- 1Key Laboratory of Oceanic and Polar Fisheries, Ministry of Agriculture and Rural Affairs, P.R.China, East China Sea Fisheries Research Institute, Chinese Academy of Fishery Sciences, Shanghai, China
- 2Laoshan Laboratory of Qingdao Marine Science and Technology Center, Qingdao, China
- 3College of Marine Living Resource Sciences and Management, Shanghai Ocean University, Shanghai, China
- 4College of Navigation and Ship Engineering, Dalian Ocean University, Dalian, China
The chub mackerel (Scomber japonicus) is one of the most influential small pelagic fish in the Northwest Pacific Ocean, and accurate modeling approaches and model selection are critical points in predicting the Scomber japonicus fishing grounds. This study investigated the changes in catches and fishing days on no moonlight and bright moonlight days (2014-2022) and compared the differences in predictive performance between the LightGBM and RF models on three datasets under the two modeling approaches [those based on the light fishing vessels operational characteristics (Approach one) and those not (Approach Two)]. The results were as follows: 1) Stronger moonlight intensity (e.g., full moon) can limit the fishing efficiency of light fishing vessels, with most years showing a trend of a higher percentage of fishing days on bright moonlight days than catches percentage, i.e., no moonlight days resulted in higher catches with lower fishing days; 2) Compared to Modeling Approach Two, under Modeling Approach one, RF model achieved better predictive performance on dataset B, while the LightGBM model achieved better predictive performance on both datasets A and B; 3) Overall, the Approach One achieved more satisfactory prediction performance, with the optimal prediction performance on the complete dataset C improved from 65.02% (F1-score of the RF model, Approach Two) to 66.52% (F1-score of the LightGBM model, Approach Two); 4) Under the optimal modeling approach (Approach One) and the optimal model (LightGBM model), the differences in the importance of the variables on dataset A (no moonlight days) and dataset B (bright moonlight days) were mainly centered on the environmental variables, with CV, SLA, and SSS being the most important in dataset A, and CV, DO, and SLA being the most important in dataset B. This study provides a more scientific and reasonable modeling undertaking for the research of light purse seine fishing vessels, which is conducive to guiding fishermen to select the operating area and operating time of the Scomber japonicus fishery more accurately and comprehensively and realizing the balanced development of fisheries in terms of ecology and economy.
1 Introduction
The Northwest Pacific Ocean, the Food and Agriculture Organization of the United Nations (FAO) Statistical Area 61, is the marine area with the highest potential catches among the 15 global fishing zones classified by FAO (Tian et al., 2019). Due to its distinctive geographic and oceanographic features, it ranks among the most productive fisheries globally (Tian et al., 2022; Yang et al., 2023a). The confluence of the Oyashio Cold Current and the Kuroshio Warm Current in the NW Pacific Ocean produces fronts and eddies of high productivity and complex ocean dynamics, providing abundant bait organisms and favorable environments for pelagic fishes such as neon flying squid (Ommastraphes bartrami), chub mackerel (Scomber japonicus), and the Pacific Sardine (Sardinops melanostictus) (Han et al., 2023; Xing et al., 2022). Pelagic commercial fish stocks with significant ecological and commercial value are the main component of the Northwest Pacific fishery resources (Jang and Cho, 2022; Kang et al., 2018; Shi et al., 2023a; Tian et al., 2022), and they are the main targets of commercial fisheries in several countries, including Japan, Russia, South Korea, and China. Of these, only China’s fishing vessels are mainly concentrated in the high seas, while the remaining countries are in the EEZ in the vicinity of their countries. Noteworthy, Scomber japonicus is the most expensive primary target species (Oozeki et al., 2018; Tong et al., 2022; Yasuda et al., 2023), overwhelmingly dominant in both quantity and quality (Zhao et al., 2022), prioritized for assessment by the North Pacific Fisheries Commission (NPFC) (Cai et al., 2023; Shi et al., 2022), and accounting for 2% of the world’s total finfish catches in 2020 (FAO, 2022).
Scomber japonicus, a small warm-water pelagic fish with high abundance and high food value (Zhao et al., 2023), is widespread in the 0-300 m water layer of the northwestern Pacific (Fan et al., 2020). It feeds mainly on fish, shrimp, and copepods, and competes for food with the Sardinops melanostictus (Han et al., 2023). The Scomber japonicus has a short life cycle and consists mainly of individuals aged 5-7 years (Shi et al., 2023b), but in the last few years, Scomber japonicus aged six years and older have been infrequent in commercial catches (Cai et al., 2022). It is a seasonal and long-distance migratory fish that migrates from south to north in search of prey of optimal size and temperature, usually in the summer to the prey zooplankton-rich waters of Oyashio Current, and in the winter migrates from north to south to the Kuroshio-Oyashio Transitional Zone for overwintering migration (Han et al., 2023; Shi et al., 2023b; Wang et al., 2021b). Scomber japonicus is highly sensitive to the marine environment, including sea surface temperature (SST), and its growth and spatial and temporal distribution are affected by environmental changes associated with climate change (Kanamori et al., 2019; Tian et al., 2022), with large fluctuations in resource abundance and changes in the location of fishing grounds (Han et al., 2023; Okunishi et al., 2020; Wang et al., 2021b). The marine environment of the Northwest Pacific Ocean and the Scomber japonicus fishing grounds have experienced dramatic changes in recent years, which has sparked interest and concern among scholars to accurately predict the Scomber japonicus fishing grounds (Chernienko and Chernienko, 2021; Han et al., 2023; Lee et al., 2018; Okunishi et al., 2020; Xiao, 2022; Yoon et al., 2020).
Predicting fishing grounds is one of the most critical research components in fishery forecasting. Accurately predicting the location of fishing grounds is of great significance to fisheries science, the management of fishery resources, and the reduction of carbon emissions associated with fishing operations (Chen et al., 2022; Han et al., 2023). Providing accurate information on the distribution of fishing grounds for exploitation by fishing vessels will be facilitated by a thorough study of the relationship between the distribution of fishery resources and the marine environment (Tan and Mustapha, 2023). Scomber japonicus are highly sensitive to the marine environment (Chernienko and Chernienko, 2021; Han et al., 2023), and exploring the potential relationship between their catches and the marine environment has become a mainstream approach to constructing predictive models of their fishing grounds. In recent years, with the continuous and in-depth exploration of optimal Scomber japonicus fishing grounds prediction models, more and more scholars have shown the importance of model selection on the performance of fishing grounds prediction and demonstrated the ability of machine learning models to adequately analyze and predict the vast amount of catches data with complex spatio-temporal information (Chernienko and Chernienko, 2021; Han et al., 2023; Xiao, 2022; Yoon et al., 2020). However, few scholars have discussed the impact of data bias on the performance, accuracy, and credibility of the model prediction. All modeling algorithms are based on the assumption of data unbiasedness (Melo-Merino et al., 2020), so machine learning models, which usually have high-quality data requirements, are not an exception (Malde et al., 2020; Yoon et al., 2020). Therefore, the usual lack of rigorous design introduces data bias and degrades model prediction performance.
In fisheries production, the abundance in the observed area can be underestimated or overestimated, influenced by the type of fishing gear (Han et al., 2024). The fishing method of Scomber japonicus fishery in the Northwest Pacific Ocean is mainly light purse seine fishing vessel (Cai et al., 2023; Tian et al., 2019), which is based on the characteristics of the phototropic behavior of pelagic fish and uses light to attract fish to be seined (Shi et al., 2013). While light trapping stands out as one of the most advanced, effective, and successful methods for capturing commercially vital species (Nguyen and Winger, 2019), catch rates of fishing vessels using lights (light fishing vessels) are highly susceptible to lunar phases, and fishery producers reduce the frequency of their operations during bright moonlight days due to lower catch rates (Giri et al., 2019; Groves et al., 2022; Han et al., 2024, 2022; Li et al., 2022; Poisson et al., 2010; Yan et al., 2015). In response to the above, Han et al. (2024) investigated the effect of data bias due to the lunar phase on the predictive performance of different purple flying squid (Sthenoteuthis oualaniensis) fishing grounds prediction models. They pointed out that non-rigorous training set selection can introduce data bias. Therefore, in the Scomber japonicus fishing grounds forecasting modeling study, we must take into account the fact that light fishing vessels have distinct operational characteristics compared to fishing vessels such as trawlers (vulnerability of the catches to the intensity of moonlight). However, few scholars have ventured into studies exploring the effects of the lunar phase on model prediction performance in the field of Scomber japonicus in the Northwest Pacific Ocean.
Machine learning, an indispensable yet dynamic technology, employs algorithms and computational methods to extract insights from data autonomously, obviating the necessity for explicit equations or instructions without making prior assumptions about the nature of the association and with the ability to process noisy data further (Meeanan et al., 2023; Tan and Mustapha, 2023). The quality and quantity of research data play a crucial role in ensuring that machine learning models are effectively trained and achieve satisfactory predictive performance (Han et al., 2024). Therefore, although rigorous training set screening can avoid data bias and improve the model’s prediction performance to a certain extent, it also reduces the amount of data, which poses a challenge for the model to be adequately and effectively trained. In order to construct a highly reliable and accurate prediction model for Scomber japonicus fishing ground, a machine learning model that can better balance the quality and quantity of research data is explored. In the thesis, the Light Gradient Boosting Machine Model (Chernienko and Chernienko, 2021; Gong et al., 2021; Nagano and Yamamura, 2023) and the Random Forest Model (Meeanan et al., 2023; Xing et al., 2022), which have demonstrated satisfactory prediction performance in studies of Scomber japonicus and other fisheries, are selected for comparative studies.
As the cost of fishing increases due to rising labor and oil costs, the construction of fishing grounds prediction models that vary with the spatial and temporal variability of the marine environment is critical to the maintenance and development of fisheries (Yoon et al., 2020). In order to more effectively promote the Scomber japonicus fishery towards low-carbon and low-cost fishing, to reduce the time and fuel spent on searching for the optimal fishing grounds, we focused on the data bias caused by the light fishing vessels operational characteristics, and to reduce the impact of this bias on the construction of the optimal prediction model. The purpose of this study is to compare the predictive effects of models constructed under two modeling approaches based on the light fishing vessels operational characteristics and those that are not based on the light fishing vessels operational characteristics and to delve into the following two aspects: 1) to explore and analyze the changes in the catches of the Scomber japonicus fishery in the northwestern Pacific Ocean in the different periods of operational characteristics (no moonlight days and bright moonlight days) from 2014 to 2022, and 2) investigate how data biases affect model prediction and the significance of environmental variables.
2 Materials and methods
2.1 Data sources
2.1.1 Overview of fisheries data and research datasets
The dataset utilized in the research of the Scomber japonicus fishery was procured from the East China Sea and Pelagic Seas Data Service Center Database. This encompassed fishing logbook records of Chinese commercial light purse seine fishing vessels from 2014 to 2022, spanning the months of March to December. These records were collected from operations conducted in the high seas of the northwestern Pacific Ocean, specifically within the geographical coordinates of 35°-45°N and 145°-165°E. A total of 70,147 fishing vessel operation records were included in this paper, and the study information included the date, operations time, and latitude/longitude coordinates of the start and end of operations, the number of operated nets, and the species composition and quantities of the catch.
In this research, the Scomber japonicus fishery dataset was reorganized into three datasets for further analysis, considering how Han et al. (2022) divided the no moonlight days and bright moonlight days. The study datasets were as follows: dataset A (only no moonlight days fishery data: lunar days 1-10 and 20-30), dataset B (only bright moonlight days fishery data: lunar days 11-19), and dataset C (all days) (Figure 1).
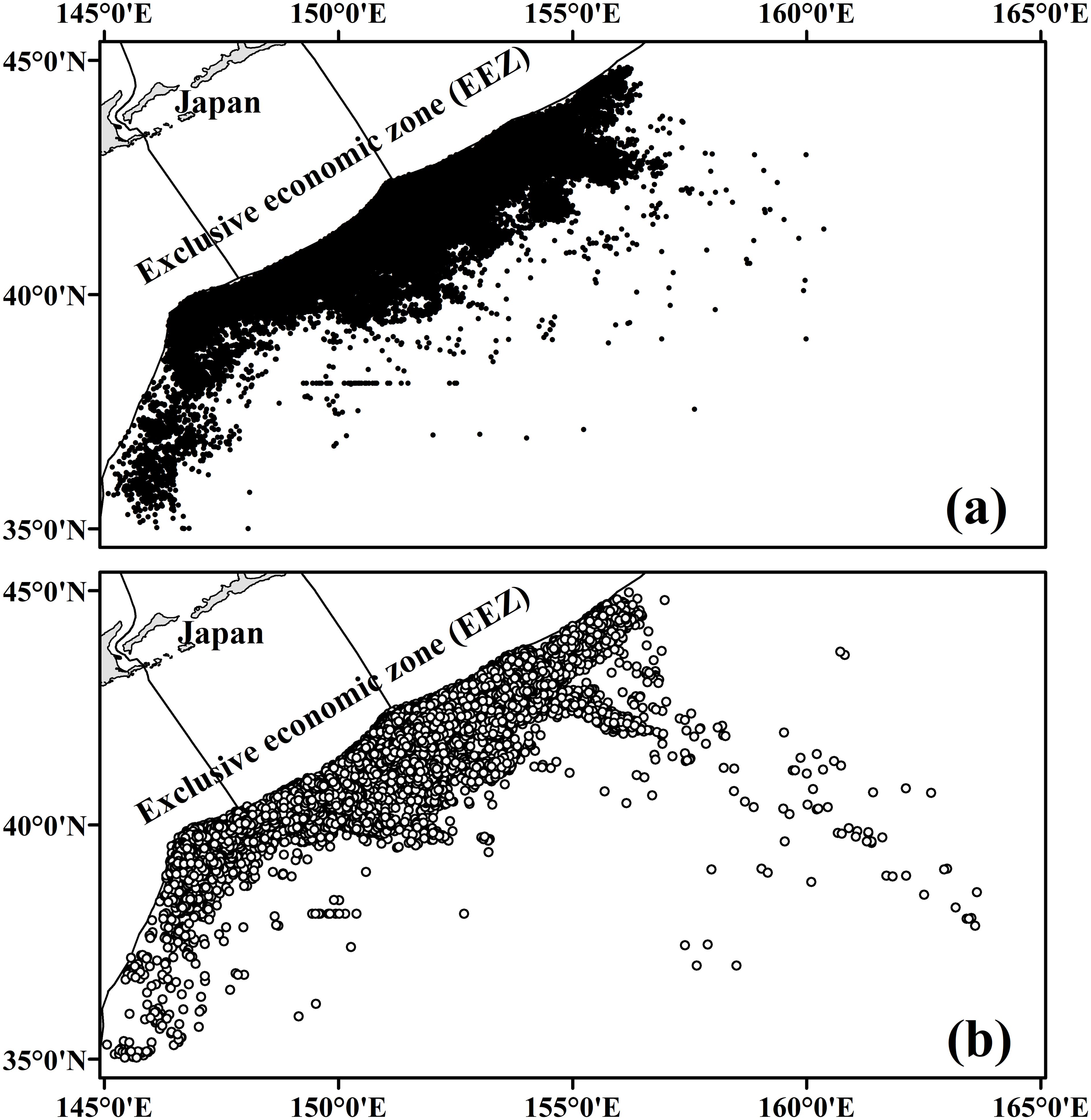
Figure 1. Temporal and spatial distribution of Scomber japonicus catches on the high seas of the Northwest Pacific Ocean on the no moonlight days and bright moonlight days, 2014-2022 [(A) no moonlight days; (B) bright moonlight days].
2.1.2 Selection of marine environment variables and overview of essential information
The number of ocean environment variables is critical to improving the model’s predictive performance and computational efficiency; too few environment variables can lead to a decrease in the model’s predictive performance, but it is worth noting that too many variables can also lead to redundancy, noise, and overfitting (Han et al., 2024). In this research article, we selected six main variables driving changes in the spatial and temporal distribution of Scomber japonicus fishing grounds to construct a forecast model (Chernienko and Chernienko, 2021; Han et al., 2023), namely Sea Surface Temperature (SST, Kelvins), Sea Surface Salinity (SSS, ‰), Chlorophyll-a (Chla, mg/m3), Current Velocity (CV, m/s), Sea Level Anomaly (SLA, m), and Dissolved Oxygen (DO, mmol/m3).
The six marine environmental variables mentioned above have significantly impacted the resources and habitat of Scomber japonicus. SST is widely recognized as having significant effects on the distribution and abundance of resources, habitat location, growth and development, migration, and the catch of Scomber japonicus (Liu et al., 2023). At the same time, temperature variation in seawater is also an important abiotic factor affecting the growth and development of Scomber japonicus (Xiao, 2022); SSS can have an effect on the survival, breeding, and fattening of Scomber japonicus. It also significantly affects the resource abundance and habitat of Scomber japonicus (Liu et al., 2023; Sun et al., 2024); Chla is an essential factor influencing the distribution of Scomber japonicus resources and is a basic indicator for estimating marine productivity. Its concentration is usually used to characterize phytoplankton biomass. It is often used to predict the location of fishing grounds because of its indirect relationship with the distribution of fishing grounds from the perspective of the food chain (Liu et al., 2023; Shi et al., 2023b; Sun et al., 2024; Zhao et al., 2022); The distribution of current velocity in the northwestern Pacific Ocean is complex, with the presence of the Kuroshio Warm Current and its tributaries, which have higher temperatures and salinities, as well as coastal currents with lower salinities. The Oyashio Cold Current and the Kuroshio Warm Current have the most pronounced impact on the resources and habitat of the Scomber japonicus. The Oyashio Cold Current and the Kuroshio Warm Current converge and merge in the Northwest Pacific Ocean, lifting the rich inorganic substances and other nutrients on its seafloor and providing a favorable environment for marine life to reproduce and survive (Liang et al., 2024; Liu et al., 2023; Sun et al., 2024); SLA, Chla and SST data overlaid with fishery data can effectively explore the impact of mesoscale eddies on the Scomber japonicus fishery. The Scomber japonicus fishing grounds were usually around the periphery of warm-core eddies, and these areas are considered to be highly productive due to the occurrence of sea surface depression and divergence or upwelling (Tian et al., 2022). The Scomber japonicus distribution and abundance are susceptible to the influence of DO (Liang et al., 2024; Liu et al., 2023);. In the Kuroshio Extension region and to its north, water masses formed in the winter mixing layer are nearly saturated with oxygen due to their exposure to the atmosphere. When these water masses, enriched with high dissolved oxygen concentrations (DO), separate from the atmosphere, they dip into the main thermocline and subsequently follow the North Pacific Subtropical Circulation path over isodense surfaces in a southwesterly direction (Nagano et al., 2016). Therefore, Scomber japonicus is concentrated in the Kuroshio Extension, and the gravity center of the fishing grounds shifts to the southwest during the winter.
This study used re-analyzed data from the Copernicus Marine Service (https://resources.marine.copernicus.eu/products) as the raw marine environmental data. The time periods were all 2014-2022, with a temporal resolution of days. The spatial ranges were 35°-45°N and 145°-165°E, and the spatial resolutions were all 0.25°×0.25°.
Of these, Vgos and Ugos were used to calculate the ocean-derived variable CV (Han et al., 2023), which is calculated in the following way:
Where, Vgos means surface geostrophic northward sea water velocity and Ugos means surface geostrophic eastward sea water velocity.
2.2 Criteria for the classification of central and non-central fishing grounds
The development of fishing grounds forecasting models with finer temporal and spatial resolution is more beneficial to the fishery in practice. It is more in line with the actual needs of fishery producers (Yoon et al., 2020). However, it is worth noting that a certain amount of data aggregation can reduce data bias, noise, and overfitting, as well as enhance the generalizability and transferability of the model (Zhou et al., 2022). Therefore, in this study, the catches were summarized according to temporal resolution (day) and spatial resolution (0.25°×0.25°), which were defined as fishing grounds.
Northwest Pacific light purse seine fishing vessels are installed with commercial echo-sounder, and before operations, the captains use the marine environment map (e.g., sea surface temperature, etc.) and commercial echo-sounder to search for the most suitable Scomber japonicus fishing grounds for their operations. This step essentially excludes a large number of non-fishing grounds with low catches (Han et al., 2023), so this article only explored the prediction study of the central and non-central fishing grounds. Given that climate, stock condition, and fishing effort vary from year to year, to improve the fit of the model and the validity of the classifications, this study has bi-classified the fishing grounds summarized above by date. The fishing grounds that were greater than or equal to the median catches of fish per day were defined as central fishing grounds (label 1), and the other fishing grounds were defined as non-central fishing grounds (label 0) (Figure 2).
2.3 Modeling and evaluation indicators
2.3.1 Random forest model
The RF model, which uses multiple decision trees to train and predict samples, is an integrated learning method proposed by Breiman (2001) and can accommodate unknown nonlinearities and complex feature interactions with minimal feature engineering (Biggs et al., 2023). It has the advantages of error balancing, high generalization ability, and fault tolerance (Xu et al., 2024). Its results are compelling and are obtained by voting or averaging from multiple weak decision trees (Wang et al., 2021a). It is also a simple, easy-to-implement, and computationally inexpensive algorithm (Zhu et al., 2020) that is faster for processing big data with multidimensional variables. It has almost no parameters to be adjusted (Biggs et al., 2023) and strong adaptability (Han et al., 2022). The RF model is well suited for the quantification of complex non-linear relationships and has been widely used in fisheries research (Brownscombe et al., 2021; Han et al., 2022; Liu et al., 2021) in recent years with the following formula:
In the context of the RF model, Vi denotes the explanatory power of the variable Xi, while Ntree represents the total number of trees, ranging from 1 to 500, as specified in this study. SXi signifies the set of nodes divided by Xi within the Random Forest model comprising Ntree trees. Additionally, G (Xi, V)indicates the Gini information gain associated with Xi at the splitting node v, representing the selection of the explanatory variable that yields the highest information gain.
2.3.2 Light Gradient Boosting Machine Model
LightGBM model is an open-source, efficient, distributed model released by Microsoft in 2017 (Ke et al., 2017), an improved gradient boosting decision tree (GBDT) algorithm to handle large-scale data and high-dimensional features effectively. It is structurally similar to the XGBoost (eXtreme Gradient Boosting) model. However, the LightGBM model constructs the tree in an intelligent way that reduces the computational load and eigenvalues, dramatically improving the model’s computational speed and prediction accuracy (Nagano and Yamamura, 2023; Ouyang et al., 2023; Sun et al., 2020). Due to its high computational efficiency, low memory consumption, and satisfactory accuracy, it has been widely used in recent years for problems such as categorical regression in fisheries research (Chernienko and Chernienko, 2021; Gong et al., 2021; Nagano and Yamamura, 2023; Ospici et al., 2022). The basic idea is to merge M weak regression trees into a single strong regression tree, one after the other, and the calculation formula is as follows (Shakeel et al., 2023):
The setting of hyperparameters can significantly affect the classification effect of the LightGBM model (Jafari and Byun, 2023; Ouyang et al., 2023; Shakeel et al., 2023; Xia et al., 2019; Yang et al., 2023b). At the same time, reasonable hyperparameter settings can also effectively improve the model forecast accuracy and computational efficiency, avoid overfitting, and reduce the time and costs associated with manual trial-and-error (Han et al., 2024). Therefore, the hyperparameters in this paper were set as follows: 1) Number of trees, num_trees: 1-500; 2) Maximum depth of tree, max_depth: 7, 9, 11, 13, 15, 17, 20; 3) Number of leaves for one tree, num_ leaves: 30, 50, 70; 4) learning rate, learning_rate: 0.01, 0.05, 0.1.
2.3.3 Spatiotemporal modeling strategy
Han et al. (2024) showed that the lack of consideration of spatiotemporal information could lead to an inability to accurately assess the impact of data bias caused by the light fishing vessels operational characteristics on model performance. Therefore, considering that neither the RF nor LightGBM models in this study could extract spatiotemporal information, we focused on fitting spatiotemporal with environmental variables.
Feature filtering helps to reduce training time and further optimizes prediction performance (Xia et al., 2019), with correlations between features approaching 1/-1, meaning that some features are redundant for model training (Caponi et al., 2023; Wang et al., 2021a). The Pearson’s correlation coefficients of the fitted variables in this study were tested to be less than 0.9, and there was no collinearity, so they were all retained (Figure 3).
In order to quantitatively assess the performance of the models, in this study, the data were divided into a training dataset (to train the models) and a test dataset (to evaluate the model performance) at 80%:20%. Cross-validation is often the primary method used to evaluate the predictive ability of models in fisheries research, reducing the risk of overfitting the model and producing a more generalized model (Coelho et al., 2020; Han et al., 2024; Meeanan et al., 2023). A grid search method and a 5-fold cross-validation method were used to determine the optimal parameters for each model (Song et al., 2023).
The two modeling approaches in this study were those based on light purse seine vessel operational characteristics (Approach One) and those not based on light purse seine vessel operational characteristics (Approach Two). Approach One: The Dataset C was divided into two subsets, no moonlight days (Dataset A) and bright moonlight data (Dataset B), based on moonlight conditions, and these two subsets were modeled; Approach Two: Unlike Approach A, this approach did not distinguish between no moonlight days and bright moonlight data, but rather modeled and analyzed dataset C as a whole.
2.3.4 Evaluation criteria for model prediction performance
Confusion matrices can be used in machine learning to describe the predictive performance of classification models, especially in statistical classification problems (Daviran et al., 2023). The F1-score is the harmonic average of recall and precision (Han et al., 2023), this research used the F1-score as the only index to evaluate the predictive performance of the fishing grounds prediction model, which was calculated by the following formula:
Where, TP (True Positive): The actual value and the predicted value are the same, both were Label 1; TN (True Negative): Actual and predicted values are the same for the non-central fishing ground (Label 0); FP (False Positive): Actual value labeled 0 was incorrectly predicted to be labeled 1; FN (False Negative): Actual value labeled 1 was incorrectly predicted to be labeled 0.
3 Results
3.1 Changes in Scomber japonicus catches and fishing days in the northwestern Pacific Ocean during periods of no moonlight days and bright moonlight days
As can be seen in Figure 4, the catches and the number of fishing days devoted to bright moonlight days as a percentage of the total catches and fishing days during the period from 2014 to 2022 range from 26.95% to 34.24% and from 28.9% to 32.87%, respectively. The results of this investigation show that the relationship between the number of fishing days and the catches is not positively proportional, e.g., in years such as 2018, a higher percentage of fishing days did not result in an equivalent increase in the catches, suggesting that other factors influenced the catch quantities.
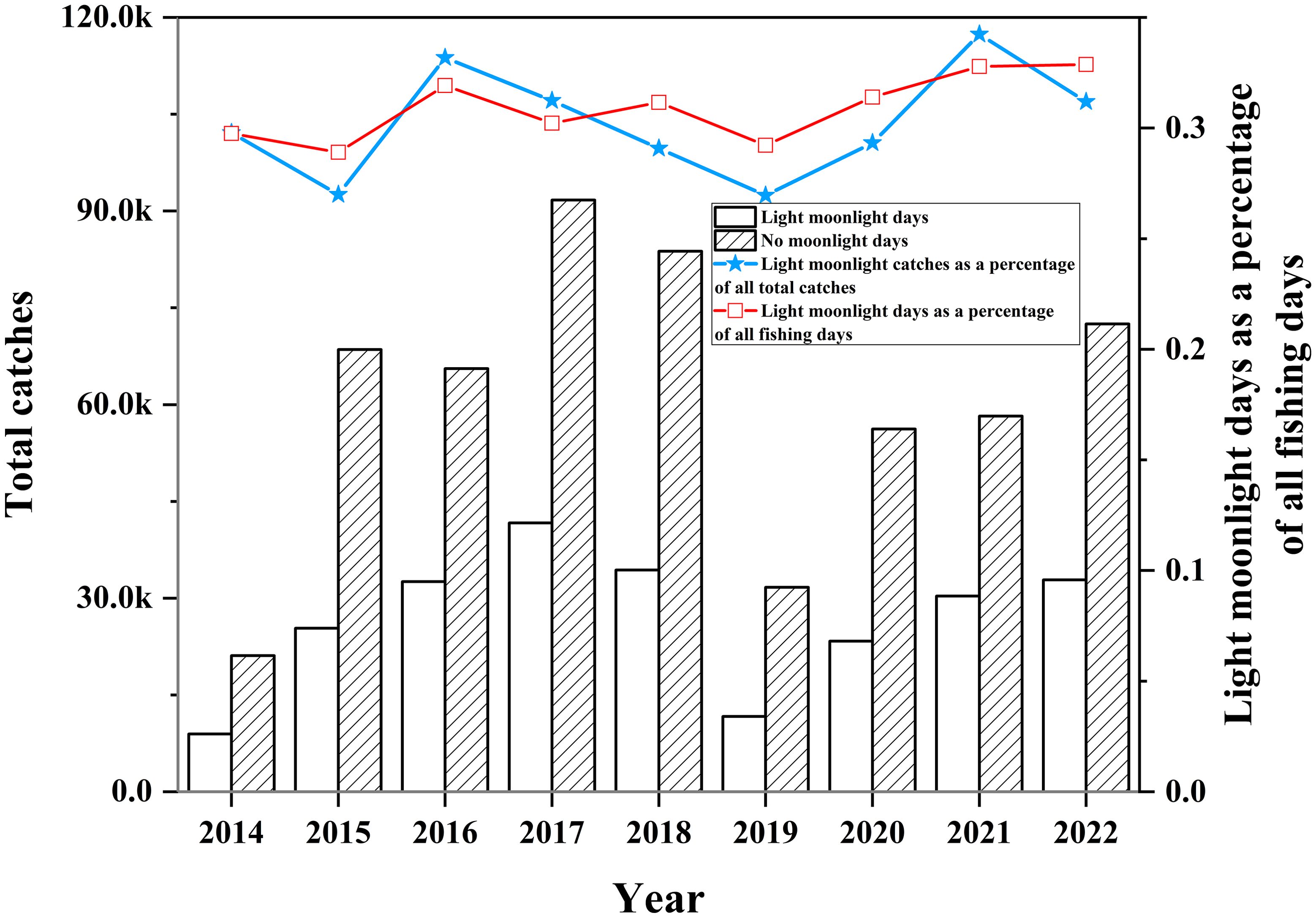
Figure 4. Changes in annual catches and annual fishing days on no moonlight days and bright moonlight days in the Pacific Northwest, 2014-2022.
During the period 2014-2022, in terms of the trends in catch changes between the no moonlight days and bright moonlight days. Although they maintained a generally consistent inter-annual trend, but some differences in the magnitude of changes were still observed (e.g., in 2021, the growth rates of the no moonlight days and bright moonlight days catches were 29.97% and 3.59%, respectively).
3.2 Differences in the results of two models under the two modeling approaches
As shown in Table 1, 1) on the same datasets A, B, and C, the RF and LightGBM models trained using different modeling approaches present different prediction results. After meticulously comparing the F1 scores of various models across different datasets under different modeling approaches, we found that the LightGBM model trained under modeling Approach One achieved the best prediction results on all three datasets; 2) The correct modeling approach (those based on the light fishing vessels operational characteristics) and model selection are crucial for the prediction performance of dataset C. Optimal prediction performance can only be achieved through the correct approach and selection of appropriate models. Choosing the wrong modeling approach may lead to incorrect model selection and thus affect the prediction performance. Overall, Approach One achieved a more satisfactory prediction performance, with the optimal prediction performance on the complete dataset C improving from 65.02% (F1 score of the RF model, approach Two) to 66.52% (F1 score of the LightGBM model, approach One).
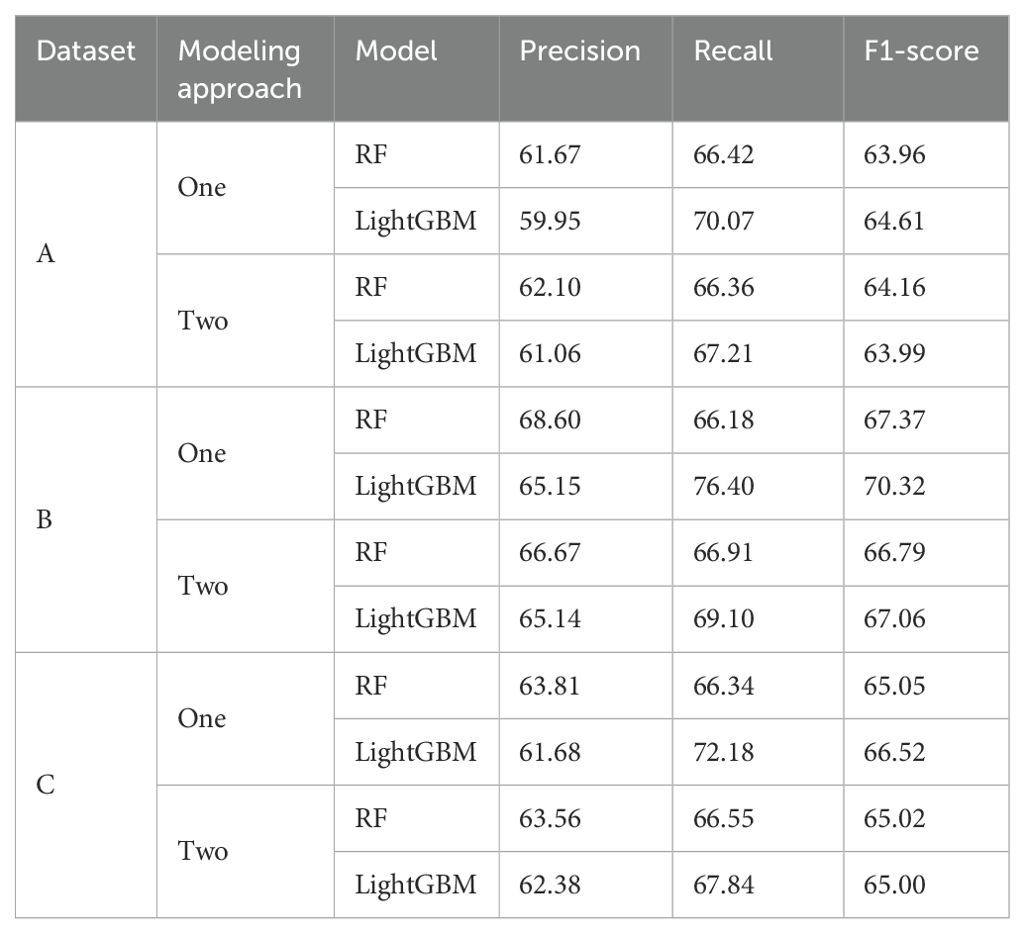
Table 1. Difference in predictive performance between RF and LightGBM models on three datasets under two modeling approaches.
On dataset C, a visual analysis of the prediction-accurate samples under the two modeling approaches revealed that (Figure 5): The RF and LightGBM models predicted the same dataset under the two modeling approaches, and while the samples with accurate predictions were mostly consistent, there was still some degree of prediction discrepancy, which was particularly evident in the predictions of the LightGBM model.
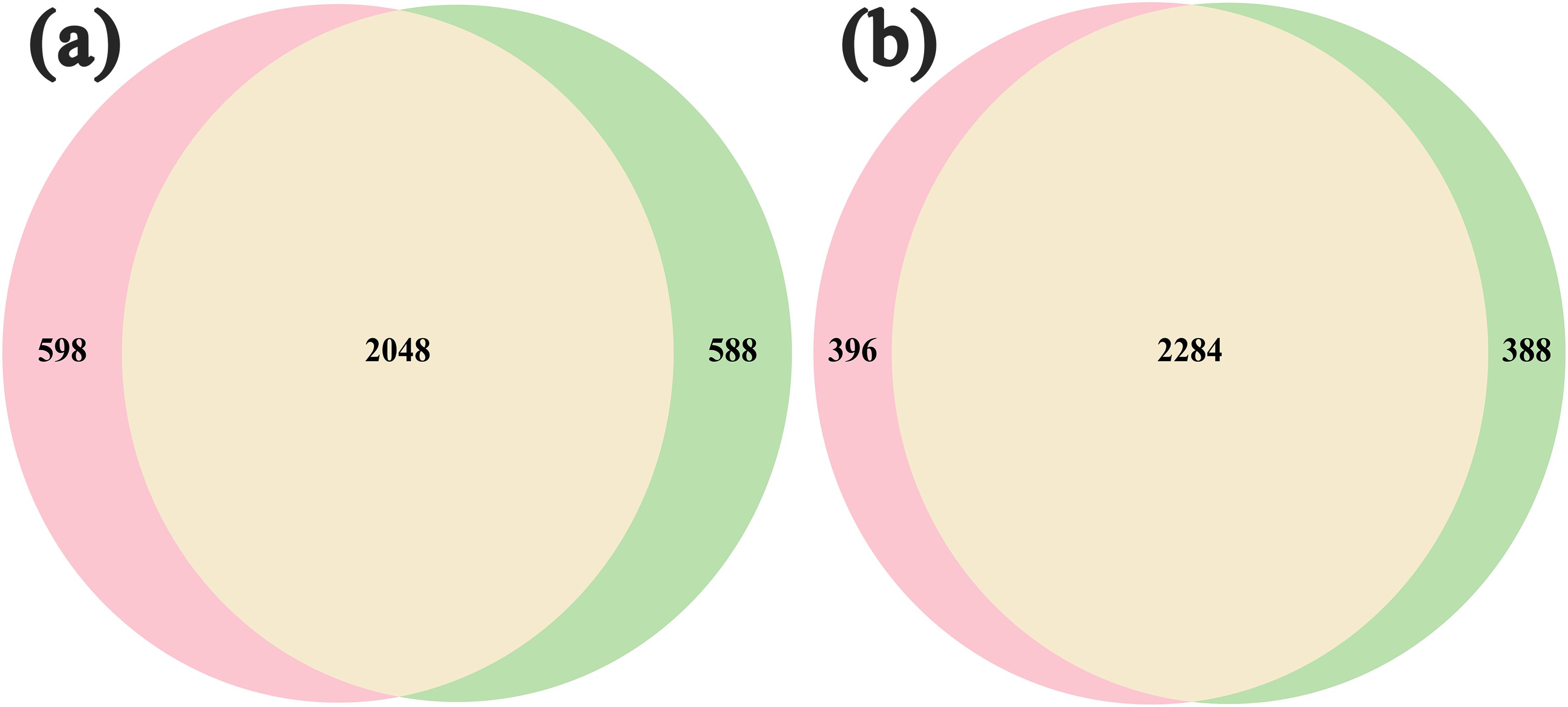
Figure 5. Samples correctly predicted by LightGBM (A) and RF (B) models in both modeling approaches on dataset C (Pink + Yellow: number of samples predicted accurately based on Approach One; Green + Yellow: number of samples predicted accurately based on Approach Two; Yellow: the number of identical samples in the samples accurately predicted by each of Approaches One and Two).
3.3 Difference in variable importance between LightGBM and RF models under two modeling approaches
The importance of datasets A and B was obtained by modeling Approach One, and dataset C was obtained by modeling Approach Two. Figure 6 showed that 1) there is some difference in the importance ranking of variables under the two different modeling approaches. Especially for the LightGBM model, the importance difference is more significant compared to the RF model; 2) in terms of the importance rankings of datasets A, B, and C, there was a higher degree of similarity between datasets A and C; and 3) both modeling approaches showed that the overall importance of environmental variables is greater than that of spatiotemporal variables.
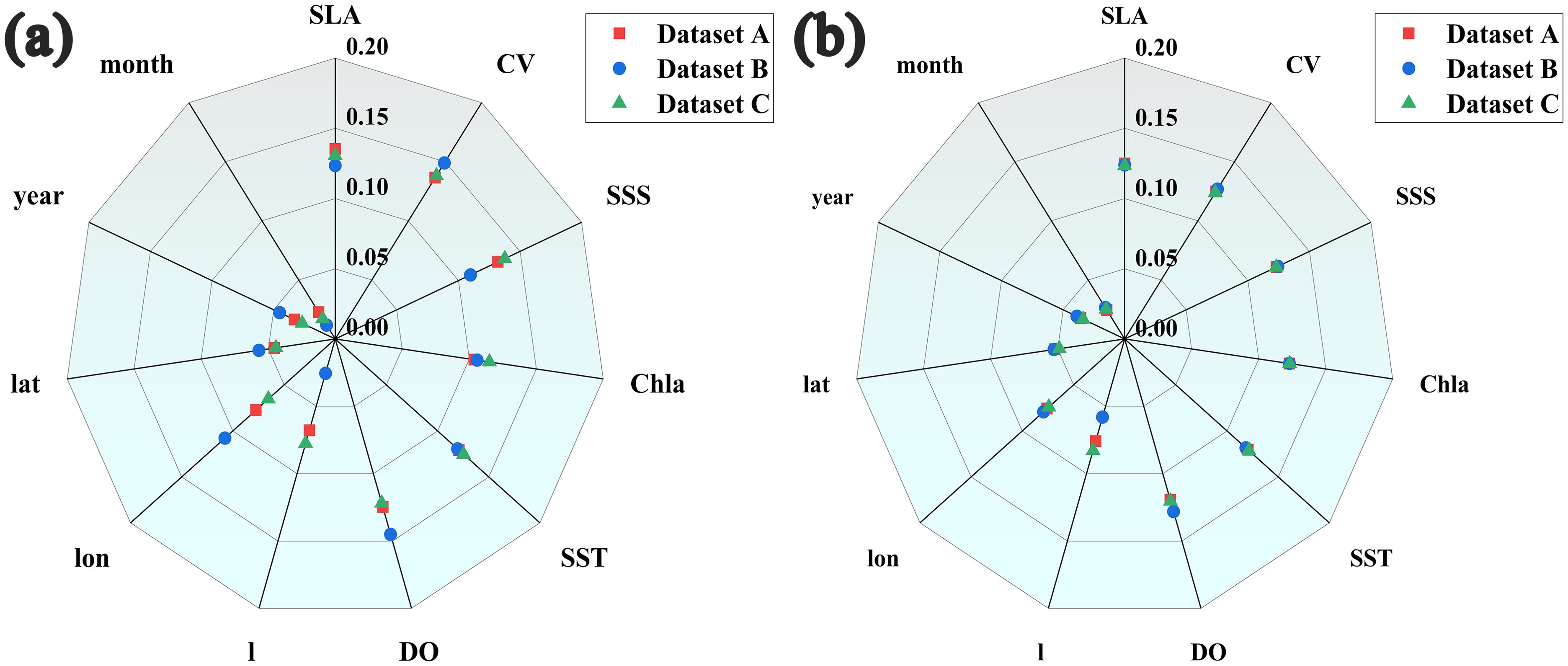
Figure 6. Importance differences on datasets A, B, and C in LightGBM (A) and RF (B) models (1) the farther from the center, the more important; 2) l: lunar phase).
From the optimal model LightGBM model trained by the optimal modeling Approach One (Figure 6A), although there are some similarities in the variables in dataset A and dataset B, the differences should not be ignored. The specific patterns were as follows: 1) From the perspective of environmental variables, the most important ones in dataset A were CV, SLA, and SSS, and the most important ones in dataset B were CV, DO, and SLA. Taken together, the least important one on datasets A and B was Chla, and meanwhile, SST has a weaker influence on the decision of the LightGBM model; 2) From the perspective of temporal variables, year and l were more critical; 3) In terms of spatial variables, both datasets A and B indicate that lon was more important than lat.
4 Discussion
4.1 An analysis of the effect of month relative to Scomber japonicus catches and fishing days in the Northwest Pacific Ocean
Over the period 2014 through 2022, the overall trend showed that the percentage of fishing days on bright moonlight days was higher than the percentage of catches on bright moonlight days in most years. This suggested that fishermen could obtain higher catches with fewer fishing days on no moonlight days. The main reason for this phenomenon is that light fishing vessels induce chub mackerel aggregation mainly using visual stimulation (Lee et al., 2019). However, the catch of the Light Fishing Vessel is easily affected by the lunar phase, especially when the full moon and the moonlight are shone on the water’s surface. Under the effect of moonlight, the effective trapping range of the artificial light source is narrowed, and this difference affects catches (Arifin et al., 2020; Chen et al., 2006). Moonlight intensity affects the vertical migration of pelagic fish, which prefer to stay in deeper waters during the day, rise to surface waters at dusk, feed at night, and then return to deeper waters as daylight approaches (Battaglia et al., 2017). When the moonlight is at its peak, this may have a similar effect to that of sunlight – that fish tend to migrate deeper during the night.
Regarding interannual variability, there are some differences in the percentage of catches and fishing days between no moonlight days and bright moonlight days. The light purse seine in the Northwest Pacific Ocean is less affected by the lunar phase compared to the results of Han et al. (2024) study on light falling gear on the high seas of the Indian Ocean. The influence of lunar phases on different fish species is a complex and multifaceted phenomenon, the magnitude of which is affected by the following factors: cloudiness [thick cloud cover counteracts the effects of intense moonlight (Giri et al., 2019; Milardi et al., 2018)], biomass, phototropic characteristics, and life stages of the main catches (Lee et al., 2019; Yan et al., 2015).
4.2 Predictive performance analysis of models under different modeling approaches
This study further shows that there is no one-size-fits-all modeling approach or model that can bring about better prediction results without considering the light fishing vessels’ operational characteristics and that the correct modeling approach (those based on the light fishing vessels operational characteristics) and model selection are essential for improving the prediction performance of the Scomber japonicus fishing grounds.
The LightGBM model has been shown to improve further the forecasting accuracy of the Scomber japonicus fishing grounds in the Pacific waters of the Russian Federation (Chernienko and Chernienko, 2021), and the LightGBM model trained under modeling Approach one in this study, achieved the best prediction results on all three datasets. However, the LightGBM model did not show an overwhelming trend on three datasets under Approach Two. This is consistent with Guo et al. (2023) conclusion that although the LightGBM model performs well on unbiased data, it does not necessarily perform better than other models when the data are biased. This is mainly due to LightGBM’s tendency to cause overfitting (Xia et al., 2019). In contrast, the RF model focuses primarily on variance reduction. While this helps to reduce the effects of overfitting and improves stability (Xia et al., 2019), the performance of the underlying learners limits RF model accuracy. Thus, the difference in predictive performance under the two modeling approaches was slight. Meanwhile, the LightGBM model can provide a more effective ranking of variable importance than the RF model (Saberi et al., 2022), which may be the main reason why the LightGBM model outperforms the RF model on datasets A and B (under the modeling Approach One).
In this study, there was a slight gap in prediction performance compared to the 3D convolution-al neural networks (3DCNN) model based on temporal scale (month) and spatial scale (1° × 1°) by Han et al. (2023). This is mainly because the fine spatial (0.25° × 0.25°) and temporal scales (day) used in this study are most similar to the needs of fishery production (Yoon et al., 2020). However, they are not the optimal modeling scales for Scomber japonicus (Li et al., 2019) and thus were still affected to a greater extent by data bias (e.g., factors such as differences in decision-making among fishermen and differences in the production capacity of fishing vessels). Therefore, subsequent studies could be based on balancing the prediction performance and production needs and could consider expanding the spatial and temporal scales to some extent to reduce these data biases. On the other hand, the LightGBM model is a non-time-series model, which is prone to overfitting past data and not adapting well to regime shifts (Nagano and Yamamura, 2023). In the later stage, and further research should be conducted by combining the spatiotemporal 3DCNN model (Ji et al., 2013), Convolutional LSTM network (ConvLSTM) model (Shi et al., 2015) and vision Transformer model (Dosovitskiy et al., 2020). However, it is worth noting that models such as 3DCNN require more data to train the model to ensure satisfactory prediction performance.
4.3 Analysis of the significance of the variables
LightGBM model merges mutually exclusive features in fisheries and marine environmental data using a histogram algorithm. It constructs a histogram and traverses the data based on its discrete values to find the optimal split point of the decision tree. Since the decision tree is a weak classifier, the use of a histogram algorithm will have the effect of regularization and can effectively prevent overfitting (Alshboul et al., 2024; Boutahir et al., 2022; Gong et al., 2021; Ke et al., 2020; Saberi et al., 2022). This may be the reason why the LightGBM model has more significant variable importance variability on three datasets than the RF model. Feature importance is mainly dependent on the training dataset (Boutahir et al., 2022), and in this article, the importance was highly similar in datasets A and C. This further illustrates that taking a modeling approach in the Northwest Pacific that does not take into account the operational characteristics of the light fishing vessels can misguide fisheries during the bright moonlight days.
Meeanan et al. (2023), studying the prediction of the short mackerel (Rastrelliger brachysoma) fishing grounds in Thailand (Andaman Sea: 6°N-10°N, 97°E-100°E), noted that spatial variables are more important than environmental variables because there is little variation in temperature, chlorophyll-a, near the coast. Unlike the results of the study by Meeanan et al. (2023), environmental variables were more critical than spatiotemporal variables in this research. This is mainly because this study is on the high seas (sea areas beyond 200 nautical miles from the baseline of the Sea of the Japanese Islands), where environmental variables are considered to be the direct determinants driving changes in the spatial and temporal distribution of the Northwest Pacific offshore Scomber japonicus fishing ground (Han et al., 2023).
In this study, the important variables on datasets A and B were CV, SLA, DO, and SSS, while those that were not important were SST and Chla. Overall, CV was the most important environmental variable affecting the distribution of the center fishing ground. The current velocity (CV) has a crucial impact on the distribution of central fishing grounds (high catches) (Dai et al., 2017; Liu et al., 2023). The Oyashio Cold Current and the Kuroshio Warm Current, which carry materials and energy, converge and merge in the northwest Pacific Ocean, providing a favorable environment for the reproduction and survival of Scomber japonicus (Liang et al., 2024; Liu et al., 2023; Sun et al., 2024). Relative to sea surface height (SSH), SLA overlaid with fisheries data can effectively explore the effects of mesoscale eddies on the Scomber japonicus fishery (Tian et al., 2022). The SLA in this article significantly affects the fishing grounds since Scomber japonicus is a warm-water pelagic fish with habitat water temperatures typically ranging from 10 to 27°C (Han et al., 2023). The “eddy edge habitat” had the highest larval abundance and number of taxa and consisted mainly of coastal pelagic and demersal species (Sánchez-Velasco et al., 2013). The Kuroshio front appears to act as an environmental barrier for fish (Saitoh et al., 1986), so Scomber japonicus fishing grounds are usually located at the edge of warm-core eddies (Tian et al., 2022). However, it is worth noting that the results of Han et al. (2023) based on large spatial and temporal scales (temporal scale: month; spatial scale: 1° × 1°) were contrary to the present study. After an in-depth visualization of a three-dimensional convolutional neural network (3DCNN)-based Scomber japonicus fishing ground forecasting model, Han et al. (2023) revealed that SLA not only has a detrimental effect in predicting the location of the center fishing ground but also that it relative importance was rather limited. This is mainly due to the fact that the differences between SLA are relatively small at monthly time scales, and thus the effect is not significant for categorical projections. DO affects the metabolic rate and swimming speed of Scomber japonicus, while the low-oxygen environment tends to cause the death of eggs and juveniles, which affects the reproduction of fish. Liang et al. (2024) pointed out that DO is one of the critical environmental factors affecting the distribution and abundance of Scomber japonicus in the Northwest Pacific Ocean, which is consistent with the present study. SSS is an important variable affecting fish migration, clustering, and habitat distribution, and it has a large influence on the behavioral characteristics of fish at all stages of growth. This research was consistent with previous studies that although SSS has a significant effect on Scomber japonicus, it is not the most important variable (Sun et al., 2024; Xue et al., 2024).
Chla is a proxy indicator of biomass and productivity, and although Scomber japonicus does not prey primarily on phytoplankton, small- and medium-sized fish located in the middle of the food chain are affected by chla distribution (Smith et al., 1986). Therefore, chla is an important indicator for studying the resources and distribution of Scomber japonicus (Fan et al., 2020; Han et al., 2023; Sun et al., 2024; Zhu et al., 2024). Chla is an extremely important environmental variable at coarse scales (e.g., time scales of months and spatial scales of 1° by 1°) (Han et al., 2023; Sun et al., 2024). However, at fine scales, Chla is not an important variable; the reason Chla was not important may have to do with the fact that Scomber japonicus eats shrimp and copepods (Cui et al., 2021). At the fine scale, the spatial extent covered by the fishing vessels is not large enough (time scale in days). There is only a slight variation in Chla concentration and minor differences in the primary productivity of the sea surface (Song et al., 2020). As a result, the disparity in Chla levels between the central fishing grounds and the non-central fishing grounds in the study is minor, exerting limited influence on the model’s classification performance. SST has an essential effect on the spatial and temporal distribution of the fishing grounds and resource abundance of Scomber japonicus (Xiao, 2022; Zhao, 2022), and in this study, contrary to the conventional view, we found that the effect of sea surface temperature (SST) on predicting the spatial and temporal distribution of the fishing grounds of Scomber japonicus was not the most important. The main reason for this finding was that during the operation of light purse seine fishing vessels, the captains usually choose their fishing locations based on real-time maps of environmental variables and their personal experience. The sea surface temperature values in the central and non-central fishing grounds will be similar to some extent due to this choice (Han et al., 2023). However, when the sea surface temperature has similar values in the central and non-central fishing grounds, it will not be conducive to the prediction of an accurate classification of the fishing grounds. At the same time, it should be noted that there were some differences in the importance of environmental variables on datasets A and B. This is mainly due to the fact that lunar phases may affect fish distribution and behavior, among other factors, through changes in environmental factors such as moonlight intensity and tides (Li et al., 2023; Nguyen and Vang, 2017).
Temporal and spatial variables were ranked almost equally in importance on datasets A and B. Therefore, in the subsequent study, we will mainly strengthen the exploration of environmental variables. By analyzing datasets A and B, we will select the most suitable environmental variables for modeling based on the datasets. Although this study used traditional methods (Importance interpretation method built into the model) to visualize the importance of each variable, it failed to accurately analyze the impact of each sample and each feature on central and non-central fishing grounds prediction, which is undoubtedly a key research direction that is missing in current fishing grounds prediction research and should be further analyzed in conjunction with SHapley Additive exPlanations (SHAP) algorithms based on game theory at a later date (Huang et al., 2024; Wang et al., 2024; Wen et al., 2021).
5 Conclusion
In this study, based on commercial catches data of Scomber japonicus in the Northwest Pacific Ocean from 2014 to 2022, the following two aspects were explored: (1) differences in catches and fishing days under different lunar phases (no moonlight days and bright moonlight days); and (2) differences in predictive performance and importance of variables under two modeling approaches, one based on the light purse seine fishing vessels operational characteristics and the other not based on the light purse seine fishing vessels operational characteristics. The main conclusions were as follows:
1. There is an effect of moonlight intensity on catches, with most years showing a trend of a higher percentage of fishing days on bright moonlight days than catches percentage, i.e., no moonlight days resulted in higher catches with lower operation days.
2. The modeling Approach One (those based on the operational characteristics of light purse seine fishing vessels) achieved more satisfactory prediction performance, with the optimal prediction performance on the complete dataset C improved from 65.02% (F1-score of the RF model, Approach Two) to 66.52% (F1-score of the LightGBM model, Approach One).
3. Under the optimal modeling approach (Approach One) and the optimal model (LightGBM model), the differences in the importance of variables on the dataset A (no moonlight days) and dataset B (bright moonlight days) were mainly centered on environmental variables, with CV, SLA, and SSS being the most important in dataset A, and CV, DO, and SLA being the most important in dataset B.
The finer spatial and temporal scale modeling may provide more accurate and reliable practical production guidance effects for fishing ground prediction decisions. Compared with previous traditional modeling approaches that ignored the possible data bias caused by lunar phase variations, this study recommends a scientifically sound, fine spatial and temporal scale modeling approach to guide fishermen in selecting operating areas and operating times more accurately.
Data availability statement
The raw data supporting the conclusions of this article will be made available by the authors, without undue reservation.
Author contributions
HH: Conceptualization, Software, Validation, Visualization, Writing – original draft, Writing – review & editing. CS: Conceptualization, Software, Validation, Visualization, Writing – original draft, Writing – review & editing. BJ: Software, Validation, Visualization, Writing – review & editing. YW: Data curation, Writing – review & editing. YL: Data curation, Writing – review & editing. DX: Data curation, Writing – original draft. HZ: Funding acquisition, Resources, Supervision, Writing – review & editing. YS: Funding acquisition, Resources, Supervision, Writing – review & editing. KJ: Funding acquisition, Resources, Supervision, Writing – review & editing.
Funding
The author(s) declare that financial support was received for the research, authorship, and/or publication of this article. This work was supported by grants from the Financially supported by the Laoshan Laboratory (LSKJ202201803); the Laoshan Laboratory (No.LSKJ202201804); the National Key Research and Development Program of China (2019YFD0901405, 2022YFC2807504); the Zhejiang ocean fishery resources exploration and capture project (CTZB-2022080076); the particular Fund for Basic Scientific Research Business Expenses of the East China Sea Fisheries Research Institute of the Chinese Academy of Fisheries Sciences at the Central Level for Public Welfare (2021M06); the Shanghai Sailing Program (22YF1459900).
Conflict of interest
The authors declare that the research was conducted in the absence of any commercial or financial relationships that could be construed as a potential conflict of interest.
The reviewer WY declared a shared affiliation with the author HH to the handling editor at the time of review.
Publisher’s note
All claims expressed in this article are solely those of the authors and do not necessarily represent those of their affiliated organizations, or those of the publisher, the editors and the reviewers. Any product that may be evaluated in this article, or claim that may be made by its manufacturer, is not guaranteed or endorsed by the publisher.
References
Alshboul O., Almasabha G., Shehadeh A., Al-Shboul K. (2024). A comparative study of LightGBM, XGBoost, and GEP models in shear strength management of SFRC-SBWS. Structures 61, 106009. doi: 10.1016/j.istruc.2024.106009
Arifin M. K., Hutajulu J., Yusrizal A. S. W., Handri M., Saputra A., Basith A., et al. (2020). The effect of moon phases upon purse seine pelagic fish catches in fisheries management area (FMA) 716, Indonesia. AACL Bioflux 13, 6, 3532–3541.
Battaglia P., Ammendolia G., Cavallaro M., Consoli P., Esposito V., Malara D., et al. (2017). Influence of lunar phases, winds and seasonality on the stranding of mesopelagic fish in the Strait of Messina (Central Mediterranean Sea). Mar. Ecol. 38, e12459. doi: 10.1111/maec.12459
Biggs M., Hariss R., Perakis G. (2023). Constrained optimization of objective functions determined from random forests. Production Operations Manage. 32, 397–415. doi: 10.1111/poms.13877
Boutahir M. K., Farhaoui Y., Azrour M., Zeroual I., Allaoui A. E. (2022). Effect of feature selection on the prediction of direct normal irradiance. Big Data Min. Analytics 5, 309–317. doi: 10.26599/BDMA.2022.9020003
Brownscombe J. W., Midwood J. D., Cooke S. J. (2021). Modeling fish habitat: model tuning, fit metrics, and applications. Aquat. Sci. 83, 44. doi: 10.1007/s00027-021-00797-5
Cai K., Kindong R., Ma Q., Han X., Qin S. (2022). Growth heterogeneity of chub mackerel (Scomber japonicus) in the northwest pacific ocean. J. Mar. Sci. Eng. 10, 301. doi: 10.3390/jmse10020301
Cai K., Kindong R., Ma Q., Tian S. (2023). Stock assessment of chub mackerel (Scomber japonicus) in the northwest pacific using a multi-model approach. Fishes 8, 80. doi: 10.3390/fishes8020080
Caponi M., Cox A., Misra S. (2023). Viscosity prediction using image processing and supervised learning. Fuel 339, 127320. doi: 10.1016/j.fuel.2022.127320
Chen X., Tian S., Qian W. (2006). Effect of moon phase on the jigging rate of Ommastrephes bartrami in the North Pacific. Mar. Fisheries 28, 136–140.
Chen X., Yu W., Wang J. (2022). “Basic principles and methods of fisheries forecasting,” in Theory and Method of Fisheries Forecasting. Ed. Chen X. (Springer Nature Singapore, Singapore), 109–131.
Chernienko E., Chernienko I. (2021). Information support for chub mackerel Scomber japonicus fishery in the Pacific waters of the Russian Federation. Izvestiya TINRO 201, 390–399. doi: 10.26428/1606-9919-2021-201-390-399
Coelho R., Infante P., Santos M. N. (2020). Comparing GLM, GLMM, and GEE modeling approaches for catch rates of bycatch species: A case study of blue shark fisheries in the South Atlantic. Fisheries Oceanography 29, 169–184. doi: 10.1111/fog.12462
Cui G., Zhu W., Dai Q., Li Z., Lu Z., Liu L., et al. (2021). Temporal and spatial distribution of the mackerel fishing ground in the northwest pacific and its relationship with sea surfaceTemperature and chlorophyll concentration. Ocean Dev. Manage. 38, 95–99. doi: 10.20016/j.cnki.hykfygl.2021.08.015
Dai S., Tang F., Fan W., Zhang H., Cui X., Guo G. (2017). Distribution of resource and environment characteristics of fishing ground of Scomber japonicas in the North Pacific high seas. Mar. Fisheries 39, 372–382. doi: 10.13233/j.cnki.mar.fish.2017.04.002
Daviran M., Shamekhi M., Ghezelbash R., Maghsoudi A. (2023). Landslide susceptibility prediction using artificial neural networks, SVMs and random forest: hyperparameters tuning by genetic optimization algorithm. Int. J. Environ. Sci. Technol. 20, 259–276. doi: 10.1007/s13762-022-04491-3
Dosovitskiy A., Beyer L., Kolesnikov A., Weissenborn D., Zhai X., Unterthiner T., et al. (2020). An image is worth 16x16 words: Transformers for image recognition at scale. arXiv preprint arXiv, 11929. doi: 10.48550/arXiv.2010.11929
Fan X., Tang F., Cui X., Yang S., Zhu W., Huang L. (2020). Habitat suitability index for chub mackerel (Scomber japonicus) in the Northwest Pacific Ocean. Haiyang Xuebao 42, 34–43. doi: 10.3969/j.issn.0253–4193.2020.12.004
FAO (2022). The State of World Fisheries and Aquaculture 2022 (Rome: Food and Agriculture Organization of the United Nations).
Giri S., Hazra S., Ghosh P., Ghosh A., Das S., Chanda A., et al. (2019). Role of lunar phases, rainfall, and wind in predicting Hilsa shad (Tenualosa ilisha) catch in the northern Bay of Bengal. Fisheries Oceanography 28, 567–575. doi: 10.1111/fog.12430
Gong P., Wang D., Yuan H., Chen G., Wu R. (2021). Fishing ground forecast model of albacore tuna based on lightGBM in the South Pacific Ocean. Fisheries Sci. 40, 762–767. doi: 10.16378/j.cnki.1003-1111.19292
Guo X., Gui X., Xiong H., Hu X., Li Y., Cui H., et al. (2023). Critical role of climate factors for groundwater potential mapping in arid regions: Insights from random forest, XGBoost, and LightGBM algorithms. Journal of Hydrology 621, 129599. doi: 10.1016/j.jhydrol.2023.129599
Groves V., Sharpe D. M. T., Nkalubo W., Chapman L. J. (2022). Trends in an emerging artisanal fishery of the African cyprinid Rastrineobola argentea in Lake Nabugabo, Uganda. Fisheries Manage. Ecol. 29, 156–168. doi: 10.1111/fme.12527
Han H., Jiang B., Xiang D., Shi Y., Liu S., Shang C., et al. (2024). Comparison of model selection and data bias on the prediction performance of purpleback flying squid (Sthenoteuthis oualaniensis) fishing ground in the Northwest Indian Ocean. Ecol. Indic. 158, 111526. doi: 10.1016/j.ecolind.2023.111526
Han H., Yang C., Jiang B., Shang C., Sun Y., Zhao X., et al. (2023). Construction of chub mackerel (Scomber japonicus) fishing ground prediction model in the northwestern Pacific Ocean based on deep learning and marine environmental variables. Mar. pollut. Bull. 193, 115158. doi: 10.1016/j.marpolbul.2023.115158
Han H., Yang C., Zhang H., Fang Z., Jiang B., Su B., et al. (2022). Environment variables affect CPUE and spatial distribution of fishing grounds on the light falling gear fishery in the northwest Indian Ocean at different time scales. Front. Mar. Sci. 9. doi: 10.3389/fmars.2022.939334
Huang J., Wen H., Hu J., Liu B., Zhou X., Liao M. (2024). Deciphering decision-making mechanisms for the susceptibility of different slope geohazards: A case study on a SMOTE-RF-SHAP hybrid model. J. Rock Mechanics Geotechnical Eng. doi: 10.1016/j.jrmge.2024.03.008
Jafari S., Byun Y. C. (2023). Optimizing battery RUL prediction of lithium-ion batteries based on harris hawk optimization approach using random forest and lightGBM. IEEE Access 11, 87034–87046. doi: 10.1109/ACCESS.2023.3304699
Jang G., Cho G. (2022). Optimal harvest strategy based on a discrete age-structured model with monthly fishing effort for chub mackerel, Scomber japonicus, in South Korea. Appl. Mathematics Comput. 425, 127059. doi: 10.1016/j.amc.2022.127059
Ji S., Xu W., Yang M., Yu K. (2013). 3D convolutional neural networks for human action recognition. IEEE Trans. Pattern Anal. Mach. Intell. 35, 221–231. doi: 10.1109/TPAMI.2012.59
Kanamori Y., Takasuka A., Nishijima S., Okamura H. (2019). Climate change shifts the spawning ground northward and extends the spawning period of chub mackerel in the western North Pacific. Mar. Ecol. Prog. Ser. 624, 155–166. doi: 10.3354/meps13037
Kang M., Hwang B.-K., Jo H.-S., Zhang H., Lee J.-B. (2018). A pilot study on the application of acoustic data collected from a korean purse seine fishing vessel for the chub mackerel. Thalassas: Int. J. Mar. Sci. 34, 437–446. doi: 10.1007/s41208-018-0091-0
Ke G., Meng Q., Finley T., Wang T., Chen W., Ma W., et al. (2017). LightGBM: a highly efficient gradient boosting decision tree. Proceedings of the 31st International Conference on Neural Information Processing Systems, Long Beach, California, USA.
Ke T., Min Q., Xing Z., Jun D., Wu F., Shuaixi L., et al. (2020). Prediction of gaseous nitrous acid based on Stacking ensemble learning model. China Environ. Sci. 40, 582–590. doi: 10.19674/j.cnki.issn1000-6923.2020.0115
Lee D., Oh W., Gim B.-M., Lee J. S., Yoon E., Lee K. (2019). Investigating the effects of different LED wavelengths on aggregation and swimming behavior of chub mackerel (Scomber japonicus). Ocean Sci. J. 54, 573–579. doi: 10.1007/s12601-019-0034-6
Lee D., Son S., Kim W., Park J. M., Joo H., Lee S. H. (2018). Spatio-temporal variability of the habitat suitability index for chub mackerel (Scomber japonicus) in the east/Japan sea and the South Sea of South Korea. Remote Sens. 10, 938. doi: 10.3390/rs10060938
Li C., Shi X., Zhang J., Xiao Y., Huang B., Shi J. (2023). Effects of lunar phases on CPUEs of trawl fisheries based on circular statistics and time series. J. Dalian Ocean Univ. 38, 340–347. doi: 10.16535/j.cnki.dlhyxb.2022-208
Li J., Qiu Y., Cai Y., Zhang K., Zhang P., Jing Z., et al. (2022). Trend in fishing activity in the open South China Sea estimated from remote sensing of the lights used at night by fishing vessels. ICES J. Mar. Sci. 79, 230–241. doi: 10.1093/icesjms/fsab260
Li Y., Chen X., Guo A., Zhou W. (2019). Comparison of habitat suitability index model for Scomber japonicus in different spatial and temporal scales. J. Fisheries China 43, 935–945. doi: 10.11964/jfc.20170410821
Liang X., Wang C., Liu Y., Yu Y., Song C. (2024). Fish diversity analysis of the Kuroshio-Oyashio confluence region in summer based on environmental DNA technology. J. Shanghai Ocean Univ. 33, 911–926. doi: 10.12024/jsou.20230904320
Liu J., Jia M., Feng W., Liu C., Huang L. (2021). Spatial-temporal distribution of Antarctic krill (Euphausia superba) resource and its association with environment factors revealed with RF and GAM models. Periodical Ocean Univ. China 51, 20–29. doi: 10.16441/j.cnki.hdxb.20200243
Liu S., Zhang H., Yang C., Fang Z. (2023). Relationship between stock dynamics and environmental variability for Japanese sardine (Sardinops sagax) and chub mackerel (Scomber japonicus) in the Northwest Pacific Ocean: a review. J. Dalian Ocean Univ. 38, 357–368. doi: 10.16535/j.cnki.dlhyxb.2022-180
Malde K., Handegard N. O., Eikvil L., Salberg A.-B. (2020). Machine intelligence and the data-driven future of marine science. ICES J. Mar. Sci. 77, 1274–1285. doi: 10.1093/icesjms/fsz057
Meeanan C., Noranarttragoon P., Sinanun P., Takahashi Y., Kaewnern M., Matsuishi T. F. (2023). Estimation of the spatiotemporal distribution of fish and fishing grounds from surveillance information using machine learning: The case of short mackerel (Rastrelliger brachysoma) in the Andaman Sea, Thailand. Regional Stud. Mar. Sci. 62, 102914. doi: 10.1016/j.rsma.2023.102914
Melo-Merino S. M., Reyes-Bonilla H., Lira-Noriega A. (2020). Ecological niche models and species distribution models in marine environments: A literature review and spatial analysis of evidence. Ecol. Model. 415, 108837. doi: 10.1016/j.ecolmodel.2019.108837
Milardi M., Lanzoni M., Gavioli A., Fano E. A., Castaldelli G. (2018). Tides and moon drive fish movements in a brackish lagoon. Estuarine Coast. Shelf Sci. 215, 207–214. doi: 10.1016/j.ecss.2018.09.016
Nagano A., Suga T., Kawai Y., Wakita M., Uehara K., Taniguchi K. (2016). Ventilation revealed by the observation of dissolved oxygen concentration south of the Kuroshio Extension during 2012–2013. J. Oceanography 72, 837–850. doi: 10.1007/s10872-016-0386-9
Nagano K., Yamamura O. (2023). Predicting catch of Giant Pacific octopus Enteroctopus dofleini in the Tsugaru Strait using a machine learning approach. Fisheries Res. 261, 106622. doi: 10.1016/j.fishres.2023.106622
Nguyen K., Vang N. (2017). Changing of sea surface temperature affects catch of spanish mackerel scomberomorus commerson in the set-net fishery. Fisheries Aquaculture J. 08, 1-7. doi: 10.4172/2150-3508.1000231
Nguyen K. Q., Winger P. D. (2019). Artificial light in commercial industrialized fishing applications: A review. Rev. Fisheries Sci. Aquaculture 27, 106–126. doi: 10.1080/23308249.2018.1496065
Okunishi T., Yokouchi K., Hasegawa D., Tanaka T., Setou T., Yukami Y., et al. (2020). Relationship between sea temperature variation and fishing ground formations of chub mackerel in the Pacific Ocean off Tohoku. Bull. Japanese Soc. Fisheries Oceanography 84, 271–284. doi: 10.34423/jsfo.84.4_271
Oozeki Y., Inagake D., Saito T., Okazaki M., Fusejima I., Hotai M., et al. (2018). Reliable estimation of IUU fishing catch amounts in the northwestern Pacific adjacent to the Japanese EEZ: Potential for usage of satellite remote sensing images. Mar. Policy 88, 64–74. doi: 10.1016/j.marpol.2017.11.009
Ospici M., Sys K., Guegan-Marat S. (2022). “Prediction of fish location by combining fisheries data and sea bottom temperature forecasting,” in Sclaroff S., Distante C., Leo M., Farinella G.M., Tombari F. (eds) Paper presented at the Image Analysis and Processing – ICIAP 2022, ICIAP 2022. Lecture Notes in Computer Science. vol 13233. Springer, Cham. doi: 10.1007/978-3-031-06433-3_37
Ouyang T., Wang L., Zhu D., Li Y. (2023). Meteorological target classification technology based on lightGBM. Radar Sci. Technol. 21, 621–629. doi: 10.3969/j.issn.1672⁃2337.2023.06.005
Poisson F., Jean-claude G., Taquet M., Jean-pierre D., Bigelow K. (2010). Effects of lunar cycle and fishing operations on longline-caught pelagic fish: Fishing performance, capture time, and survival of fish. Fishery Bull. 108, 268–281.
Saberi A. N., Belahcen A., Sobra J., Vaimann T. (2022). LightGBM-based fault diagnosis of rotating machinery under changing working conditions using modified recursive feature elimination. IEEE Access 10, 81910–81925. doi: 10.1109/ACCESS.2022.3195939
Saitoh S.-i., Kosaka S., Iisaka J. (1986). Satellite infrared observations of Kuroshio warm-core rings and their application to study of Pacific saury migration. Deep Sea Res. Part A. Oceanographic Res. Papers 33, 1601–1615. doi: 10.1016/0198-0149(86)90069-5
Sánchez-Velasco L., Lavín M. F., Jiménez-Rosenberg S. P. A., Godínez V. M., Santamaría-del-Angel E., Hernández-Becerril D. U. (2013). Three-dimensional distribution of fish larvae in a cyclonic eddy in the Gulf of California during the summer. Deep Sea Res. Part I: Oceanographic Res. Papers 75, 39–51. doi: 10.1016/j.dsr.2013.01.009
Shakeel A., Chong D., Wang J. (2023). District heating load forecasting with a hybrid model based on LightGBM and FB-prophet. J. Cleaner Production 409, 137130. doi: 10.1016/j.jclepro.2023.137130
Shi J., Qian W., Yang L. (2013). The theoretical study on suitable spacing between of light purse seine vessels for chub mackerel (Scomber japonicus). South China Fisheries Sci. 9, 82–86. doi: 10.3969/j.issn.2095-0780.2013.04.014
Shi X., Chen Z., Wang H., Yeung D.-Y., Wong W.-K., Woo W.-C. (2015). “Convolutional LSTM Network: a machine learning approach for precipitation nowcasting,” in Paper presented at the Proceedings of the 28th International Conference on Neural Information Processing Systems, Montreal, Canada, Vol. 1, arXiv:1506.04214.
Shi Y., Han H., Tang F., Zhang S., Fan W., Zhang H., et al. (2023a). Evaluation performance of three standardization models to estimate catch-per-unit-effort: A case study on pacific sardine (Sardinops sagax) in the northwest pacific ocean. Fishes 8, 606. doi: 10.3390/fishes8120606
Shi Y., Zhang X., He Y., Fan W., Tang F. (2022). Stock assessment using length-based bayesian evaluation method for three small pelagic species in the northwest pacific ocean. Front. Mar. Sci. 9. doi: 10.3389/fmars.2022.775180
Shi Y., Zhang X., Yang S., Dai Y., Cui X., Wu Y., et al. (2023b). Construction of CPUE standardization model and its simulation testing for chub mackerel (Scomber japonicus) in the Northwest Pacific Ocean. Ecol. Indic. 155, 111022. doi: 10.1016/j.ecolind.2023.111022
Smith R. C., Dustan P., Au D., Baker K. S., Dunlap E. A. (1986). Distribution of cetaceans and sea-surface chlorophyll concentrations in the California Current. Mar. Biol. 91, 385–402. doi: 10.1007/BF00428633
Song L., Li T., Zhang T., Sui H., Li B., Zhang M. (2023). Comparison of machine learning models within different spatial resolutions for predicting the bigeye tuna fishing grounds in tropical waters of the Atlantic Ocean. Fisheries Oceanography 32, 509–526. doi: 10.1111/fog.12643
Song L., Xu H., Chen M., Narcisse E. N. (2020). Relationship between spatiotemporal distribution of chub mackerel and marine environment variables in the waters near Mauritania. J. Shanghai Ocean Univ. 29, 868–877. doi: 10.12024/jsou.20190702746
Sun X., Liu M., Sima Z. (2020). A novel cryptocurrency price trend forecasting model based on LightGBM. Finance Res. Lett. 32, 101084. doi: 10.1016/j.frl.2018.12.032
Sun Y., Zhang H., Jiang K., Xiang D., Shi Y., Huang S., et al. (2024). Simulating the changes of the habitats suitability of chub mackerel (Scomber japonicus) in the high seas of the North Pacific Ocean using ensemble models under medium to long-term future climate scenarios. Mar. pollut. Bull. 207, 116873. doi: 10.1016/j.marpolbul.2024.116873
Tan M. K., Mustapha M. A. (2023). Application of the random forest algorithm for mapping potential fishing zones of Rastrelliger kanagurta off the east coast of peninsular Malaysia. Regional Stud. Mar. Sci. 60, 102881. doi: 10.1016/j.rsma.2023.102881
Tian H., Liu Y., Tian Y., Alabia I. D., Qin Y., Sun H., et al. (2022). A comprehensive monitoring and assessment system for multiple fisheries resources in the Northwest pacific based on satellite remote sensing technology. Front. Mar. Sci. 9. doi: 10.3389/fmars.2022.808282
Tian H., Liu Y., Tian Y., Liu S., Yan L., Chen G., et al. (2019). Detection of Pacific saury (Cololabis saira) fishing boats in the Northwest Pacific using satellite nighttime imaging data. J. Fisheries China 43, 2359–2371. doi: 10.11964/jfc.20181011507
Tong J., Xue M., Zhu Z., Wang W., Tian S. (2022). Impacts of morphological characteristics on target strength of chub mackerel (Scomber japonicus) in the northwest pacific ocean. Front. Mar. Sci. 9. doi: 10.3389/fmars.2022.856483
Wang H., Liang Q., Hancock J. T., Khoshgoftaar T. M. (2024). Feature selection strategies: a comparative analysis of SHAP-value and importance-based methods. J. Big Data 11, 44. doi: 10.1186/s40537-024-00905-w
Wang L., Ma S., Liu Y., Li J., Liu S., Lin L., et al. (2021b). Fluctuations in the abundance of chub mackerel in relation to climatic/oceanic regime shifts in the northwest Pacific Ocean since the 1970s. J. Mar. Syst. 218, 103541. doi: 10.1016/j.jmarsys.2021.103541
Wang A., Xu L., Li Y., Xing J., Chen X., Liu K., et al. (2021a). Random-forest based adjusting method for wind forecast of WRF model. Comput. Geosciences 155, 104842. doi: 10.1016/j.cageo.2021.104842
Wen X., Xie Y., Wu L., Jiang L. (2021). Quantifying and comparing the effects of key risk factors on various types of roadway segment crashes with LightGBM and SHAP. Accident Anal. Prev. 159, 106261. doi: 10.1016/j.aap.2021.106261
Xia H., Wei X., Gao Y., Lv H. (2019). “Traffic prediction based on ensemble machine learning strategies with bagging and lightGBM,” in Paper presented at the 2019 IEEE International Conference on Communications Workshops (ICC Workshops), 2019 IEEE International Conference on Communications Workshops (ICC Workshops), 20-24 May 2019.
Xiao G. (2022). Construction and Comparison of Fishing Ground Forecast Model of Chub mackerel (Scomber japonicus) in Pacific Northwest (China: Shanghai Ocean University). doi: 10.27314/d.cnki.gsscu.2022.000371
Xing Q., Yu H., Liu Y., Li J., Tian Y., Bakun A., et al. (2022). Application of a fish habitat model considering mesoscale oceanographic features in evaluating climatic impact on distribution and abundance of Pacific saury (Cololabis saira). Prog. Oceanography 201, 102743. doi: 10.1016/j.pocean.2022.102743
Xu Y., Dai Y., Guo L., Chen J. (2024). Leveraging machine learning to forecast carbon returns: Factors from energy markets. Appl. Energy 357, 122515. doi: 10.1016/j.apenergy.2023.122515
Xue M., Tong J., Zhu Z., Lyu S. (2024). Modelling of Chub mackerel (Scomber japonicus) habitat in the summer of 2021 in Northwest Pacific Ocean using Acoustic Index Analysis. J. Shanghai Ocean Univ. 33, 974–984. doi: 10.12024/jsou.20240404503
Yan L., Zhang P., Yang L., Yang B., Chen S., Li Y., et al. (2015). Effect of moon phase on fishing rate by light falling-net fishing vessels of Symplectoteuthis oualaniensis in the South China Sea. South China Fisheries Sci. 11, 16–21. doi: 10.3969/j.issn.2095-0780.2015.03.003
Yang C., Han H., Zhang H., Shi Y., Su B., Jiang P., et al. (2023a). Assessment and management recommendations for the status of Japanese sardine Sardinops melanostictus population in the Northwest Pacific. Ecol. Indic. 148, 110111. doi: 10.1016/j.ecolind.2023.110111
Yang H., Chen Z., Yang H., Tian M. (2023b). Predicting coronary heart disease using an improved lightGBM model: performance analysis and comparison. IEEE Access 11, 23366–23380. doi: 10.1109/ACCESS.2023.3253885
Yasuda T., Kinoshita J., Niino Y., Okuyama J. (2023). Vertical migration patterns linked to body and environmental temperatures in chub mackerel. Prog. Oceanography 213, 103017. doi: 10.1016/j.pocean.2023.103017
Yoon Y.-J., Cho S., Kim S., Kim N., Lee S.-J., Ahn J., et al. (2020). An artificial intelligence method for the prediction of near- and off-shore fish catch using satellite and numerical model data. Korean J. Remote Sens. 36, 41–53. doi: 10.7780/KJRS.2020.36.1.4
Zhao G. (2022). Study on Fishery Biology and Fishing Ground Changes of Chub Mackerel (Scomber Japonicus) In The High Seas of the Northwest Pacific. doi: 10.27314/d.cnki.gsscu.2022.000901
Zhao G., Chen J., Zhang H., Tang F., Chen Y., HE J. (2023). Biological characteristics of Scomber japonicus in the high seas of the Northwest Pacific. Mar. Fisheries 45, 385–402. doi: 10.13233/j.cnki.mar.fish.2023.04.008
Zhao G., Shi Y., Fan W., Cui X., Tang F. (2022). Study on main catch composition and fishing ground change of light purse seine in Northwest Pacific. South China Fisheries Sci. (China: Shanghai Ocean University) 18, 33–42. doi: 10.12131/20210086
Zhou X., Ma S., Cai Y., Yu J., Chen Z., Fan J. (2022). The influence of spatial and temporal scales on fisheries modeling—An example of sthenoteuthis oualaniensis in the nansha islands, South China Sea. J. Mar. Sci. Eng. 10, 1840. doi: 10.3390/jmse10121840
Zhu Y., Xu W., Luo G., Wang H., Yang J., Lu W. (2020). Random Forest enhancement using improved Artificial Fish Swarm for the medial knee contact force prediction. Artificial Intelligence in Medicine 103, 101811. doi: 10.1016/j.artmed.2020.101811
Keywords: lunar phase, machine learning, Northwest Pacific Ocean, Scomber japonicus, data bias
Citation: Han H, Shang C, Jiang B, Wang Y, Li Y, Xiang D, Zhang H, Shi Y and Jiang K (2024) A new modeling strategy for the predictive model of chub mackerel (Scomber japonicus) central fishing grounds in the Northwest Pacific Ocean based on machine learning and operational characteristics of the light fishing vessels. Front. Mar. Sci. 11:1451104. doi: 10.3389/fmars.2024.1451104
Received: 18 June 2024; Accepted: 24 September 2024;
Published: 14 October 2024.
Edited by:
Stephen J. Newman, Western Australian Fisheries and Marine Research Laboratories, AustraliaReviewed by:
Wei Yu, Shanghai Ocean University, ChinaRobinson Mugo, Regional Centre for Mapping of Resources for Development, Kenya
Copyright © 2024 Han, Shang, Jiang, Wang, Li, Xiang, Zhang, Shi and Jiang. This is an open-access article distributed under the terms of the Creative Commons Attribution License (CC BY). The use, distribution or reproduction in other forums is permitted, provided the original author(s) and the copyright owner(s) are credited and that the original publication in this journal is cited, in accordance with accepted academic practice. No use, distribution or reproduction is permitted which does not comply with these terms.
*Correspondence: Heng Zhang, emhhbmd6aXFpYW4wNjAxQDE2My5jb20=; Yongchuang Shi, c3ljMTMwNTIzMjYwOTFAMTYzLmNvbQ==; Keji Jiang, amlhbmdrakBlY3NmLmFjLmNu
†These authors have contributed equally to this work