- 1Brain Korea 21 School of Earth Environmental Systems, Pusan National University, Busan, South Korea
- 2Center of Remote Sensing and Geographic Information System, Korea Polar Research Institute, Incheon, South Korea
- 3Department of Oceanography, Pusan National University, Busan, South Korea
- 4National Center for AgroMeteorology, Seoul National University, Seoul, South Korea
Reduced amounts of aerosols blowing into the Yellow Sea (YS), owing to the temporary lockdown of factories in China during COVID-19, resulted in a 15% decrease in spring chlorophyll-a concentration (CHL) in March 2020 compared to its mean March values from 2003 to 2021. Particularly, the effect of land-based AOD is insignificant compared with that of atmospheric aerosols flowing into the YS, as indicated by the currents and wind directions. Hence, the main objective of this study was to understand the relationship between atmospheric aerosols and CHL by quantitatively considering relevant environmental changes using a Random Forest (RF) algorithm. Various input physical forcing variables to RF were employed, including aerosol optical depth (AOD), sea surface temperature (SST), mixed layer depth (MLD), wind divergence (WD), and total precipitation (TP). From the RF-based analysis, we estimated the relative contribution of each physical forcing variable to the difference in CHL during and after the COVID-19 lockdown period. The sensitivity of the RF model to changes in aerosol levels indicated positive effects of increased amounts of aerosols during spring blooms. Additionally, we calculated the quantitative contribution of aerosols to CHL changes. When SST was warmer and TP was lower than their climatology in March 2020, CHL increased by 0.22 mg m-3 and 0.02 mg m-3, respectively. Conversely, when MLD became shallower and AOD was lower than their climatology, CHL decreased as much as 0.01 mg m-3 and 0.20 mg m-3. Variations in WD caused no significant change in CHL. Overall, the specific estimations for reduced spring blooms were caused by a reduction in aerosols during the COVID-19 lockdown period. Furthermore, the RF developed in this study can be used to examine CHL changes and the relative role of significant environmental changes in biological blooms in the ocean for any normal year.
1 Introduction
The outbreak of Coronavirus disease 2019 (COVID-19) in Wuhan, Hubei Province, China, was officially reported to the World Health Organization on December 31, 2019 (https://covid19.who.int). At the end of January 2020, public transportation in Wuhan was closed to minimize the spread of the virus. Furthermore, industrial activities at manufacturing plants were temporarily shut down in most provinces in China, including Hubei, Zhejiang, Xinjiang, Hebei, Shannxi, and Henan. This lockdown was decontrolled in early April 2020, which is referred to as the COVID-19 lockdown period (Bauwens et al., 2020; Shakoor et al., 2020). Many studies have reported improved air quality from the reduced anthropogenic aerosol emissions, owing to the enforcement of the COVID-19 lockdown policy (He et al., 2020; Timmermann et al., 2020). Generally, aerosols in the atmosphere are anthropogenic in origin as they are mainly from emissions from Chinese factory operations, motor vehicle pollution, and seasonal dust such as Asian dust (Yu et al., 2013; Park et al., 2017). Aerosols from China can reach the North Pacific Ocean through the Korean Peninsula via the westerlies. Specifically, the region most affected by aerosols in the North Pacific Ocean is the Yellow Sea (YS), which is located on the western Korean Peninsula adjacent to northeastern China (Kim et al., 2009; Qi et al., 2013; Zhang et al., 2013; Lee et al., 2019). This is because this area of China has many industrial cities (Li et al., 2003; Wang and Su, 2020; Yoon et al., 2021). Aerosols can influence long-range transboundary air pollution (Oh et al., 2015; Lim et al., 2019) and ocean–atmosphere interactions (Tao et al., 2012; Liu et al., 2013; Kripalani et al., 2022). In addition, aerosols scatter radiance from solar radiation in short wavelengths, which can disrupt the radiative energy balance of the earth–atmosphere system. This process leads to low sea surface temperatures (Yue et al., 2011; Kripalani et al., 2022) or makes smaller cloud droplets, thereby reducing precipitation Cheng et al. (2021). However, humid environments can also increase precipitation (Khain et al., 2008).
Anthropogenic nitrogen (NOx and NHy) in aerosols can be considered an important factor that can change the marine ecosystem when deposited into the ocean (Tan et al., 2011; Liu et al., 2013; Wei et al., 2015; Moon et al., 2021). The supply of nitrogen, a limiting factor, to the ocean temporarily promotes phytoplankton growth and increases primary production in the YS (Wang et al., 2003; Mahowald et al., 2005; Zhang et al., 2010; Liu et al., 2013; Mahowald et al., 2018). Kang et al. (2017) showed that phytoplankton’s biochemical composition, including the carbohydrates, proteins, and lipids, may change upon exposure to the additional supply of nitrogen from the atmosphere (Moon et al., 2021). Additionally, changes in the biochemical composition of phytoplankton, which comprise the first trophic level of the food web, may be influenced by higher trophic levels (e.g., biomass and productivity of fishery resources) (Kang et al., 2017).
Liu et al. (2013) and Qi et al. (2013) investigated the marine ecosystem using in-situ measurements after aerosols were imported into the ocean. Since then, many studies have used satellite data to monitor and evaluate the effects of continuous and long-range aerosol transport in near real-time and wide-area to complement the limitations of field observations. Using aerosol optical depth (AOD) from the MODerate Resolution Imaging Spectroradiometer (MODIS)-Terra, Lien et al. (2017) reported that phytoplankton blooms increase when Asian dust increases. Yoon et al. (2019) also used the aerosol index (AI) provided by the Visible Infrared Imaging Radiometer Suite (VIIRS) to investigate the increase in spring blooms in the North Pacific owing to the increase in Asian dust (Tan et al., 2011). Through many studies conducted over a long period of time, we attempted to confirm the effect of aerosols on marine ecosystems. However, previous studies have mainly focused on identifying the relative relationships among the contributing factors of the phytoplankton blooms, rather than on the quantitative contribution of each factor (Tan and Shi, 2012a; Yoon et al., 2021). As a result, previous studies have been limited in their ability to evaluate the quantitative contribution of aerosols to marine ecosystems in the YS.
In general, phytoplankton blooms can be estimated using the chlorophyll-a concentration (CHL), which indicates phytoplankton biomass (Arteaga et al., 2016). CHL is not only affected by aerosols, but also by physical forcing variables, such as sea surface temperature (SST) (Zhao et al., 2019) and mixed layer depth (MLD) (Tan and Shi, 2012b; Lu et al., 2021). An increase in photosynthetically active radiation (PAR) may increase SST, resulting in shallow MLD. The effects of each factor on CHL are as follows. Phytoplankton growth has a positive relationship with SST that reaches its highest peak when SST is between 9°C and 13°C; however, it has a negative relationship when SST is over 13°C (Xu et al., 2013; Zhao et al., 2019; Lu et al., 2021). PAR also has a positive relationship with CHL because CHL increases as light intensity increases (Tan and Shi, 2012b); however, phytoplankton growth declines when it exceeds a threshold intensity level. Furthermore, the deepening of the MLD, owing to more intense wind speeds, increases the CHL (Tan and Shi, 2012b; Lu et al., 2021), whereas the decrease in anthropogenic aerosols is correlated with a reduction in spring phytoplankton biomass (Yoon et al., 2021). In addition, precipitation deposits aerosols directly into the ocean, thereby increasing phytoplankton growth (Tan and Shi, 2012a). However, some studies have reported that precipitation can inhibit phytoplankton growth through metal components, such as Cu and Pb, within rain drops (Paytan et al., 2009; Liu et al., 2013).
Thus, CHL changes relate to various physical parameters, as discussed. Hence, many studies have investigated the complex effects on CHL using machine learning (ML). ML is a technique that can solve nonlinear relationships and complex interactions between ecological factors, such as CHL and physical variables, rather than by using linear statistical methods (Kotsiantis et al., 2006; Cutler et al., 2007). Cheng et al. (2021) reported that the accuracy of the random forest (RF) model in predicting phytoplankton blooms, using multiple predictors, was higher than that of other the ML methods such as support vector machines and decision trees (Liu and Wu, 2017). Park et al. (2019) also found that the CHL reconstructed for multiple variables through the RF model more accurately simulated spatial patterns and CHL values when compared with other techniques. In addition, Park et al. (2020) qualitatively and quantitatively studied the effects of various factors on CHL through RF.
Anthropogenic aerosol emissions into the atmosphere have declined due to the COVID-19 lockdown policy in China. This phenomenon may provide a natural experimental environment that can facilitate the quantitative evaluation of changes in environmental conditions, including the effects of reducing anthropogenic aerosols, which can cause changes in the marine ecosystem. In doing so, we required an answer for the following scientific question: what happened during spring blooms in the YS under reduced aerosol levels (especially anthropogenic aerosols) during the COVID-19 lockdown? To answer this critical question, we examined the contributions of all relevant environmental changes to phytoplankton blooms in the YS based on the RF model using satellite ocean color data (Sections 3.1 to 3.3). Furthermore, we estimated the quantitative contribution of aerosols to CHL through partial dependence (PD) analysis of the RF model (Sections 3.4 to 3.5).
2 Data and Methodology
2.1. Study Area
The YS, located between China and Korea, is a semi-enclosed marginal sea in the Pacific Northwest with an average depth of 44 m (Jin et al., 2013). The YS is predominantly affected by aerosols, as it is near the northeastern part of China where there is significant industrial activity. As a result, this area is influenced by atmospheric nitrogen deposition and it exhibits high primary productivity (Yoon et al., 2021). In addition, the YS is influenced by river inflows containing suspended sediment. This study examined the influence of aerosols on phytoplankton growth in an area that minimizes the effects of river discharge and suspended sediment. The central region of the YS (Central Yellow Sea [CYS]; 121.5–125.5°E, 34–37°N) was selected (Figure 1) (Shi et al., 2017; Fu et al., 2018) because it was the region where spring blooms mainly occurred in the YS (Xu et al., 2013). In addition, the sources of land-based AOD in the study area did not make a substantial contribution to atmospheric aerosols in the CYS, as per the ocean currents and wind directions. Hence help intensively investigate the effect of aerosols on the CHL. According to Xu et al. (2013), spring blooms in the CYS were defined as a CHL higher than 1.2 mg m-3.
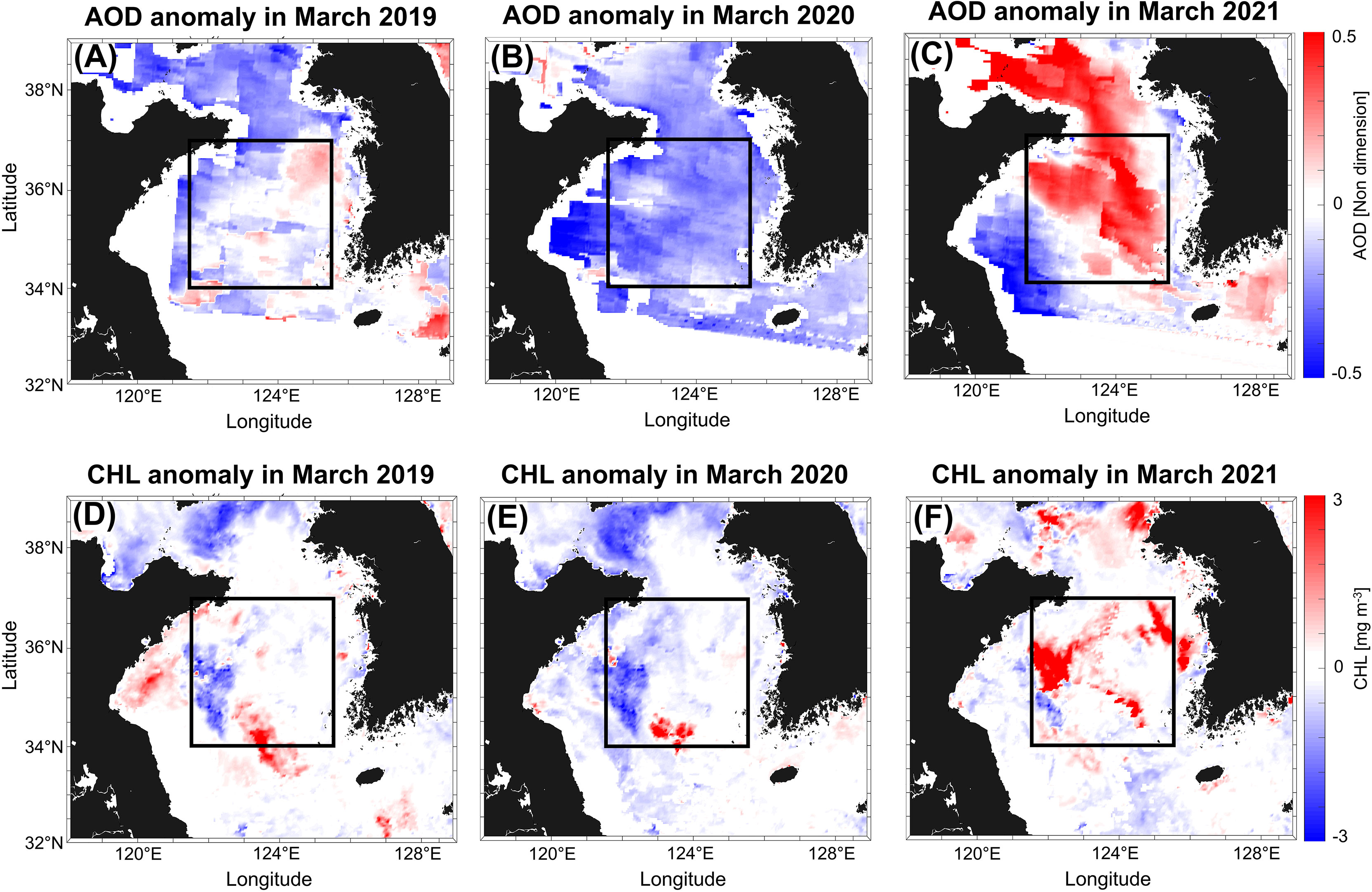
Figure 1 Central Yellow Sea (CYS), was analyzed to determine AOD (A–C) and CHL (D–F) anomalies in March 2019, 2020, and 2021.
2.2.Data
2.2.1. AOD Data
The MODIS-Terra aerosol product provided daily observations for bright land surfaces based on the Deep Blue algorithm and daily observations of AOD at the global scale. This was performed at 0.55 μm over vegetated land (Kaufman et al., 1997; Levy et al., 2013) and ocean surfaces (Tanré et al., 1997; Levy et al., 2013) based on the Dark Target land and Dark Target ocean algorithms, respectively (Hsu et al., 2004; Hsu et al., 2006; Hsu et al., 2013). AOD datasets, combined with Deep Blue and Dark Target algorithms (Hsu et al., 2013; Levy et al., 2013), were obtained from Ocean Color (https://lads-web.modaps.eosdis.nasa.gov). Its temporal and spatial resolutions are daily and 10 km × 10 km (at nadir), respectively, as provided by Level-2 MODIS-Terra. On comparing various satellite observations with AERONET around the CYS, the AOD from MODIS-Terra was more accurate than other sensor measurements.
Anthropogenic aerosols from northeastern China were considered to be the main factor influencing AOD during the lockdown because the emergence of Asian dust did not occur in March 2020. Yoon et al. (2021) found that the AOD in March 2020 was predominantly influenced by aerosols originating from northern China. In addition, it is worth noting that while there are strong coastal currents and thus very weak intrusion to the CYS in spring season (March to May), strong southwesterly winds toward the CYS usually transport aerosol in this season. Thus, aerosols transported from coastal regions into the CYS are likely minimal. Hence, this study considered only aerosols generated in the industrialized regions of China.
2.2.2. Ocean Color, Numerical Model and Reanalysis Data
The MODIS-Aqua phytoplankton biomass product provided daily and monthly CHL measurements. Monthly Level-3 MODIS-Aqua CHL datasets with a 4 km spatial resolution were obtained from the NASA Ocean Biology Processing Group (https://oceandata.sci.gsfc.nasa.gov). In addition, monthly Level-3 SST data provided by MODIS-Aqua were used and its spatial resolution was the same as that of the CHL data.
The Hybrid Coordinate Ocean Model provided temperature and salinity datasets at an ~8 km (1/12°) spatial resolution with 3-hour intervals. The MLD was calculated using a density threshold of 0.03 kg m-3 from a depth of 10 m, according to de Boyer Montégut et al. (2004). In addition, the European Center for Medium-range Weather Forecasts Reanalysis v5 (ERA5) provided the eastward component of the 10 m wind (U10) and the northward component of the 10 m wind (V10) datasets at ~25 km × 25 km (1/4° × 1/4°) with a monthly temporal resolution. Wind divergence (WD), ∂u/∂x+∂v/∂y , which estimates the atmospheric inflow of aerosols into the ocean, was calculated using U10 and V10 (Freitas et al., 2017). The total precipitation (TP) data, provided at the same resolution in ERA5, were used to consider the effect of precipitation, which enables atmospheric aerosol information to enter the ocean directly.
We used re-mapped monthly mean data rather than daily or weekly due to missing measurement by cloud presence in ocean color satellite measurements (Table 1). Thus, we cannot address the specific lags of 5 to 14 days between aerosol sink to the sea surface and CHL, as reported by Tan et al. (2011); Shi et al. (2012); Tan and Wang (2014) , and Yoon et al. (2019). In addition, we used data for March of each year from 2003 to 2021 because the COVID-19 lockdown period was from the end of February 2020 to the beginning of April 2020.
2.3. Method
According to previous studies, among the ML techniques, the RF model simulates fairly well the complex effect of various factors on CHL (Liu et al., 2013; Liu and Wu, 2017; Park et al., 2019). Thus, we performed a regression analysis to evaluate the CHL response to AOD and other physical forcing variables (SST, MLD, WD, and TP) of the RF model. The RF model, an ensemble learning method, can perform classification and regression analyses to evaluate the CHL response to AOD and other physical forcing variables (SST, MLD, WD, and TP). Decision tree-based algorithms, such as RF, can measure the variable importance (VI) of the features used in model development (Breiman, 2001; Ali et al., 2012). After training, this score was calculated for each feature and the result was normalized such that the total sum of importance was 1. Thus, the VI, which indicates the effect of the predictors used in the RF model on CHL, could be evaluated (Cutler et al., 2007; Park et al., 2020; Pham et al., 2021).
Generally, the RF model sets the number of single decision trees (Ntrees) and the number of features (Mtry) to generate the best performance in each tree node. For regression, Mtry was set to the maximum number of features and for classification, it was set to the square root of the number of features (Geurts et al., 2006). Then, for the feature selected at each node, the node was divided into two child nodes based on the optimal partitioning condition (i.e., a small mean squared error, MSE) and a leaf node was calculated (Park et al., 2020). Finally, the results produced by each tree were ensembled on average to draw a conclusion. The critical parameters considered in the RF model were Ntrees and Mtry. Ntrees was set to 50 through an empirical trial and Mtry was set to 6 according to the convention.
Six predictors (variables) were used as the inputs for the RF model. The predictors used were the AOD, physical forcing variables (SST, MLD, WD, and TP), and climatology of the CHL (CHLc) during spring (March 1 to March 31) from 2003 to 2021. As inputs for the RF model, we used CHLc because it is considered the factor illustrating the environmental variables (Park et al., 2020). It is worth noting that PAR was not used; instead, we used AOD as input because both have directly inverse relations (Li et al., 2020). The target was the MODIS-Aqua CHL (CHLMODIS). The significance test of the predictors was conducted using the Boruta algorithm and all the data were normalized. In addition, for CHLc and CHLMODIS, which have skewed data, a logarithmic transformation was performed to approximate a normal distribution (Gregor et al., 2017; Hahm et al., 2019).
In Section 3.2, the performance of the RF models was evaluated using various statistical metrics, such as the determined coefficient (R2), root mean square error (RMSE), mean absolute error (MAE), and MSE (Popoola et al., 2019; Park et al., 2020). The R2 indicates how well the estimated linear model fits the given data in statistics. RMSE is a measure commonly used when dealing with the difference between the in-situ data (X) and predicted value (Y). MAE means errors between paired observations expressing the same phenomenon. In other words, MAE is the mean absolute difference between the X and Y. MSE shows the mean of the squares of the error. The closer the MSE is to 0, the higher the accuracy is because the guessed value is closer to the original (Chicco et al., 2021). In addition, using the RF model, we determined whether CHL could increase when only AOD was additionally supplied to environmental conditions in March 2020 (Section 3.3). Subsequently, the quantitative contribution of each factor, including AOD to CHL in the RF model, was evaluated using a partial dependence (PD) plot (Section 3.4). A partial dependence (PD) plot depicts the relationship between input variables and predictions (Friedman. 2001). These results show how the predictions partially depend on the values of the input variables of interest. This method can be used to interpret a trained model that can identify linear, monotonic, or more complex relationships between predictors and targets (Molnar, 2020). Furthermore, the PD plot quantitatively describes the response probabilities of the predictors (Friedman, 2001; Greenwell, 2017; Zeng et al., 2017). In other words, the PD plot describes the dependence between the target and predictors of interest, marginalizing over the value of all other predictors. We considered that this PD analysis could represent the quantitative effect on the absolute contribution of each physical variable to CHL, that is, the amount of change in CHL (Park et al., 2020). PD analysis was performed as follows:
where represents the number of features in the RF model (p = 6 in this study). In addition, when we divide x by the set of selected Xs and complement (Xc ) the response variable in the PD plot for Xa is defined by Eq. (1). In other words, Xs is the feature (i.e., variables and inputs) to be calculated through the model and XCis all variables except Xs used feature. pc(Xc) represents the marginal probability density of Xc.Xs is set to one variable, and Xc is the average value that the remaining inputs are affected by the change of Xs . This means that the effects of all the other predictors in the model were average. For more details, refer to Park et al. (2020). Eq. (1) can be evaluated using the training set, as follows:
where Xi,c (i=1, 2, ..., n) represents the value of Xc in the training samples.
The methodology of this study was based on that of Park et al. (2020) (Park et al., 2019). However, unlike the referenced papers on the RF approach, in this study, regression analysis was used among the RF model features to understand the effect of each factor on CHL. In addition, after quantitatively evaluating the impact of each element on spring blooms, the effect was formulated as an equation. Accordingly, this study attempted to extract critical information on how AOD was quantitatively related to spring blooms during the COVID-19 lockdown period.
3 Results and Discussions
3.1. Environmental Conditions of the COVID-19 Lockdown Period
First, we determined how much the aerosol decreased during the COVID-19 lockdown period compared to the other years; therefore, we compared AOD and CHL in March from 2019 to 2021 (Figure 1). During the COVID-19 lockdown period in 2020, local patches were inferred to be small spring blooms (Figure 1E). Especially, it was confirmed that the levels of both data in March 2020, during the COVID-19 lockdown period, decreased significantly compared to those of the other years (Figures 1B, E). In 2019 and 2021, we observed that regions with high AOD distribution showed high CHL levels, confirming that both variables had similar spatial distributions (Figures 1A, C, D, F). From Figure 1, it can be inferred that the abnormally low CHL levels in 2020 was affected by low AOD. We also investigated the changes in other factors to determine whether the effect of reduced aerosol weakened spring blooms during the COVID-19 lockdown period.
Figure 2 shows that AOD and CHL in March 2020 were lower than the average values from March 2003 to 2021, by approximately 50% (from 0.50 to 0.26) for AOD and approximately 15% (from 2.18 mg m-3 to 1.90 mg m-3) for CHL (Figures 2A, F). In contrast, Figure 2B shows that SST increased by approximately 20% from 7.61°C to 9.34°C during the same period. This warmer SST is likely due to the reduced amounts of aerosols, which might have caused the increase in SST due to higher incoming solar radiation (Yue et al., 2011; Kripalani et al., 2022). Accordingly, an increased SST can induce strengthened thermal stratification and a shallower MLD (Tan and Shi, 2012a; Lu et al., 2021). Consequently, the MLD in 2020 became 25% more shallow, from 44.37 m to 33.09 m (Figure 2C). In addition, phytoplankton biomass generally decreases as the MLD becomes more shallow (Lu et al., 2021). This was also seen for the CHL in 2020 and 2021. Additionally, the WD indicated that the airflow from the atmosphere to the ocean was slightly weakened by 13% (from -2.13 × 10-6 sec-1 to -2.39 × 10-6 sec-1), but this value was not significantly different than that of other years. TP also decreased by 45%, from 7.38 × 10-5 mm to 3.92 × 10-5 mm, which is presumed to be an effect of aerosol reduction in humide environmental conditions (Figures 2D, E) (Khain et al., 2008).
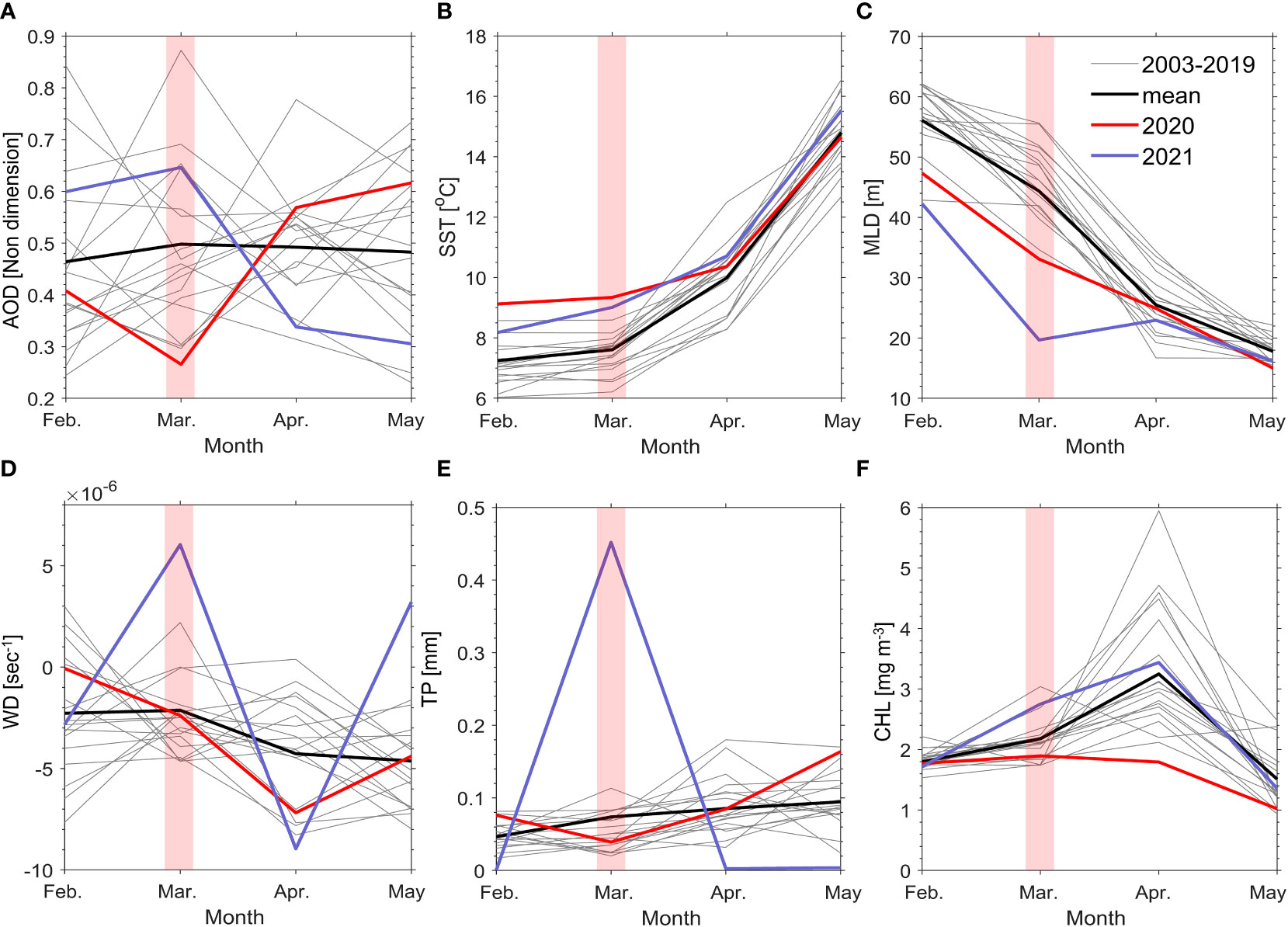
Figure 2 Time series of monthly means of AOD (A), SST (B), MLD (C), WD (D), TP (E), and CHL (F) from February (Feb.) 2003 to May 2021. Gray lines indicate the monthly mean predictor in each year from 2003 to 2019. Red lines show from February 2020 to May 2020 in the COVID-19 lockdown period (red shaded) and blue lines represent from February 2021 to May 2021.
In the case of 2021, many factors showed a significant change compared with the average values from March 2003 to 2021. AOD showed a sharp increase compared with the same period, and SST showed a similar increasing pattern in 2020. Therefore, the thermal stratification was strengthened owing to the increased SST, and the MLD became shallow, as in the COVID-19 lockdown period. In contrast, the WD and TP in 2021 showed much higher levels than the mean values throughout the period, and the increased WD strengthened the flow from the atmosphere into the ocean. The increase in TP may have been because of increased aerosols, and atmospheric aerosols may have possibly directly entered the ocean through precipitation. Consequently, in 2021, with an increase in aerosols, more aerosols could flow into the sea from the atmosphere due to the physical environment’s conditions. We confirmed that 2021 showed a higher CHL than 2020 under different physical environmental conditions during the COVID-19 lockdown period (e.g., increased SST and decreased MLD). Accordingly, the spring blooms in the study area were mainly controlled by AOD changes, as shown in Figures 1, 2. Ultimately, we focused on the qualitative and quantitative effects of aerosol changes that significantly influenced spring blooms.
3.2. RF Model Performance to CHL
To identify the effect of each factor on CHL, we developed a RF model using the above-mentioned data. The performance of the training set with 80% of the total data (n = 94,480) showed R2 = 0.98, RMSE = 0.22 mg m-3, MAE = 0.07 mg m-3, and MSE = 0.05 mg m-3 with high accuracy and low RMSE (Figure 3A; Table 2). It is worth noting that the splitting ratio of 80% to 20% for training and validation dataset shows slightly better results compared to other splitting ratios. According to Despotovic et al. (2016), relative RMSE (RRMSE) evaluates model construction accuracy and is considered good when 10 % ≤ RRMSE < 20 % (i.e., 10.49 % indicates a good level), excellent if RRMSE < 10 %, fair if 20 % ≤ RRMSE < 30 %, and poor if 30 % ≤ RRMSE (Jamieson et al., 1991; Heinemann et al., 2012; Li et al., 2013). Hence, the training set almost coincided with CHLMODIS and the reconstructed CHL derived from the RF model (CHLRF) (Figure 3A). The performance of the validation set with 20 % of the total data (n = 23,620) had accuracy with R2 = 0.85, RMSE = 0.54 mg m-3, MAE = 0.17 mg m-3, and MSE = 0.29 mg m-3, and RRMSE = 25.37 %. Although the validation set had a lower R2 and higher RMSE than those of the training set, thus, the reconstructed result was significant (Figure 3B; Table 2). Additionally, we determined the relative contribution of each predictor to the RF model through the VI (Figure 3C). The relative contributions of CHLc, TP, SST, WD, MLD, and AOD were 46.10 %, 14.42 %, 12.87 %, 9.64 %, 8.71 %, and 8.27 %, respectively. In addition, the standard deviations of CHLc, TP, SST, WD, MLD, and AOD were ±0.29 %, ±0.47 %, ±0.44 %, ±0.44 %, ±0.49 %, and ±0.39 %, respectively. CHLc, the average CHL from March 2003 to 2021, had the most significant effect on the CHL prediction because it reflects the CHL trend. Hence, CHLc was excluded from the physical forcing variables that directly influenced CHL variation.
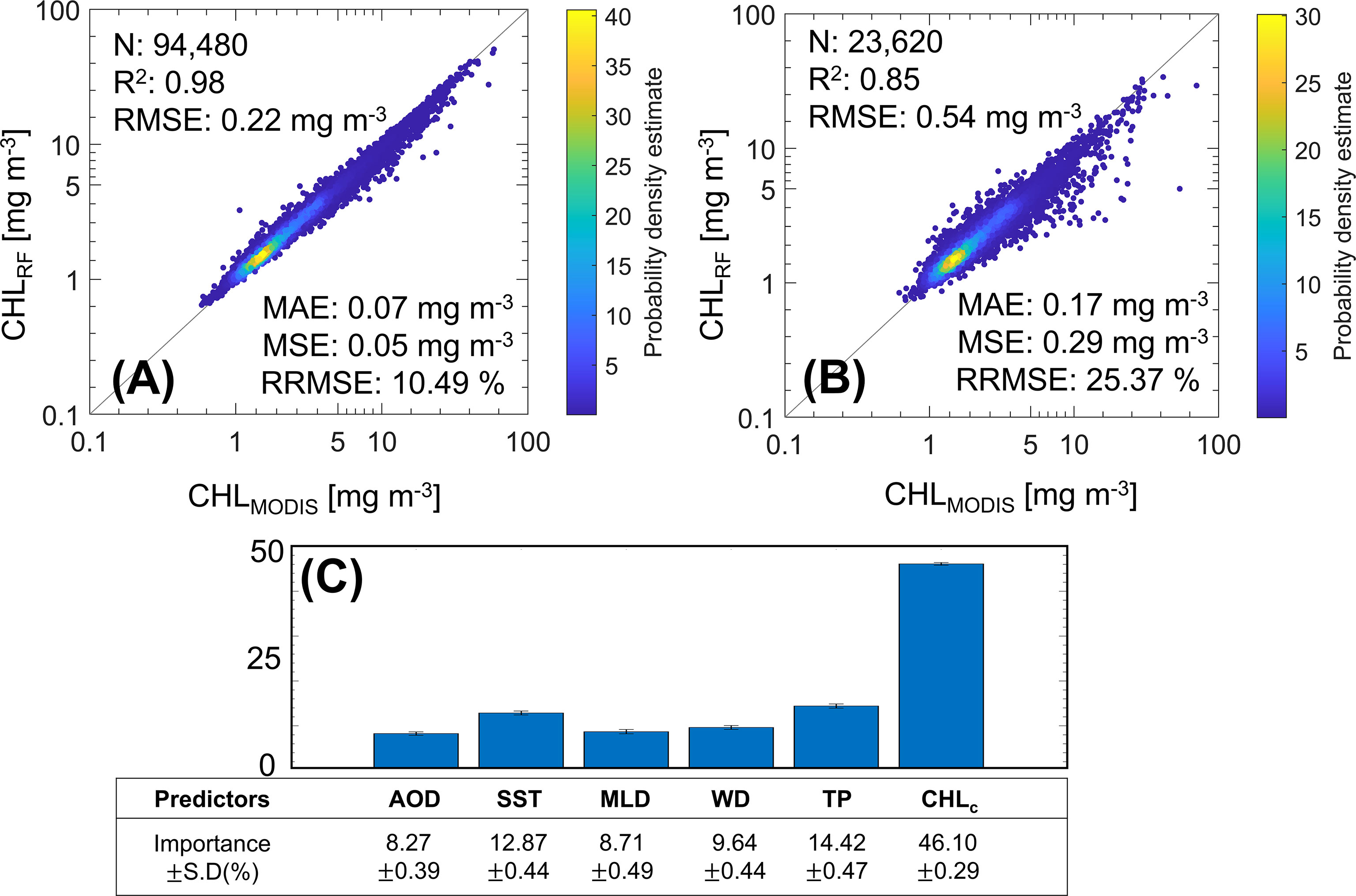
Figure 3 Performance evaluation of RF model for the training set (A) and validation set (B) between MODIS-Aqua CHL (CHLMODIS) and the RF model-derived CHL (CHLRF) The evaluation of variable importance (VI) of each predictor is also included here (C).
To determine whether the RF model could simulate the regional pattern, we compared the spatial distributions of CHLMODIS and CHLRF (Figure 4). The locations and intensities of the spring blooms were also closely simulated each year. The CHLMODIS was 2.09 mg m-3, 1.89 mg m-3, and 2.71 mg m-3, and CHLRF was 2.01 mg m-3, 1.82 mg m-3, and 2.66 mg m-3 in 2019, 2020, and 2021, respectively. If we estimated CHLRF without CHLc, the CHLRF predicted an overestimate (not shown). This result may be because CHLc reflects the CHL information trend. In particular, spring blooms in 2020, which occurred on a small scale and were weak compared to those in other years, were simulated reasonably well. Therefore, it was confirmed that the RF model was well-reconstructed based on the intensity and spatial pattern of CHL (Figures 4B, E).
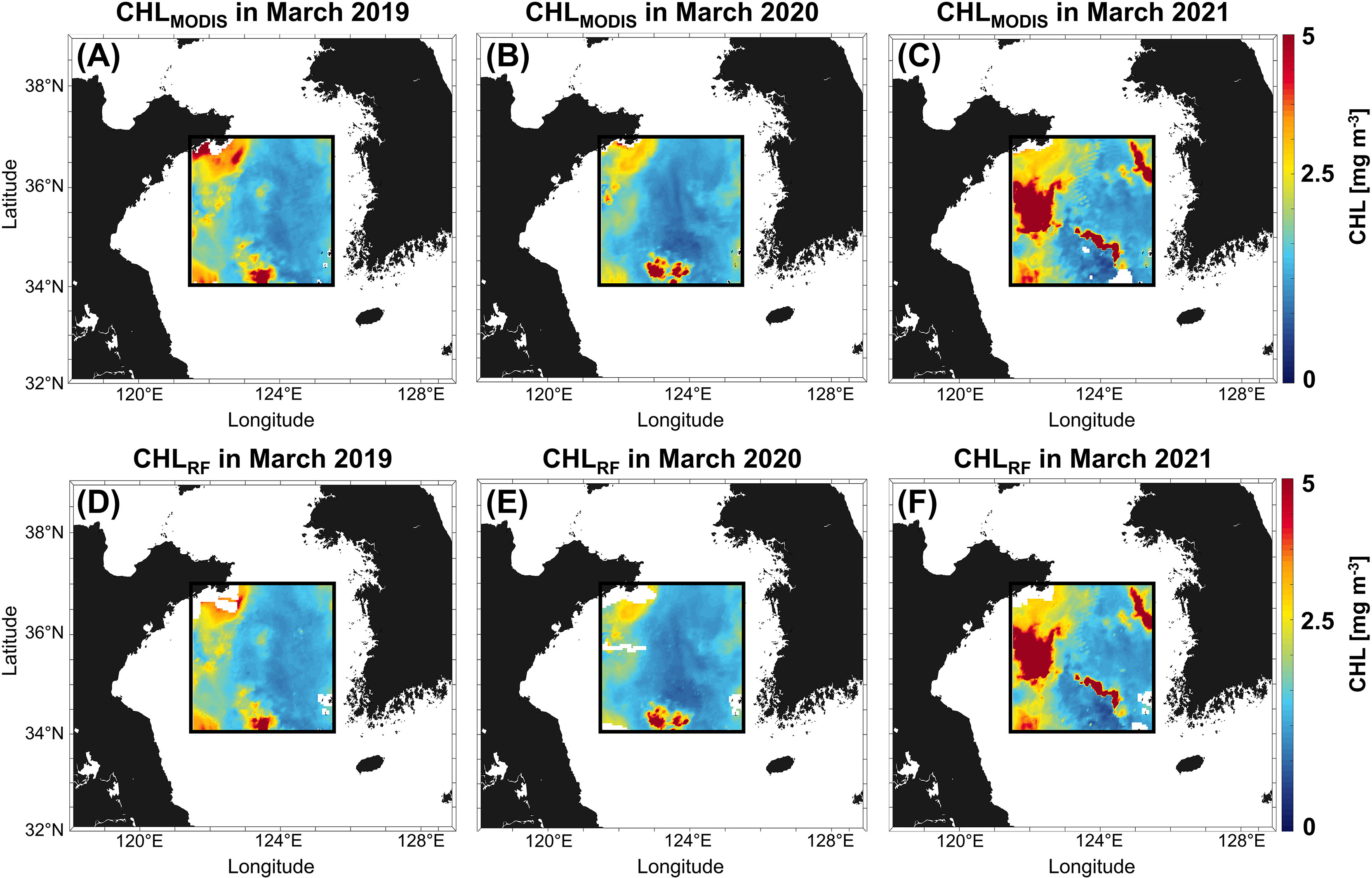
Figure 4 Comparison of spatial patterns between the CHLMODIS(A–C) and CHLRF(D–F) from 2019 to 2021. The black boxes indicate the study area.
The established RF model simulated the spatial pattern and CHL value using six factors. The five factors that directly affect CHL had slight differences, but their relative contributions to the RF model were almost similar. Previous studies have shown that CHL is positively related to SST (Zhao et al., 2019) and negatively related to the MLD and TP (Liu et al., 2013; Lu et al., 2021). The influence of each factor (SST, MLD, WD, and TP), which changed as AOD decreased, was discussed in Section 3.1. Therefore, we concentrated more on the spring blooms based on CHL affected by AOD, which changed the most during the COVID-19 lockdown.
3.3. Relative Contribution of Aerosol to CHL
We focused on the relative effect of AOD on spring blooms, which contributed the least to the RF model but showed the most significant change in 2020 (Section 3.2). This section describes the sensitivity experiments for CHLRF to AOD variations under artificially changing aerosol levels (Figures 4, 5). CHLRF satisfactorily represents the spring blooms that occur each year. However, spring blooms had lessened in the COVID-19 lockdown period of 2020 compared to that in other years (Figure 4E). Therefore, we attempted to confirm the change in spring blooms by artificially increasing and decreasing aerosols during the COVID-19 lockdown period through a sensitivity experiment using the RF model.
Two sensitivity experiment conditions were constructed to investigate changes in CHL variation by adjusting AOD. The two conditions are with AOD ± two sigma (2 σ ± 95%), according to the empirical rule (three sigma rule) of statistics; where 2 σ is derived by assuming the Gaussian fitting for the distribution of AOD climatology. Furthermore, the sensitivity experiments are conducted with AOD + σ and AOD − σ while all other predictors remain unchanged. In other words, when only the AOD either increased or decreased, all other predictors except AOD are constant. The CHL changes to high (CHLHAOD) and low (CHLLAOD) AOD were estimated using the following equations:
The CHLHAOD increased by approximately 95% in AOD and showed an increased CHL in most areas when compared with the existing CHLRF (Figures 5A–C). When aerosols were artificially increased during the COVID-19 lockdown period (in March 2020), phytoplankton biomass increased rapidly in most regions compared with the biomass values from other years (Figure 5B). In particular, although differences occur depending on the region, CHLRF increased by about 60% or more on average in 2019, 2020, and 2021. Based on this result, if the AOD had additional supplies, it could have generated intense spring blooms in March 2020. The CHLLAOD decreased by approximately 95% in AOD and also exhibited a decreased CHL in most areas when compared with CHLRF (Figures 5D–F). Furthermore, Figures 5D, 5F show that the region had significantly lower CHL when the AOD was decreased by approximately 95%. However, the CHLLAOD in 2020 did not represent a significant decrease in most areas when compared to CHLRF because the AOD in March 2020 was already reduced by approximately 50% (0.50 to 0.26) (Figure 5E). This result indicates that the effect of AOD on CHL during the COVID-19 lockdown period was analogous to that of CHLLAOD. This also proves that the experimental results suitably simulated the CHL response to the actual change in the aerosols in the environment.
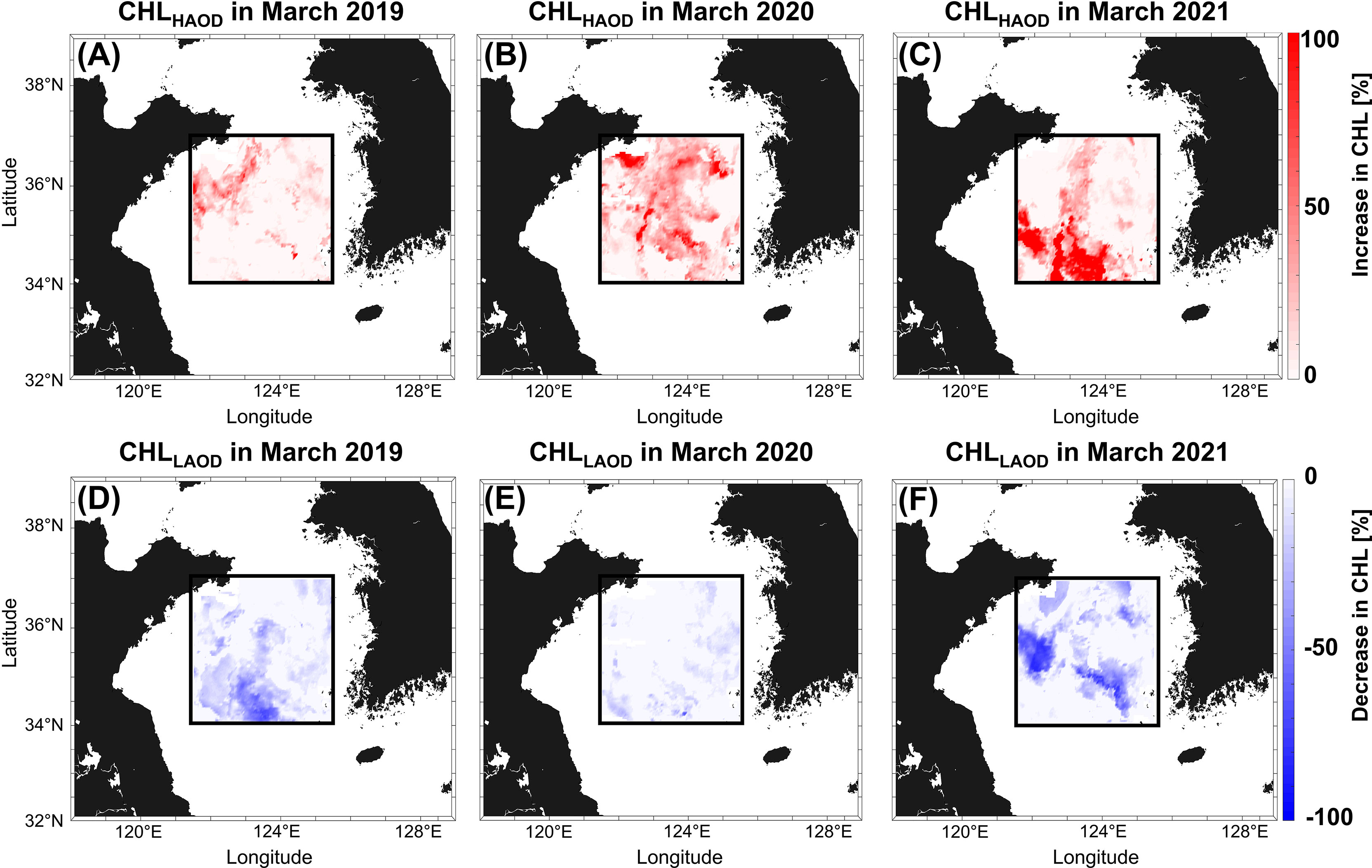
Figure 5 Result of CHLRF according to changes in AOD. The upper panels (A–C) represent the result of CHLRF when AOD is increased by two sigma (2σ, increasing about 95%) and the lower panels (D–F) show when AOD is reduced by two sigma (2σ,increasing about 95%) for a given year.
Therefore, in Section 3.3, we presented the experimental results for predicting CHL changes according to real environmental conditions through CHL reactions, based on artificial AOD fluctuations. These results suggest that AOD (i.e., atmospheric aerosol deposition) significantly affects spring blooms in the CYS.
3.4. PD Analysis for the Six Predictors
In Sections 3.2 and 3.3, we calculated the relative contribution of each factor to spring blooms. Relative contribution refers to the relative value that each factor can influence when various physical factors affect the CHL in combination. However, in Section 3.4, the quantitative contribution was considered as the absolute impact of each variable on CHL individually. This means that the relative contribution and quantitative contribution are different. Therefore, in this section we evaluated the quantitative contribution of each factor’s absolute effect, including AOD, on spring blooms using a PD plot. The contribution of predictors to fluctuating CHL was expressed as the CHL<predictor>. The predictors included AOD (CHLAOD), SST (CHLSST), MLD (CHLMLD), TP (CHLTP), and CHLc (CHLCHLc). To quantitatively reveal the fluctuation of CHL according to the increase or decrease in AOD, we fixed the values of other predictors, except for AOD, during the entire period (i.e., an average of variables for March 2003 to 2021), as shown in Eq. (2). The fluctuating CHL according to the change in AOD was then determined using Eq. (1). For other predictors (e.g., SST, MLD, WD, TP, and CHLc), the fluctuating CHL was simulated using a method similar to that of AOD.
Figure 6 shows the estimated CHLRF through the PD plots. This analysis can explain the fluctuating CHLRF responses to each variable. When AOD increased from 0 to 1, CHLAOD increased from 1.31 mg m-3 to 1.76 mg m-3 (Figure 6A). When AOD was less than approximately 0.3, CHLAOD slowly increased. However, the peak value of CHLAOD appeared at 0.3 and the CHL fluctuation for AOD was approximately 1.40 mg m-3. Hence, a low AOD during the COVID-19 lockdown period induced a slight variation in CHLAOD. Therefore, through the PD plot for AOD, AOD reduction was presumed to cause weaker spring blooms in March 2020 than in other years. As a result, the AOD during the COVID-19 lockdown period (March 2020) recorded an abnormally low CHL (Section 3.1 and Figure 2). CHLSST increased from 1.35 mg m-3 to 2.20 mg m-3 when SST changed from 5°C to 11°C (Figure 6B). From approximately 7°C to 8.5°C , where SST was low, phytoplankton grew slowly and even decreased in abundance (Xuan et al., 2011). However, SST gradually increased and reached 8°C to 12°C (the average SST in the CYS ranges from 8°C to 10°C ), showing a spring bloom peak (Lu et al., 2021). Moreover, the changing CHL pattern influenced by SST matched the generated spring bloom pattern conditions in the CYS (Xu et al., 2013; Zhao et al., 2019).
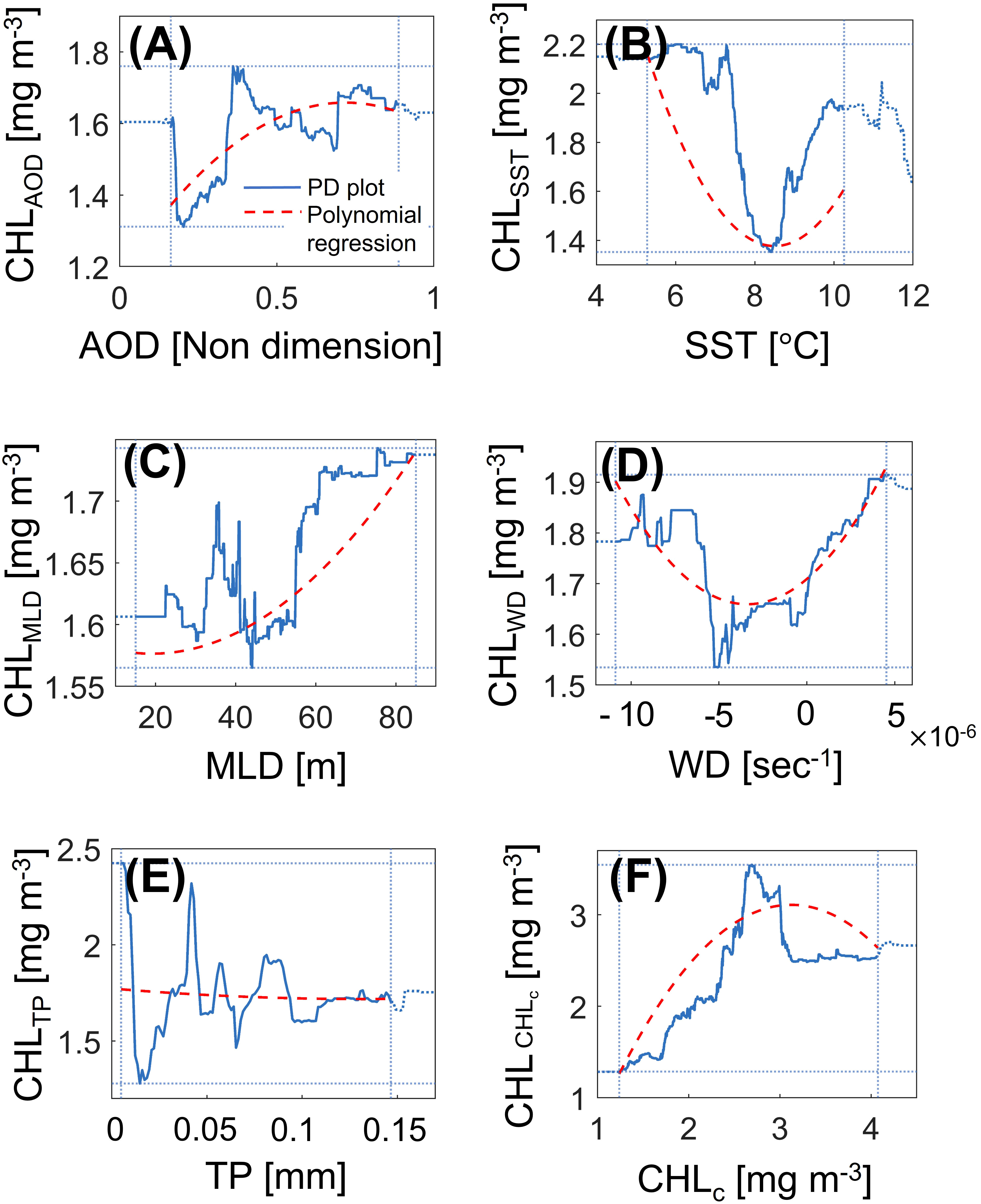
Figure 6 Partial dependence (PD) plots of the CHL for all predictors including AOD, SST, MLD, WD, TP, and CHLC. The blue dotted lines show PD plots and red dashed lines represent the empirical polynomial regression.
When MLD changed from 15 m to 85 m, CHLMLD changed slightly from 1.57 mg m-3 to 1.74 mg m-3 (Figure 6C), thereby reflecting the mean MLD (~ 60 m) in the CYS. Furthermore, the CHLMLD increased with MLD depth. In the CYS, nutrients that affect phytoplankton growth accumulate in the bottom water during autumn and winter. The accumulated nutrients are then moved from the lower layer to the upper layer by vertical mixing in the spring (Kim et al., 2000). Additionally, the YS is affected by anthropogenic aerosols in the atmosphere (Lin et al., 2005; Wei et al., 2015). However, comparing Figures 6A, C, the rate of increase in CHL by MLD was relatively weaker than that in the case of AOD. This result is supported by the data in Figure 2. Both 2020 and 2021 showed a trend of shallower MLD than the average; however, CHL showed the opposite trend (Section 3.2 and Figure 2) and AOD showed the same variability as CHL during the same period. Consequently, we could indirectly estimate that CHL was changed by AOD (Figures 2A, F). These results are consistent with those of previous studies. According to Moon et al. (2021), since 2010, the YS has been significantly affected by anthropogenic nitrogen deposition in the atmosphere. Further, Yoon et al. (2021) noted that most of the new nitrogen input in the YS was caused by atmospheric nitrogen deposition. Therefore, we found that the fluctuation in CHL was more affected by AOD than by MLD.
When WD ranged from − 1.09 × 10-5 sec-1 to 4.53 × 10-6 sec-1, CHLWD fluctuated from 1.53 mg m-3 to 1.68 mg m-3 (Figure 6D). Negative divergence refers to the WD flow from the sea surface to the atmosphere. If the WD flow from the sea surface to the atmosphere decreases, WD flow from the atmosphere to the ocean (positive divergence) increases. As WD increased, CHLWD decreased and then gradually increased (Figure 6D). The WD for March 2020 showed negative divergence. In addition, it revealed partially restricted industrial activities owing to the COVID-19 lockdown policy and showed that anthropogenic aerosol emissions decreased. As a result, the WD flow from the atmosphere to the sea surface weakened and the amount of aerosols deposited on the sea surface was quickly reduced. Conversely, in 2021, the flow from the atmosphere to the sea surface increased, which could have added abundant amounts of aerosols to the ocean. This process may act as a factor limiting the occurrence of spring blooms, thereby supporting the hypothesis that reducing AOD caused a decline in phytoplankton biomass. When TP increased from 0 mm to 0.15 mm, CHLTP decreased from 2.42 mg m-3 to 1.28 mg m-3 (Figure 6E). These results suggest that phytoplankton growth decreased with precipitation. Furthermore, according to previous studies, phytoplankton growth is indirectly estimated to be limited due to the toxic effects of metal components, such as Cu and Pb, in precipitation (Liu et al., 2013). When CHLc increased from 1.29 mg m-3 to 3.55 mg m-3, CHLCHLC increased from 1.24 mg m-3 to 4.07 mg m-3 (Figure 6F). Because CHLc represents the climatology of CHL in the CYS, CHLCHLC increased with increasing CHLc.
3.5. Quantitative Contribution of Aerosol to CHL
Based on the results of the quantitative contributions in Section 3.4, we created an equation that can express the CHL fluctuation for each predictor. Thus, this equation can estimate the amount of CHL fluctuation exhibiting different responses to each factor by summing the amount of change by the deviation of each factor in a year. Section 3.5 reveals the quantitative contribution of each predictor to CHL under natural conditions. The resulting equations are as follows:
where αn is the empirically determined regression coefficient for each predictor (Figure 6; Table 3). Additionally, Δ CHL<predictor> (difference CHL<predictor>) represents the difference between x and x−1 , where x represents each year of the study periods 2021, 2020, 2019, … and 2004. Hence, Δ CHL<predictor> was calculated for each variable variation simulated in the PD plot. Subsequently, the fluctuation CHL was calculated by summing the values of each Δ CHL<predictor>.
Although the PD plot in Figure 6 explains the responsive function of each predictor to CHL changes, we cannot estimate specific contributions of each predictor in different years. Subsequently, the αn (empirical regression coefficients, 2aX + b, X is predictor) was defined by calculating the first derivation based on the empirical polynomial regression. The first derivative can represent the effect of a predictor on CHL (e.g., positive or negative linear trends) (Boelkins et al., 2018). All regression coefficients are listed in Table 3. We calculated the quantitative contribution of each predictor to CHL under natural conditions only in March 2020 (during the COVID-19 lockdown period). The αn for each selected predictor was applied to Eq. (5). According to the variation in each predictor, the CHL variability was then calculated (Table 3). The difference in CHL with the variational predictor (Δ CHL<predictor>) was calculated as the difference between the COVID-19 lockdown period and the average from March 2003 to 2021 (climatology). Δ CHLAOD is the difference in CHLAOD, which indicates the change between the COVID-19 lockdown period and climatology after fixing the variable conditions, except AOD, as in Section 3.4. The other variables were calculated using the same process. Using the αn based on Eq. (5), Δ CHL<predictor> was quantitatively calculated for each predictor. Figure 7 shows the effects of each variable on Δ CHL<predictor> when it decreased by at least 90% and increased by 90% at most, compared to the climatology. The variation range for the predictor is the resulting value for all conditions in which each factor may change, including the COVID-19 lockdown period. Furthermore, Figure 7 shows the individual effect of the absolute contribution of each parameter to the CHL. As a result, Δ CHLAOD decreased by 0.20 mg m-3 due to an AOD less than that of the climatology. However, Δ CHLSST increased by 0.22 mg m-3 because SST was warmer than that measured in the same period in other years (Figure 7). Moreover, MLD became more shallow than that of the climatology, reducing Δ CHLMLD by 0.01 mg m-3. Additionally, the negative WD yielded a stable Δ CHLWD, but less TP increased Δ CHLTP by 0.02 mg m-3. Similary, we estimated Δ CHLAOD in 2019 and 2021 using the linear model of the RF in Figure 7, showing as much as -0.05 mg m-3 and + 0.13 mg m-3, respectively. However, Δ CHLSST increased by 0.07 mg m-3 and 0.18 mg m-3 in 2019 and 2021, respectively. Thus, the linear model allows us to estimate the CHL chages due to the various predictors
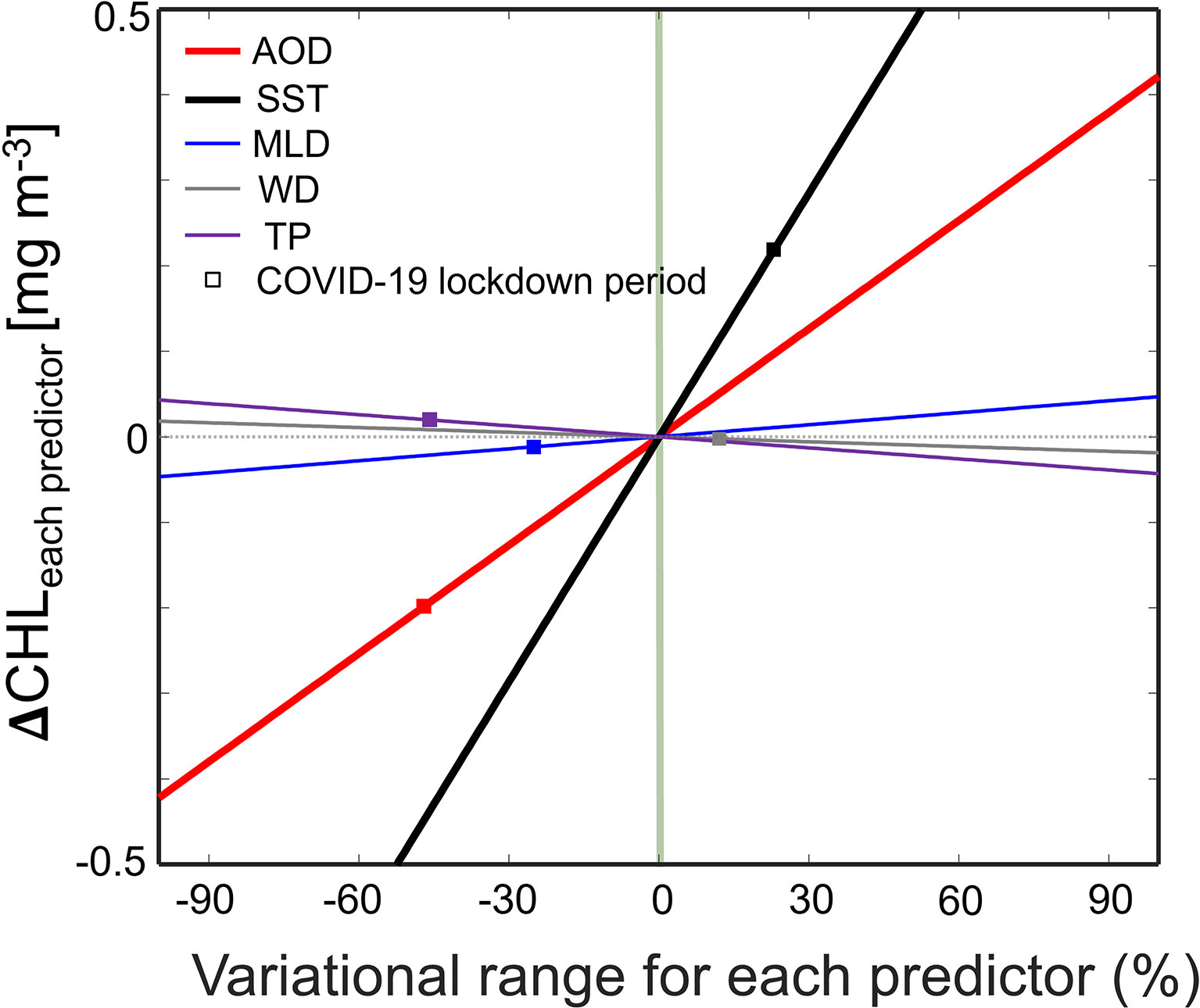
Figure 7 Empirical variation coefficient of each predictor with the climatology of each variable from March 2003 to 2021. Red and black lines indicate the difference for CHL along variational AOD (ΔCHLAOD) and SST (ΔCHLSST), respectively. Blue, gray, and purple lines represent the ΔCHLMLD, ΔCHLWD, and ΔCHLTP, respectively. Square boxes defined CHL according to the conditions of each predictor in the COVID-19 lockdown period. The green line and gray dot show CHL’s zero and climatology condition for ΔCHLeach predictor, respectively.
From analyzing the individual effects of each factor, it was determined that the decreased AOD and shallower MLD, compared to those of climatology, affected the CHL reduction. Among them, the leading cause of the decreasing spring blooms during the COVID-19 lockdown period was the sharply reduced AOD from the shutdown policy in China. In addition, it was found that the increased SST and decreased TP effect on the increasing CHL. As a consequence, AOD and MLD were the causes that led to the decrease in CHL and, among them, the effect of AOD reduction was approximately 10 times greater than that of the shallower MLD. In addition, the quantitative (absolute) contribution of the individual factors to CHL, shown in Figure 7, was largest in SST, followed by those in AOD, MLD, TP, and WD. For comparison, they are listed in absolute value order without mathematical symbols. This differs from the relative contributions presented in Section 3.2, suggesting that the strength of spring blooms in the YS can be controlled mainly by SST and AOD. In addition to the relationship already revealed in Figure 7, the final results of this study, which is the quantitative relationship between CHL and each factor, which was limited in previous studies, are presented together.
Accordingly, we determined a causal relationship between AOD and CHL through time series analysis for each factor to investigate the cause of spring blooms that were weakened during the COVID-19 lockdown period. In addition, intense spring blooms were induced when additional AOD was supplied in March 2020 using the RF model. Finally, as a result of estimating the quantitative contribution of each factor to CHL, it was observed that the decrease in aerosols led to a decline in CHL. Overall, the weakened spring blooms during the COVID-19 lockdown period could have resulted from complex factors instigated by the temporary closure of industrial activities.
4 Conclusions
The quantitative evaluation using the RF-based model and PD results was applied to understand the decrease of CHL in the CYS, which was mainly due to the reduction in aerosol during the COVID-19 lockdown period. Consequently, the following specific results were obtained:
1. We identified the aerosol effect on CHL by checking the spatial distribution of CHL, according to AOD ± 2σ , through the established RF model. In particular, as AOD decreased by about 95%, CHL also reduced by about 50% or more through sensitivity experiments. This result proves that decreased anthropogenic aerosol emissions due to the COVID-19 lockdown policy decreased spring blooms in the CYS. Therefore, aerosol must be considered essential to increasing spring blooms in the CYS (Section 3.3).
2. We performed the quantitative analysis in CHL according to the adjustment for each predictor using a PD plot and empirical polynomial regression analysis. These methods evaluated the quantitative effect of predictors affecting CHL reduction during the COVID-19 lockdown period in 2020. The CHL was affected by AOD (− 0.20 mg m-3), MLD (− 0.01 mg m-3), WD (± 0.00 mg m-3), TP (+ 0.02 mg m-3), and SST (+ 0.22 mg m-3); among them, AOD had the predominant reduction effect (Section 3.5).
3. There, we elicited an abnormally low CHL during the COVID-19 lockdown period, affected by AOD, based on sensitivity tests for the RF model and various analysis methods. These results suggest that the effect of each factor, especially AOD, that affected the COVID-19 lockdown period could be quantitatively evaluated.
The limitations of this study are as follows: (1) Regarding aerosol sinking into the sea, Liu et al. (2013) showed the extent to which aerosols (including Asian dust) could indirectly induce CHL blooms in the YS. Furthermore, although the Cloud-Aerosol Lidar and Infrared Pathfinder Satellite Observation can provide aerosol subtype data, it cannot provide the amount of aerosol entering the ocean (e.g., Tan and Wang, 2014; Yoon et al., 2019; Yoon et al., 2021). Thus, we could not quantitatively estimate the amount of aerosol (anthropogenic aerosol) absorbed into the sea surface in the current analysis. (2) Regarding nutrient loads, AOD contains anthropogenic nitrogen (NOx and NHy) and minerals such as iron (Fe). Aerosols containing Fe (i.e., Asian dust) are also a significant factor that can cause spring blooms in the CYS (Shi et al., 2012; Yoo et al., 2018; Yoon et al., 2019). Thus, spring blooms can be accelerated by the nitrogen (excluding anthropogenic nitrogen) supplied by nearby rivers. Since there were no nutrient observations during the COVID-19 lockdown period in YS, the central area of the YS was selected as the study area to avoid the effects of nitrogen from the surrounding river. Additionally, we confirmed that Asian dust did not occur during that period, nor was any additionally supplied Fe (Section 2.2.1). Thus, further research may be needed to measure directly how much aerosol sinks into the sea and nutrient load through ocean currents from coastal regions.
Data Availability Statement
The original contributions presented in the study are included in the article/supplementary material. Further inquiries can be directed to the corresponding author.
Author Contributions
Conceptualization: J-YB, JP, and Y-HJ. Data curation: J-YB, J-YL, and S-JL. Methodology: J-YB, JP, and Y-HJ. Formal analysis: J-YB, JP, D-WK, J-SL, and Y-HJ. Writing-original draft: J-YB. Writing—review and editing: J-YB, JP, and Y-HJ. All authors have read and agreed to the published version of the manuscript.
Funding
This study was supported by a grant from the National Research Foundation of Korea (NRF) funded by the Korean government (MSIP) (NRF-2018R1A2B2006555). In addition, this research was part of the project “Integrated management of marine environment and ecosystems around Saemangeum,” funded by the Ministry of Oceans and Fisheries, Korea (grant number 20140257).
Conflict of Interest
The authors declare that the research was conducted in the absence of any commercial or financial relationships that could be construed as a potential conflict of interest.
Publisher’s Note
All claims expressed in this article are solely those of the authors and do not necessarily represent those of their affiliated organizations, or those of the publisher, the editors and the reviewers. Any product that may be evaluated in this article, or claim that may be made by its manufacturer, is not guaranteed or endorsed by the publisher.
References
Ali J., Khan R., Ahmad N., Maqsood I. (2012). Random Forests and Decision Trees. Int. J. Comput. Sci. Issues (IJCSI) 9 (5), 272.
Arteaga L., Pahlow M., Oschlies A. (2016). Modeled Chl: C Ratio and Derived Estimates of Phytoplankton Carbon Biomass and its Contribution to Total Particulate Organic Carbon in the Global Surface Ocean. Global Biogeochemical Cycles 30 (12), 1791–1810. doi: 10.1002/2016GB005458
Bauwens M., Compernolle S., Stavrakou T., Müller J. F., Van Gent J., Eskes H., et al. (2020). Impact of Coronavirus Outbreak on NO2 Pollution Assessed Using TROPOMI and OMI Observations. Geophysical Res. Lett. 47 (11), e2020GL087978. doi: 10.1029/2020GL087978
Boelkins M., Austin D., Schlicker S. (2018). Active calculus 2.1. (Allendale, MI. United State of America: Grand Valley State University Libraries). Availabale at: https://activecalculus.org/single/frontmatter.html
Cheng Y., Bhoot V. N., Kumbier K., Sison-Mangus M. P., Brown J. B., Kudela R., Newcomer M. E. (2021). A Novel Random Forest Approach to Revealing Interactions and Controls on Chlorophyll Concentration and Bacterial Communities During Coastal Phytoplankton Blooms. Sci. Rep. 11 (1), 1–11. doi: 10.1038/s41598-021-98110-9
Cheng Y., Lohmann U., Zhang J., Luo Y., Liu Z., Lesins G. (2005). Contribution of Changes in Sea Surface Temperature and Aerosol Loading to the Decreasing Precipitation Trend in Southern China. J. Climate 18 (9), 1381–1390. doi: 10.1175/JCLI3341.1
Chicco D., Warrens M. J., Jurman G. (2021). The Coefficient of Determination R-Squared is More Informative Than SMAPE, MAE, MAPE, MSE and RMSE in Regression Analysis Evaluation. PeerJ Comput. Sci. 7, e623. doi: 10.7717/peerj-cs.623
Cutler D. R., Edwards T. C. Jr., Beard K. H., Cutler A., Hess K. T., Gibson J., et al. (2007). Random Forests for Classification in Ecology. Ecology 88 (11), 2783–2792. doi: 10.1890/07-0539.1
de Boyer Montégut C., Madec G., Fischer A. S., Lazar A., Iudicone D. (2004). Mixed Layer Depth Over the Global Ocean: An Examination of Profile Data and a Profile-Based Climatology. J. Geophysical Res. Oceans 109 (C12). doi: 10.1029/2004JC002378
Despotovic M., Nedic V., Despotovic D., Cvetanovic S. (2016). Evaluation of Empirical Models for Predicting Monthly Mean Horizontal Diffuse Solar Radiation. Renewable Sustain. Energy Rev. 56, 246–260. doi: 10.1016/j.rser.2015.11.058
Freitas A. C., Frederiksen J. S., O’Kane T. J., Ambrizzi T. (2017). Simulated Austral Winter Response of the Hadley Circulation and Stationary Rossby Wave Propagation to a Warming Climate. Climate Dynamics 49 (1), 521–545. doi: 10.1007/s00382-016-3356-4
Friedman J. H. (2001). Greedy Function Approximation: A Gradient Boosting Machine. Annals of Statistics 29 (5), 1189–1232.
Fu M., Sun P., Wang Z., Wei Q., Qu P., Zhang X., et al. (2018). Structure, Characteristics and Possible Formation Mechanisms of the Subsurface Chlorophyll Maximum in the Yellow Sea Cold Water Mass. Continental Shelf Res. 165, 93–105. doi: 10.1016/j.csr.2018.07.007
Geurts P., Ernst D., Wehenkel L. (2006). Extremely Randomized Trees. Mach. Learn. 63 (1), 3–42. doi: 10.1007/s10994-006-6226-1
Greenwell B. M. (2017). Pdp: An R Package for Constructing Partial Dependence Plots. R J. 9 (1), 421. doi: 10.32614/RJ-2017-016
Gregor L., Kok S., Monteiro P. (2017). Empirical Methods for the Estimation of Southern Ocean CO 2: Support Vector and Random Forest Regression. Biogeosciences 14 (23), 5551–5569. doi: 10.5194/bg-14-5551-2017
Hahm D., Park S., Choi S.-W., Kang D.-J., Rho T., Lee T. (2019). Estimation of Surface F CO2 in the Southwest East Sea Using Machine Learning Techniques (in Korea). J. Korean Soc. Oceanogr. 24 (3), 375–388. doi: 10.7850/jkso.2019.24.3.375
Heinemann A. B., Van Oort P. A., Fernandes D. S., Maia A. D. H. N. (2012). Sensitivity of APSIM/ORYZA Model Due to Estimation Errors in Solar Radiation. Bragantia 71 (4), 572–582. doi: 10.1590/S0006-87052012000400016
He G., Pan Y., Tanaka T. (2020). The Short-Term Impacts of COVID-19 Lockdown on Urban Air Pollution in China. Nat. Sustainability 3 (12), 1005–1011. doi: 10.1038/s41893-020-0581-y
Hsu N. C., Jeong M. J., Bettenhausen C., Sayer A. M., Hansell R., Seftor C. S., et al. (2013). Enhanced Deep Blue Aerosol Retrieval Algorithm: The Second Generation. J. Geophysical Research: Atmospheres 118 (16), 9296–9315. doi: 10.1002/jgrd.50712
Hsu N. C., Tsay S. C., King M. D., Herman J. R. (2004). Aerosol Properties Over Bright-Reflecting Source Regions. IEEE Trans. Geosci. Remote Sens. 42 (3), 557–569. doi: 10.1109/TGRS.2004.824067
Hsu N. C., Tsay S. C., King M. D., Herman J. R. (2006). Deep Blue Retrievals of Asian Aerosol Properties During ACE-Asia. IEEE Trans. Geosci. Remote Sens. 44 (11), 3180–3195. doi: 10.1109/TGRS.2006.879540
Jamieson P. D., Porter J. R., Wilson D. R. (1991). A Test of the Computer Simulation Model ARCWHEAT1 on Wheat Crops Grown in New Zealand. Field Crops Res. 27 (4), 337–350. doi: 10.1016/0378-4290(91)90040-3
Jin J., Liu S. M., Ren J. L., Liu C. G., Zhang J., Zhang G. L. (2013). Nutrient Dynamics and Coupling With Phytoplankton Species Composition During the Spring Blooms in the Yellow Sea. Deep Sea Res. Part II: Topical Stud. Oceanogr. 97, 16–32. doi: 10.1016/j.dsr2.2013.05.002
Kang J. J., Joo H., Lee J. H., Lee J. H., Lee H. W., Lee D., et al. (2017). Comparison of Biochemical Compositions of Phytoplankton During Spring and Fall Seasons in the Northern East/Japan Sea. Deep Sea Res. Part II: Topical Stud. Oceanogr. 143, 73–81. doi: 10.1016/j.dsr2.2017.06.006
Kaufman Y. J., Tanré D., Remer L. A., Vermote E. F., Chu A., Holben B. N. (1997). Operational Remote Sensing of Tropospheric Aerosol Over Land From EOS Moderate Resolution Imaging Spectroradiometer. J. Geophysical Res. Atmospheres 102 (D14), 17051–17067. doi: 10.1029/96JD03988
Khain A. P., BenMoshe N., Pokrovsky A. (2008). Factors Determining the Impact of Aerosols on Surface Precipitation From Clouds: An Attempt at Classification. J. Atmospheric Sci. 65 (6), 1721–1748. doi: 10.1175/2007JAS2515.1
Kim K. H., Lee J. H., Shin K. S., Pae S. J., Yoo S. J., Chung C. S., et al. (2000). Springtime Distribution of Inorganic Nutrients in the Yellow Sea: Its Relation to Water Mass. Sea: J. OF THE KOREAN Soc. OF OCEANOGR. 5 (3), 224–232.
Kim Y. J., Woo J. H., Ma Y. I., Kim S., Nam J. S., Sung H., et al. (2009). Chemical Characteristics of Long-Range Transport Aerosol at Background Sites in Korea. Atmospheric Environ. 43 (34), 5556–5566. doi: 10.1016/j.atmosenv.2009.03.062
Kotsiantis S. B., Zaharakis I. D., Pintelas P. E. (2006). Machine Learning: A Review of Classification and Combining Techniques. Artif. Intell. Rev. 26 (3), 159–190. doi: 10.1007/s10462-007-9052-3
Kripalani R., Ha K. J., Ho C. H., Oh J. H., Preethi B., Mujumdar M., et al. (2022). Erratic Asian Summer Monsoon 2020: COVID-19 Lockdown Initiatives Possible Cause for These Episodes? Climate Dynamics, 1–14. doi: 10.1007/s00382-021-06042-x
Lee H. J., Jo H. Y., Kim S. W., Park M. S., Kim C. H. (2019). Impacts of Atmospheric Vertical Structures on Transboundary Aerosol Transport From China to South Korea. Sci. Rep. 9 (1), 1–9. doi: 10.1038/s41598-019-49691-z
Levy R. C., Mattoo S., Munchak L. A., Remer L. A., Sayer A. M., Patadia F., et al. (2013). The Collection 6 MODIS Aerosol Products Over Land and Ocean. Atmospheric Measurement Techniques 6 (11), 2989–3034. doi: 10.5194/amt-6-2989-2013
Lien W. H., Lin T. H., Liu G. R., Tseng K. H., Tsai F. A., Liu C. Y. (2017). Associations Between Aerosol Types and Chlorophyll-A Concentration Over Coastal Area in East Asia From Satellite Observations. Int. J. @ Mar. Environ. Sci. 1 (1), 15–24. doi: 10.13140/RG.2.2.20575.56484
Li X., Liang H., Cheng W. (2020). Spatio-Temporal Variation in AOD and Correlation Analysis With PAR and NPP in China From 2001 to 2017. Remote Sens. 12 (6), 976. doi: 10.3390/rs12060976
Li C., Mao J., Lau K. H. A., Chen J. C., Yuan Z., Liu X., et al, 2003 Characteristics of Distribution and Seasonal Variation of Aerosol Optical Depth in Eastern China with MODIS Products. Chinese Science Bulletin48222488–2495. doi: 10.1360/03wd0224
Lim Y. K., Kim J., Lee H. C., Lee S. S., Cha J. W., Ryoo S. B. (2019). Aerosol Physical Characteristics Over the Yellow Sea During the KORUS-AQ Field Campaign: Observations and Air Quality Model Simulations. Asia-Pacific J. Atmospheric Sci. 55 (4), 629–640. doi: 10.1007/s13143-018-00100-x
Lin C. L., Ning X. R., Su J. L., Lin Y., Xu B. (2005). Environmental Changes and the Responses of the Ecosystems of the Yellow Sea During 1976–2000. J. Mar. Syst. 55 (3-4), 223–234. doi: 10.1016/j.jmarsys.2004.08.001
Li M. F., Tang X. P., Wu W., Liu H. B. (2013). General Models for Estimating Daily Global Solar Radiation for Different Solar Radiation Zones in Mainland China. Energy conversion Manage. 70, 139–148. doi: 10.1016/j.enconman.2013.03.004
Liu M., Liu X., Jiang J., Xia X., – 2013 Artificial Neural Network and Random Forest Approaches for Modeling of Sea Surface Salinity. International J. Remote Sensing App. 34229235. doi: 10.14355/ijrsa.2013.0304.08
Liu Y., Wu H. (2017). Water Bloom Warning Model Based on Random Forest. In 2017 International Conference on Intelligent Informatics and Biomedical Sciences (ICIIBMS), IEEE, 45–48. doi: 10.1109/ICIIBMS.2017.8279712
Liu Y., Zhang T. R., Shi J. H., Gao H. W., Yao X. H. (2013). Responses of Chlorophyll a to Added Nutrients, Asian Dust, and Rainwater in an Oligotrophic Zone of the Yellow Sea: Implications for Promotion and Inhibition Effects in an Incubation Experiment. J. Geophysical Research: Biogeosciences 118 (4), 1763–1772. doi: 10.1002/2013JG002329
Lu X., Liu C., Niu Y., Yu S. (2021). Long-Term and Regional Variability of Phytoplankton Biomass and its Physical Oceanographic Parameters in the Yellow Sea, China. Estuarine Coast. Shelf Sci. 260, 107497. doi: 10.1016/j.ecss.2021.107497
Mahowald N. M., Baker A. R., Bergametti G., Brooks N., Duce R. A., Jickells T. D., et al. (2005). Atmospheric Global Dust Cycle and Iron Inputs to the Ocean. Global biogeochemical cycles 19 (4). doi: 10.1029/2004GB002402
Mahowald N. M., Hamilton D. S., Mackey K. R., Moore J. K., Baker A. R., Scanza R. A., et al. (2018). Aerosol Trace Metal Leaching and Impacts on Marine Microorganisms. Nat. Commun. 9 (1), 1–15. doi: 10.1038/s41467-018-04970-7
Molnar C. (2020). Interpretable Machine Learning: A Guide for Making Black Box Models Explainable Leanpub:Victoria. BC. Canada. Lulu. https://christophm.github.io/interpretable-ml-book/.
Moon J. Y., Lee K., Lim W. A., Lee E., Dai M., Chae J, Choi Y. H.(2021). Anthropogenic Nitrogen is Changing the East China and Yellow Seas From Being N Deficient to Being P Deficient. Limnology Oceanography 66 (3), 914–924. doi: 10.1002/lno.11651
Oh H. R., Ho C. H., Kim J., Chen D., Lee S., Choi Y. S., et al. (2015). Long-Range Transport of Air Pollutants Originating in China: A Possible Major Cause of Multi-Day High-PM10 Episodes During Cold Season in Seoul, Korea. Atmospheric Environ. 109, 23–30. doi: 10.1016/j.atmosenv.2015.03.005
Park T., Ahn J., Choi J., Lim Y., Park J., Kim J., et al. (2017). Physico-Chemical Characteristics of Submicron Aerosol at West Inflow Regions in the Korean Peninsula III. Physical-Chemical Behavior and Long-Range Transport of PM 1. J. Korean Soc. Atmospheric Environ. 33 (2), 124–138. doi: 10.5572/KOSAE.2017.33.2.124
Park J., Kim H. C., Bae D., Jo Y. H. (2020). Data Reconstruction for Remotely Sensed Chlorophyll-A Concentration in the Ross Sea Using Ensemble-Based Machine Learning. Remote Sens. 12 (11), 1898. doi: 10.3390/rs12111898
Park J., Kim J. H., Kim H. C., Kim B. K., Bae D., Jo Y. H., et al. (2019). Reconstruction of Ocean Color Data Using Machine Learning Techniques in Polar Regions: Focusing on Off Cape Hallett, Ross Sea. Remote Sens. 11 (11), 1366. doi: 10.3390/rs11111366
Paytan A., Mackey K. R., Chen Y., Lima I. D., Doney S. C., Mahowald N., et al. (2009). Toxicity of Atmospheric Aerosols on Marine Phytoplankton. Proc. Natl. Acad. Sci. 106 (12), 4601–4605. doi: 10.1073/pnas.0811486106
Pham L. T., Luo L., Finley A. (2021). Evaluation of Random Forests for Short-Term Daily Streamflow Forecasting in Rainfall-and Snowmelt-Driven Watersheds. Hydrology Earth System Sci. 25 (6), 2997–3015. doi: 10.5194/hess-25-2997-2021
Popoola S. I., Jefia A., Atayero A. A., Kingsley O., Faruk N., Oseni O. F., et al. (2019). Determination of Neural Network Parameters for Path Loss Prediction in Very High Frequency Wireless Channel. IEEE Access 7, 150462–150483. doi: 10.1109/ACCESS.2019.2947009
Qi J. H., Shi J. H., Gao H. W., Sun Z. (2013). Atmospheric Dry and Wet Deposition of Nitrogen Species and its Implication for Primary Productivity in Coastal Region of the Yellow Sea, China. Atmospheric Environ. 81, 600–608. doi: 10.1016/j.atmosenv.2013.08.022
Shakoor A., Chen X., Farooq T. H., Shahzad U., Ashraf F., Rehman A., et al. (2020). Fluctuations in Environmental Pollutants and Air Quality During the Lockdown in the USA and China: Two Sides of COVID-19 Pandemic. Air Qual. Atmosphere Health 13 (11), 1335–1342. doi: 10.1007/s11869-020-00888-6
Shi J. H., Gao H. W., Zhang J., Tan S. C., Ren J. L., Liu C. G., et al. (2012). Examination of Causative Link Between a Spring Bloom and Dry/Wet Deposition of Asian Dust in the Yellow Sea, China. J. Geophysical Res. Atmospheres 117 (D17). doi: 10.1029/2012JD017983
Shi J., Liu Y., Mao X., Guo X., Wei H., Gao H. (2017). Interannual Variation of Spring Phytoplankton Bloom and Response to Turbulent Energy Generated by Atmospheric Forcing in the Central Southern Yellow Sea of China: Satellite Observations and Numerical Model Study. Continental Shelf Res. 143, 257–270. doi: 10.1016/j.csr.2016.06.008
Tanré D., Kaufman Y. J., Herman M., Mattoo S. (1997). Remote Sensing of Aerosol Properties Over Oceans Using the MODIS/EOS Spectral Radiances. J. Geophysical Res. Atmospheres 102 (D14), 16971–16988. doi: 10.1029/96JD03437
Tan S. C., Shi G. Y. (2012a). Correlation of Dust Storms in China With Chlorophyll a Concentration in the Yellow Sea Between 1997–2007. Atmospheric Oceanic Sci. Lett. 5 (2), 140–144. doi: 10.1080/16742834.2012.11446980
Tan S. C., Shi G. Y. (2012b). The Relationship Between Satellite-Derived Primary Production and Vertical Mixing and Atmospheric Inputs in the Yellow Sea Cold Water Mass. Continental Shelf Res. 48, 138–145. doi: 10.1016/j.csr.2012.07.015
Tan S. C., Shi G. Y., Shi J. H., Gao H. W., Yao X. (2011). Correlation of Asian Dust With Chlorophyll and Primary Productivity in the Coastal Seas of China During the Period From 1998 to 2008. J. Geophysical Res. Biogeosciences 116 (G2). doi: 10.1029/2010JG001456
Tan S. C., Wang H. (2014). The Transport and Deposition of Dust and its Impact on Phytoplankton Growth in the Yellow Sea. Atmospheric Environ. 99, 491–499. doi: 10.1016/j.atmosenv.2014.10.016
Tao W. K., Chen J. P., Li Z., Wang C., Zhang C. (2012). Impact of Aerosols on Convective Clouds and Precipitation. Rev. Geophysics 50 (2). doi: 10.1029/2011RG000369
Timmermann A., Lee S. S., Chu J. E., Chung E. S., Lee J. Y. (2020). COVID-19-Related Drop in Anthropogenic Aerosol Emissions in China and Corresponding Cloud and Climate Effects. Eartharxiv. doi: 10.31223/osf.io/z5dm8
Wang Q., Su M. (2020). A Preliminary Assessment of the Impact of COVID-19 on Environment–A Case Study of China. Science of the Total Environment728, 138915. doi: 10.1016/j.scitotenv.2020.138915
Wang B. D., Wang X. L., Zhan R. (2003). Nutrient Conditions in the Yellow Sea and the East China Sea. Estuarine Coast. Shelf Sci. 58 (1), 127–136. doi: 10.1016/S0272-7714(03)00067-2
Wei Q., Yao Q., Wang B., Wang H., Yu Z. (2015). Long-Term Variation of Nutrients in the Southern Yellow Sea. Continental Shelf Res. 111, 184–196. doi: 10.1016/j.csr.2015.08.003
Xuan J. L., Zhou F., Huang D. J., Wei H., Liu C. G., Xing C. X. (2011). Physical Processes and Their Role on the Spatial and Temporal Variability of the Spring Phytoplankton Bloom in the Central Yellow Sea. Acta Ecologica Sin. 31 (1), 61–70. doi: 10.1016/j.chnaes.2010.11.011
Xu Y., Ishizaka J., Yamaguchi H., Siswanto E., Wang S. (2013). Relationships of Interannual Variability in SST and Phytoplankton Blooms With Giant Jellyfish (Nemopilema Nomurai) Outbreaks in the Yellow Sea and East China Sea. J. Oceanogr. 69 (5), 511–526. doi: 10.1007/s10872-013-0189-1
Yoon J. E., Lim J. H., Shim J. M., Kwon J. I., Kim I. N. (2019). Spring 2018 Asian Dust Events: Sources, Transportation, and Potential Biogeochemical Implications. Atmosphere 10 (5), 276. doi: 10.3390/atmos10050276
Yoon J. E., Son S., Kim I. N.2021Capture of Decline in Spring Phytoplankton Biomass Derived from COVID-19 Lockdown Effect in the Yellow Sea Offshore Waters. Marine Pollution Bulletin174, 113175. doi: 10.1016/j.marpolbul.2021.113175
Yue X., Liao H., Wang H. J., Li S. L., Tang J. P. (2011). Role of Sea Surface Temperature Responses in Simulation of the Climatic Effect of Mineral Dust Aerosol. Atmospheric Chem. Phys. 11 (12), 6049–6062. doi: 10.5194/acp-11-6049-2011
Yu L., Wang G., Zhang R., Zhang L., Song Y., Wu B., et al. (2013). Characterization and Source Apportionment of PM2. 5 in an Urban Environment in Beijing. Aerosol air Qual. Res. 13 (2), 574–583. doi: 10.4209/aaqr.2012.07.0192
Zeng C., Yang L., Zhu A. X. (2017). Construction of Membership Functions for Soil Mapping Using the Partial Dependence of Soil on Environmental Covariates Calculated by Random Forest. Soil Sci. Soc. America J. 81 (2), 341–353. doi: 10.2136/sssaj2016.06.0195
Zhang H. H., Yang G. P., Liu C. Y., Su L. P. (2013). Chemical Characteristics of Aerosol Composition Over the Yellow Sea and the East China Sea in Autumn. J. atmospheric Sci. 70 (6), 1784–1794. doi: 10.1175/JAS-D-12-0232.1
Zhang Y., Yu Q., Ma W., Chen L. (2010). Atmospheric Deposition of Inorganic Nitrogen to the Eastern China Seas and its Implications to Marine Biogeochemistry. J. Geophysical Res. Atmospheres 115 (D7). doi: 10.1029/2009JD012814
Keywords: COVID-19 lockdown, aerosol, spring bloom, random forest, Central Yellow Sea
Citation: Baek J-Y, Park J, Kim D-W, Lee J-S, Lee J-Y, Lee S-J and Jo Y-H (2022) Role of Aerosols in Spring Blooms in the Central Yellow Sea During the COVID-19 Lockdown by China. Front. Mar. Sci. 9:911819. doi: 10.3389/fmars.2022.911819
Received: 03 April 2022; Accepted: 08 June 2022;
Published: 23 September 2022.
Edited by:
Cédric Jamet, UMR8187 Laboratoire d’océanologie et de géosciences (LOG), FranceReviewed by:
Julien Brajard, Nansen Environmental and Remote Sensing Center (NERSC), NorwayBing Han, National Marine Technology Center, China
Copyright © 2022 Baek, Park, Kim, Lee, Lee, Lee and Jo. This is an open-access article distributed under the terms of the Creative Commons Attribution License (CC BY). The use, distribution or reproduction in other forums is permitted, provided the original author(s) and the copyright owner(s) are credited and that the original publication in this journal is cited, in accordance with accepted academic practice. No use, distribution or reproduction is permitted which does not comply with these terms.
*Correspondence: Young-Heon Jo, am95b3VuZ0BwdXNhbi5hYy5rcg==