro Soc. 112, 1177–1194. doi: 10.1002/qj.49711247414
Madec G., the NEMO team (2016). NEMO ocean engine, Note du Pôle de modélisation de l’Institut Pierre-Simon Laplace No 27, (No. ISSN No 1288-1619).
Marmain J., Molcard A., Forget P., Barth A., Ourmières Y. (2014). Assimilation of HF radar surface currents to optimize forcing in the northwestern Mediterranean Sea. Nonlin. Processes Geophys. 21, 659–675. doi: 10.5194/npg-21-659-2014
Marsaleix P., Auclair F., Estournel C. (2006). Considerations on open boundary conditions for regional and coastal ocean models. J. Atmospheric Oceanic Technol. 23, 1604–1613. doi: 10.1175/JTECH1930.1
Marsaleix P., Auclair F., Floor J. W., Herrmann M. J., Estournel C., Pairaud I., et al. (2008). Energy conservation issues in sigma-coordinate free-surface ocean models. Ocean Model. 20, 61–89. doi: 10.1016/j.ocemod.2007.07.005
Miyazawa Y., Varlamov S. M., Miyama T., Guo X., Hihara T., Kiyomatsu K., et al. (2017). Assimilation of high-resolution sea surface temperature data into an operational nowcast/forecast system around Japan using a multi-scale three-dimensional variational scheme. Ocean Dynamics 67, 713–728. doi: 10.1007/s10236-017-1056-1
Moore A. M., Arango H. G., Broquet G., Edwards C., Veneziani M., Powell B., et al. (2011). The Regional Ocean Modeling System (ROMS) 4-dimensional variational data assimilation systems. Part III – Observation impact and observation sensivity in the California Current System. Prog. Oceanography 91, 74–94. doi: 10.1016/j.pocean.2011.05.005
Moore A. M., Arango H. G., Edwards C. A. (2018). Reduced-rank array modes of the california current observing system. JGR Oceans 123, 452–465. doi: 10.1002/2017JC013172
Moore A. M., Arango H. G., Wilkin J., Edwards C. A. (2023). Weak constraint 4D-Var data assimilation in the Regional Ocean Modeling System (ROMS) using a saddle-point algorithm: Application to the California Current Circulation. Ocean Model. 186, 102262. doi: 10.1016/j.ocemod.2023.102262
Moore A. M., Jacox M. G., Crawford W. J., Laughlin B., Edwards C. A., Fiechter J. (2017). The impact of the ocean observing system on estimates of the California current circulation spanning three decades. Prog. Oceanography 156, 41–60. doi: 10.1016/j.pocean.2017.05.009
Moore A. M., Levin J., Arango H. G., Wilkin J. (2021). Assessing the performance of an ocean observing, analysis and forecast System for the Mid-Atlantic Bight using array modes. Ocean Model. 164, 101821. doi: 10.1016/j.ocemod.2021.101821
Moore A., Zavala-Garay J., Arango H. G., Edwards C. A., Anderson J., Hoar T. (2020). Regional and basin scale applications of ensemble adjustment Kalman filter and 4D-Var ocean data assimilation systems. Prog. Oceanography 189, 102450. doi: 10.1016/j.pocean.2020.102450
Morrow R., Carret A., Birol F., Nino F., Valladeau G., Boy F., et al. (2017). Observability of fine-scale ocean dynamics in the northwestern Mediterranean Sea. Ocean Sci. 13, 13–29. doi: 10.5194/os-13-13-2017
Mourre B., Alvarez A. (2012). Benefit assessment of glider adaptive sampling in the Ligurian Sea. Deep Sea Res. Part I: Oceanographic Res. Papers 68, 68–78. doi: 10.1016/j.dsr.2012.05.010
Mourre B., Chiggiato J. (2014). A comparison of the performance of the 3-D super-ensemble and an ensemble Kalman filter for short-range regional ocean prediction. Tellus A: Dynamic Meteorology Oceanography 66, 21640. doi: 10.3402/tellusa.v66.21640
Mourre B., De Mey P., Ménard Y., Lyard F., Le Provost C. (2006). Relative performance of future altimeter systems and tide gauges in constraining a model of North Sea high-frequency barotropic dynamics. Ocean Dynamics 56, 473–486. doi: 10.1007/s10236-006-0081-2
Ngodock H., Carrier M., Fabre J., Zingarelli R., Souopgui I. (2017). A variational data assimilation system for the range dependent acoustic model using the representer method: Theoretical derivations. J. Acoustical Soc. America 142, 186–194. doi: 10.1121/1.4989541
Nguyen-Duy T., Ayoub N. K., De-Mey-Frémaux P., Ngo-Duc T. (2023). How sensitive is a simulated river plume to uncertainties in wind forcing? A case study for the Red River plume (Vietnam). Ocean Model. 186, 102256. doi: 10.1016/j.ocemod.2023.102256
Oke P. R., Allen J. S., Miller R. N., Egbert G. D., Kosro P. M. (2002). Assimilation of surface velocity data into a primitive equation coastal ocean model. J.-Geophys.-Res 107 (C9), 3122. doi: 10.1029/2000JC000511
Oke P. R., Brassington G. B., Griffin D. A., Schiller A. (2008). The Bluelink ocean data assimilation system (BODAS). Ocean Model. 21, 46–70. doi: 10.1016/j.ocemod.2007.11.002
Oke P. R., Larnicol G., Jones E. M., Kourafalou V., Sperrevik A. K., Carse F., et al. (2015). Assessing the impact of observations on ocean forecasts and reanalyses: Part 2, Regional applications. J. Operational Oceanogr. 8, s63–s79. doi: 10.1080/1755876X.2015.1022080
Oke P. R., Sakov P. (2008). Representation error of oceanic observations for data assimilation. J. Atmospheric Oceanic Technol. 25, 1004–1017. doi: 10.1175/2007JTECHO558.1
Paduan J. D., Shulman I. (2004). HF radar data assimilation in the Monterey Bay area. J. Geophys. Res. 109, 2003JC001949. doi: 10.1029/2003JC001949
Pan C., Zheng L., Weisberg R. H., Liu Y., Lembke C. E. (2014). Comparisons of different ensemble schemes for glider data assimilation on West Florida Shelf. Ocean Model. 81, 13–24. doi: 10.1016/j.ocemod.2014.06.005
Pascual A., Ruiz S., Olita A., Troupin C., Claret M., Casas B., et al. (2017). A multiplatform experiment to unravel meso- and submesoscale processes in an intense front (AlborEx). Front. Mar. Sci. 4. doi: 10.3389/fmars.2017.00039
Pasmans I., Kurapov A. L., Barth J. A., Ignatov A., Kosro P. M., Shearman R. K. (2019). Why gliders appreciate good company: glider assimilation in the oregon-washington coastal ocean 4DVAR system with and without surface observations. JGR Oceans 124, 750–772. doi: 10.1029/2018JC014230
Pasmans I., Kurapov A. L., Barth J. A., Kosro P. M., Shearman R. K. (2020). Ensemble 4DVAR (En4DVar) data assimilation in a coastal ocean circulation model. Part II: Implementation offshore Oregon–Washington, USA. Ocean Model. 154, 101681. doi: 10.1016/j.ocemod.2020.101681
Petrenko B., Ignatov A., Kihai Y., Dash P. (2016). Sensor-specific error statistics for SST in the advanced clear-sky processor for oceans. J. Atmospheric Oceanic Technol. 33, 345–359. doi: 10.1175/JTECH-D-15-0166.1
Pimentel S., Tse W.-H., Xu H., Denaxa D., Jansen E., Korres G., et al. (2019). Modeling the near-surface diurnal cycle of sea surface temperature in the mediterranean sea. JGR Oceans 124, 171–183. doi: 10.1029/2018JC014289
Powell B. S. (2017). Quantifying how observations inform a numerical reanalysis of hawaii. JGR Oceans 122, 8427–8444. doi: 10.1002/2017JC012854
Pujol M.-I., Dobricic S., Pinardi N., Adani M. (2010). Impact of multialtimeter sea level assimilation in the mediterranean forecasting model. J. Atmospheric Oceanic Technol. 27, 2065–2082. doi: 10.1175/2010JTECHO715.1
Raghukumar K., Edwards C. A., Goebel N. L., Broquet G., Veneziani M., Moore A. M., et al. (2015). Impact of assimilating physical oceanographic data on modeled ecosystem dynamics in the California Current System. Prog. Oceanography 138, 546–558. doi: 10.1016/j.pocean.2015.01.004
Rainwater S., Bishop C. H., Campbell W. F. (2015). The benefits of correlated observation errors for small scales. Quart J. R. Meteoro Soc. 141, 3439–3445. doi: 10.1002/qj.2582
Ren L., Nash S., Hartnett M. (2016). Forecasting of surface currents via correcting wind stress with assimilation of high-frequency radar data in a three-dimensional model. Adv. Meteorology 2016, 1–12. doi: 10.1155/2016/8950378
Reynolds R. W., Rayner N. A., Smith T. M., Stokes D. C., Wang W. (2002). An improved in situ and satellite SST analysis for climate. J. Climate 15, 1609–1625. doi: 10.1175/1520-0442(2002)015<1609:AIISAS>2.0.CO;2
Robinson A. R., Brink K. H., Ducklow H. W., Jahnke R. A., Rothschild B. J. (2004). “Interdisciplinary multiscale coastal dynamical processes and interactions,” in The global coastal ocean: multiscale interdisciplinary processes, the sea (Cambridge, MA USA and London England: Harvard University Press), 3–35.
Röhrs J., Sperrevik A. K., Christensen K. H. (2018). NorShelf: A reanalysis and data-assimilative forecast model for the Norwegian Shelf Sea. Zenodo. doi: 10.5281/ZENODO.2384124
Ruggiero G. A., Cosme E., Brankart J.-M., Le Sommer J., Ubelmann C. (2016). An efficient way to account for observation error correlations in the assimilation of data from the future SWOT high-resolution altimeter mission. J. Atmospheric Oceanic Technol. 33, 2755–2768. doi: 10.1175/JTECH-D-16-0048.1
Scott K. A., Buehner M., Caya A., Carrieres T. (2012). Direct assimilation of AMSR-E brightness temperatures for estimating sea ice concentration. Monthly Weather Rev. 140, 997–1013. doi: 10.1175/MWR-D-11-00014.1
Shay L. K., Jaimes B., Brewster J. K., Meyers P., McCaskill E. C., Uhlhorn E., et al. (2011). “Airborne ocean surveys of the loop current complex from NOAA WP-3D in support of the deepwater horizon oil spill,” in Geophysical monograph series. Eds. Liu Y., MacFadyen A., Ji Z.-G., Weisberg R. H. (American Geophysical Union, Washington, D. C.), 131–151. doi: 10.1029/2011GM001101
Shay L. K., Uhlhorn E. W. (2008). Loop current response to hurricanes isidore and lili. Monthly Weather Rev. 136, 3248–3274. doi: 10.1175/2007MWR2169.1
Shchepetkin A. F., McWilliams J. C. (2005). The regional oceanic modeling system (ROMS): a split-explicit, free-surface, topography-following-coordinate oceanic model. Ocean Model. 9, 347–404. doi: 10.1016/j.ocemod.2004.08.002
Shulman I., Paduan J. D. (2009). Assimilation of HF radar-derived radials and total currents in the Monterey Bay area. Deep Sea Res. Part II: Topical Stud. Oceanography 56, 149–160. doi: 10.1016/j.dsr2.2008.08.004
Shulman I., Rowley C., Anderson S., DeRada S., Kindle J., Martin P., et al. (2009). Impact of glider data assimilation on the Monterey Bay model. Deep Sea Res. Part II: Topical Stud. Oceanography 56, 188–198. doi: 10.1016/j.dsr2.2008.08.003
Siripatana A., Kerry C., Roughan M., Souza J. M. A. C., Keating S. (2020). Assessing the impact of nontraditional ocean observations for prediction of the east Australian current. JGR Oceans 125, e2020JC016580. doi: 10.1029/2020JC016580
Song H., Edwards C. A., Moore A. M., Fiechter J. (2016). Data assimilation in a coupled physical-biogeochemical model of the California current system using an incremental lognormal 4-dimensional variational approach: Part 3—Assimilation in a realistic context using satellite and in situ observations. Ocean Model. 106, 159–172. doi: 10.1016/j.ocemod.2016.06.005
Sotillo M. G., Cailleau S., Lorente P., Levier B., Aznar R., Reffray G., et al. (2015). The MyOcean IBI Ocean Forecast and Reanalysis Systems: operational products and roadmap to the future Copernicus Service. J. Operational Oceanography 8, 63–79. doi: 10.1080/1755876X.2015.1014663
Stammer D.. (1997). Steric and wind-induced changes in TOPEX/POSEIDON large-scale sea surface topography observations. . Geophys. Res. 102 (C9), 20987–21009. doi: 10.1029/97JC01475
Storto A., De Magistris G., Falchetti S., Oddo P. (2021). A neural network-based observation operator for coupled ocean-acoustic variational data assimilation. Monthly Weather Rev. 149 (6), 1967-1985. doi: 10.1175/MWR-D-20-0320.1
Storto A., Dobricic S., Masina S., Di Pietro P. (2011). Assimilating along-track altimetric observations through local hydrostatic adjustment in a global ocean variational assimilation system. Monthly Weather Rev. 139, 738–754. doi: 10.1175/2010MWR3350.1
Storto A., Falchetti S., Oddo P., Jiang Y., Tesei A. (2020). Assessing the impact of different ocean analysis schemes on oceanic and underwater acoustic predictions. JGR Oceans 125, e2019JC015636. doi: 10.1029/2019JC015636
Storto A., Masina S., Dobricic S. (2013). Ensemble spread-based assessment of observation impact: application to a global ocean analysis system. Quart J. R. Meteoro Soc. 139, 1842–1862. doi: 10.1002/qj.2071
Storto A., Oddo P. (2019). Optimal assimilation of daytime SST retrievals from SEVIRI in a regional ocean prediction system. Remote Sens. 11, 2776. doi: 10.3390/rs11232776
Storto A., Oddo P., Cozzani E., Coelho E. F. (2019). Introducing along-track error correlations for altimetry data in a regional ocean prediction system. J. Atmospheric Oceanic Technol. 36, 1657–1674. doi: 10.1175/JTECH-D-18-0213.1
Talagrand O., Courtier P. (1987). Variational assimilation of meteorological observations with the adjoint vorticity equation. I: Theory. Quart J. R. Meteoro Soc. 113, 1311–1328. doi: 10.1002/qj.49711347812
Taylor K. E. (2001). Summarizing multiple aspects of model performance in a single diagram. J. Geophys. Res. 106, 7183–7192. doi: 10.1029/2000JD900719
Trémolet Y. (2008). Computation of observation sensitivity and observation impact in incremental variational data assimilation. Tellus A: Dynamic Meteorology Oceanography 60, 964. doi: 10.1111/j.1600-0870.2008.00349.x
Turpin V., Remy E., Le Traon P. Y. (2016). How essential are Argo observations to constrain a global ocean data assimilation system? Ocean Sci. 12, 257–274. doi: 10.5194/os-12-257-2016
Vandenbulcke L., Beckers J.-M., Barth A. (2017). Correction of inertial oscillations by assimilation of HF radar data in a model of the Ligurian Sea. Ocean Dynamics 67, 117–135. doi: 10.1007/s10236-016-1012-5
Verrier S., Le Traon P.-Y., Remy E. (2017). Assessing the impact of multiple altimeter missions and Argo in a global eddy-permitting data assimilation system. Ocean Sci. 13, 1077–1092. doi: 10.5194/os-13-1077-2017
Vervatis V. D., De Mey-Frémaux P., Ayoub N., Karagiorgos J., Ghantous M., Kailas M., et al. (2021). Assessment of a regional physical–biogeochemical stochastic ocean model. Part 1: Ensemble generation. Ocean Model. 160, 101781. doi: 10.1016/j.ocemod.2021.101781
While J., Mao C., Martin M. J., Roberts-Jones J., Sykes P. A., Good S. A., et al. (2017). An operational analysis system for the global diurnal cycle of sea surface temperature: implementation and validation. Quart J. R. Meteoro Soc. 143, 1787–1803. doi: 10.1002/qj.3036
Wilkin J. L., Hunter E. J. (2013). An assessment of the skill of real-time models of Mid-Atlantic Bight continental shelf circulation. JGR Oceans 118, 2919–2933. doi: 10.1002/jgrc.20223
Xu J., Zhang A., Kurapov A., Seroka G., Bayler E. (2022). Implementation of the West Coast Operational Forecast System (WCOFS) and the semi-operational nowcast/forecast skill assessment. Silver Spring, MD, USA. doi: 10.25923/A9MJ-ZM71
Xue Y., Forman B. A. (2017). “Integration of satellite-based passive microwave brightness temperature observations and an ensemble-based land data assimilation framework to improve snow estimation in forested regions,” in 2017 IEEE international geoscience and remote sensing symposium (IGARSS) (IEEE, Fort Worth, TX), 311–314. doi: 10.1109/IGARSS.2017.8126958
Yaremchuk M., D’Addezio J. M., Panteleev G., Jacobs G. (2018). On the approximation of the inverse error covariances of high-resolution satellite altimetry data. Quart J. R. Meteoro Soc. 144, 1995–2000. doi: 10.1002/qj.3336
Yu P., Kurapov A. L., Egbert G. D., Allen J. S., Kosro P. M. (2012). Variational assimilation of HF radar surface currents in a coastal ocean model off Oregon. Ocean Model. 49–50, 86–104. doi: 10.1016/j.ocemod.2012.03.001
Keywords: coastal ocean circulation modeling, data assimilation, observation impact, observing system experiment, observing system simulation experiment, array modes
Citation: Edwards CA, De Mey-Frémaux P, Barceló-Llull B, Charria G, Choi B-J, Halliwell GR, Hole LR, Kerry C, Kourafalou VH, Kurapov AL, Moore AM, Mourre B, Oddo P, Pascual A, Roughan M, Skandrani C, Storto A, Vervatis V and Wilkin JL (2024) Assessing impacts of observations on ocean circulation models with examples from coastal, shelf, and marginal seas. Front. Mar. Sci. 11:1458036. doi: 10.3389/fmars.2024.1458036
Received: 01 July 2024; Accepted: 23 September 2024;
Published: 25 October 2024.
Edited by:
Yosuke Fujii, Japan Meteorological Agency, JapanReviewed by:
Naoki Hirose, Kyushu University, JapanCopyright © 2024 Edwards, De Mey-Frémaux, Barceló-Llull, Charria, Choi, Halliwell, Hole, Kerry, Kourafalou, Kurapov, Moore, Mourre, Oddo, Pascual, Roughan, Skandrani, Storto, Vervatis and Wilkin. This is an open-access article distributed under the terms of the Creative Commons Attribution License (CC BY). The use, distribution or reproduction in other forums is permitted, provided the original author(s) and the copyright owner(s) are credited and that the original publication in this journal is cited, in accordance with accepted academic practice. No use, distribution or reproduction is permitted which does not comply with these terms.
*Correspondence: Christopher A. Edwards, Y2Vkd2FyZHNAdWNzYy5lZHU=
Disclaimer: All claims expressed in this article are solely those of the authors and do not necessarily represent those of their affiliated organizations, or those of the publisher, the editors and the reviewers. Any product that may be evaluated in this article or claim that may be made by its manufacturer is not guaranteed or endorsed by the publisher.
Research integrity at Frontiers
Learn more about the work of our research integrity team to safeguard the quality of each article we publish.