- 1Institute of Oceanography, Hellenic Centre for Marine Research (HCMR), Anavyssos, Greece
- 2Marine Biology Station, National Institute of Biology (NIB), Piran, Slovenia
- 3Remote Sensing Laboratory, National Technical University of Athens (NTUA), Zographou, Greece
Marine primary producers are highly sensitive to environmental deterioration caused by natural and human-induced stressors. Following the Water Framework Directive and the Marine Strategy Framework Directive requirements, the importance of using the different primary producers of the coastal marine ecosystem (pelagic: phytoplankton and benthic: macroalgae and angiosperms) as appropriate tools for an integrated assessment of the ecological status of the coastal environment has been recognized. However, the processes by which water column characteristics and phytobenthic indicators are linked have not been systematically studied. Based on a large dataset from three Mediterranean sub-basins (Adriatic, Ionian and Aegean Seas) with different trophic conditions, this study aims to explore the coupled responses of benthic and pelagic primary producers to eutrophication pressures on a large scale, focusing on the structural and functional traits of benthic macroalgal and angiosperm communities, and to investigate the key drivers among the different eutrophication-related pelagic indicators (such as nutrient and Chl-a concentrations, water transparency, etc.) that can force the benthic system indicators to low ecological quality levels. In addition to the effects of high nutrient loading on phytoplankton biomass, our results also show that increased nutrient concentrations in seawater have a similar effect on macroalgal communities. Indeed, increasing nutrient concentrations lead to increased coverage of opportunistic macroalgal species at the expense of canopy-forming species. Most structural traits of Posidonia oceanica (expressed either as individual metrics: shoot density, lower limit depth and lower limit type, or in the context of PREI index) show opposite trends to increasing levels of pressure indicators such as ammonium, nitrate, phosphate, Chl-a and light attenuation. Furthermore, our results highlight the regulating effect of light availability on the ecological status of seagrass meadows (Posidonia oceanica and Cymodocea nodosa). Increasing leaf length values of C. nodosa are closely associated with higher turbidity values linked to higher phytoplankton biomass (expressed as Chl-a). Overall, the coupling of pelagic and benthic primary producers showed consistent patterns across trophic gradients at the subregional scale.
Introduction
The use of biological indicators to monitor the status and trends of aquatic ecosystems has attracted much attention in recent decades (Martínez-Crego et al., 2010; Pawlowski et al., 2018). In the context of European directives, i.e., the Water Framework Directive (WFD, 2000/60/EC) and the Marine Strategy Framework Directive (MSFD, 2008/56/EC), biological indicators have been recognized as valuable monitoring tools as they enable ecosystem integrity by focusing on biological communities and not on only chemical and physical characteristics (Orfanidis et al., 2020).
Marine primary producers (phytoplankton and macrophytes) are very sensitive to environmental deterioration caused by natural and human-induced stressors. Therefore, both pelagic (phytoplankton) and benthic communities (macroalgae and angiosperms) are considered reliable ecological indicators that can integrate and adequately reflect natural and anthropogenic pressures in the coastal marine ecosystem (Orlando-Bonaca et al., 2015; Gerakaris et al., 2017; Varkitzi et al., 2018a; Rombouts et al., 2019; Orfanidis et al., 2001; Orfanidis et al., 2020). The importance of using the various marine primary producers as valuable indicators of coastal ecosystems’ health is widely recognized (Orfanidis et al., 2001; Devlin et al., 2007; Orfanidis et al., 2007; Tweddle et al., 2018).
In accordance with WFD and MSFD requirements, phytoplankton, macroalgae and marine angiosperms are used as biological quality elements (BQEs) or indicators for the assessment of ecological and environmental status (ES) of EU coastal waters. In particular, in the context of the WFD, the Mediterranean water types, reference conditions and boundaries for each BQE were defined during the intercalibration process for Mediterranean coastal waters in European Member States (MS). For the Mediterranean pelagic habitats, only Chlorophyll-a (Chl-a) concentrations have been identified as an operational intercalibrated indicator so far (UNEP/MAP, 2017; Varkitzi et al., 2018a). On the contrary, several indices were intercalibrated for the macrophyte communities: two biotic indices for macroalgae, namely the EEI-c index (Orfanidis et al., 2001; Orfanidis et al., 2011) and the CARLIT index (Ballesteros et al., 2007) and a few biotic indices for seagrasses, namely POMI (Romero et al., 2007a; Romero et al., 2007b), PREI (Gobert et al., 2009) and Valencian CS (Fernadez-Torquemada et al., 2008) for Posidonia oceanica (L.) Delile and CymoSkew (Orfanidis et al., 2007; Orfanidis et al., 2010; Orfanidis et al., 2020) and MediSkew (Orlando-Bonaca et al., 2015; Orlando-Bonaca et al., 2016) for Cymodocea nodosa (Ucria) Ascherson.
The efficiency of the proposed indicators and biotic indices for the pelagic and benthic ecosystem for robust environmental assessments is well-documented (e.g., Orfanidis et al., 2001; Orlando-Bonaca et al., 2015; Gerakaris et al., 2017; Varkitzi et al., 2018a; Orfanidis et al., 2020). Most phytoplankton indicators (Chl-a and diversity) are able to discriminate between the highest and lowest pressure levels and maintain this sensitivity across latitudinal and longitudinal gradients; thus, their use at broad spatial scales has been recommended to cover broader gradients of natural and anthropogenic stress (Varkitzi et al., 2018a; Magliozzi et al., 2021; Magliozzi et al., 2021; Francé et al., 2021). The two indices for C. nodosa (CymoSkew and MediSkew) take advantage of the high phenotypic plasticity of the species (e.g., asymmetry of leaf length distribution) attributed to limiting resources such as nutrient availability (Mvungi and Mamboya, 2012; Marbà et al., 2013) and light (Abal et al., 1994; Orfanidis et al., 2010) and successfully use it as an early warning indicator of coastal ecosystem status and trends. Regarding macroalgal communities, EEI-c has been selected as the most appropriate index for assessing the ES of coastal waters in the eastern Mediterranean and northern Adriatic basins (MED-GIG, 2011). It takes into account the functional characteristics of the species in order to indicate changes in resource availability (mainly nutrients and light) that can alter species composition and abundance (Orfanidis et al., 2011). Finally, the proposed indices for P. oceanica are more complex and use different metrics that provide information at different organization levels (i.e., individual, population, and community), on different types of pressures (e.g., water transparency, nutrient concentrations and eutrophication, sediment dynamics), and with different response times (Gobert et al., 2009; Martínez-Crego et al., 2010).
If eutrophication is the main driving factor of environmental quality, the associated pelagic indicators (such as nutrient and Chl-a concentrations, water transparency, etc.) are expected to drive the benthic system indicators to low ecological quality levels (Graf, 1992). To date, the literature has mainly reported modelling approaches to describe the relationships between pelagic primary production and oxygen demand in sediments (Hargrave, 1973; Vaquer-Sunyer and Duarte, 2008), organic matter content in sediments (Brady et al., 2013), and sediment biogeochemical features (Grangeré et al., 2012). Sparse attempts to quantify the impacts of pelagic parameters on benthic communities suggest some concentrations of Chl-a and dissolved oxygen in the seawater column as thresholds above which zoobenthos in Scottish lochs is suggested to deteriorate (CSTT, 1997; Tett et al., 2008). Another study from the oligotrophic eastern Mediterranean Sea investigated the quantitative relationship between benthic macrofaunal communities (macroinvertebrates) and seawater column variables and showed a strong link between pelagic indicators (Chl-a and Eutrophication Index - EI) and various benthic indicators describing macrofaunal diversity (Dimitriou et al., 2015).
To the best of our knowledge, the processes by which water column characteristics and phytobenthic indicators are linked have not been systematically studied. In the eastern Mediterranean, primary producers, such as macroalgae and phytoplankton, generally appear to be more sensitive to nitrogen and particulate matter in seawater, while benthic macroinvertebrate indicators are more sensitive to phosphate in seawater (Simboura et al., 2016). A follow-up study reported that nutrients in seawater and macroalgae are components with a stronger temporal response to mitigation measures taken (Pavlidou et al., 2019). However, how changes in trophic conditions in pelagic habitats may affect the structural and functional traits of macroalgal and angiosperm communities has not been studied in detail.
Based on the analysis of a pooled dataset of sampling sites in three Mediterranean sub-basins (northern Adriatic, eastern Ionian and Aegean Seas) reflecting different trophic conditions, this study aims to improve our understanding of how different benthic and pelagic primary producers are linked under natural and human-induced pressure gradients in coastal ecosystems. To this end, the main objectives of this study were (i) to explore the coupled responses of benthic and pelagic primary producers (macroalgae, angiosperms, and phytoplankton) to eutrophication pressure at large scales, (ii) to examine how different trophic conditions of the pelagic habitat may affect the structural and functional traits of benthic macroalgal and angiosperm communities, and (iii) to investigate which are the key drivers among the various eutrophication-related pelagic indicators (such as nutrient and Chl-a concentrations, water transparency, etc.) that can force the benthic system indicators to low ecological quality levels.
Materials & Methods
Dataset Description
Three different datasets were used, consisting of samples collected in different scientific and monitoring programmes in different sub-regions of the Mediterranean Sea (northern (N.) Adriatic, Eastern (E.) Ionian and Aegean Seas) (Figure 1). The dataset used in the present study includes 65 sampling sites from Greece (25 in the Ionian Sea, 40 in the Aegean Sea) and 26 sampling sites from the Slovenian part of the Gulf of Trieste (N. Adriatic). The dataset is based on a compilation of published data (Orlando-Bonaca et al., 2008; Orlando-Bonaca et al., 2015; Giovanardi et al., 2018; Orlando-Bonaca and Rotter, 2018; Orlando-Bonaca et al., 2019; Gerakaris et al., 2021; Orlando-Bonaca et al., 2021) and unpublished data from the Hellenic Centre of Marine Research and the National Institute of Biology (Table 1).
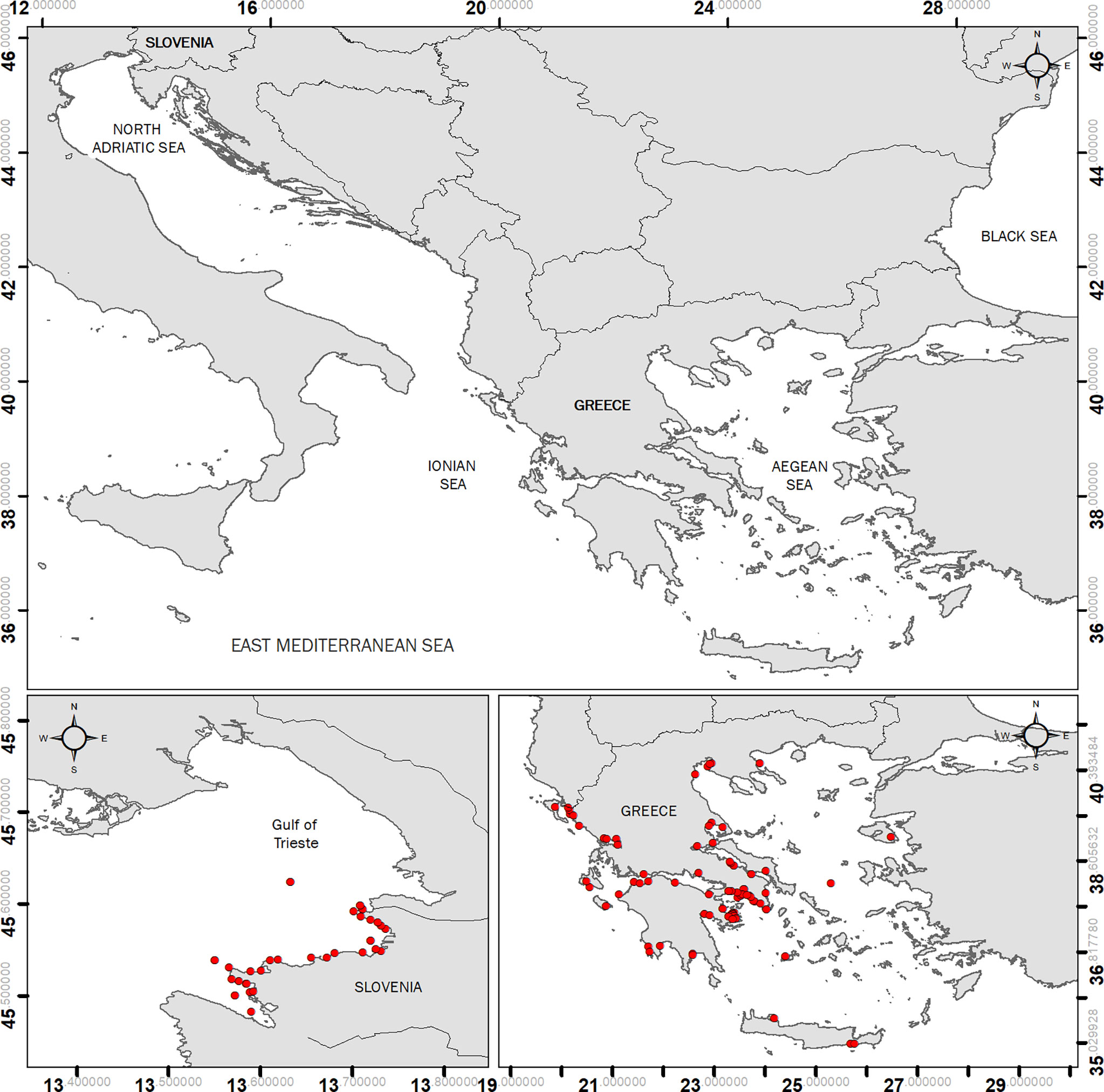
Figure 1 Map of the study area with the three Mediterranean sub-regions (upper panel) and the distribution of the sampling sites on local scale (lower panels).
The dataset for the study of macroalgae was collected in the Greek and Slovenian coastal areas and includes two subsets (Figure 1). The first subset includes data (111 observations) collected in the Greek coastal areas, while the second subset (111 observations) is from the Slovenian coastal waters. The dataset for the study of the seagrass C. nodosa was collected in the Greek and Slovenian coastal areas and includes two subsets. The first subset includes data (16 observations) collected in the Greek coastal areas, while the second (16 observations) is from the Slovenian coastal waters. The dataset for the study on seagrass P. oceanica was collected only in the Greek coastal areas. The dataset includes data (50 observations) from the E. Ionian and the Aegean Seas.
Pelagic Indicators
Pelagic variables (seawater column) and indicators included concentrations of inorganic nutrients (phosphate, nitrate, ammonium), Chlorophyll-a (Chl-a) and dissolved oxygen (DO), temperature, salinity, pH, and diffuse attenuation coefficient (Kd). Data for the pelagic variables were obtained from the open access data repository Copernicus Marine Environment Monitoring Service (CMEMS). Table 2 summarizes the pelagic variables studied with the corresponding CMEMS products we used. For each sampling station, in the case of products with a resolution of 0.042° x 0.042°, we used a 3 x 3 km pixel window to estimate the mean values of the variables studied and then calculate the annual mean values or the means for the previous three months (see also Data analysis below). For Kd and Chl-a products with 1 km resolution, an 11 x 11 km pixel window was used to keep the same study region (pixel area) for all variables. Experiments with pixel windows smaller than 11 x 11 km, such as 5 x 5 km, yielded similar results for Kd and Chl-a products.

Table 2 The collected CMEMS products for the pelagic variables, with the temporal and spatial resolutions of each product.
Pelagic variables from the Slovenian dataset were obtained during the National Monitoring Program campaigns from 2007 to 2020. Seawater samples for Chl-a and nutrients were collected monthly using Niskin bottles, while temperature, salinity and dissolved oxygen concentration were obtained using a CTD probe (Sea & Sun Technology GmbH, Germany). Chl-a concentrations were analyzed fluorimetrically with a Turner Designs Trilogy fluorimeter (Holm-Hansen et al., 1965). For the period 2007 - 2013, concentrations of inorganic nutrients were measured using standard colorimetric methods (Grasshoff et al., 1999). For the period 2014 - 2020, nutrient concentrations were determined spectrophotometrically using the QuAAtro autoanalyzer (Seal Analytical).
Benthic Indicators
A suite of indicators was selected for the benthic ecosystem to assess the status of coastal waters using macroalgae and seagrasses (C. nodosa and P. oceanica).
The Ecological Evaluation Index continuous formula (EEI-c) provides information on the response of benthic macrophytes to anthropogenic pressures (Orfanidis et al., 2011). This method divides macrophyte taxa into two Ecological State Groups (ESGs). ESG I includes thick perennial (IA), thick plastic (IB) and shade-adapted plastic (IC) species in coastal waters and angiosperm plastic (IA), thick plastic (IB) and shade-adapted plastic (IC) species in transitional waters. ESG II includes fleshy opportunistic (IIB) and filamentous leafy opportunistic (IIA) species in both coastal and transitional waters (Orfanidis et al., 2011). The relative abundance (%) of opportunistic species was also examined.
Two indices were used to assess the status of C. nodosa meadows: CymoSkew for the assessment of the data from the E. Ionian and the Aegean Sea and MediSkew for the data from the N. Adriatic Sea. The CymoSkew index is a quantitative expression of photosynthetic leaf length asymmetry of C. nodosa used as an index of early warning response (Orfanidis et al., 2010; Orfanidis et al., 2020) and calibrated against the anthropogenic stress index MA-LUSI (Papathanasiou and Orfanidis, 2018). The MediSkew index is a combination of two metrics, both based on C. nodosa leaf length: Deviation from the reference median length (Medi-) and Skewness of length-frequency distribution (-Skew), with greater importance given to the first, and was calibrated with a Pressure Index for Seagrass Meadows (Orlando-Bonaca et al., 2015). Median Leaf length of C. nodosa was used as an additional metric in the analysis.
The PREI (Posidonia oceanica Rapid Easy Index) is a multimetric index based on the integration of five individual metrics of P. oceanica: Shoot density, Shoot leaf surface, Epiphytic biomass/Leaf biomass ratio, Depth of Lower Limit, and type of the Lower Limit (Gobert et al., 2009). The P. oceanica metrics (indicators) used in the PREI index provide information on the vitality of the meadow (at the individual and population level) for a wide range of disturbances, including light deprivation, nutrient concentrations, and eutrophication (Gobert et al., 2009; Martínez-Crego et al., 2010).
Ecological quality ratio (EQR) values were calculated for the EEI-c, Cymoskew, Mediskew, and PREI biotic indices and used in the analyses. EQR values for all of these indices range from 0 for worst status to 1 for the best status. For the C. nodosa dataset (hereafter referred to as CymodoceaEQR), EQR values for the E. Ionian and Aegean Seas were calculated following the conversion formula of Orfanidis et al. (2020), while the equation EQR = 1 – MediSkew was used for the N. Adriatic data (Orlando-Bonaca et al., 2015).
Data Analysis
Prior to statistical analyses, all pelagic variables were averaged for the three months preceding the sampling of macroalgae and seagrass C. nodosa, while annual means were used for P. oceanica.
Multivariate analysis was performed in the R Environment (R Core Team, 2021). Prior to analysis, data were tested for normality using the shapiro.test() function. If normality was not achieved, the bestNormalize() function was used to find the best transformation of the data. The Kaiser–Meyer–Olkin measure (KMO) checked the sampling adequacy for the analysis. All KMO values for each item were > 0.70, which is well above the acceptable threshold of 0.6. Principal Component Analysis (PCA) was performed for both benthic and pelagic indicators and variables to check for the presence of common patterns. Prior to ordination, a Kendall’s Tau correlation coefficient matrix was created between benthic and pelagic indicators and variables to highlight parameters with high and statistically significant correlation. All plots were created using the package “ggplot2” (Wickham, 2016).
Results
Macroalgae Indicators vs Pelagic Indicators
When benthic and pelagic variables and indicators were considered for the macroalgae dataset (Table 3), significant negative correlations (p < 0.01) were found between the benthic index EEI-c and the pelagic eutrophication variables of nutrients (phosphate, nitrate), Chl-a and oxygen, according to Kendall’s Tau correlation coefficient. The abundance of opportunistic species (%) of macroalgae showed a strong negative correlation with EEI-c and a positive correlation with Chl-a, phosphate, ammonium and pH. Chl-a was significantly correlated with all other pelagic variables (p < 0.01), negatively with temperature and salinity, and positively with nutrients, oxygen, and pH.
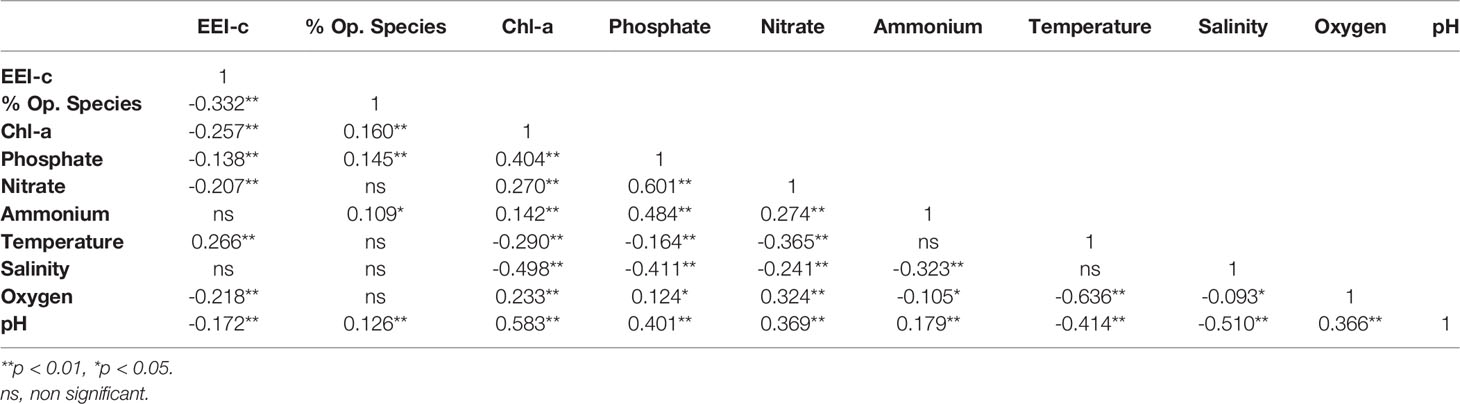
Table 3 Kendall’s Tau correlation coefficient matrix between pelagic and benthic indicators and variables for macroalgae dataset.
Principal component analysis (PCA) of the pelagic variables and benthic indicators EEI-c and Opportunistic Species (%) considered for the macroalgae dataset revealed two significant principal components (PCs; i.e., eigenvalues>1) that together account for 68.3% (PCA for EEI-c) and 66.8% (PCA for Opportunistic Species %) of the total variance in the dataset (Table 4). This is due to the fact that all other pelagic indicators (with the exception of ammonium) have strong loadings (>0.70) that fall under these two components.
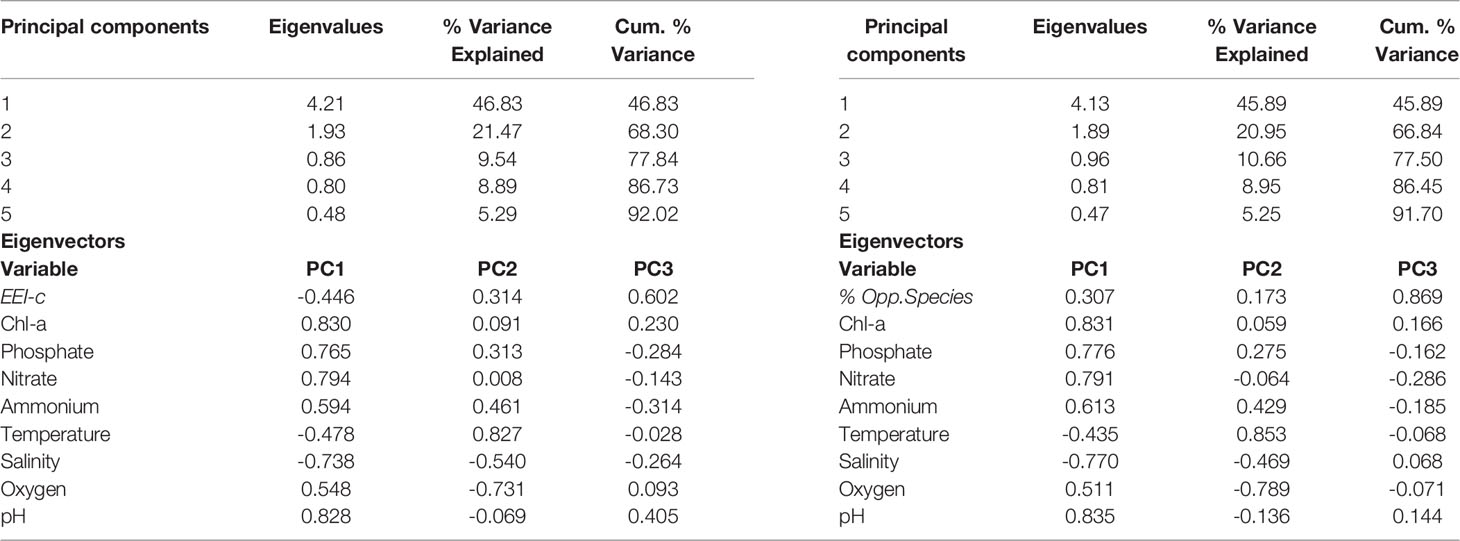
Table 4 Results of the PCA applied on pelagic and benthic indicators for macroalgae (EEI-c as EQR and % Opportunistic species).
Looking at the EEI-c index, the studied macroalgal sites can be divided into two major clusters (ordination biplot, Figure 2A): the cluster of sites in N. Adriatic Sea, which is mainly located in the positive part of PC1, and the cluster of sites in the E. Aegean and Ionian Seas, which is mainly located in the negative part of PC1. The Aegean - Ionian Sea cluster is better associated with increasing trends of EEI-c, salinity and temperature towards the negative PC1. In contrast, most of the N. Adriatic cluster is better associated with increasing trends of Chl-a, nutrients (phosphate, nitrate, ammonium), oxygen, and pH on the positive PC1. EEI-c contributed similarly to both PCs (see Table 4 for PC1 and PC2), while pointing in the opposite direction to the pelagic eutrophication indicators (Chl-a, nutrients, and oxygen).
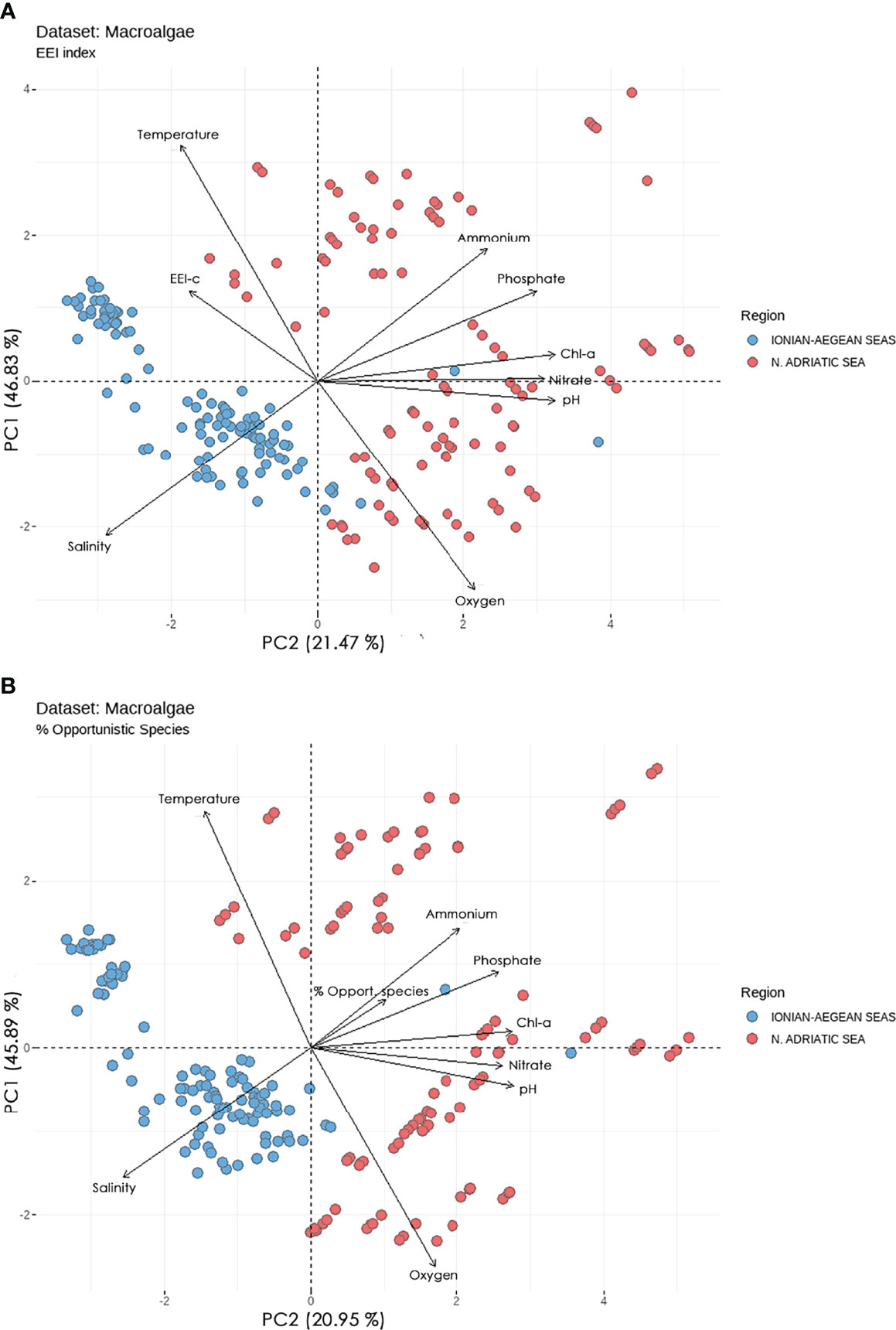
Figure 2 Principal component analysis (PCA) biplots of studied sites and pelagic and benthic indicators (A) EEI-c index, and (B) Opportunistic Species (%). The biplot shows the PCA scores of the indicators as vectors (in black) and sites as circles (blue circles = Ionian-Aegean seas, red circles = N. Adriatic Sea).
When considering Opportunistic Species (%), the studied sites are divided into the same two clusters (ordination biplot, Figure 2B) already highlighted for EEI-c. Furthermore, the vectors of pelagic indicators and variables showed a very similar ordination and trend as the PCA biplot for EEI-c; however, the vector of Opportunistic Species (%) is reversely ordinated. Opportunistic Species (%) seemed to be mainly associated with nutrients and pH.
Cymodocea nodosa Indicators vs Pelagic Indicators
The results of Kendall’s Tau correlation coefficient calculated between pelagic and benthic indicators for the C. nodosa dataset (Table 5) showed a significant negative correlation (p < 0.01 and p < 0.05) between CymodoceaEQR and Leaf Length. Among all pelagic variables, Leaf Length values are significantly correlated only with nitrate, while CymodoceaEQR is not correlated with any pelagic variable. Chl-a is significantly correlated with all pelagic variables and showed strong positive correlations with phosphate, Kd, pH and a strong negative correlation with salinity.
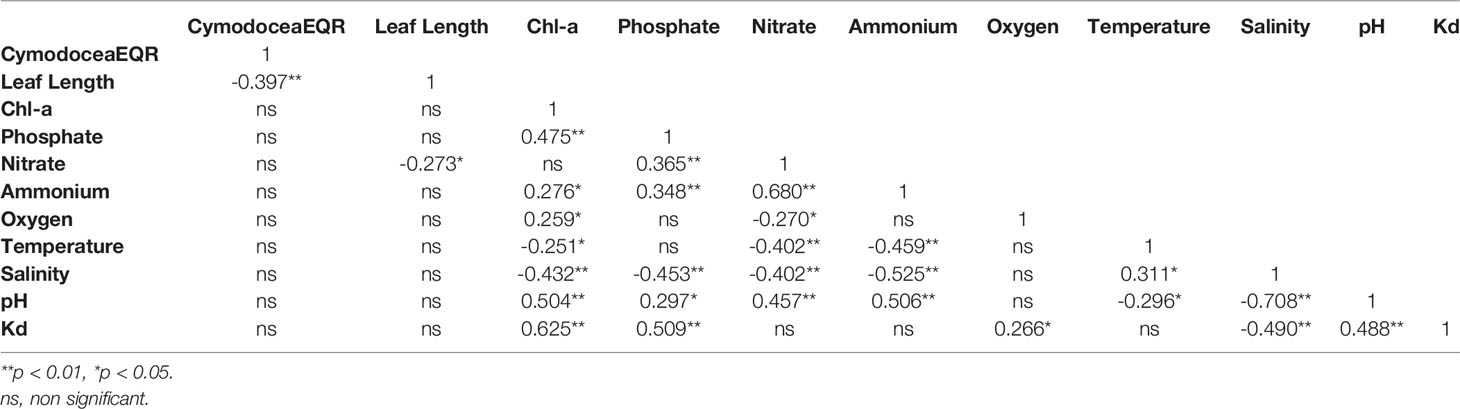
Table 5 Kendall’s Tau correlation matrix between the benthic and pelagic indicators from the Cymodocea nodosa dataset.
Principal component analysis (PCA) of the pelagic variables and benthic indicator CymodoceaEQR, considered for the C. nodosa dataset, revealed three significant principal components (PCs; i.e., eigenvalues>1) that together account for 80.6% of the total variance in the dataset; with PC1 and PC2 accounting for > 65% of the total variance (Table 6). This is because 75% of the pelagic indicators analyzed have a strong loading (> 0.75) that falls under these two components.
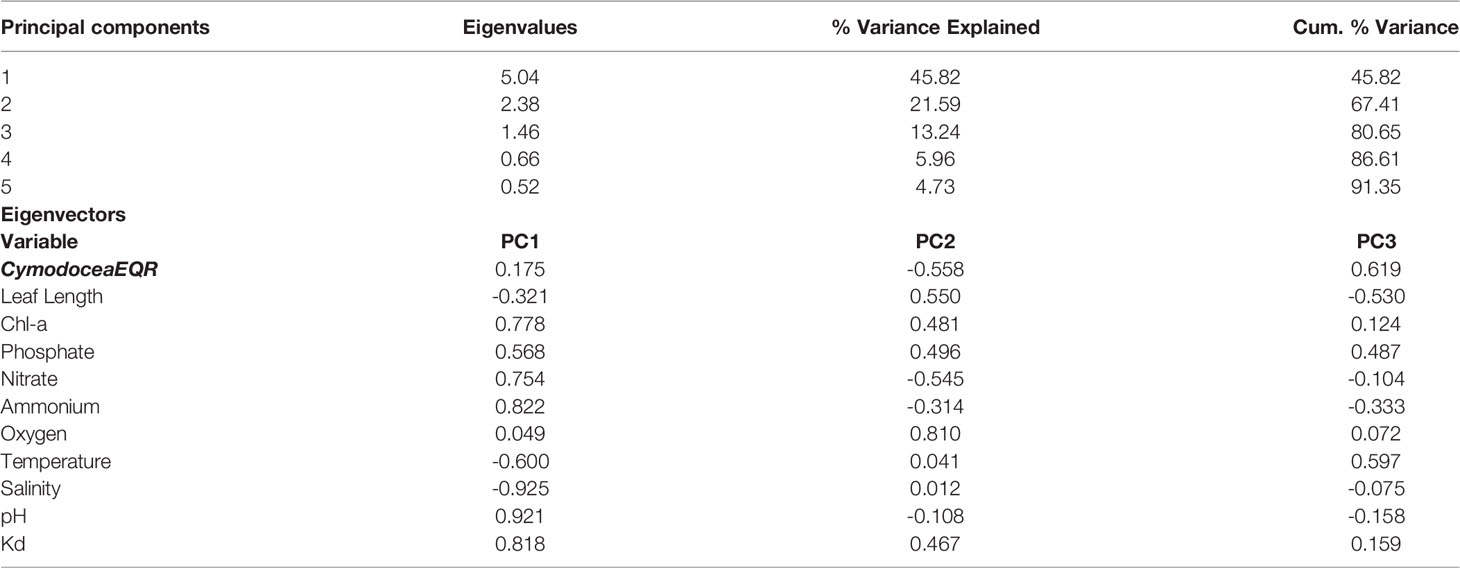
Table 6 Results of the PCA applied on pelagic and benthic indicators for C. nodosa (EQR, and median Leaf Length).
The studied sites are divided into three clusters (ordination biplot, Figure 3). Cluster 1, on the negative part of PC1, is characterized mainly by higher values of temperature and salinity, while cluster 2, on the positive part of PC1, is characterized by higher values of pelagic eutrophication indicators (nutrients, Chl-a, Kd) and pH. Cluster 3, formed remotely from some sites in the Aegean Sea on the positive part of PC2, is characterized by higher oxygen values.
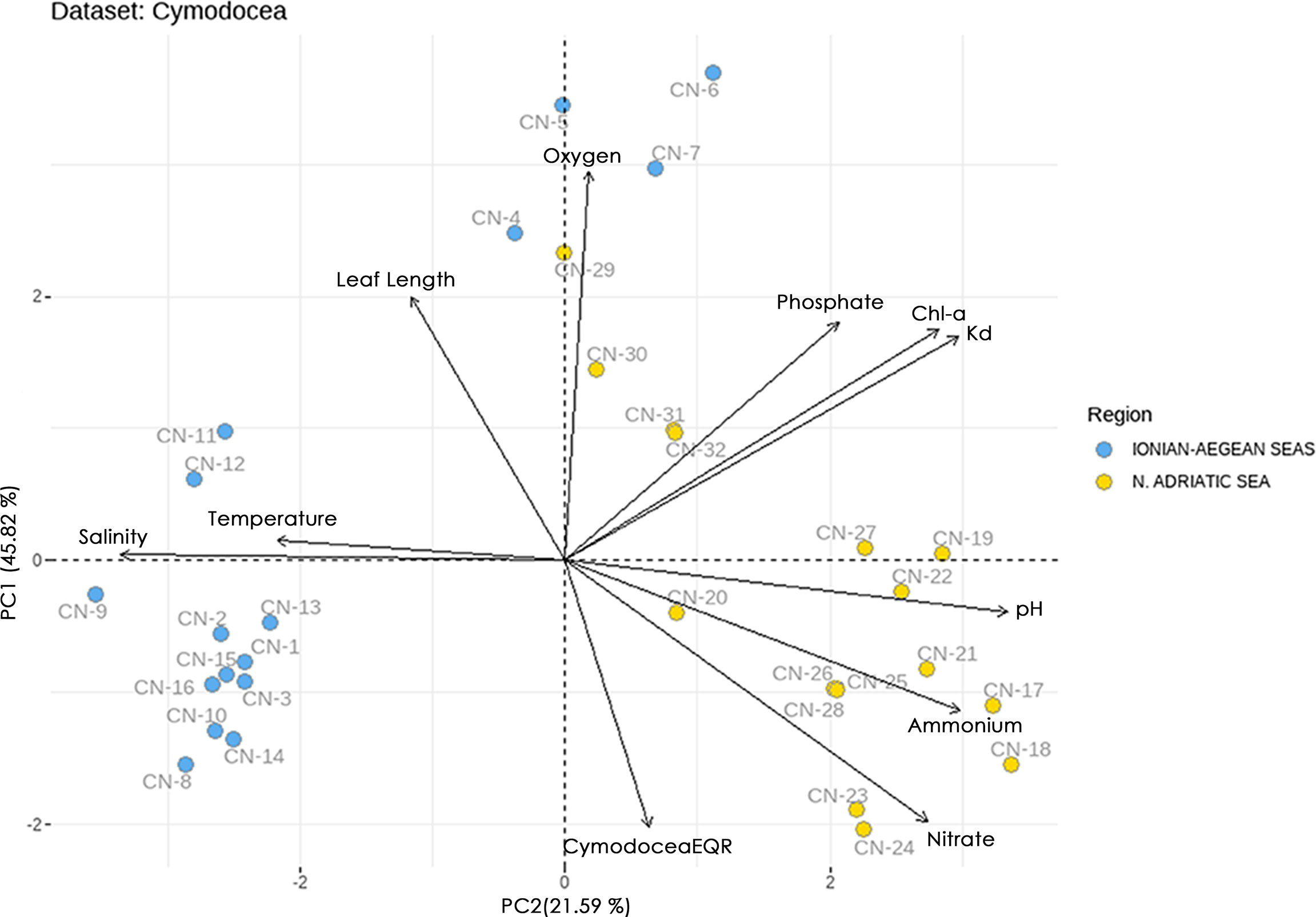
Figure 3 Principal component analysis (PCA) biplots of studied sites and pelagic and benthic indicators for C. nodosa dataset. The biplot shows the PCA scores of the indicators as vectors (in black) and sites (blue circles = Ionian-Aegean seas, yellow circles = N. Adriatic Sea).
Attempting to elucidate further this clustering, the Ecological Status (ES) of the studied sites, as assessed by the biotic indices CymoSkew and MediSkew and expressed as CymodoceaEQR was introduced to the ordination biplot (Figure 4). The sites in the positive part of PC2 have lower CymodoceaEQR values (i.e., poor, bad and most moderate ES), while the remaining sites in the negative part of PC2 have higher CymodoceaEQR values (high and good ES).
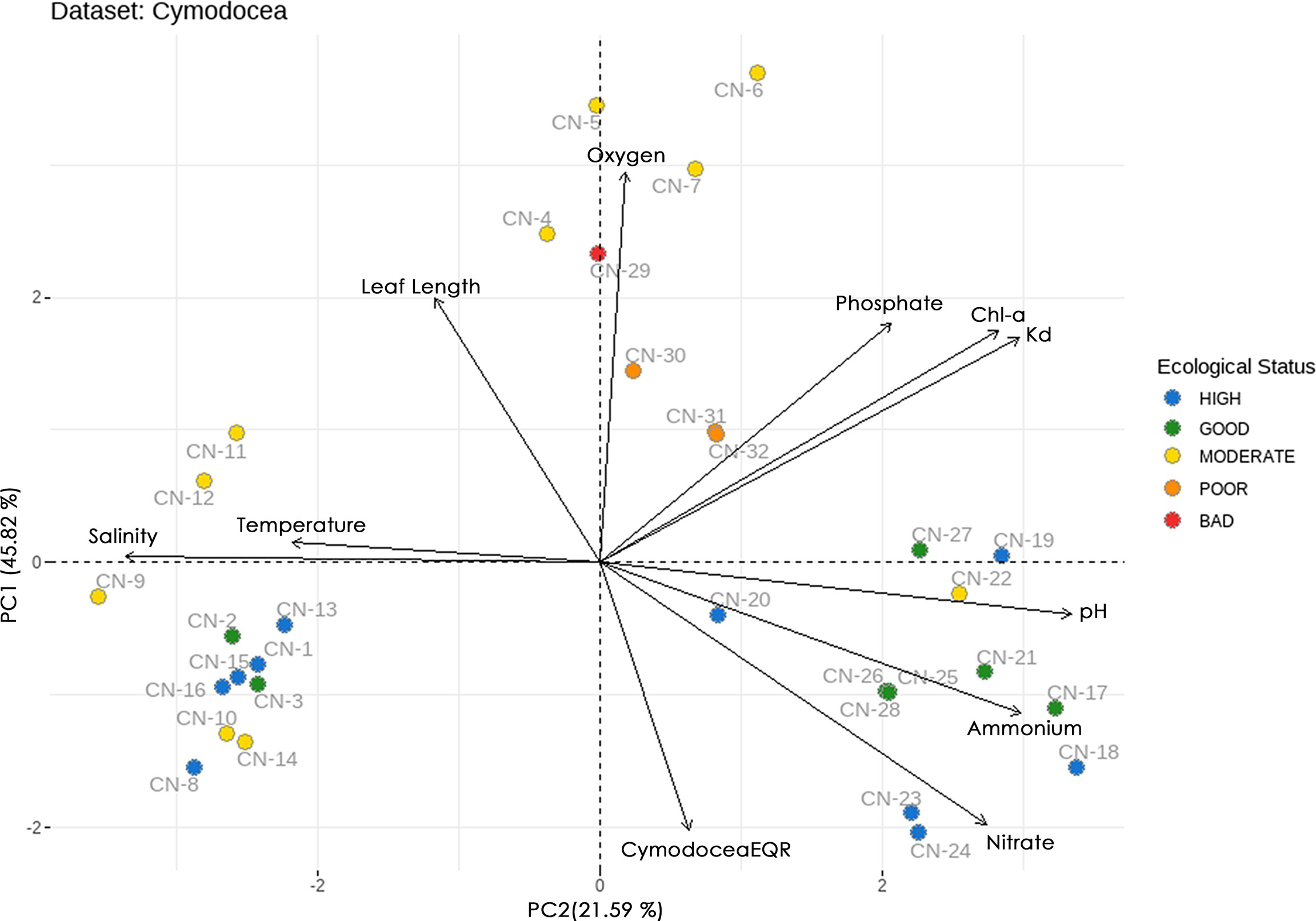
Figure 4 Principal component analysis (PCA) biplots of studied sites and pelagic and benthic indicators for C. nodosa dataset. The biplot shows the PCA scores of the indicators as vectors (in black) and sites (coloured based on their ecological status assessment).
Posidonia oceanica Indicators vs Pelagic Indicators
The results of Kendall’s Tau correlation coefficients calculated between pelagic and benthic indicators for the P. oceanica dataset (Table 7) show significant correlations (p < 0.01 and p < 0.05) between Chl-a and LL depth and most pelagic indicators and variables. The PREI index is positively correlated with all P. oceanica metrics (except E/L Biomass) and nitrate while negatively correlated with Chl-a and Kd. As for nutrients, Chl-a showed a strong negative correlation with phosphate and nitrate but a non-significant correlation with ammonium. Kd and Chl-a showed a strong positive correlation as expected, because suspended particulate matter, including phytoplankton, contributes to high Kd.
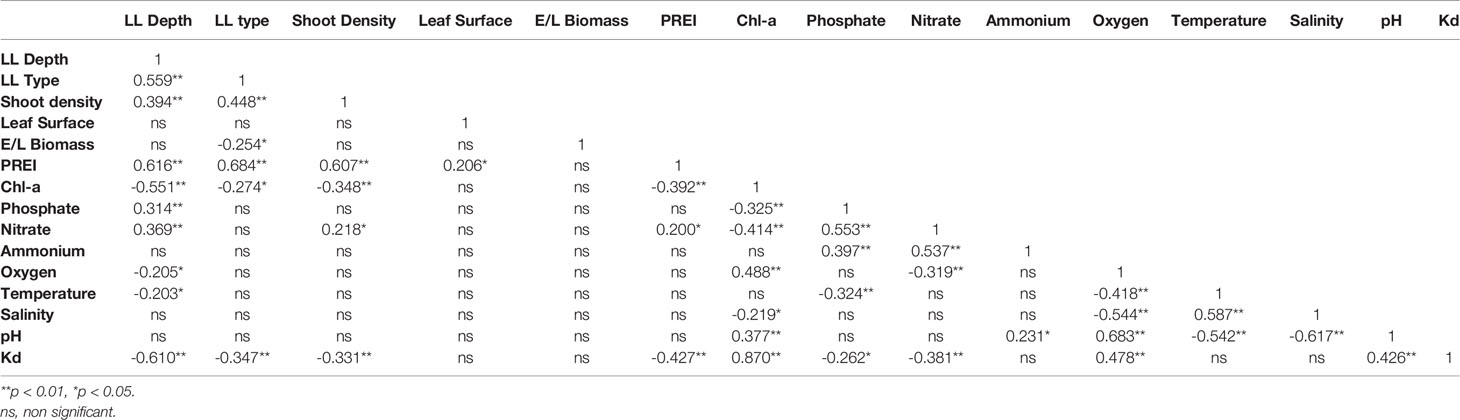
Table 7 Kendall’s Tau correlation matrix between the benthic and pelagic indicators for P. oceanica dataset.
Principal component analysis (PCA) of the pelagic and benthic indicators considered for the P. oceanica dataset revealed three significant principal components (PCs; i.e., eigenvalues>1) that together account for 90.5% (PCA for PREI) and 79% (PCA for P. oceanica metrics-indicators) of the total variance in the dataset; with PC1 and PC2 accounting for > 60% of the total variance (Table 8). This is due to the fact that > 80% of the analyzed pelagic indicators have strong loadings (>0.5) that fall under these two components.
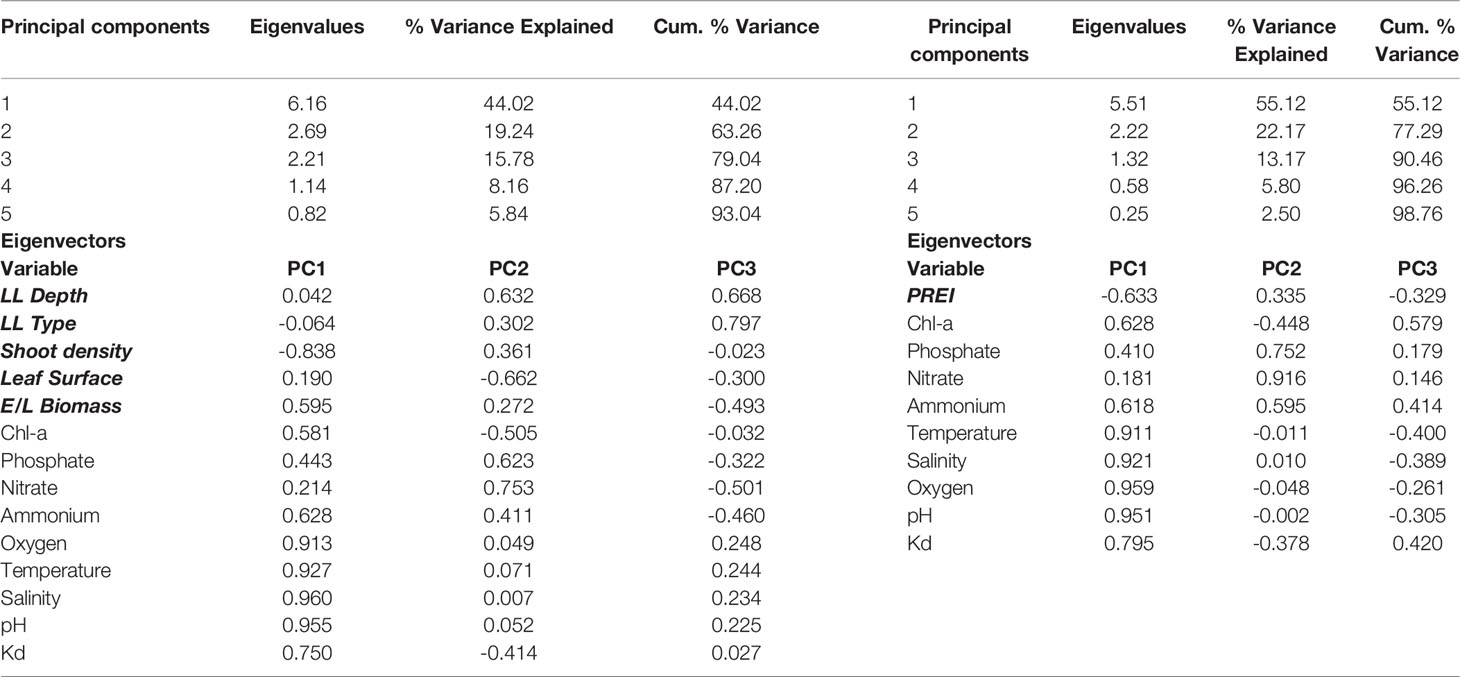
Table 8 Results of the PCA applied on pelagic and benthic seagrass indicators (P. oceanica metrics, and PREI, respectively).
Looking at the P. oceanica metrics, the studied sites can be divided into three clusters (ordination biplot, Figure 5A). Cluster 1, in the positive part of PC1, is characterized by high values for nutrients, temperature, salinity, oxygen, pH, Kd, Chl-a, and E/L Biomass and low values for Shoot density. Cluster 2, in the negative part of PC2, is characterized by high values for Chl-a, Kd, and Leaf Surface and low values for nutrients, LL Depth, LL type, and Shoot density. Cluster 3, in the negative part of PC1, is characterized by high values for Shoot density, LL Depth, LL type and low values for Kd, oxygen, Chl-a, pH, salinity, temperature, E/L Biomass, Leaf Surface and ammonium.
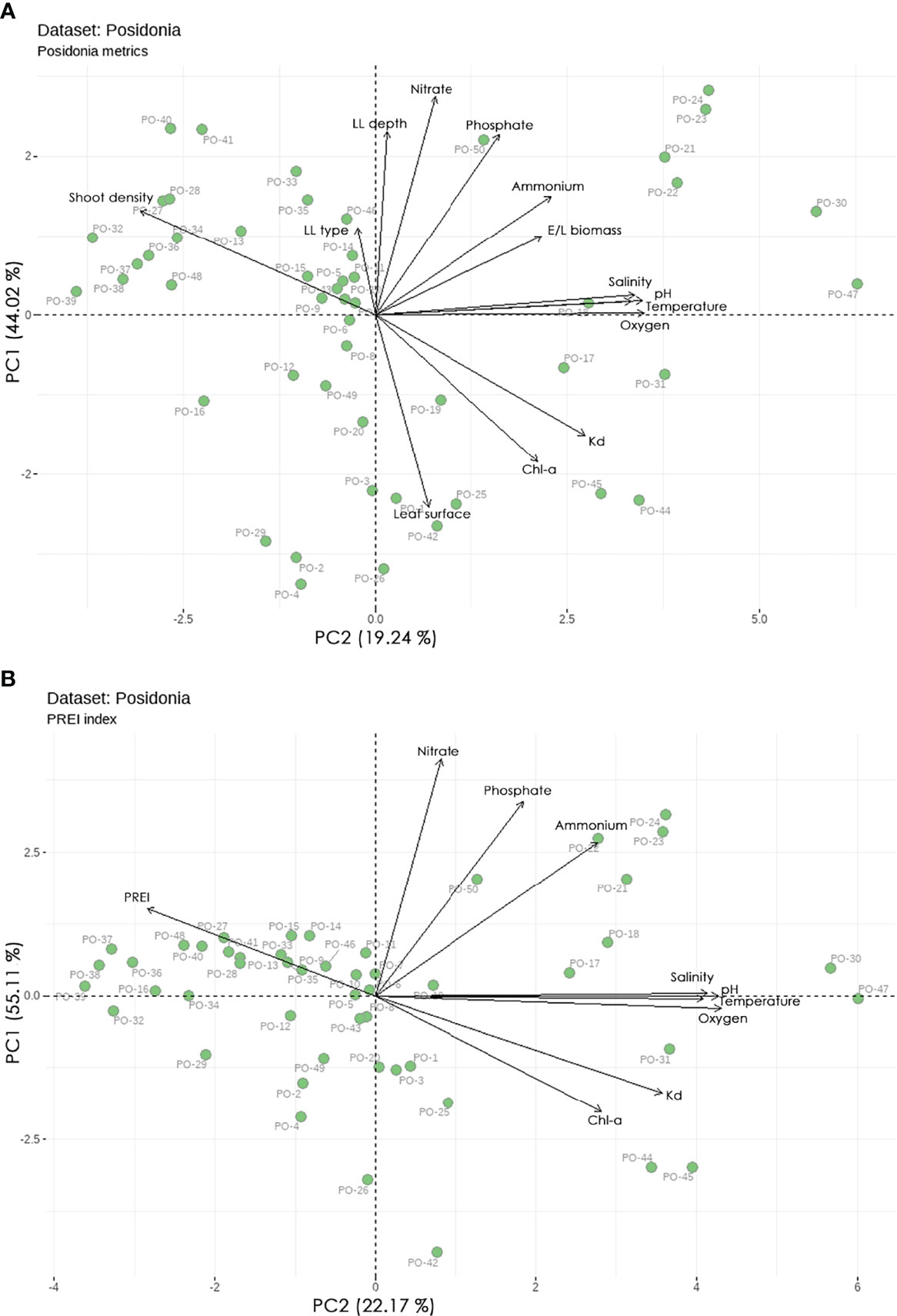
Figure 5 Principal component analysis (PCA) biplot of studied sites and pelagic and benthic indicators (A) P. oceanica metrics, and (B) PREI index. The biplot shows the PCA scores of the indicators as vectors (in black) and sites (green circles).
Focusing on the PREI index, the studied sites can also be divided into three clusters, with the total explained variance increasing to 77.3% (ordination biplot, Figure 5B). Cluster 1, in the negative part of PC1, is characterized by high values for PREI and low values for Chl-a, Kd, oxygen, pH, salinity, temperature, and ammonium. Cluster 2, in the positive part of PC1, is characterized by high values for nutrients, temperature, salinity, oxygen, pH, Kd, and Chl-a, and low values for PREI. Cluster 3, in the negative part of PC2, is characterized by high values for Chl-a, and Kd, and low values for nutrients.
Discussion
Pelagic (phytoplankton) and benthic communities (macroalgae and angiosperms) are considered robust bioindicators that efficiently reflect natural and human-induced pressures in the coastal marine ecosystem (Orfanidis et al., 2007; Orlando-Bonaca et al., 2008; Orfanidis et al., 2010; Orfanidis et al, 2011; Orlando-Bonaca et al., 2015; Varkitzi et al., 2018a; Rombouts et al., 2019; Orfanidis et al., 2020; Orlando-Bonaca et al., 2021); however, their response to stressors is related to their structural complexity and specificity (e.g., Adams and Greeley, 2000). To this end, much of the research has focused on the biology and ecology of pelagic and benthic primary producers and their responses to different stressors or impacts at different scales (physiology, population dynamics, community composition trends).
Relationships between benthic and pelagic primary producers are mainly associated with competition for light and nutrients in relatively shallow coastal ecosystems (Krause-Jensen et al., 2012; Orfanidis et al., 2020). Phytoplankton can take advantage of favorable light conditions in the upper water layers but is exposed to unstable nutrient supply, while benthic vegetation can benefit from the vast reservoir of nutrients in the sediment but is often light-limited (e.g., Duarte, 1991; Cloern et al., 2014). Excessive nutrient enrichment in the pelagic habitat stimulates rapid growth of phytoplankton and opportunistic macroalgae at the expense of seagrasses and perennial macroalgae, which are eventually eliminated by increasing light attenuation (i.e., shading) and other eutrophication effects such as hypoxia and anoxia (e.g., Valiela et al., 1992; Harlin, 1995; Schramm and Nienhuis, 1996; McGlathery et al., 2007).
Correlations between benthic primary producers and pelagic pressure indicators (nutrients) almost consistently show that the link between these stressors and the benthic community of primary producers is challenging to demonstrate. The data pooling conducted in this study was designed to improve our understanding of the effects of stressors and to facilitate the interpretation of the linkages between benthic and pelagic primary producers under complex natural and human-induced pressure gradients in the coastal ecosystems of three Mediterranean sub-basins (N. Adriatic, E. Ionian, and Aegean Seas). According to our results, Chlorophyll a (Chl-a) was significantly correlated with all other pelagic variables, such as nutrients, oxygen, pH, temperature and salinity, in all analyzed datasets for macroalgae and seagrass (C. nodosa, P. oceanica) indicators in all studied coastal waters. Apart from the fact that Chl-a measurements are simple, reproducible and time- and cost-effective, and the results are easily comparable, ensuring high data availability (Domingues et al., 2008), there is also a well-documented pressure-impact relationship between nutrient enrichment and response of phytoplankton biomass (e.g., Håkanson and Eklund 2010; Giovanardi et al., 2018). In contrast, the relationship between pressures and phytoplankton biodiversity parameters is much looser and often masked by the high complexity and dynamics of phytoplankton assemblages (Rombouts et al., 2019).
In addition to the effects of high nutrient loads on the phytoplankton community, our results also show that increased nutrient concentrations in seawater have a similar effect on the macroalgal community. Indeed, our analysis revealed that increasing nutrient levels lead to increased coverage of opportunistic macroalgal species at the expense of canopy-forming species, as previously suggested (Orfanidis et al., 2011; Orlando-Bonaca and Rotter, 2018). Opportunistic species (characterized by Orfanidis et al. (2011) as ESG II: species with high growth rates and short life history) were found to be significantly associated with eutrophication indicators (e.g., Chl-a, phosphate, ammonium), confirming that anthropogenic pressure shifts the ecosystem from a pristine state, where late-successional species (such as Cystoseira s.l. spp.) predominate, to a degraded state, where opportunistic, nitrophilous species are dominant (Orfanidis et al., 2001; Orfanidis et al., 2011). Given the above results, it is not surprising that opportunistic macroalgal species were negatively correlated with EEI-c values. Since the EEI-c index was developed to assess the impact of chronic pressures such as eutrophication and organic matter pollution (Orfanidis et al., 2001; Orfanidis et al., 2011), the significant negative correlation between this index, nutrients and Chl-a further confirms these properties of the EEI-c.
The regulating effect of light availability on the growth and depth distribution of the P. oceanica meadows studied is further supported by our results. Indeed, the clusters of sites with meadows that share higher shoot densities, LL depths, and LL type (i.e., progressive limit) show higher values of PREI index, i.e., better ecological status. In contrast, the clusters of sites with meadows that have higher values of E/L Biomass (the case of epiphytes overgrowth due to nutrient enrichment), Shoot Leaf Surface (leaves elongation as an acclimation response), along with higher values of light attenuation coefficient Kd, Chl-a, and nutrients, show lower ecological status.
As with all seagrass species, light is considered the most critical factor in regulating the distribution and growth dynamics of P. oceanica meadows (Ralph et al., 2007; Gerakaris et al., 2021). Fluctuations in light attenuation in the coastal marine environment due to direct (e.g., high turbidity due to abiotic and biotic factors) or indirect causes (e.g., shading by epiphytes/macroalgal overgrowth due to nutrient enrichment) results in specific photoadaptive physiological and morphological responses (e.g., elongation of leaves, thinning of shoot density, reduction in depth distribution, e.g., Greve and Binzer, 2004).
The P. oceanica metrics (indicators) considered in this study are the same as metrics used in the PREI index. Therefore, it is not surprising that both the PREI and the other metrics share common patterns of variation when examined in the context of the pelagic indicators. In fact, all P. oceanica indicators exhibited the expected covariance (positive or negative depending on the indicator) with the pelagic indicators examined and adequately reflected the increasing magnitude of human-induced pressures. This is particularly evident with increasing levels of the pressure indicators, such as ammonium, nitrate, phosphate, Chl-a and Kd, along which the P. oceanica indicators (PREI, Shoot density, LL depth and LL type) show the opposite trend of decreasing values.
Cymodocea nodosa is a perennial leaf self-shading species with high light requirements (Kenworthy and Fonseca, 1996; Sand-Jensen and Borum, 1991), which, as with most seagrass species, are much higher than those of macroalgae and phytoplankton (Duarte, 1991). Indeed, seagrasses exhibit photoadaptive responses under light-deficient conditions that vary widely among seagrass taxa (Ralph et al., 2007). Our results show that increasing Leaf Length values of C. nodosa are closely associated with higher turbidity levels (expressed as Kd), which could be due to higher phytoplankton biomass (expressed as Chl-a). This implies that photoacclimation of the studied C. nodosa populations is expressed with increasing leaf size to maximize the amount of captured light (Goldberg, 1996; Touchette and Burkholder, 2000; Lee et al., 2007; Orfanidis et al., 2020). The fact that CymodoceaEQR values were correlated only with Leaf Length values (negative correlation) and with none of the pelagic variables examined suggests that CymodoceaEQR responses to such pelagic stressors are not straightforward. However, PCA showed that low CymodoceaEQR were associated with increasing trends in pelagic eutrophication indicators (phosphate, Chl-a, and Kd), highlighting them as significant drivers that may force C. nodosa benthic system indicators to deteriorate in ecological quality. Moreover, the observed negative correlation between Leaf Length and nitrate concentration suggests that in our case, the increased productivity and biomass of C. nodosa leaves are not the linear result of nutrient enrichment (Lee et al., 2007).
Cymodocea nodosa thrives in highly variable environments that receive fresh water and nutrient pulses. However, the increased amounts of nutrients in the water column are not taken up by C. nodosa directly, but are trapped in sedimented particulate matter and become available to angiosperms when released in sediment pore water (Tyerman, 1989; Hemminga and Duarte, 2000). Other factors are also known to have important effects on the health of C. nodosa meadows. High sedimentation and resuspension rates (both natural and due to anthropogenic activities) are known to limit light availability and are among the main factors leading to longer seagrass leaves and lower ecological status (Orlando-Bonaca et al., 2015).
Another interesting result of the analyses of the C. nodosa dataset was the formation of a distinct group with sites in the Aegean associated with higher oxygen levels. These sampling sites are located close to Thermaikos Gulf (N. Aegean Sea), an area with direct influence of oxygen-rich freshwater inputs from five rivers, well-documented eutrophication problems, and frequent harmful algal blooms (Pagou, 2005; Ignatiades and Gotsis-Skretas, 2010; Varkitzi et al., 2013).
The sampling sites in the Aegean and Ionian Seas were distributed mainly on the positive side of the vectors for salinity and temperature, while the sampling sites in the N. Adriatic were mainly distributed around the positive sides of the vectors for the pelagic eutrophication indicators (Chl-a, nutrients, Kd). On this basis, a clear distribution pattern emerged with two distinct groups of sampling sites in the N. Adriatic and the Ionian-Aegean, which persisted for three of the benthic indicators EEI-c, Cymoskew and Mediskew (expressed as CymodoceaEQR). We hypothesize that these trends are driven by the characteristics and trophic conditions of the N. Adriatic Sea, which is colder, less saline and has higher nutrient inputs from rivers and higher concentrations of Chl-a (Brush et al., 2021; Zhang et al., 2021) compared to the waters of the Ionian-Aegean (Pavlidou et al., 2015; Varkitzi et al., 2018b; Varkitzi et al., 2020). As we move from the N. Adriatic to the Ionian and Aegean waters, this decreasing trophic gradient in pelagic habitats is well coupled with the responses and sensitivity of the benthic indices EEI-c, Cymoskew, and Mediskew in our dataset analyses.
In the N. Adriatic, the relationship between phytoplankton biomass and nutrients is evidenced by the process of oligotrophication observed from the beginning of this century onwards (Mozetič et al., 2010; Brush et al., 2021). Persistent low trophic conditions have been associated with a nutrient imbalance in the N. Adriatic, particularly due to severely reduced phosphate concentrations in the coastal waters of the N. Adriatic (Grilli et al., 2020). Nonetheless, there is recent evidence of a reversal in the trend toward increasing Chl-a at the southern edge of the N. Adriatic (Grilli et al., 2020). The decline in the trophic status of the Gulf of Trieste, the study area of the N. Adriatic, is reflected not only in the decrease in phytoplankton biomass but also in the increasing dominance of the nano- and picoplankton size classes (Flander-Putrle et al., 2022). However, while Chl-a concentrations in the Gulf of Trieste remain low nowadays, macroalgae have shown signs of deterioration (Orlando-Bonaca et al., 2021).
Despite the typical oligotrophic conditions in the waters of the Aegean and Ionian Seas, some coastal areas are hot spots of eutrophication, such as the well-studied Saronikos Gulf in the Aegean Sea. Thanks to the operation of a wastewater treatment plant for almost three decades, dissolved inorganic nitrogen and soluble reactive phosphorus loads in the waters of Saronikos Gulf have decreased, leading to an improvement in trophic status in the pelagic habitat (Pavlidou et al., 2019). Long-term data analyses have shown that the response of macroalgal communities to this improvement is the reduction in percent coverage of nitrophilous algae (Tsiamis et al., 2013). In addition, other studies indicate that macroalgae exhibit high divergence in status assessment relative to overall status resulting from different integration methods due to high spatial and temporal variability and localized pressures on rocky shores (Simboura et al., 2015).
The response of primary producers in the pelagic and benthic habitats of the study areas to eutrophication parameters is consistent with the findings of Elser et al. (2007), who conducted a large-scale meta-analysis of the response of primary producers to nutrient enrichment. On the global scale, phytoplankton responds most strongly to nutrient enrichment of marine environments, followed by hard-bottom macroalgae, while soft-bottom seagrasses show the weakest response to the enrichment (Elser et al., 2007). Our study shows that the coupling of pelagic and benthic primary producers across trophic gradients exhibits consistent patterns at the subregional scale.
Conclusions
Based on the outcomes of the present study, the responses of phytoplankton and macroalgal primary producers were coupled against all or most pelagic stressors/drivers. It is well known that phytoplankton is closely linked to water column turnovers. However, macroalgal communities may also include species that respond rapidly to nutrient inputs or other drivers in the pelagic habitat. Responses of P. oceanica traits to some of the pelagic drivers were coupled with the responses of phytoplankton, whereas the responses of C. nodosa traits were more loose. The relations between benthic primary producers and pelagic pressure indicators (nutrients) almost consistently indicate that it is difficult to demonstrate the link between these stressors and the benthic primary producers. The reasons for this may be multiple. Angiosperms do not respond directly to the availability of nutrients in the water column since nutrients released from the sediments are a luxurious pool for benthic vegetation (Duarte, 1991). On the other hand, with its shorter response time scales, phytoplankton is better associated with nutrient availability in the water column (Giovanardi et al., 2018). In addition, the documented oligotrophication of formerly nutrient-rich waters in some Mediterranean gulfs, such as the Gulf of Trieste in the N. Adriatic (Mozetič et al., 2010; Mozetič et al., 2012) and the Saronikos Gulf in the Aegean Sea (Tsiamis et al., 2013; Pavlidou et al., 2019), may indicate that the improvement in pelagic trophic status is slowly driving benthic vegetation status to higher levels in the absence of other pressures. Furthermore, benthic primary producers are sampled near the coast, but rarely are stressors assessed at the same site so close to the coast. Typically, stressors are measured at a distance and then generalized to the entire water body, as in this study. However, all seagrass indicators sampled were closely associated with light attenuation and phytoplankton biomass, confirming the key role of light availability in the distribution and status of benthic primary producers. In the context of EU directives (WFD and MSFD), phytoplankton, macroalgae and marine angiosperms are known to be designed as biological quality elements (BQEs) or indicators that are complementary. Therefore, they can be used in combination to assess the ecological and environmental status (ES) of different marine habitats and processes related to ecological deterioration, thus showing the effects of different pressures and not duplicating the assessment outcomes. Although phytoplankton and macroalgae react to increased nutrient inputs, the impact may be localized and highlighted by only one of the two BQEs (for example, in-shore and off-shore areas). Moreover, the response time is generally different, capturing the deterioration in different time windows, if needed.
Data Availability Statement
The raw data supporting the conclusions of this article will be made available by the authors, without undue reservation.
Author Contributions
VG: Conceptualization, Investigation, Data curation, Methodology, Formal analysis, Writing - original draft, Writing - review & editing. IV: Conceptualization, Investigation, Methodology, Formal analysis, Writing - original draft, Writing - review & editing. MO-B: Conceptualization, Investigation, Data curation, Writing - original draft, Writing - review & editing. KK: Satellite data analyses, Writing - original draft, Writing - review & editing. PM: Data curation, Writing - review & editing. PI-L: Data curation, Writing - review & editing. KT: Data curation, Writing - review & editing. JF: Conceptualization, Investigation, Data curation, Writing - original draft, Writing - review & editing. All authors contributed to the article and approved the submitted version.
Conflict of Interest
The authors declare that the research was conducted in the absence of any commercial or financial relationships that could be construed as a potential conflict of interest.
Publisher’s Note
All claims expressed in this article are solely those of the authors and do not necessarily represent those of their affiliated organizations, or those of the publisher, the editors and the reviewers. Any product that may be evaluated in this article, or claim that may be made by its manufacturer, is not guaranteed or endorsed by the publisher.
Acknowledgments
The authors gratefully acknowledge financial support from the Operational Programme “Competitiveness, Entrepreneurship and Innovation 2014-2020 (EPAnEK)” (MIS 5045792) “DRESSAGE”. VG would also like to thank Dr. Yiannis Issaris, and Dr. Maria Salomidi for their assistance during the fieldwork and data collection. IV and KK acknowledge support from the EU Horizon 2020 project “Marine-EO: Bridging Innovative Downstream Earth Observation and Copernicus enabled Services for Integrated maritime environment, surveillance and security” (Grant agreement ID: 730098). MOB, JF and PM gratefully acknowledge financial support from the Slovenian Research Agency (research core funding No. P1-0237), the Slovenian Environment Agency, and the Ministry of Environment and Spatial Planning of the Republic of Slovenia. In addition, MO-B, JF and PM would like to thank all colleagues involved in the fieldwork, laboratory work, and nutrient analyses. The authors would also like to thank the two anonymous reviewers for their constructive comments.
References
Abal E. G., Loneragan N., Bowen P., Perry C. J., Udy J. W., Dennison W. C. (1994). Physiological and Morphological Responses of the Seagrass Zostera Capricorni Aschers, to Light Intensity. J. Exp. Mar. Biol. Ecol. 178, 113–129. doi: 10.1016/0022-0981(94)90228-3
Adams S. M., Greeley M. S. (2000). Ecotoxicological Indicators of Water Quality: Using Multi-Response Indicators to Assess the Heath of Aquatic Ecosystems. Water Air Soil Pollut. 123, 103–115. doi: 10.1023/A:1005217622959
Ballesteros E., Torras X., Pinedo S., García M., Mangialajo L., de Torres M. (2007). A New Methodology Based on Littoral Community Cartography Dominated by Macroalgae for the Implementation of the European Water Framework Directive. Mar. Pollution Bull. 55, 172–180. doi: 10.1016/j.marpolbul.2006.08.038
Brady D. C., Testa J. M., Di Toro D. M., Boynton W. R., Kemp W. M. (2013). Sediment Flux Modeling: Calibration and Application for Coastal Systems. Estuar Coast Shelf Sci. 117, 107–124. doi: 10.1016/j.ecss.2012.11.003
Brush M. J., Mozetič P., Francé J., Bernardi Aubry F., Djakovac T., Faganeli J., et al. (2021). “Phytoplankton Dynamics in a Changing Environment,” in Coastal Ecosystems in Transition: A Comparative Analysis of the Northern Adriatic and Chesapeake Bay. Eds. Malone T. C., Malej A., Faganeli J. (Hoboken: American Geophysical Union), 49–74. doi: 10.1002/9781119543626.ch4
Cloern J. E., Foster S. Q., Kleckner A. E. (2014). Phytoplankton Primary Production in the World's Estuarine-Coastal Ecosystems. Biogeosciences 11, 2477–2501. doi: 10.5194/bg-11-2477-2014
CSTT (1997). “Comprehensive Studies for the Purposes of Article 6 of Directive 91/271 EEC),” in The Urban Waste Water Treatment Directive (Edinburgh: Forth River Purification Board).
Devlin M., Besyt M., Haynes D. (2007). Implementation of the Water Framework Directive in European Marine Waters. Mar. Pollut. Bull. 55 (1–6), 1–2. doi: 10.1016/j.marpolbul.2006.09.020
Dimitriou P. D., Papageorgiou N., Arvanitidis C., Assimakopoulou G., Pagou K., Papadopoulou K. N., et al (2015). One Step Forward: Benthic Pelagic Coupling and Indicators for Environmental Status. PLoS ONE 10, e0141071. doi: 10.1371/journal.pone.0141071
Domingues R. B., Barbosa A., Galvão H. (2008). Constraints on the Use of Phytoplankton as a Biological Quality Element Within the Water Framework Directive in Portuguese Waters. Mar. Pollut. Bull. 56 (8), 1389–95. doi: 10.1016/j.marpolbul.2008.05.006.
Duarte C. M. (1991). Seagrass Depth Limits. Aquat Bot. 40, 363–377. doi: 10.1016/0304-3770(91)90081-F
Elser J. J., Bracken M. E. S., Cleland E. E., Gruner D. S., Harpole W. S., Hillebrand H., et al. (2007). Global Analysis of Nitrogen and Phosphorus Limitation of Primary Producers in Freshwater, Marine and Terrestrial Ecosystems. Ecol. Lett. 10, 1135–1142. doi: 10.1111/j.1461-0248.2007.01113.x
Fernández-Torquemada Y., Díaz-Valdés M., Colilla F., Luna B., Sánchez-Lizaso J. L., Ramos-Esplá A. A. (2008). Descriptors From Posidonia Oceanica (L.) Delile Meadows in Coastal Waters of Valencia, Spain, in the Context of the EU Water Framework Directive. ICES J. Mar. Sci. 65 (8), 1492–1497. doi: 10.1093/icesjms/fsn146
Flander-Putrle V., Francé J., Mozetič P. (2022). Phytoplankton Pigments Reveal Size Structure and Interannual Variability of the Coastal Phytoplankton Community (Adriatic Sea). Water 14, 23. doi: 10.3390/w14010023
Francé J., Varkitzi I., Stanca E., Cozzoli F., Skejić S., Ungaro N., et al. (2021). Large-Scale Testing of Phytoplankton Diversity Indices for Environmental Assessment in Mediterranean Sub-Regions (Adriatic, Ionian and Aegean Seas). Ecol. Indic. 126, 107630. doi: 10.1016/j.ecolind.2021.107630
Gerakaris V., Panayotidis P., Vizzini S., Nicolaidou A. (2017). Effectiveness of Posidonia Oceanica Biotic Indices for Assessing the Ecological Status of Coastal Waters in the Saronikos Gulf (Aegean Sea, Eastern Mediterranean). Mediterranean Mar. Sci. 18, 161–178. doi: 10.12681/mms.1893
Gerakaris V., Papathanasiou V., Salomidi M., Issaris Y., Panayotidis P. (2021). Spatial Patterns of Posidonia Oceanica Structural and Functional Features in the Eastern Mediterranean (Aegean and E. Ionian Seas) in Relation to Large-Scale Environmental Factors. Marine Environ. Res. 165, 1–13. doi: 10.1016/j.marenvres.2020.105222
Giovanardi F., Francé J., Mozetič P., Precali R. (2018). ). Development of Ecological Classification Criteria for the Biological Quality Element Phytoplankton for Adriatic and Tyrrhenian Coastal Waters by Means of Chlorophyll a, (2000/60/EC WFD). Ecol. Indic. 93, 316–332. doi: 10.1016/j.ecolind.2018.05.015
Gobert S., Sartoretto S., Rico-Raimondino V., Andral B., Chery A., Lejeune P., et al. (2009). Assessment of the Ecological Status of Mediterranean French Coastal Waters as Required by the Water Framework Directive Using the Posidonia Oceanica Rapid Easy Index: PREI. Mar. Pollut. Bull. 58 (11), 1727–1733. doi: 10.1016/j.marpolbul.2009.06.012
Goldberg D. (1996). Competitive Ability: Definitions, Contingency and Correlated Traits. Philos. Trans. Biol. Sci. 351, 1377–1385.
Graf G. (1992). Benthic-Pelagic Coupling: A Benthic View. Oceanogr. Mar. Biol. Annu. Rev. Vol 30, 149–190.
Grangeré K., Lefebvre S., Blin J. L. (2012). Spatial and Temporal Dynamics of Biotic and Abiotic Features of Temperate Coastal Ecosystems as Revealed by a Combination of Ecological Indicators. Estuar Coast Shelf Sci. 108, 109–118. doi: 10.1016/j.ecss.2012.02.020
Grasshoff K., Ehrhardt M., Kremling K. (1999). Methods of Seawater Analysis, Third, Completely Revised and Extended Edition. Ed. Weinheim W.-V.. doi: 10.1002/9783527613984
Greve T. M., Binzer T.. (2004). Which Factors Regulate Seagrass Growth and Distribution. In: The Four European Seagrass Species Borum J., Duarte C. M., Krause-Jensen D., Greve T. M. Eds., European Seagrasses: An Introduction to Monitoring and Management, EU Project Monitoring and Managing of European Seagrasses (M&MS), 88.
Grilli F., Accoroni S., Acri F., Bernardi Aubry F., Bergami C., Cabrini M., et al. (2020). Seasonal and Interannual Trends of Oceanographic Parameters Over 40 Years in the Northern Adriatic Sea in Relation to Nutrient Loadings Using the EMODnet Chemistry Data Portal. Water 12, 2280. doi: 10.3390/w12082280
Håkanson L., Eklund J. M. (2010). Relationships Between Chlorophyll, Salinity, Phosphorus, and Nitrogen in Lakes and Marine Areas. J Coast Res, 412–23. doi: 10.2112/08-1121.1
Hargrave B. T. (1973). Coupling Carbon Flow Though Some Pelagic and Benthic Communities. J. Fish Res. Board Can. 30, 1317–1326. doi: 10.1139/f73-212
Harlin M. M. (1995). “Changes in Major Plant Groups Following Nutrient Enrich- Ment,” in Eutrophic Shallow Estuaries and Lagoons. Ed. MC Comb A. J. (Murdoch, Australia: Institute for Environmental Science, Murdoch University, CRC Press), p.173–p.187.
Hemminga M. A., Duarte C. M. (2000). Seagrass Ecology (Cambridge: Cambridge University Press). doi: 10.1017/CBO9780511525551
Holm-Hansen O., Lorenzen C. J., Holmes R. W., Strickland J. D. H. (1965). Fluorometric Determination of Chlorophyll. J. Cons. Perm. Int. Expl. Mer. 30, 3–15. doi: 10.1093/icesjms/30.1.3
Ignatiades L., Gotsis-Skretas O. (2010). A Review on Toxic and Harmful Algae in Greek Coastal Waters (E. Mediterranean Sea). Toxins 2 (5), 1019–1037. doi: 10.3390/toxins2051019
Ignatiades L., Gotsis-Skretas O., Pagou K., Krasakopoulou E. (2009). Diversification of Phytoplankton Community Structure and Related Parameters Along a Large-Scale Longitudinal East–West Transect of the Mediterranean Sea. J. Plankton Res. 31, 411–428. doi: 10.1093/plankt/fbn124
Kenworthy W. J., Fonseca M. S. (1996). Light Requirements of seagrassesHalodule Wrightii and Syringodium Filiforme Derived From the Relationship Between Diffuse Light Attenuation and Maximum Depth Distribution. Estuaries 19, 740–750.
Krause-Jensen D., Markager S., Dalsgaard T. (2012). Benthic and Pelagic Primary Production in Different Nutrient Regimes. Estuaries Coasts 35, 527–545. doi: 10.1007/s12237-011-9443-1
Lee K.-S., Park S. R., Kim Y. K. (2007). Effects of Irradiance, Temperature, and Nutrients on Growth Dynamics of Seagrasses: A Review. J. Exp. Mar. Biol. Ecol. 350 (1-2), 144–175. doi: 10.1016/j.jembe.2007.06.016
Magliozzi C., Druon J. N., Palialexis A., Aguzzi L., Alexande B., Antoniadis K., et al. (2021). Pelagic Habitats Under the MSFD D1: Scientific Advice of Policy Relevance (Luxembourg: EUR 30671 EN, Publications Office of the European Union), ISBN: ISBN 978-92-76-35958-6. doi: 10.2760/081368
Magliozzi C., Druon J.-N., Palialexis A., Artigas L. F., Boicenco L., González-Quirós R., et al. (2021). Pelagic Habitats Under MSFD D1: Current Approaches and Priorities (Luxembourg: EUR 30619 ENPublications Office of the European Union), ISBN: ISBN 978-92-76-30988-8. doi: 10.2760/942589
Marbà N., Krause-Jensen D., Alcoverro T., Birk S., Pedersen A., Neto J. M., et al. (2013). Diversity of European Seagrass Indicators: Patterns Within and Across Regions. Hydrobiologia 704 (1), 265–278. doi: 10.1007/s10750-012-1403-7
Martínez-Crego B., Alcoverro T., Romero J. (2010). Biotic Indices for Assessing the Status of Coastal Waters: A Review of Strengths and Weaknesses. J. Environ. Monitoring 12 (5), 1013–1028. doi: 10.1039/b920937a
McGlathery K. J., Sundback K., Anderson I. C. (2007). Eutrophication in Shallow Coastal Bays and Lagoons: The Role of Plants in the Coastal Filter. Mar. Ecol. Prog. Ser. 348, 1–18. doi: 10.3354/meps07132
MED-GIG (2011). “WFD Intercalibration Phase 2: Milestone 6 Report,” in Coastal Waters, Mediterranean Sea GIG, Macroalgae, 25 pp.
Mozetič P., France J., Kogovšek T., Talaber I., Malej A. (2012). Plankton Trends and Community Changes in a Coastal Sea (Northern Adriatic): Bottom-Up vs. Top-Down Control in Relation to Environmental Drivers. Estuarine Coastal Shelf Sci. 115, 138–148. doi: 10.1016/j.ecss.2012.02.009
Mozetič P., Solidoro C., Cossarini G., Socal G., Precali R., France J., et al. (2010). Recent Trends Towards Oligotrophication of the Northern Adriatic: Evidence From Chlorophyll a Time Series. Estuaries Coasts 33, 362–375. doi: 10.1007/s12237-009-9191-7
Mvungi E. F., Mamboya F. A. (2012). Photosynthetic Performance, Epiphyte Biomass and Nutrient Content of Two Seagrass Species in Two Areas With Different Level of Nutrients Along the Dar Es Salaam Coast. Afr. J. Marine Sci. 34 (3), 323–330. doi: 10.2989/1814232X.2012.709957
Orfanidis S., Panayotidis P., Stamatis N. (2001). Ecological Evaluation of Transitional and Coastal Waters: A Marine Benthic Macrophytes-Based Model. Mediterranean Mar. Sci. 2 (2), 45–65. doi: 10.12681/mms.266
Orfanidis S., Panayotidis P., Ugland K. I. (2011). Ecological Evaluation Index Continuous Formula (EEI-C) Application: A Step Forward for Functional Groups, the Formula and Reference Condition Values. Mediterr. Mar. Sci. 12 (1), 199–231. doi: 10.12681/mms.60
Orfanidis S., Papathanasiou V., Gounaris S. (2007). Body Size Descriptor of Cymodocea Nodosa Indicates Anthropogenic Stress in Coastal Ecosystem. Transitional Waters Bull. 2, 1–7. doi: 10.1285/i1825229Xv1n2p1
Orfanidis S., Papathanasiou V., Gounaris S., Theodosiou T. (2010). Size Distribution Approaches for Monitoring and Conservation of Coastal Cymodocea Habitats. Aquat. Conservation: Marine Freshwater Ecosystems 20, 177–188. doi: 10.1002/aqc.1069
Orfanidis S., Papathanasiou V., Mittas N., Theodosiou T., Ramfos A., Tsioli S., et al. (2020). Further Improvement, Validation, and Application of CymoSkew Biotic Index for the Ecological Status Assessment of the Greek Coastal and Transitional Waters. Ecol. Indic. 118, 106727. doi: 10.1016/j.ecolind.2020.106727
Orlando-Bonaca M., Francé J., Mavrič B., Grego M., Lipej L., Flander-Putrle V., et al. (2015). A New Index (MediSkew) for the Assessment of the Cymodocea Nodosa (Ucria) Ascherson Meadow's Status. Marine Environ. Res. 110, 132–141. doi: 10.1016/j.marenvres.2015.08.009
Orlando-Bonaca M., Francé J., Mavrič B., Lipej L. (2019). Impact of the Port of Koper on Cymodocea Nodosa Meadow. Annales Ser. Hist. Naturalis 29 (2), 187–194. doi: 10.19233/ASHN.2019.18
Orlando-Bonaca M., Lipej L., Francé J. (2016). The Most Suitable Time and Depth to Sample Cymodocea Nodosa (Ucria) Ascherson Meadows in the Shallow Coastal Area. Experiences northern Adriatic Sea Acta Adriatica 57 (2), 251–262.
Orlando-Bonaca M., Lipej L., Orfanidis S. (2008). Benthic Macrophytes as a Tool for Delineating, Monitoring and Assessing Ecological Status: The Case of Slovenian Coastal Waters. Mar. Pollut. Bull. 56 (4), 666–676. doi: 10.1016/j.marpolbul.2007.12.018
Orlando-Bonaca M., Pitacco V., Lipej L. (2021). Loss of Canopy-Forming Algal Richness and Coverage in the Northern Adriatic Sea. Ecol. Indic. 125, 107501. doi: 10.1016/j.ecolind.2021.107501
Orlando-Bonaca M., Rotter A. (2018). Any Signs of Replacement of Canopy-Forming Algae by Turf-Forming Algae in the Northern Adriatic Sea? Ecol. Indic. 87, 272–284. doi: 10.1016/j.ecolind.2017.12.059
Pagou K. (2005). “Eutrophication in Hellenic Coastal Areas,” in State of the Hellenic Marine Environment. Inst. Oceanography. Eds. Papathanassiou E., Zenetos A. (Athens: HCMR publ.), 311–317.
Papathanasiou V., Orfanidis S. (2018). Anthropogenic Eutrophication Affects the Body Size of Cymodocea Nodosa in the North Aegean Sea: A Long-Term, Scale-Based Approach. Mar. Pollut. Bull. 134, 38–48. doi: 10.1016/j.marpolbul.2017.12.009
Pavlidou A., Simboura N., Pagou K., Assimakopoulou G., Gerakaris V., Hatzianestis I., et al. (2019). Using a Holistic Ecosystem-Integrated Approach to Assess the Environmental Status of Saronikos Gulf, Eastern Mediterranean. Ecol. Indic 96, 336–350. doi: 10.1016/j.ecolind.2018.09.007
Pavlidou A., Simboura N., Rousselaki E., Tsapakis M., Pagou K., Drakopoulou P., et al. (2015). Methods of Eutrophication Assessment in the Context of the Water Framework Directive: Examples From the Eastern Mediterranean Coastal Areas. Cont Shelf Res. 108, 156–168. doi: 10.1016/j.csr.2015.05.013
Pawlowski J., Kelly-Quinn M., Altermatt F., Apothéloz-Perret-Gentil L., Beja P., Boggero A., et al. (2018). The Future of Biotic Indices in the Ecogenomic Era: Integrating (E) DNA Metabarcoding in Biological Assessment of Aquatic Ecosystems. Sci. Total Environ. 637, 1295–1310. doi: 10.1016/j.scitotenv.2018.05.002
R Core Team (2021). R: A Language and Environment for Statistical Computing. Vienna, Austria: R Foundation for Statistical Computing. URL https://www.R-project.org/
Ralph P. J., Durako M. J., Enríquez S., Collier C. J., Doblin M. A. (2007). Impact of Light Limitation on Seagrasses. J. Exp. Marine Biol. Ecol. 350 (1-2), 176–193. doi: 10.1016/j.jembe.2007.06.017
Rombouts I., Simon N., Aubert A., Cariou T., Feunteun E., Guérin L., et al (2019). Changes in Marine Phytoplankton Diversity: Assessment Under the Marine Strategy Framework Directive. Ecol. Indic. 102, 265–77. doi: 10.1016/j.ecolind.2019.02.009
Romero J., Martinez-Crego B., Alcoverro T., Perez M. (2007a). A Multivariate Index Based on the Seagrass Podidonia Oceanica (POMI) to Assess Ecological Status of Coastal Waters Under the Water Framework Directive (WFD). Marine Pollution Bull. 55, 196–204. doi: 10.1016/j.marpolbul.2006.08.032
Romero J., Martinez-Crego B., Alcoverro T., Perez M. (2007b). Corrigendum of: "A Multivariate Index Based on the Seagrass Podidonia Oceanica (POMI) to Assess Ecological Status of Coastal Waters Under the Water Framework Directive (WFD) in Marine Pollution Bulletin 55, 196–204". Marine Pollution Bull. 54, 631. doi: 10.1016/j.marpolbul.2007.02.008
Sand-Jensen K., Borum J. (1991). Interactions Among Phytoplankton, Periphyton, and Macrophytes in Temperate Freshwaters and Estuaries. Aquatic Botany 41 (1–3), 137–175. doi: 10.1016/0304-3770(91)90042-4
Schramm W., Nienhuis P. H. (1996). “Marine Benthic Vegetation,” in Recent Changes and the Effects of Eutrophica- Tion (New York: Springer), 470 pp.
Simboura N., Tsapakis M., Pavlidou A., Assimakopoulou G., Pagou K., Kontoyiannis H., et al. (2015). Assessment of the Environmental Status in Hellenic Coastal Waters (Eastern Mediterranean): From the Water Framework Directive to the Marine Strategy Water Framework Directive. Mediterranean Mar. Sci. 16 (1), 46–64. doi: 10.12681/MMS.960
Simboura N., Pavlidou A., Bald J., Tsapakis M., Pagou K., Zeri C., et al. (2016). Response of Ecological Indices to Nutrient and Chemical Contaminant Stress Factors in Eastern Mediterranean Coastal Waters. Ecol. Ind. 70, 89–105. doi: 10.1016/j.ecolind.2016.05.018
Tett P., Carreira C., Mills D. K., Van Leeuwen S., Foden J., Bresnan E., et al. (2008). Use of a Phytoplankton Community Index to Assess the Health of Coastal Waters. ICES J. Mar. Sci. 65, 1475–1482. doi: 10.1093/icesjms/fsn161
Touchette B. W., Burkholder J. M. (2000). Overview of the Physiological Ecology of Carbon Metabolism in Seagrasses. J. Exp. Marine Biol. Ecol. 250, 169–205. doi: 10.1016/S0022-0981(00)00196-9
Tsiamis K., Balanika K., Kleinteich J., Salomidi M., Pavlidou A., Küpper F. C., et al. (2013). Macroalgal Community Response to Re-Oligotrophication: A Case Study From Saronikos Gulf (Eastern Mediterranean, Greece). Mar. Ecol. Prog. Ser. 472, 73–85. doi: 10.3354/meps10060
Tweddle J. F., Gubbins M., Scott B. E. (2018). Should Phytoplankton be a Key Consideration for Marine Management? Mar. Policy 97, 1–9. doi: 10.1016/j.marpol.2018.08.026
Tyerman S. D. (1989). “Solute and Water Relations of Seagrasses,” in Biology of Seagrasses: A Treatise on the Biology of Seagrasses With Special Reference to the Australian Region. Ed. Larkum A. W. D. (Amsterdam, The Netherlands: Elsevier), 723–759.
UNEP/MAP. (2017). Integrated Monitoring and Assessment Programme of theMediterranean Sea and Coast and Related Assessment Criteria. United NationsEnvironment Programme / Mediterranean Action Plan, Athens, Greece.
Valiela I., Foreman K., LaMontagne M., Hersh D., Costa J., Peckol P., et al. (1992). Couplings of Watersheds and Coastal Waters: Sources and Consequences of Nutrient Enrichment in Waquoit Bay, Massachusetts. Estuaries 15, 443–457. doi: 10.2307/1352389
Vaquer-Sunyer R., Duarte C. M. (2008). Thresholds of Hypoxia for Marine Biodiversity. Proc. Natl. Acad. Sci. U.S.A 105, 15452–15457. doi: 10.1073/pnas.0803833105
Varkitzi I., Francé J., Basset A., Cozzoli F., Stanca E., Zervoudaki S., et al. (2018a). Pelagic Habitats in the Mediterranean Sea: A Review of Good Environmental Status (GES) Determination for Plankton Components and Identification of Gaps and Priority Needs to Improve Coherence for the MSFD Implementation. Ecol. Ind. 95, 203–218. doi: 10.1016/j.ecolind.2018.07.036
Varkitzi I., Markogianni V., Pantazi M., Pagou K., Pavlidou A., Dimitriou E. (2018b). Effect of River Inputs on Environmental Status and Potentially Harmful Phytoplankton in a Coastal Area of Eastern Mediterranean (Maliakos Gulf, Greece). Mediterranean Mar. Sci. 19 (2), 326–343. doi: 10.12681/mms.14591
Varkitzi I., Pagou K., Granéli E., Hatzianestis I., Krasakopoulou E., Pavlidou A. (2013). “Spatio-Temporal Distribution of Dinophysis Spp. In Relation to Organic Matter Availability and Other Parameters in Thermaikos Gulf, Greece (Eastern Mediterranean),” in Proceedings of the 14th International Conference on Harmful Algae, International Society for the Study of Harmful Algae and Intergovernmental Oceanographic Commission of UNESCO, 1-5 November 2013 (Greece: Hersonissos-Crete), 269.
Varkitzi I., Psarra S., Assimakopoulou G., Pavlidou A., Krasakopoulou E., Velaoras D., et al. (2020). Phytoplankton Dynamics and Bloom Formation in the Oligotrophic Eastern Mediterranean: Field Studies in the Aegean, Levantine and Ionian Seas. Deep Sea Res. Part II 171, 104662. doi: 10.1016/j.dsr2.2019.104662
Zhang Q., Cozzi S., Palinkas S., Giani M. (2021). “Recent Status and Long-Term Trends in Freshwater Discharge and Nutrient Inputs,” in Coastal Ecosystems in Transition: A Comparative Analysis of the Northern Adriatic and Chesapeake Bay. Eds. Malone T. C., Malej A., Faganeli J. (Hoboken: American Geophysical Union), 7–20. doi: 10.1002/9781119543626.ch2
Keywords: macroalgae, seagrasses, phytoplankton, eutrophication, Mediterranean Sea
Citation: Gerakaris V, Varkitzi I, Orlando-Bonaca M, Kikaki K, Mozetič P, Lardi P-I, Tsiamis K and Francé J (2022) Benthic-Pelagic Coupling of Marine Primary Producers Under Different Natural and Human-Induced Pressures’ Regimes. Front. Mar. Sci. 9:909927. doi: 10.3389/fmars.2022.909927
Received: 31 March 2022; Accepted: 10 May 2022;
Published: 13 June 2022.
Edited by:
Christian GRENZ, UMR7294, Institut Méditerranéen d’océanographie (MIO), FranceReviewed by:
Pierre Boissery, Agence de l’eau Rhône Méditerranée Corse, FranceMaria Flavia Gravina, University of Rome “Tor Vergata”, Italy
Copyright © 2022 Gerakaris, Varkitzi, Orlando-Bonaca, Kikaki, Mozetič, Lardi, Tsiamis and Francé. This is an open-access article distributed under the terms of the Creative Commons Attribution License (CC BY). The use, distribution or reproduction in other forums is permitted, provided the original author(s) and the copyright owner(s) are credited and that the original publication in this journal is cited, in accordance with accepted academic practice. No use, distribution or reproduction is permitted which does not comply with these terms.
*Correspondence: Vasilis Gerakaris, vgerakaris@hcmr.gr