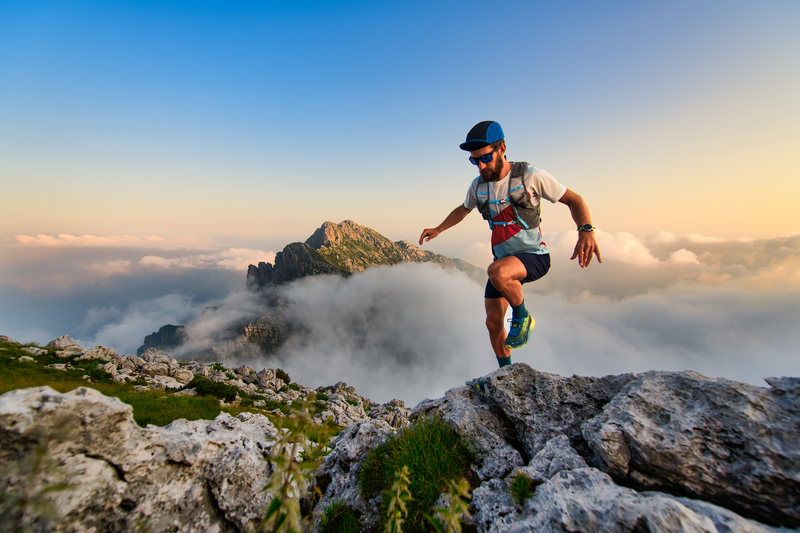
94% of researchers rate our articles as excellent or good
Learn more about the work of our research integrity team to safeguard the quality of each article we publish.
Find out more
ORIGINAL RESEARCH article
Front. Mar. Sci. , 09 May 2022
Sec. Marine Conservation and Sustainability
Volume 9 - 2022 | https://doi.org/10.3389/fmars.2022.877301
This article is part of the Research Topic Remote Sensing for Coastal Sustainability View all 23 articles
It is not deep enough that the theoretical and empirical analysis of economic drivers on the urban heat island (UHI) effect have been researched. Consequently, the study of economic drivers of the UHI effect is not only conducive to the governance of the UHI problem but also conducive to deepening the study of related issues in the economic field. The Yangtze River Delta (YRD) region of China has developed economic status and the special geography of estuaries and coasts. Therefore, 26 central cities in the YRD region are selected as research samples, and the period from 2003 to 2017 is taken as the observation period. The Spatio-temporal variability of UHI intensity caused by industrialization and urbanization is analyzed by using MODIS land surface temperature (LST) data and related yearbook data. The conclusions are as follows:(1) The UHI intensity of cities in the YRD region is significant and fluctuates to a certain extent. (2) Empirical analysis shows that the agglomeration of single economic factors in the process of industrialization and urbanization does correlate with the UHI intensity in the YRD region, but the correlation may show a downward trend due to the influence of exogenous factors suc0h as physical geography. (3) In terms of economic drivers, the combination of multiple factors can produce a greater UHI intensity. The model of their impacts on UHI intensity is increasingly-changed, which is from a model of Fixed Asset Investment and the Motor Vehicle Population as dominant factors to a model of multiple overlapping economic factors, and the latter has a greater impact.
Since 1833, when Howard observed that the temperature in downtown London was higher than that in neighboring rural areas and proposed the concept of the ‘Urban Heat Island’ and the ‘hot-island Effect’ (Howard, 1833), human beings have been studying the Urban Heat Island (UHI) effect for nearly two centuries. As a special urban climate, the UHI effect not only has a negative impact on urban objectives such as urban air quality, building energy consumption, etc. (Swamy et al., 2017; Akkose et al., 2021) but also causes concern and research relating to the health problems of urban residents (Lin et al., 2009; Michelozzi et al., 2009; Lee et al., 2017). Therefore, a large number of studies have been devoted to exploring the influencing factors and driving mechanism of the UHI effect, namely the motivation research, which aims to control the intensity of the UHI by analyzing the main causes of the UHI effect.
These studies can be divided into heat island factors category and cold island factors category according to their results, while they can also be divided into physical geography and economic society categories according to their attributes. Among them, the studies on the physical geographical circle are basically about cold island factors category, and the motivations involved include mountainous area (Estoque and Murayama, 2016), residual natural mountain range (Chen et al., 2021), river (Park et al., 2019), distance from water (Cheval et al., 2020), and atmospheric circulation (Zak et al., 2020). To sum up, existing studies have fully affirmed the differential impacts of urban’s heterogeneous physical geographical factors on UHI. In the field of society and economy, a large amount of anthropogenic heat emission caused by human social and economic activities, especially in the era of industrialization and urbanization, has increasingly aroused research attention. There is not only the cold island factors category, such as a park (green space) (Salerno et al., 2018; Peng et al., 2020) or the configuration of the landscape (Sun et al., 2018); more common are heat island factors, which include city size (Imhoff et al., 2010), construction rate, permeability ratio, average height and average surface area of buildings (Badaro-Saliba et al., 2021), building density (Gabriel and Endlicher, 2011), building environment (Susca and Pomponi, 2020), anthropogenic heat emission (automobile exhaust, etc.) (Sun et al., 2019a), urban polycentric and industrial Layout (Yue et al., 2019), population and human factors (Dewan et al., 2021), population, GDP and fixed asset investment, etc. (Feyisa et al., 2014; Li et al., 2020).
With the deepening of industrialization and urbanization, the impact of these factors may not only deepen but also become more and more complex in their various ways of influence (Li et al., 2020; Feng et al., 2021) These studies undoubtedly greatly enrich people’s understanding of the causes of the urban heat island effect. However, through the review of the above studies on the economic drivers of the UHI effect, we found that there are at least two knowledge gaps in present studies: i) For UHI, there are a lot of studies on economic drivers, and most of these studies select several factors to independently explore their impacts on UHI. However, economic activities have their own scientific laws. How should we distinguish and define different economic drivers and extract key influencing factors to measure their impact on UHI with the help of relevant economic theories? There seems to be not enough consensus in the current research. ii) On this basis, different cities have different basic conditions in terms of physical geographical factors and economic factors. How should we separately measure the different impacts of economic drivers on UHI of a certain city or certain type of cities? Moreover, from the perspective of empirical analysis, how should we clarify the relationship between different economic factors and analyze their mode of action on UHI through constructing an appropriate analysis model? These questions still need to be further explored.
To try to answer these questions, the rest of this paper will be a study based on the existing theory of urbanization and industrialization, summarize them as the economic motive mechanisms of the impact of the UHI effect, and make use of the relevant data of YRD cities to carry on the empirical analysis, summed up the Spatio-temporal characteristics of economic drivers, and put forward the corresponding policy implications. The marginal contribution of this paper is as follows: i) try to use relevant economic theories to tease out the main economic influencing factors; ii) select urban agglomerations with the significant theoretical influence of economic drivers and use appropriate methods to empirically test their influence.
According to the literature review in the introduction, urbanization and industrialization are the two main economic drivers of the UHI effect. Urbanization is a concept with rich connotations. In the field of economics, there has not been a unified definition that can be recognized by the global economic circle. As a branch of economics, development economics began to probe into the economic and social development of developing countries including China since the 1940s, including the theme of urbanization. Zhao (2013) believes that the essence of the modern economy is modernization, industrialization, and urbanization. Zhang and Yang (1999) studied China’s industrialization and modernization, argued that “urbanization originated from industrialization, and subsequent population in urban agglomeration is caused by industrialization”, so urbanization can be measured by the proportion of the urban population.
The urbanization of land is another important characteristic of urbanization. It refers to the process that the land use attribute changes from agricultural land to urban construction land and the land property right attribute changes from rural collective land to state-owned land, which is accompanied by the change of the natural attributes and social-economic attributes of land. Since economic growth can rapidly increase the price of urban land, the rapid growth of land price in the short term contributes to the change of land use types, so economic development is the fundamental motivation of land urbanization (Yu, 2017). Urbanization changes urban land use types, and the latter changes surface albedo, absorption rate, and emissivity, which are important reasons for the UHI effect (Zhao et al., 2014). Further studies show that in the process of urbanization, the expansion of non-vegetation land is a continuous process, which leads to the fragmentation of vegetation land space. Affected by the edge effect, the continuous expansion of the former leads to the enhancement of the UHI effect, while the fragmentation of the latter leads to the weakening of the cold island effect (Lin et al., 2018). In addition, the process of urbanization is closely related to economic influencing factors such as investment and consumption (Zhao, 2013), which can be regarded as common elements in the process of urbanization.
On the other hand, there are abundant theoretical and empirical studies on industrialization. In most developed countries, industrialization is done by the private sector through the market, and the process is relatively slow. For developing countries, industrialization developed by the government or industrialization jointly developed by industry and the government is more common, so its process is more abrupt (Li, 2013). However, the process of industrialization is also closely related to the UHI effect due to population migration, production and consumption patterns, and changes in land-use patterns.
In all, urbanization is the inevitable result of industrialization, and industrialization is the necessary condition and driving factor of urbanization. In other words, industrialization is the economic essence of urbanization, and urbanization is the spatial expression of industrialization (Zhao, 2013). To sum up, urbanization and industrialization have an interrelated and inseparable relationship, and both include factors that affect the UHI effect.
In addition, according to regional economic theory, agglomeration formed by multiple industries in urban space is called “Urbanization economies”, which breaks through the external economies of scale of single industry agglomeration and brings benefits to all enterprises in the city (Lemelin et al., 2016). In other words, the essence of urbanization is the spatial agglomeration of various economic elements. Agglomeration is also the requirement of industrialization. Weber, the founder of industrial location theory, pointed out that agglomeration helps industrial enterprises reduce costs. Agglomeration brings the proximity effect, division of labor effect, structure effect, scale effect, and “gravitational field” effect, which not only improves economic benefits but also strengthens agglomeration itself and becomes an irreversible trend (Lemelin et al., 2016). All in all, agglomeration is the fundamental characteristic of industrialization and urbanization, and the objects of agglomeration not only include population, buildings, motor vehicles, etc., but also the economic activities derived therefrom. For example, agglomeration leads to the expansion of building land in a spatial continuous way, and building land contributes greatly to the UHI effect among various land-use types (Tian et al., 2021). Accordingly, production, consumption, service, and other economic activities also come into being with the emergence of buildings. Consequently, this paper considers that various economic influencing factors and activity intensity of industrialization and urbanization agglomeration are important drivers of the UHI effect at the socioeconomic level.
Since the essential feature of industrialization and urbanization is the agglomeration of economic influencing factors and activities, on the one hand, it increases the density of various economic influencing factors (population, buildings, industrial enterprises, and motor vehicles) in cities, and on the other hand, it increases the intensity of various economic activities (production, transportation, storage, and consumption, which constitutes GDP). Together, these economic influencing factors and activities promote the UHI effect (Figure 1). Therefore, in this paper, the Gross Domestic Production (GDP), the Permanent Urban Population (POP), the Fixed Asset Investment (FIX), the Industrial Output Value (IND), and the Motor Vehicle Population (MOT) are selected as the single influencing factors of the economic drivers of the UHI effect. Although most of its effect has been confirmed by existing studies from the perspective of a single factor. However, due to the agglomeration of economic influencing factors and activities in the urban area, they are concentrated and superimposed on each other in the urban space, and there are fluctuations and periodic changes, which may bring Spatio-temporal effects on the UHI effect.
China is the largest developing country in the world. Since the launch of Reform and Opening-up in 1978, the process of urbanization in China has accelerated. Along with this process, the UHI effect and its influence have become increasingly prominent in many Chinese cities. The Yangtze River Delta (YRD) is one of the regions with the highest degree of urbanization after the Reform and Opening-up in China and is also the location of major cities with significant UHI effect. Located on estuary and coast, it is vulnerable to climate change, so it has attracted a large number of similar studies (Shen et al., 2013; Li et al., 2020).
Within coastal countries and regions, the coastal zone is highly concentrated with population, industry, urban, economic activities. Being the meeting point of the two natural geographical units of the sea and land, it is vulnerable to sea-level rise, storm surge, saltwater intrusion, coastal erosion, wetland degradation, and coastal marine disasters such as the influence of ecological events. Especially the estuary of the coast is most vulnerable because it is located in the land, river, and confluence of the sea, being a strong human activity and nature-artificial compound ecological system, it is complex, sensitive with superposition of these two factors (Liu, 2014).
The YRD is located in the lower reaches of the Yangtze River and the middle of the Coastal Zone of China. Bordering the Yellow Sea and the East China Sea, it is located at the junction of the river and the sea, which is rich with marine resources. They constitute a typical estuary city and estuary coastal areas. Due to the simultaneous effects of artificial construction and sediment deposition and other natural factors, the coastline of this region is still in a state of dynamic change (Wu et al., 2022). Therefore, it is not only one of the most economically developed regions in China, but also one of the urbanization regions with the most complex human-land relationship and the most fragile ecological system. By the end of 2019, the YRD had a population of 227 million and an area of 358,000 square kilometers. In 2020, the GDP of the YRD reach 24.5 trillion CNY. The urbanization rate of permanent residents exceeded 60%, making it one of the top five urban clusters1 They include the Yangtze River Delta, the Pearl River Delta, the Beijing-Tianjin-Hebei Region, the Middle Reaches of the Yangtze River, and the Chengdu-Chongqing urban agglomeration.
Remote sensing data have been widely used to reveal large-scale changes in land surface temperature in China, which are highly correlated with atmospheric temperature observations (Li et al., 2020). Land surface temperature (LST) derived from MODIS LST datasets As a typical representative of satellite remote sensing data, Moderate Resolution Imaging Spectroradiometer (MODIS) has been frequently used to measure the UHI effect in recent years. This article chooses a Chinese land surface temperature MODIS data set combined with weather station data by Zhao et al. (2020), this data set fully captures the year, quarter and monthly scale spatial and temporal variations of surface temperature, high accuracy (Zhao et al., 2020), The observation period is from 2003 to 2017, which basically corresponds to the regional integration, urbanization and industrialization of the YRD, so it is suitable for the research needs of this paper.
The land use data and urban boundaries in the YRD were extracted from the Chinese land use data in 2000 and 2015 (from the Data Center for Resource and Environmental Science of the Chinese Academy of Sciences, http://www.resdc.cn). This data set took the Landsat remote sensing image data of the United States as the main information source and then generated by artificial visual interpretation. In this paper, the construction land in the first-level land use classification is used to extract the boundaries of cities and towns in the YRD region (satellite cities and patches far from urban areas are regarded as independent units).
As demonstrated in section 2 of this paper, the Gross Domestic Production (GDP), the Permanent Urban Population (POP), the Fixed Asset Investment (FIX), the Industrial Output Value (IND), and the Motor Vehicle Population (MOT)are selected as the single influencing factors of the economic drivers of the UHI effect. Related data come from the Statistical Yearbook of Chinese Cities (2004-2018) and the official websites of local statistical departments.
This study examined the economic influencing mechanism of UHI effects and its Spatio-temporal characteristics based on the economic theory of urbanization and industrialization. Firstly, the UHI intensity in YRD regions is calculated by using MODIS land surface temperature (LST) data. Secondly, the Spatio-temporal characteristics of UHI intensity and economic influencing factors are analyzed. Thirdly, correlation analysis and the geographical detector are used to analyze the influencing mechanism of economic influencing factors on UHI. Finally, based on the discussion of the analysis results, our conclusions and policy implications are put forward. A work flowchart is shown in Figure 3.
At present, the evaluation method of UHI intensity has not formed a unified standard. According to different pixel selection strategies in urban and rural areas, the measurement methods of UHI intensity can be divided into two categories: the pixel method and the buffer method. The former is a method of selecting a certain number of representative reference pixels in cities and surrounding rural lands as typical sample areas to calculate the temperature difference between urban and rural areas based on land use classification data (Zhao et al., 2014). The latter is also a mainstream method to estimate UHI intensity. The first step is to find an equal-area buffer zone or 20-25 km equal-distance buffer outward as the rural area (Yao et al., 2017; Sun et al., 2019b) based on describing the urban boundary range, and then calculate the temperature difference between urban and rural areas. To avoid the subjectivity of pixel selection and the consideration of Spatio-temporal comparability of UHI intensity, this paper uses the equal-area buffer method to estimate the UHI intensity of the YRD region. The specific approach can be divided into four steps: (1) The invariant pixels of construction land in 2000 and 2015 are taken as the urban core area; (2) The boundary of urban core area to the boundary of built-up area in 2015 is defined as urban expansion area, that is, the urban area is divided into urban core area and urban expansion area; (3) The urban area is made equal-area buffer outward, and the resulting area is taken as the rural area; (4) The average surface temperature difference between the urban area and the surrounding rural area was used as the UHI intensity of each urban patch Figure 4A. Taking Shanghai as an example, urban and rural areas generated by using the above methods are shown in Figure 4B. The advantage of this method is that the calculated values of UHI intensity in different periods are longitudinally comparable and consistent, which can be used for a comparison of the large-scale UHI effect.
Figure 4 Divisions of urban-rural gradient zones. (A) The diagrammatic drawing of divisions. (B) The divisions result of Shanghai.
Spatial autocorrelation analysis can be divided into global spatial correlation analysis and local spatial correlation analysis, which can be represented by global Moran’s I and local Moran’s I respectively. The global Moran’s I is used to analyze the spatial distribution of UHI intensity and economic influencing factors in the YRD. For specific analysis ideas and steps, refer to relevant literature (Guo et al., 2020). The calculation formula of global Moran’s I is as follows:
where n is the total number of regions in the study area, wij is the spatial weight, W is the sum of the spatial weights, xi and xj are the attributes of region i and region j, respectively. is the average value of attributes. According to the corresponding relationship shown in Table 1, this paper uses global Moran’s I to conduct spatial autocorrelation analysis on the corresponding indexes of UHI intensity and economic influencing factors of cities in the YRD region.
Pearson correlation coefficient is used to analyze the correlation of various economic drivers affecting UHI and judge the degree and order of their relationship with UHI. For specific analysis ideas and steps, refer to relevant literature (Lei et al., 2021). In the observed samples, the technical formula of the Pearson correlation coefficient is:
where r represents the sample correlation coefficient, Xi and Yi represent the sample observations of independent variable i and dependent variable j, respectively. , represent the sample mean values of the independent variable and dependent variable, respectively.
The geographical detector is a tool to judge whether independent variables and dependent variables are consistent in spatial distribution from the perspective of spatial stratified heterogeneity. In other words, if independent variables have an influence on dependent variables, they should also tend to be consistent in spatial distribution (Lei et al., 2021). It has the advantages of independent variable collinearity immunity (Jinfeng and Chengdong, 2017) and can detect the interaction between different independent variables. The research objective of this paper is to study the influence of different types of independent variables in economic circles on the UHI effect (dependent variable). Since there are many possible independent variables in economic circles and the existence of collinearity is common, their interaction may produce different types of influence on the dependent variable, and the spatial heterogeneity is also very significant. Consequently, it is suitable to use geographic detectors to analyze this influence. For specific analysis ideas and steps, refer to the relevant literature (Feng et al., 2021). The geographic detector consists of four detectors: factor detection, interaction detection, risk detection, and ecological detection. In this paper, factor detectors are mainly used to detect the extent to which economic influencing factor X explains the spatial differentiation of UHI, and interaction is used to evaluate whether economic influencing factors X1 and X2 will increase or weaken the explanatory power of dependent variable UHI when they work together, or whether the influences of these factors on UHI are independent. The factor detection result is measured by q value, and the expression is:
where i = 1,…L is the strata of dependent variable UHI or economic influence factor X. In this paper, h is the division of 26 central cities in the YRD region. Ni and N are the number of units in city i and the YRD, respectively. and σ2 are the variances of the UHI of city i and YRD region, respectively. SSW and SST are the Within Sum of Squares and Total Sum of Squares, respectively. The range of q lies between zero and one, and the higher the q value is, the more significant the spatial differentiation of UHI is. If stratification is generated by economic influencing factor X, the higher the q value is, the stronger the explanatory power of this economic influencing factor to UHI is (and vice versa).
The method of interaction detection is to first calculate the q values of two economic influencing factors X1 and X2 on UHI respectively: q(X1)and q(X2), and calculate the q values of their interaction (the new polygon distribution formed by the tangency of the two layers of superimposed variables X1 and X2): q(X1∩X2) and compare q(X1), q(X2) and q(X1∩X2). The relationship between the two factors can be classified as follows:
Nonlinear-weaken: q(X1∩X2) <Min(q(X1), q(X2))
Uni-weaken: Min(q(X1), q(X2)) < q(X1∩X2) < Max(q(X1), q(X2))
Nonlinear-enhance: q(X1∩X2)>q(X1) +q(X2)
Bi-enhance: Max(q(X1), q(X2)) < q(X1∩X2) < q(X1) +q(X2)
Independent: q(X1∩X2) = q(X1) +q(X2)
The land surface temperature dataset of China from 2003 to 2017 made by Zhao et al. (2020), using MODIS satellite data, is used to calculate the UHI intensity of 26 urban core areas and buffer zones in the YRD from 2003 to 2017 (the results are in the Appendix). Descriptive statistics are made for the results, and the mean UHI intensity of each city during the observation period is arranged in descending order. As shown in Table 1. Overall, 18 of the 26 cities had a positive average UHI intensity during the observation period. Based on the average UHI intensity of 26 cities, the mean heat island intensity of cities in the YRD during the observation period was 0.143 ± 0.108°C(mean± standard deviation), indicating that it was a significant UHI effect in YRD. From 2003 to 2006, the UHI intensity in the YRD increased from 0.136°C to 0.196°C. The period from 2007 to 2014 was a period of shock adjustment, with the lowest 0.054°C in 2009 and the highest 0.186°C in 2013. From 2015 to 2016, the intensity increased to 0.202°C, which was the highest in previous years. For cities, Ningbo, Hefei, Yancheng, Nanjing, and Shanghai (> 0.4°C) had the highest mean UHI intensity in YRD. While Shanghai, Xuancheng, Yancheng, Ningbo, and Chizhou had the highest variance, indicating that these five cities had the most dramatic changes in UHI during the observation period. The geographical distribution of these cities is scattered, but this does not seem to matter to whether they ranked highly in UHI intensity or degree of change, which seems not consistent with the economic agglomeration distribution trend represented by industrialization and urbanization in the YRD region. Thus, in the next section we analyze the spatial relevance between UHI and economic influencing factors.
In terms of spatial distribution and variation, the UHI intensities of cities in 2003, 2010, and 2017 during the observation period were selected, and the UHI intensity level of corresponding years was grouped by Jenks natural breaks classification method (Figure 5). In 2003, Nanjing, Changzhou, Hefei, Hangzhou, Ningbo, and Shanghai were the cities with the maximum level, while Taizhou, Maanshan, and Chizhou were the cities with the minimum level. In 2010, four cities maintained the maximum level. Nanjing, Hefei, Ningbo, and Shanghai, Yancheng and Xuancheng increased to the maximum level, Zhenjiang and Tongling decreased to the minimum level based on the three cities in 2003. In 2017, Yancheng, Xuancheng, Hefei, and Ningbo remained at the maximum level, while Wuhu and Taizhou joined the minimum level club in addition to Maanshan and Chizhou. In terms of the change range of levels, except for Xuancheng, Tongling, Hangzhou, and Jinhua, the change of level reached two, and the change of other cities did not exceed one. In general, cities with high UHI intensity are mainly distributed in economically developed regions, while cities with low UHI intensity are mainly distributed in economically backward regions.
In this paper, Moran’s I was used to test the spatial autocorrelation of UHI intensity and its influencing factors in the YRD in 2003, 2010, and 2017, and preliminarily judge the spatial correlation of UHI intensity and its influencing factors in the YRD (Table 2). The results of Moran’s I show that the spatial autocorrelation of UHI intensity in the YRD is not significant and tends to be random distribution. In terms of the distribution of influencing factors (a total of 15), except for FIX, IND in 2010, and IND in 2017, the other 12 factors all showed a certain spatial autocorrelation and tended to be concentrated. This indicates that there are some differences in the spatial distribution of UHI intensity and economic drivers in the YRD, that is, the regions with a concentrated distribution of economic drivers are not necessarily the regions with high UHI intensity.
To further explore the spatial correlation between UHI intensity and economic drivers, referring to the ideas of similar studies (Shi et al., 2018), the Pearson correlation coefficient was first used to analyze the correlation of influencing factors, and then geographical detectors were used to analyze the Spatio-temporal differentiation of influencing factors of 26 cities in the YRD (Table 3). In 2003, Pearson correlation coefficients of the five influencing factors were all about 0.6, indicating a high correlation with the local UHI intensity. The coefficients of FIX, MOT, GDP, IND, and POP were in descending order. In 2010, Pearson correlation coefficients of five influencing factors all decreased, and the order was FIX, MOT, POP, GDP, and IND. In 2017, Pearson’s correlation coefficient of five influencing factors continued to decline, ranking as FIX, MOT, IND, POP, and GDP. The decreasing correlation coefficient indicates that the influence of urbanization and industrialization on UHI intensity in the YRD is decreasing. The high ranking of FIX and MOT indicates that the large increase of buildings and anthropogenic heat emission caused by motor vehicles may be the leading factors affecting UHI intensity.
Affected by the spatial stratified heterogeneity, the q values obtained by the geographical detector are all lower than the corresponding Pearson correlation coefficient (Table 3). In 2003, FIX, GDP, IND, POP, and MOT were ranked as the main factors affecting UHI intensity in the YRD considering the spatial stratified heterogeneity. In 2010, the q value increased, indicating that the five factors have a great impact on UHI intensity. MOT, POP, FIX, IND, and GDP were ranked in that order. In 2017, q values of all influencing factors except MOT were low, which was consistent with the overall downward trend of the Pearson correlation coefficient. MOT, FIX, POP, GDP, and IND were ranked in that order. In general, FIX and MOT are still in the top position, indicating that these two factors still have a significant positive correlation with the UHI intensity of the YRD after considering the spatial stratified heterogeneity.
The interaction of five influencing factors on the UHI effect was measured by interaction detection. A total of 30 pairs of interaction effects were generated in 2003, 2010, and 2017 (Table 4). The results show that 25 out of 30 pairs are bi-enhancing, 5 pairs are nonlinear-enhancing, and there is no weakening or independent relationship. It shows that the influence of any two factors is stronger than that of a single factor. In 2003, the top 5 most influential factors after interaction are FIX∩POP(0.553), FIX∩IND(0.509), FIX∩MOT(0.464), POP∩GDP(0.447), and FIX∩GDP(0.419) The results show that the combination of FIX and other factors is the most important economic driver of UHI intensity in the YRD. In 2010, the top 5 most influential factors after interaction are POP∩MOT(0.629), POP∩IND(0.625), FIX∩MOT(0.622), IND∩MOT(0.621), and GDP∩MOT(0.586). The results showed that MOT was the most important economic driver of UHI intensity in the YRD in 2010. In 2017, the q values of single factors were all low, but the top 5 factors with the strongest influence after interaction were all nonlinear-enhancing relationships. FIX∩MOT(0.653), POP∩MOT(0.645), IND∩MOT(0.627), FIX∩IND(0.582), and GDP∩IND(0.425) The results indicate that the economic drivers of UHI intensity in the YRD in 2017 are more complex than before. Except for MOT, the superposition of IND and FIX can produce a more significant UHI effect than a single factor.
The results of the interaction detection can reflect the deepening of economic development and urbanization, the influencing mechanism of the UHI effect of YRD was in a complicated evolution. From the previous model of FIX and MOT as dominant factors, it gradually transited to a more diverse model, in other words, the economic factors influencing UHI intensity are increasingly diversified. Overall, the UHI intensity with overlapping factors is more significant.
Although the results of The Moran’s test in the YRD in 4.1.1 show that regional UHI intensity and economic drivers have different spatial distribution patterns, that is, regions with a high concentration of economic influencing factors represented by urbanization and industrialization are not necessarily regions with a concentrated distribution of high UHI intensity. However, the subsequent analysis of the Pearson correlation coefficient and q value shows that the economic drivers dominated by FIX and MOT still have a significant positive correlation with the UHI intensity of cities in the YRD. This is basically consistent with the conclusion of similar studies. In terms of reasons, it may be that climatic background and other factors in the physical geographical circle also have a great impact on the UHI intensity of cities in the YRD (Zhao et al., 2014; Li et al., 2020), or that the increase of per capita income is influenced by a relationship of environmental Kuznets curve (Li et al., 2020). More importantly, the above conclusions also partially verify the theoretical influencing mechanism of urbanization and industrialization on the UHI effect in section 2. Besides, the interaction detector analysis by geographical detector confirmed the theoretical influencing mechanism proposed above, that is, with the deepening of industrialization and urbanization, cities overlapped with more influencing factors are more likely to promote high UHI intensity. And the development process of influencing factors also makes the development of the UHI effect more complicated.
By reviewing the economic and social development of the YRD, this paper argues that the above analysis can be more consistent and understanding. The industrialization process of cities in the YRD started in modern China. Compared with other regions in China (such as the Pearl River Delta), the YRD is an area with the prominent characteristics of strong government and strong capital combination (Wang et al., 2018). Such a pattern makes the industrialization process of the YRD more influenced by public policy. In this pattern, fixed-asset investment led by the government used to be an important engine of economic growth in the YRD, but its growth rate and the proportion have gradually declined since 2003, while the proportion of GDP in China tends to rise (Hou and Feng, 2011). This is basically consistent with the increasingly-changing trend of influencing mode, which is from fixed-asset investment as a dominant factor to diversified influencing factors of UHI intensity analyzed above.
With the development of urbanization, the YRD’s rising attracted attention and studies of related automobile exhaust pollution (Xu et al., 2019; Zhou et al., 2020). As an important source of anthropogenic heat emission, motor vehicles are accounted for the UHI effect, and studies on their correlation are verified year by year (Lee et al., 2014; Abutaleb et al., 2015; Alqasemi et al., 2021). In consequence, it is reasonable that motor vehicles, as a dominant influencing factor of the UHI effect in the YRD in 2010, produced more significant UHI effects after superimposing other influencing factors.
As one of the regions with the most developed economy and the deepest degree of urbanization in China, the economic development of YRD is also affected by the process of regional integration. This regional integration is increasingly reflected in the coordination of administration, public services, and infrastructure. In 1997, the first joint meeting of mayors of cities in the Yangtze River Delta was held, which opened the prelude of cooperation among cities. In 2003, the fourth Yangtze River Delta Mayors’ Summit was held, during which the concept of ‘Yangtze River Delta People’ was first proposed, and a 16-city framework structure that has been stable and widely recognized for a long time has been established. In 2010, The Chinese State Council approved the implementation of the Regional Plan for the Yangtze River Delta region, which expanded the original 16 cities to 25 for the first time at the national strategic level and explicitly proposed the construction of world-class city clusters with strong international competitiveness. In 2018, Chinese President Xi Jinping proposed to support the integrated development of the Yangtze River Delta region and made it a national strategy. Based on these arrangements, it embodies the integration of YRD as the national macro-level willingness to promote, and it is also a deepening process of economic integration for regional administration and the demand for public services and infrastructure, which indirectly reflects the fact that, with the improvement of city’s economic development, the process of urbanization and industrialization in the YRD was changing to a stage of greater complexity and diversity than ever before. In addition, their interrelated and inseparable relationship is not only in theory, but also confirmed by some studies on industrialization and urbanization in the YRD (Dewen and Yilun, 2013; Kang et al., 2021), indicating that the industrialization and urbanization in the YRD itself is also a process of mutual superposition. Consequently, their impacts on UHI intensity were increasingly complex and diversified.
Economic geography focuses on the study of the relationship between economic circles and other circles, such as human circles and layers of physical geography (Li et al., 2012). The idea of this paper is to investigate the influence of economic geographical circles on physical geographical circles and explore the Spatio-temporal differentiation of the influence of economic drivers on the UHI effect. Through the analysis of the YRD, this paper tries to bridge the knowledge gap proposed in the introduction, and the following main conclusions are drawn:
(1) During the observation period from 2003 to 2017, the UHI intensity of major cities in the YRD region is significant and fluctuates to a certain extent.
(2) The UHI intensity of the YRD has a certain correlation with the economic drivers mainly characterized by urbanization and industrialization, but this correlation tends to weaken during the observation period due to the influence of exogenous factors such as physical geographical factors.
(3) Industrialization and urbanization are the main influencing factors of the UHI effect in the YRD from the perspective of economic motivation alone. The essence of industrialization and urbanization is the agglomeration of related economic factors in the urban space. Through the test of this paper, the economic influencing factors of UHI intensity in the YRD during the early observation period are mainly the Fixed Asset Investment and the Motor Vehicles Population but gradually transited to multiple superimposed factors. Cities with more economic influencing factors are more likely to promote higher UHI intensity.
Urbanization and industrialization, as the main economic drivers, have a certain degree of correlation with the UHI effect in the YRD. In recent years, with the deepening of urbanization and industrialization, this correlation tends to weaken, but it is still worth the attention of public service providers that the superposition of various economic factors may promote more serious impacts. To this, the policy suggestion of this paper is the YRD regional policymakers should pay attention to multiple superimposed economic factors. Combined with local progress of urbanization and industrialization, policy regulation and guidance need to be balanced to avoid in a particular city space falling within the scope of the superposition of multiple economic factors and highly-concentrated activities. At the same time, it is worthy to invest in and optimize the configuration of cold islands, such as parks, green space, and landscape configuration.
In terms of temporal segmentation, the UHI intensity of the same year fluctuates across seasons and between day and night. In terms of spatial segmentation, there are also certain differences in UHI intensity between counties and urban areas under the jurisdiction of each city. However, due to the limited granularity of relevant data used to analyze economic drivers, this study cannot match such Spatio-temporal heterogeneity and is worthy of further study.
The original contributions presented in the study are included in the article/supplementary material. Further inquiries can be directed to the corresponding author.
YY planned the study, collected, and analyzed the data, and wrote the manuscript. JL improved the research design and research model. YS co-analyzed the data and co-improved the research design. PT co-analyzed the data and co-drew the figures. YY and JL did the main main manuscript revision and editing. All authors listed have made a substantial, direct, and intellectual contribution to the work, and approved it for publication. The authors extend their appreciation to the reviewers who gave constructive comments that helped bring considerable improvements to a previous version of the manuscript.
This research was funded by the National Natural Science Foundation of China (41976209) and Ningbo Soft Science Research Project (2021R027).
The authors declare that the research was conducted in the absence of any commercial or financial relationships that could be construed as a potential conflict of interest.
All claims expressed in this article are solely those of the authors and do not necessarily represent those of their affiliated organizations, or those of the publisher, the editors and the reviewers. Any product that may be evaluated in this article, or claim that may be made by its manufacturer, is not guaranteed or endorsed by the publisher.
Abutaleb K., Ngie A., Darwish A., Ahmed M., Arafat S., Ahmed F. (2015). Assessment of Urban Heat Island Using Remotely Sensed Imagery Over Greater Cairo, Egypt. Adv. Remote Sens. 4 (1), 35–47. doi: 10.4236/ars.2015.41004
Akkose G., Meral Akgul C., Dino I. G. (2021). Educational Building Retrofit Under Climate Change and Urban Heat Island Effect. J. Build. Eng. 40, 102294V. doi: 10.1016/j.jobe.2021.102294
Alqasemi A. S., Hereher M. E., Kaplan G., Al-Quraishi A. M. F., Saibi H. (2021). Impact of COVID-19 Lockdown Upon the Air Quality and Surface Urban Heat Island Intensity Over the United Arab Emirates. Sci. Total Environ. 767, 144330. doi.10.1016/j.scitotenv.2020.144330
Badaro-Saliba N., Adjizian-Gerard J., Zaarour R., Najjar G. (2021). LCZ Scheme for Assessing Urban Heat Island Intensity in a Complex Urban Area (Beirut, Lebanon). Urban Climate 37, 100846. doi: 10.1016/j.uclim.2021.100846
Chen X., Wang Z., Bao Y. (2021). Cool Island Effects of Urban Remnant Natural Mountains for Cooling Communities: A Case Study of Guiyang, China. Sustain. Cities Soc. 71, 102983. doi: 10.1016/j.scs.2021.102983
Cheval S., Popa A.-M., Șandric I., Iojă I.-C. (2020). Exploratory Analysis of Cooling Effect of Urban Lakes on Land Surface Temperature in Bucharest (Romania) Using Landsat Imagery. Urban Climate 34, 100696. doi: 10.1016/j.uclim.2020.100696
Dewan A., Kiselev G., Botje D., Mahmud G. I., Bhuian M. H., Hassan Q. K. (2021). Surface Urban Heat Island Intensity in Five Major Cities of Bangladesh: Patterns, Drivers and Trends. Sustain. Cities Soc. 71, 102926. doi: 10.1016/j.scs.2021.102926
Dewen Y., Yilun Y. (2013). The Influence of Industrialization on Urbanization: A Case Study of Yangtze River Delta Region. Ind. Organ. Rev. 7 (4), 83–97.
Estoque R. C., Murayama Y. (2016). Quantifying Landscape Pattern and Ecosystem Service Value Changes in Four Rapidly Urbanizing Hill Stations of Southeast Asia. Landscape Ecol. 31 (7), 1481–1507. doi: 10.1007/s10980-016-0341-6
Feng R., Wang F., Wang K., Wang H., Li L. (2021). Urban Ecological Land and Natural-Anthropogenic Environment Interactively Drive Surface Urban Heat Island: An Urban Agglomeration-Level Study in China. Environ. Int. 157, 106857. doi: 10.1016/j.envint.2021.106857
Feyisa G. L., Dons K., Meilby H. (2014). Efficiency of Parks in Mitigating Urban Heat Island Effect: An Example From Addis Ababa. Landscape Urban Plann. 123, 87–95. doi: 10.1016/j.landurbplan.2013.12.008
Gabriel K. M. A., Endlicher W. R. (2011). Urban and Rural Mortality Rates During Heat Waves in Berlin and Brandenburg, Germany. Environ. Pollut. 159 (8-9), 2044–2050. doi: 10.1016/j.envpol.2011.01.016
Guo A., Yang J., Xiao X., Xia J., Jin C., Li X. (2020). Influences of Urban Spatial Form on Urban Heat Island Effects at the Community Level in China. Sustain. Cities Soc. 53, 101972. doi: 10.1016/j.scs.2019.101972
Hou X., Feng C. (2011). Fixed Asset Investment and the Transformation of Economic Growth Pattern: An Empirical Analysis Based on the Data of the Yangtze River Delta. East China Econ. Manage. 25 (11), 18–22. doi: 10.3969/j.issn.1007-5097.2011.11.005
Imhoff M. L., Zhang P., Wolfe R. E., Bounoua L. (2010). Remote Sensing of the Urban Heat Island Effect Across Biomes in the Continental USA. Remote Sens. Environ. 114 (3), 504–513. doi: 10.1016/j.rse.2009.10.008
Jinfeng W., Chengdong X. (2017). Geodetectors: Principles and Prospects. Acta Geographica Sin. 72(1), 116–134. doi: 10.11821/dlxb201701010
Kang J., Xu W., Ning Y. (2021). Spatial Agglomeration of Manufacturing Industry Based on Localization, Urbanization and Globalization: A Case Study of Yangtze River Delta. Sci. Geographica Sin. 41 (10), 1773–1782. doi: 10.13249/j.cnki.sgs.2021.10.009
Lee Y. Y., Din M. F. M., Ponraj M., Noor Z. Z., Iwao K., Chelliapan S. (2017). Overview of Urban Heat Island (Uhi) Phenomenon Towards Human Thermal Comfort. Environ. Eng. Manage. J. 16 (9), 2097–2111. doi: 10.30638/eemj.2017.217
Lee J. S., Kim J. T., Lee M. G. (2014). Mitigation of Urban Heat Island Effect and Greenroofs. Indoor Built Environ. 23 (1):62–69. doi: 10.1177/1420326X12474483
Lei J., Xingyu C., Hong Z. (2021). Spatial Distribution Characteristics and Causes of Differentiation of Urban Nursing Homes in China. Acta Geographica Sin. 76 (8), 1951–1964. doi: 10.11821/dlxb202108010
Lemelin A., Rubiera-Morollón F., Gómez-Loscos A. (2016). Measuring Urban Agglomeration: A Refoundation of the Mean City-Population Size Index. Soc. Indic. Res. 125 (2), 589–612. doi: 10.1007/s11205-014-0846-9
Li X., Luo Q., Zhu Y. (2012). The Distinction Between Economic Geography and Regional Economics. Econ. Geogr (in Chinese) 32 (7), 1–5. doi: 10.15957/j.cnki.jjdl.2012.07.001
Lin Y., Jim C. Y., Deng J., Wang Z. (2018). Urbanization Effect on Spatiotemporal Thermal Patterns and Changes in Hangzhou (China). Build. Environ. 145, 166–176. doi: 10.1016/j.buildenv.2018.09.020
Lin S., Luo M., Walker R. J., Liu X., Hwang S.-A., Chinery R. (2009). Extreme High Temperatures and Hospital Admissions for Respiratory and Cardiovascular Diseases. Epidemiology 20 (5), 738–746. doi: 10.1097/EDE.0b013e3181ad5522
Li Y., Sun Y., Li J., Gao C. (2020). Socioeconomic Drivers of Urban Heat Island Effect: Empirical Evidence From Major Chinese Cities. Sustain. Cities Soc. 63, 102425. doi: 10.1016/j.scs.2020.102425
Liu Y. (2014). Social and Economic Impacts of Global Climate Change on Coastal Areas of Yangtze River Estuary (Shanghai: D, East China Normal University).
Michelozzi P., Accetta G., De Sario M., D'Ippoliti D., Marino C., Baccini M., et al. (2009). High Temperature and Hospitalizations for Cardiovascular and Respiratory Causes in 12 European Cities. Am. J. Respir. Crit. Care Med. 179 (5), 383–389. doi: 10.1164/rccm.200802-217OC
Park C. Y., Lee D. K., Asawa T., Murakami A., Kim H. G., Lee M. K., et al. (2019). Influence of Urban Form on the Cooling Effect of a Small Urban River. Landscape Urban Plann. 183, 26–35. doi: 10.1016/j.landurbplan.2018.10.022
Peng J., Hu Y., Dong J., Liu Q., Liu Y. (2020). Quantifying Spatial Morphology and Connectivity of Urban Heat Islands in a Megacity: A Radius Approach. Sci. Total Environ. 714, 136792. doi: 10.1016/j.scitotenv.2020.136792
Salerno F., Gaetano V., Gianni T. (2018). Urbanization and Climate Change Impacts on Surface Water Quality: Enhancing the Resilience by Reducing Impervious Surfaces. Water Res. 144, 491–502. doi: 10.1016/j.watres.2018.07.058
Shen T., Chow D. H. C., Darkwa J. (2013). Simulating the Influence of Microclimatic Design on Mitigating the Urban Heat Island Effect in the Hangzhou Metropolitan Area of China. Int. J. Low-Carbon Technol. 11 (1), 130–139. doi: 10.1093/ijlct/ctt050
Shi T., Hu Z., Shi Z., Guo L., Chen Y., Li Q., et al. (2018). Geo-Detection of Factors Controlling Spatial Patterns of Heavy Metals in Urban Topsoil Using Multi-Source Data. Sci. Total Environ. 643, 451–459. doi: 10.1016/j.scitotenv.2018.06.224
Sun Y., Gao C., Li J., Wang R., Liu J. (2019b). Evaluating Urban Heat Island Intensity and its Associated Determinants of Towns and Cities Continuum in the Yangtze River Delta Urban Agglomerations. Sustain. Cities Soc. 50, 101659. doi: 10.1016/j.scs.2019.101659
Sun R., Lü Y., Yang X., Chen L. (2019a). Understanding the Variability of Urban Heat Islands From Local Background Climate and Urbanization. J. Cleaner Prod. 208, 743–752. doi: 10.1016/j.jclepro.2018.10.178
Sun R., Xie W., Chen L. (2018). A Landscape Connectivity Model to Quantify Contributions of Heat Sources and Sinks in Urban Regions. Landscape Urban Plann. 178, 43–50. doi: 10.1016/j.landurbplan.2018.05.015
Susca T., Pomponi F. (2020). Heat Island Effects in Urban Life Cycle Assessment: Novel Insights to Include the Effects of the Urban Heat Island and UHI-mitigation Measures in LCA for Effective Policy Making. J. Ind. Ecol. 24 (2), 410–423. doi: 10.1111/jiec.12980
Swamy G., Nagendra S. M. S., Schlink U. (2017). Urban Heat Island (UHI) Influence on Secondary Pollutant Formation in a Tropical Humid Environment. J. Air Waste Manage. Assoc. 67 (10), 1080–1091. doi: 10.1080/10962247.2017.1325417
Tian P., Li J., Cao L., Pu R., Wang Z., Zhang H., et al. (2021). Assessing Spatiotemporal Characteristics of Urban Heat Islands From the Perspective of an Urban Expansion and Green Infrastructure. Sustain. Cities Soc. 74, 103208. doi: 10.1016/j.scs.2021.103208
Wang J., Fan L., Zhang S. (2018). Regional Differences of Industrialization Model and Migrant Workers' Problems: A Comparative Study Based on the Pearl River Delta and the Yangtze River Delta. Sociol. Stud. 33(4), 109–136+244. doi: 10.19934/j.cnki.shxyj.2018.04.005
Wu Q., Miao S., Huang H., Guo M., Zhang L., Yang L., et al. (2022). Quantitative Analysis on Coastline Changes of Yangtze River Delta Based on High Spatial Resolution Remote Sensing Images. Remote Sens. 14 (2), 310. doi: 10.3390/rs14020310
Xu W., Sun J., Liu Y., Xiao Y., Tian Y., Zhao B., et al. (2019). Spatiotemporal Variation and Socioeconomic Drivers of Air Pollution in China During 2005-2016. J. Environ. Manage. 245, 66–75. doi: 10.1016/j.jenvman.2019.05.041
Yao R., Wang L., Huang X., Niu Z., Liu F., Wang Q. (2017). Temporal Trends of Surface Urban Heat Islands and Associated Determinants in Major Chinese Cities. Sci. Total Environ. 609, 742–754. doi: 10.1016/j.scitotenv.2017.07.217
Yu H. (2017). International Theoretical Frontiers of Urbanization Research (Shanghai: Shanghai Academy of Social Sciences Press).
Yue W., Qiu S., Xu H., Xu L., Zhang L. (2019). Polycentric Urban Development and Urban Thermal Environment: A Case of Hangzhou, China. Landscape Urban Plann. 189, 58–70. doi: 10.1016/j.landurbplan.2019.04.008
Zak M., Nita I.-A., Dumitrescu A., Cheval S. (2020). Influence of Synoptic Scale Atmospheric Circulation on the Development of Urban Heat Island in Prague and Bucharest. Urban Climate 34, 100681–100681. doi: 10.1016/j.uclim.2020.100681
Zhao L., Lee X., Smith R. B., Oleson K. (2014). Strong Contributions of Local Background Climate to Urban Heat Islands. Nature 511 (7508), 216–219. doi: 10.1038/nature13462
Zhao B., Mao K., Cai Y., Shi J., Li Z., Qin Z., et al. (2020). A Combined Terra and Aqua MODIS Land Surface Temperature and Meteorological Station Data Product for China From 2003 to 2017. Earth Syst. Sci. Data 12, 2555–2577. doi: 10.5194/essd-12-2555-2020
Zhou M., Wang X., Lin X., Yang S., Zhang J., Chen J. (2020). Automobile Exhaust Particles Retention Capacity Assessment of Two Common Garden Plants in Different Seasons in the Yangtze River Delta Using Open-Top Chambers. Environ. Pollut. 263, 114560. doi: 10.1016/j.envpol.2020.114560
Keywords: urban heat island intensity, remote sensing data, Yangtze River Delta, geographical detector, economic drivers
Citation: Yan Y, Sun Y, Tian P and Li J (2022) Spatio-Temporal Variation of Economic Drivers of Urban Heat Island Effect in Yangtze River Delta. Front. Mar. Sci. 9:877301. doi: 10.3389/fmars.2022.877301
Received: 16 February 2022; Accepted: 16 March 2022;
Published: 09 May 2022.
Edited by:
Chao Chen, Zhejiang Ocean University, ChinaReviewed by:
Venkata Subrahmanyam Mantravadi, Zhejiang Ocean University, ChinaCopyright © 2022 Yan, Sun, Tian and Li. This is an open-access article distributed under the terms of the Creative Commons Attribution License (CC BY). The use, distribution or reproduction in other forums is permitted, provided the original author(s) and the copyright owner(s) are credited and that the original publication in this journal is cited, in accordance with accepted academic practice. No use, distribution or reproduction is permitted which does not comply with these terms.
*Correspondence: Jialin Li, bmJuajIwMDFAMTYzLmNvbQ==
Disclaimer: All claims expressed in this article are solely those of the authors and do not necessarily represent those of their affiliated organizations, or those of the publisher, the editors and the reviewers. Any product that may be evaluated in this article or claim that may be made by its manufacturer is not guaranteed or endorsed by the publisher.
Research integrity at Frontiers
Learn more about the work of our research integrity team to safeguard the quality of each article we publish.