- 1Research Center for Eco-Environmental Sciences, Chinese Academy of Sciences, Beijing, China
- 2University of Chinese Academy of Sciences, Chinese Academy of Sciences, Beijing, China
Deoxyribonucleic acid methylation and gene transcription have been proved as two underlying mechanisms involved in rapid plastic response to environmental stresses. However, it remains elusive on how DNA methylation regulates gene transcription under acute and recurring environmental challenges to form the stress memory, further contributing to invasion success during range expansions. Using a model invasive species Ciona robusta, we investigated the regulatory roles of DNA methylation on gene transcription and their contribution to the formation of stress memory at 30 genes under acute and recurring osmotic challenges simulated during the invasion process. We found the bimodal distribution of methylation level for the 68 mCpGs identified across all the genes after challenges, but only five sites were significantly correlated with the expression of their corresponding genes. These genes participated in the biological processes of Ca2+ transport and metabolism of lipid and proline. At the DNA methylation level, we found two early-responding and four tardy-responding sites of stress memory and these sites were functionally related to genes involved in the biosynthesis of proline, metabolism of lipid, and transport of taurine and Ca2+. At the transcriptional level, three tardy-responding and five early-responding memory genes were involved in the transport of ions, regulation of water channels, biosynthesis of taurine, and metabolism of lipid. Altogether, the findings here suggest that DNA methylation and gene transcription should work in concert to facilitate the formation of stress memory, thus further improving the performance of invaders under recurring environmental challenges during biological invasions.
Introduction
Nowadays, species constantly suffer from various environmental challenges derived from multiple interacting factors such as global climate change and frequent anthropogenic activities. The ability of species to rapidly respond and adapt to fluctuating environments is pivotal for their survival, particularly for invasive species. During the multi-stage invasion process, invaders are constantly and inevitably challenged by acute and recurring environmental stresses (Zhan et al., 2017; Li et al., 2020). Taking shipping-mediated range expansions as an example, marine invaders adhered to the hull of shipping vessels or sucked into ballast tanks must endure severe environmental challenges during subsequent shipping voyages such as salinity and temperature fluctuations ~15% and ~20°C, respectively (Briski et al., 2013). Such dramatic environmental fluctuations can be recurring during the whole shipping voyage such as during ballast water exchanges and environmental changes in trans-oceans (Ibrahim and El-Naggar, 2012; Gollasch and David, 2021). However, the underlying mechanisms of rapid environmental adaptation and assimilation still remain elusive.
Recent studies have largely dissected the crucial roles of phenotypic plasticity in rapid environmental response and adaptation (Heckwolf et al., 2020; Liew et al., 2020; Huang and Zhan, 2021). Among several well-studied mechanisms for phenotypic plasticity, DNA methylation represents a rapidly functional strategy by effectively regulating multiple downstream biological processes such as gene expression (Ozaki et al., 2017). For the marine invader Ciona savignyi, remarkable divergence of genome-wide DNA methylation patterns could be rapidly (~1 h) detected after acute osmotic and thermal stresses, suggesting DNA methylation as a potential facilitator of rapid response under environmental challenges (Huang et al., 2017). For the lizard colonizing new habitats, alterations in DNA methylome were functionally related to the genes responsible for rapid response to environmental shifts (Hu et al., 2019). Methylation of inducer of CBF expression 1 (ICE1), a transcription factor required for activation and regulation of the C-repeat (CRT)-binding factor (CBF) pathway under cold conditions, was verified to participate in rapid response and adaptation of plant species (Xie et al., 2019, 2020). All the available evidences support that DNA methylation-mediated phenotypic plasticity could produce adaptive phenotypes more rapidly, thus “buying time” for genetic adaptation to catch up under rapidly changing environments (Heckwolf et al., 2020). Interestingly, organisms could remember past environmental stresses and respond more rapidly or robustly under the subsequent same stresses, a phenomenon termed as “stress memory” (Ding et al., 2012). Several studies have revealed the existence of DNA methylation-mediated stress memory under environmental cues. For rice cultivars, DNA methylation could regulate the activity of transposable elements and gene expression to cope with the recurring drought stresses (Kou et al., 2021). DNA methylation-mediated stress memory could also contribute to the enhanced tolerance for Boea hygrometrica under recurring dehydration stresses via upregulation of memory genes related to the accumulation of oligosaccharides and vacuolar amino acids (Sun et al., 2021).
Despite the fact that the functions of phenotypic plasticity in rapid environmental response have been well-accepted, its downstream regulatory roles are still under hot debates (Foret et al., 2012; Neri et al., 2017; Li et al., 2018, 2021; Smith et al., 2020). In a “standard” model, the methylation of CpG islands within promoter region is generally correlated with the transcriptional repression (Halpern et al., 2014). However, the role of promoter methylation in transcriptional quiescence has been increasingly questioned. For the mouse Igf2 gene, DNA hypermethylation of a differentially methylation region within promoter was associated with its active transcription (Murrell et al., 2001). During the endodermal development, hypermethylation of one CpG island within Forkhead box A2 (FOXA2) promoter might contribute to the active transcription of this gene (Halpern et al., 2014). In contrast to the widely studied functions of promoter methylation, the role of gene body methylation is still uncertain and perplexing, as it has been reported to be involved in negative/positive regulations at the transcript levels, alternative splicing, mitigation of transcriptional noise, and maintenance of transcriptional homeostasis (Foret et al., 2012; Neri et al., 2017; Li et al., 2018, 2021). Collectively, these results suggest that the regulatory roles of DNA methylation in transcription might be far more complicated than what we have known. Given that DNA methylation is tightly correlated with the regulation of gene expression, we hypothesize that DNA methylation might regulate the transcription of key genes under acute and recurring stresses and further facilitate the formation of stress memory. Indeed, our previous study have already identified two memory genes involved in the DNA methylation pathway, i.e., DNA methyltransferase 3a1 (DNMT3a1) and ten-eleven translocation protein 1 (TET1) and the transcriptional memory of which might facilitate the formation of DNA methylation-mediated stress memory under recurring environmental stresses (Fu et al., 2021). However, it remains largely unknown upon which downstream memory genes might be regulated by DNA methylation and further contributes to the formation of stress memory.
The highly invasive tunicate Ciona robusta (C. robusta), which is presumably native to the Northwest Pacific, could tolerate various environmental conditions and has successfully invaded coastal areas worldwide (Caputi et al., 2007). Recently, this invader has successfully colonized a Red Sea Marina, suggesting its rapidly responding ability to harsh environments of extreme temperature and salinity (Shenkar et al., 2017; Chen et al., 2018). Salinity can greatly affect multiple crucial biological processes of marine organisms such as survival, growth, and particularly reproduction (Epelbaum et al., 2009). For marine organisms under osmotic pressure, the maintenance of intracellular osmotic homeostasis can be accomplished by regulation of salt stress effectors such as aquaporins (AQPs), ions, and free amino acids and are generally accompanied by increased energy expenditure to fuel those processes (Meng et al., 2013; Frouin et al., 2018). These salt stress effectors are indispensable for transport and metabolism of osmolytes or facilitating water transport, thus playing crucial roles in acclimation to osmotic stresses (Towle et al., 2011; Meng et al., 2013; Pu and Zhan, 2017; Liu et al., 2019). Hence, in this study, we selected 30 genes involved in the transport of water and osmolytes (ions and free amino acids) as well as metabolism of lipid and energy (Table 1) and simulated the acute and recurring hyperosmotic stresses during the invasion process in a controlled experimental system. For the transport of water molecules, we selected 4 AQP encoding genes (Table 1) that could facilitate the transport of water molecules through lipid bilayers (Madeira et al., 2016). For the regulation of inorganic and organic osmolytes, we focused on genes involved in transport of Na+, K+, Ca2+ as well as metabolism of taurine, proline, and glycine (Table 1). These salt stress effectors have been demonstrated to be the main driving force for maintaining intracellular homeostasis (Towle et al., 2011; Meng et al., 2013; Pu and Zhan, 2017; Liu et al., 2019). Since the transport of certain osmolytes is energy consuming, genes related to fatty acid metabolism were chosen to investigate the molecular response of energy metabolism under recurring hyperosmotic stresses (Table 1). By integrating the DNA methylation data and gene expression data of these candidate genes, we aim to study the regulatory roles of DNA methylation on gene transcription and further characterize how such regulatory relationships contribute to rapid response and formation of stress memory under recurring environmental stresses during successful invasions into different saline environments.
Materials and Methods
Sample Collection
Mature C. robusta individuals were collected from the artificially established substrates in Longwangtang, Dalian, Liaoning Province, China (38°49′19″N, 121°24′28″E) and subsequently domesticated for 3 days under ambient conditions. Details with respect to the domestication were described in our previously published study (Fu et al., 2021).
To simulate the recurring hyperosmotic pressure encountered in the invasion process, we conducted repetitive stress treatment (Figure 1). In brief, C. robusta individuals were challenged with osmotic stress (40%) for 72 h (S1 stage), followed by 24 h of recovery in ambient conditions (R stage), and subsequently challenged with osmotic stress (40%) again for another 72 h (S2 stage). Healthy individuals were collected at the time points of 0, 24, and 48 h of the S1 stage, 24 h of the R stage, and 24 and 48 h of the S2 stage. To minimize the effect of genetic composition on DNA methylation and transcription, all the individuals were randomly collected from different pieces of artificially established substrates. Six biological replicates were collected at each sampling time point, but failed samples after sequencing library construction were discarded for downstream analyses. Somatic muscles were dissected and then immediately preserved in 100% ethanol for DNA extraction and flash frozen in liquid nitrogen for RNA extraction. All the preserved samples were stored at −80°C before downstream analyses.
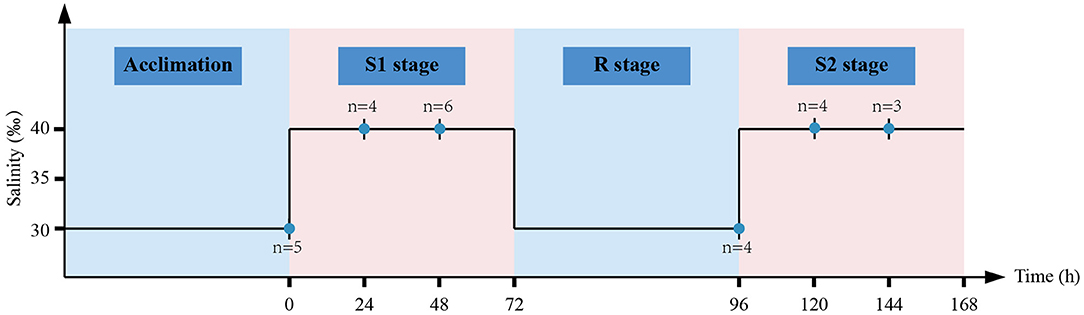
Figure 1. Schematic illustration of the experimental design. After acclimation for 3 days, healthy Ciona robusta (C. robusta) individuals were randomly selected for subsequent hyperosmotic stress experiment. C. robusta individuals were treated with hyperosmotic stress (40%) for 72 h (S1 stage), then transferred into ambient condition (30%) for 24 h (R stage), and finally subjected to another round of hyperosmotic stress (40%) for 72 h (S2 stage). Short vertical black lines with blue dots represent sampling time points and the letter “n” represents the number of biological replicates at each time point.
Nucleic Acid Isolation, Library Construction, and Sequencing
Genomic DNA (gDNA) was isolated using the classical CTAB-phenol-chloroform method. The quality and quantity of each DNA sample were assessed by agarose gel electrophoresis and Qubit® 2.0 (Thermo Fisher Scientific, Massachusetts, USA). To obtain the DNA methylation data, we constructed whole-genome bisulfite sequencing (WGBS) libraries following the instruction of the EZ DNA Methylation-GoldTM Kit (Zymo Research, California, USA). After library construction, insert size was measured using the Agilent 2100 Bioanalyzer (Agilent Technologies, California, USA). Each library was quantified again with the StepOnePlusTM Real-Time PCR System (Applied Biosystems, Waltham, Massachusetts, USA) to ensure the effective nucleic acid concentration.
Total RNA was extracted using Trizol reagent (Ambion, Massachusetts, USA) according to the instruction of the manufacturer and then treated with DNase to remove potential genomic DNA contamination. The purity, quantity, and integration of each RNA sample were assessed by the NanoPhotometer® (Implen, California, USA), the Qubit® 3.0 Fluorometer (Life Technologies, California, USA), and the Agilent 2100 Bioanalyzer (Agilent Technologies, California, USA). RNA sequencing (RNA-seq) libraries were prepared following the instruction of the NEBNext® Ultra™ RNA Library Prep Kit for Illumina® (New England Biolabs, Massachusetts, USA). Insert size was measured using the Agilent 2100 Bioanalyzer (Agilent Technologies, California, USA). Each library was quantified again with the CFX96 Touch Real-Time PCR Detection System (Bio-Rad Laboratories, California, USA) to ensure the effective nucleic acid concentration. All the WGBS and RNA-seq libraries were sequenced on the Illumina HiSeq X Ten Sequencing System (Illumina, California, USA) with the PE150 strategy.
Identification of Candidate Genes
We selected 30 genes involved in the transport of ions, free amino acids, water, as well as lipid metabolism, and investigated rapid response of DNA methylation dynamics of these genes under recurring hyperosmotic stresses (Table 1). These genes have been previously demonstrated to be involved in the regulation of cellular osmotic homeostasis as well as acclimation or adaptation to saline environment of various aquatic ecosystems (Towle et al., 2011; Meng et al., 2013; Pu and Zhan, 2017; Frouin et al., 2018; Liu et al., 2019). We downloaded complete sets of amino acid sequences of candidate genes from the Ghost database (http://ghost.zool.kyoto-u.ac.jp/default_ht.html). To further confirm the identity of our candidate genes, amino acid sequences of candidate genes were submitted to the National Center for Biotechnology Information (NCBI) Batch CD-Search tool for protein domain analysis. Genes with amino acid sequences harboring a full set of conserved domains were retained as candidate genes (Table 1).
Quantification of DNA Methylation Levels and Identification of Stress-Responsive Sites
Raw reads were trimmed and quality controlled using Trimmomatic version 0.39 (Bolger et al., 2014) and FastQC version 0.11.9 (Babraham Institute, https://www.bioinformatics.babraham.ac.uk/projects/fastqc/), respectively. The Bismark 2.0 pipeline was used to extract methylation status of individual cytosines (Krueger and Andrews, 2011). Specifically, clean reads were aligned to the latest C. robusta reference genome (HT version, http://ghost.zool.kyoto-u.ac.jp/default_ht.html) using bismark, deduplication was performed using deduplicate_bismark, and methylation status of individual cytosines were extracted using bismark_methylation_extractor with default parameters. Bisulfite conversion efficiency of each sample was calculated by aligning clean reads against lambda genome using Bismark 2.0 (Krueger and Andrews, 2011). Samples with conversion efficiencies higher than 99% were used in subsequent analyses.
Subsequently, cytosine reports produced by the Bismark 2.0 pipeline were read using the R package “methylKit” to obtain methylation data of individual CpGs. To be specific, CpGs with read coverage <5 were discarded. To obtain methylation status of individual CpG sites, percMethylation function in “methylKit” was used to calculate the methylation levels. The R package “GenomicRanges” was used to select the methylation data for the candidate genes. Differential methylation analysis was performed using calculateDiffMeth function in “methylKit” package using the chi-squared test (p < 0.05). CpG sites with methylation levels significantly differentiated between the control group (0 h) and any of the hyperosmotic treatment groups (24/48/120/144 h) as well as the recovery group (96 h) (p < 0.05) were considered as stress-responsive sites and genes harboring stress-responsive sites within gene body and promoter regions were considered as DNA methylation-related stress-responsive genes (DMSG). In this study, gene body region is defined as an entire region from the transcription start site (the location where DNA is being transcribed by RNA polymerase) to the transcription end site (the location where the transcription of DNA terminates) and promoter region is defined as 2,000 bp upstream of transcription start sites. Histogram of site methylation levels of the candidate genes was illustrated using the “hist” function in “graphics” package. The variation of site methylation levels among samples was illustrated with principal component analysis (PCA) using “prcomp” function built in the R Stats Package and “ggord” R package.
Quantification of Gene Expression Levels
Raw reads were trimmed and quality controlled using trimmomatic version 0.39 (Bolger et al., 2014) and FastQC version 0.11.9 (Babraham Institute, https://www.bioinformatics.babraham.ac.uk/projects/fastqc/), respectively. Clean reads were then aligned to the C. robusta genome using HISAT2 pipeline (Kim et al., 2019). StringTie version 2.1.4 (Kovaka et al., 2019) and DESeq2 version 1.30.0 (Love et al., 2014) were used in the calculation of raw read counts and identification of differentially expressed genes (DEGs), respectively. Candidate genes with log2FoldChange value > 1 and q value < 0.05 in comparison to the control group were considered as DEGs. The expression level of each gene in each sample was quantified by transcripts per kilobase million (TPM) and was subsequently used in PCA to illustrate the variation of gene expression levels among samples.
Identification of CpG Sites and Genes Involved in Stress Memory
We analyzed DNA methylation variation and gene expression trends over time series to identify mCpGs and gene clusters that were potentially involved in the formation of stress memory. An online platform, OmicShare (https://www.omicshare.com/), was used to perform the analysis. We adapted the category of memory genes defined by Ding et al. (2012) and Georgoulis et al. (2021) and hereon assigned CpG sites and genes involved in the formation of stress memory into five categories: (1) faster-responding sites/genes with more rapid response during the S2 stage; (2) tardy-responding sites/genes with postponed response during the S2 stage; (3) stronger-responding sites/genes with greater amplitude during the S2 stage; (4) early-responding sites/genes that merely responded during the S1 stage; and (5) late-responding sites/genes that merely responded during the S2 stage.
Correlation of DNA Methylation With Corresponding Gene Expression Levels
To interrogate the regulatory role of DNA methylation on gene transcription, we correlated methylation levels of stress-responsive sites with expression levels of corresponding genes using samples from the control group and each of the hyperosmotic treatment groups as well as the recovery group. The Spearman's correlation coefficient was calculated using the R function “cor.test” at the significance level of p < 0.05. The R package “ggplot2” was used to perform linear regression analysis and validate the relationship between methylation levels of significantly correlated stress-responsive sites and corresponding gene expression levels.
Results
Feature-Dependent DNA Methylation of Candidate Genes
We obtained 29,683,794 clean reads per sample on average, among which 6,930,066 reads were mapped to the C. robusta genome (HT version), covering ~89% of the whole genome at a mean depth of 15× coverage. Cytosines in C. robusta genome were methylated in three different sequence contexts, with ~28, 7.8, and 7.9% of all the CpG, CHG, and CHH sites being methylated, respectively.
Overall, 21 out of 30 candidate genes were methylated and the rest nine genes were completely unmethylated, including genes encoding AQPs, ATPase Na+/K+ transporting subunit (NKA_subunit_α and NKA_subunit_β1β2), taurine transporter 1 (TAUT1), serine hydroxymethyltransferase 1 (SHMT1), and pyrroline-5-carboxylate reductase 1.1 (PYCR1.1), suggesting that the regulation of these genes was independent of DNA methylation (Figure 2; Supplementary Table 1).
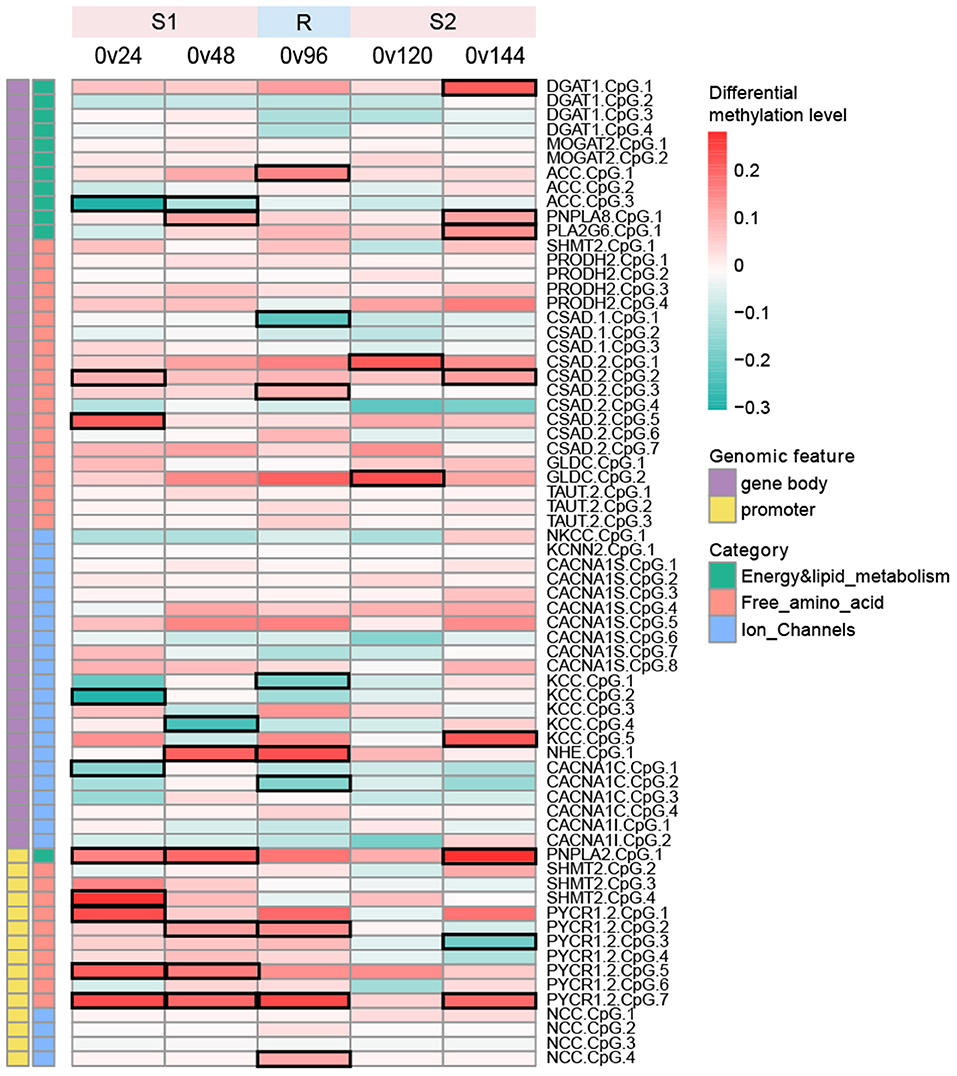
Figure 2. Heatmap depicting differential methylation levels of all the CpG sites between the control group (0 h) and each of the hyperosmotic treatment groups (24/48/120/144 h) as well as the recovery group (96 h). The mean methylation difference of each CpG site between two groups was calculated using weighted read coverage and the statistical significance of methylation differences was determined using the chi-squared test. CpG sites with methylation levels significantly differentiated between two groups (p < 0.05) were considered as stress-responsive sites and were denoted by black frames in the heatmap. Color scales represent differentially methylation levels in the treatment group in comparison with the control group.
We detected a total of 68 mCpGs across 21 methylated genes, with 53 and 15 mCpGs located within gene bodies and promoter regions, respectively (Figure 2; Supplementary Table 1). Methylated sites within each gene ranged from 1 to 8. Specifically, merely 1 mCpG was detected within PNPLA8, PLA2G6, NKCC, KCNN2, NHE, and PNPLA2. In contrast, the gene body of CACNA1S was enriched with 8 methylated sites. The methylation patterns of the 68 CpG sites were bimodal, with pronounced hypo- and hypermethylation peaks around 0–10 and 80–90%, respectively (Supplementary Figure 1). We observed that the majority (20/21) of the genes were methylated either within promoter regions or gene body regions (Figure 2). Three genes, including PYCR1.2, PNPLA2, and NCC, were methylated within promoter regions rather than gene body regions, whereas the rest of the methylated genes (except for SHMT2) was methylated within gene bodies instead of promoter regions, suggesting that DNA methylation of our candidate genes should depend on the genomic feature (i.e., promoter or gene body) (Figure 2).
Stage-Specific Dynamic Response of DNA Methylation
To investigate whether the recurring hyperosmotic challenges induced the differentiation of DNA methylation patterns, PCA was performed using methylation levels of all the CpG sites and stress-responsive CpG sites, respectively (Supplementary Figures 2A–E; Figures 3A–E). The results indicated that DNA methylation patterns could not be evidently differentiated between the control group and the hyperosmotic treatment groups (Supplementary Figures 2A,B,D,E; Figures 3A,B,D,E).
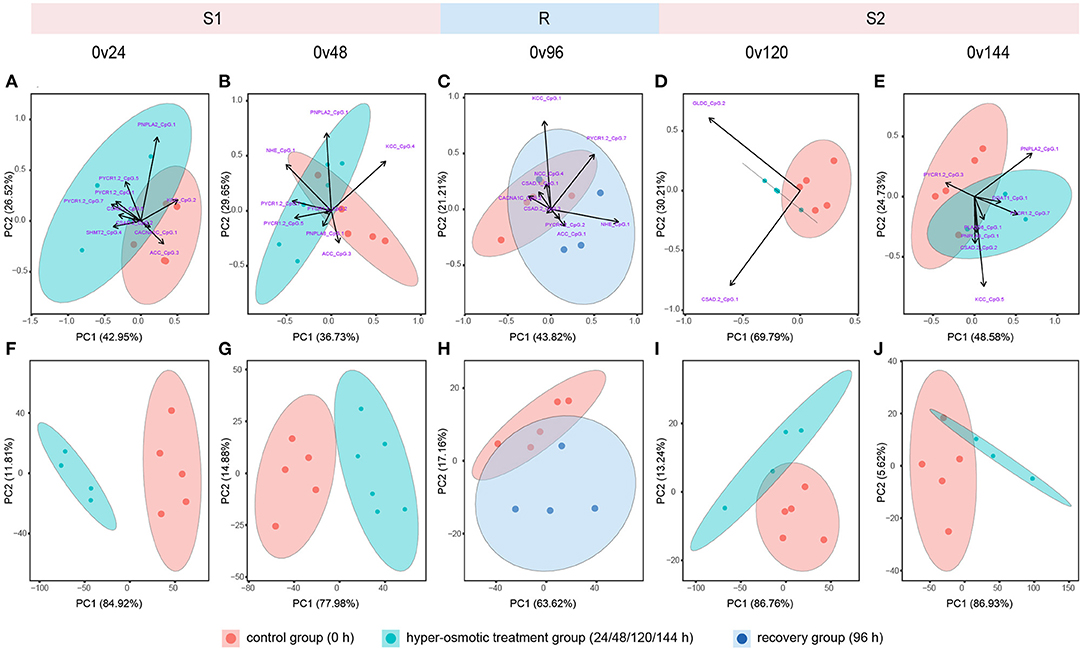
Figure 3. Principal component analysis (PCA) of methylation levels of stress-responsive sites and corresponding gene expression levels. (A–E) Represent PCA based on methylation levels of stress-responsive sites. (F–J) Represent PCA based on transcriptional levels of genes harboring stress-responsive sites. Red, blue, and purple dots indicate samples from the control group (0 h) and the hyperosmotic treatment groups (24/48/120/144 h) as well as the recovery group (96 h), respectively. Each ellipse represents a 95% confidence region.
A total of 26 stress-responsive sites within 14 genes were identified (Figure 2), with eight and 18 stress-responsive sites located within promoter regions and gene bodies, respectively (Figure 2), illustrating a higher proportion of stress-responsive sites within promoter regions (8/18) when compared to gene bodies (18/53). In addition, we identified mCpGs that mutually responded during both the S1 or S2 stages as well as stage-specific mCpGs that merely responded during either S1 or S2 stages. On terms of DMSG, nine genes responded significantly during the S1 stage, among which SHMT2 responded specifically during the S1 stage, whereas the remaining eight genes simultaneously responded during the R or S2 stage (Figure 2). For the S2 stage, a total of 8 DMSGs responded significantly, including three stage-specific responding genes (DGAT1, PLA2G6, and GLDC), and five genes that simultaneously responded (Figure 2). All these results demonstrate that the dynamic responses of CpG methylation were stage specific, suggesting a complex regulatory role of DNA methylation under recurring environmental stresses.
Distinct Transcriptional Responses During the S1 and S2 Stages
Overall, recurring hyperosmotic stresses clearly separated individuals from the control group and the hyperosmotic treatment groups along PC1, accounting for 55.16–69.86% of the variance (Supplementary Figures 2F,G,I,J). Recovery for 24 h (96 h) inclined to restore the overall transcriptional pattern of our candidate genes to that of the ambient condition (Supplementary Figure 2H). For the transcriptional patterns of DMSGs, individuals from the control group and the hyperosmotic treatment groups were clearly separated along PC1, accounting for 77.96–86.93% of the variance (Figures 3F,G,I,J). During the R stage, transcription of DMSGs could not be differentiated along PC1, illustrating transcriptional restoration during the recovery period (Figure 3H).
A total of 13 genes were significantly differentially expressed (Figure 4). A total of five genes responded significantly during both the S1 and S2 stages, among which AQP8.2 and AQP8.3 were significantly downregulated, whereas CSAD1, TAUT2, and MOGAT2 were significantly upregulated, indicating persistent regulation of water channels, metabolism of taurine, and lipid under recurring hyperosmotic stresses (Figure 4). We also detected stage-specific DEGs. Genes encoding aquaporin 9 (AQP9) and glycine decarboxylase (GLDC) significantly responded during the S1 stage, whereas genes encoding potassium/chloride transporter (KCC), serine hydroxymethyltransferase 2 (SHMT2), and phospholipase A2 group 6 (PLA2G6) responded specifically during the S2 stage, implying that distinct mechanisms were involved in rapid response when challenged with environmental stresses for the first time and for the multiple times (Figure 4). Unexpectedly, most of the genes involved in the regulation of ion homeostasis were not identified as DEGs, whereas genes involved in the metabolism of free amino acids such as TAUT, CSAD1, and CSAD2 were significantly differentially expressed, suggesting that the regulation of free amino acids might be a more important mechanism of rapid response during the invasion process (Figure 4).
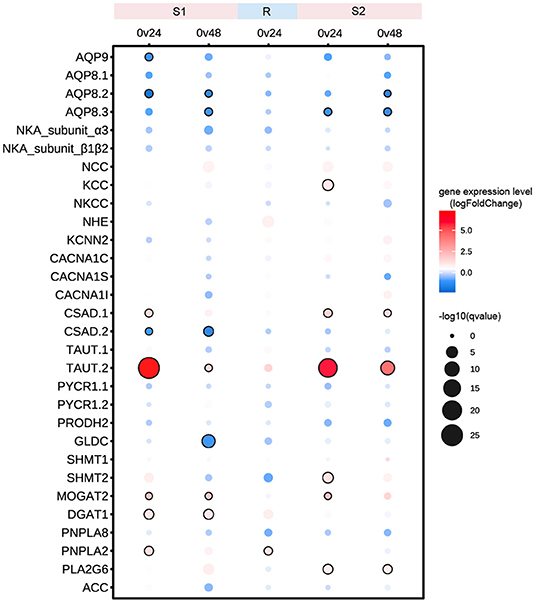
Figure 4. Expression profiles of candidate genes under recurring hyperosmotic stresses. Color scales represent log2FoldChange values in the hyperosmotic treatment groups in comparison with the control group. The size of the circle indicates the statistical significance of gene expression differences between two groups. Black circles denote significantly differentially expressed genes with p < 0.5 and log2FoldChange value > 1.
Time-Dependent Variation of DNA Methylation and Gene Expression Levels
To identify memory methylation sites and memory genes involved in the formation of stress memory, we performed time series analysis based on DNA methylation and gene expression and compared the variation of methylation levels and gene expression levels between the S1 and S2 stages (Figure 5). Time series analysis based on methylation levels identified two early-responding sites and four tardy-responding sites involved in the formation of stress memory (Figures 5A,B). Two early-responding sites, i.e., TAUT1.CpG1 and MOGAT2.CpG1, were upmethylated during the S1 stage, but subsequently returned to the unmethylated status during the R and S2 stages (Figure 5A). For four tardy-responding sites (PYCR1.2.CpG7, PNPLA2.CpG1, CACNA1S.CpG8, and PNPLA8.CpG1), the upmethylation of these sites was postponed by recurring hyperosmotic stresses, suggesting that these four sites might be more insensitive to recurring stresses (Figure 5B).
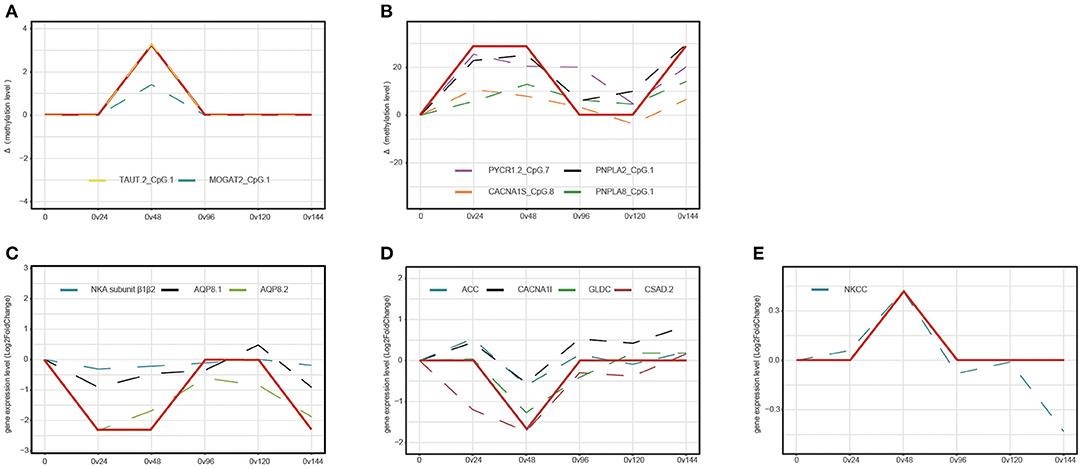
Figure 5. Time series analysis based on DNA methylation level and gene expression levels. The y-axis represents variation of mean methylation levels (A,B) and mean gene expression levels (C–E) between two groups. (A) Early-responding sites that specifically regulated during the S1 stage. (B) Tardy-responding sites with postponed response during the S2 stage. (C) Tardy-responding genes with postponed transcription during the S2 stage. (D,E) Early-responding genes that specifically regulated during the S1 stage. Red solid lines represent the general variation trend of site methylation levels and dashed lines represent DNA methylation variation trend of each mCpG.
At the transcriptional level, we identified eight genes that facilitated the formation of stress memory, including three tardy-responding genes and five early-responding genes (Figures 5C–E). For three tardy-responding genes, i.e., NKA subunit β1β2, AQP8.1, and AQP8.2, the gene expression levels were immediately downregulated after hyperosmotic stress treatment during the S1 stage; however, recurring hyperosmotic stresses postponed the downregulation of these genes during the S2 stage (Figure 5C). Hyperosmotic stress initiated the upregulation of ACC, CACNA1I, GLDC, and CSAD2 and downregulation of NKCC during the S1 stage; however, recurring hyperosmotic stresses did not provoke altered transcriptional patterns of the five early-responding genes during the S2 stage (Figures 5D,E).
Potential Regulatory Role of DNA Methylation on Gene Transcription
To investigate the impact of stress-responsive methylated sites on the transcription of corresponding genes, we eventually identify five significantly correlated sites including ACC.CpG3, CACNA1C.CpG1, PNPLA2.CpG1, DGAT1.CpG1, and PYCR1.2.CpG3 (Figure 6). During the S1 stage, we identified three significantly correlated sites. ACC.CpG3 and CACNA1C.CpG1 located in the introns of ACC and CACNA1C, respectively (Figures 6A,B). These two sites were significantly downmethylated when challenged with hyperosmotic stress for 24 h for the first time, whereas the transcriptional levels of ACC and CACNA1C were significantly upregulated, suggesting the negative correlation between the DNA methylation levels of ACC.CpG3, CACNA1C.CpG1, and the transcription level of corresponding genes (Figures 6A,B). At 48 h during the S1 stage, the DNA methylation level of PNPLA2.CpG1 was positively correlated with the transcription level of PNPLA2 (Figure 6C). The gene expression level of PNPLA2 was upregulated by hyperosmotic stress, whereas the DNA methylation level of PNPLA2.CpG1 varied at a wide range, with that of most samples upregulated in comparison with the control group (Figure 6C).
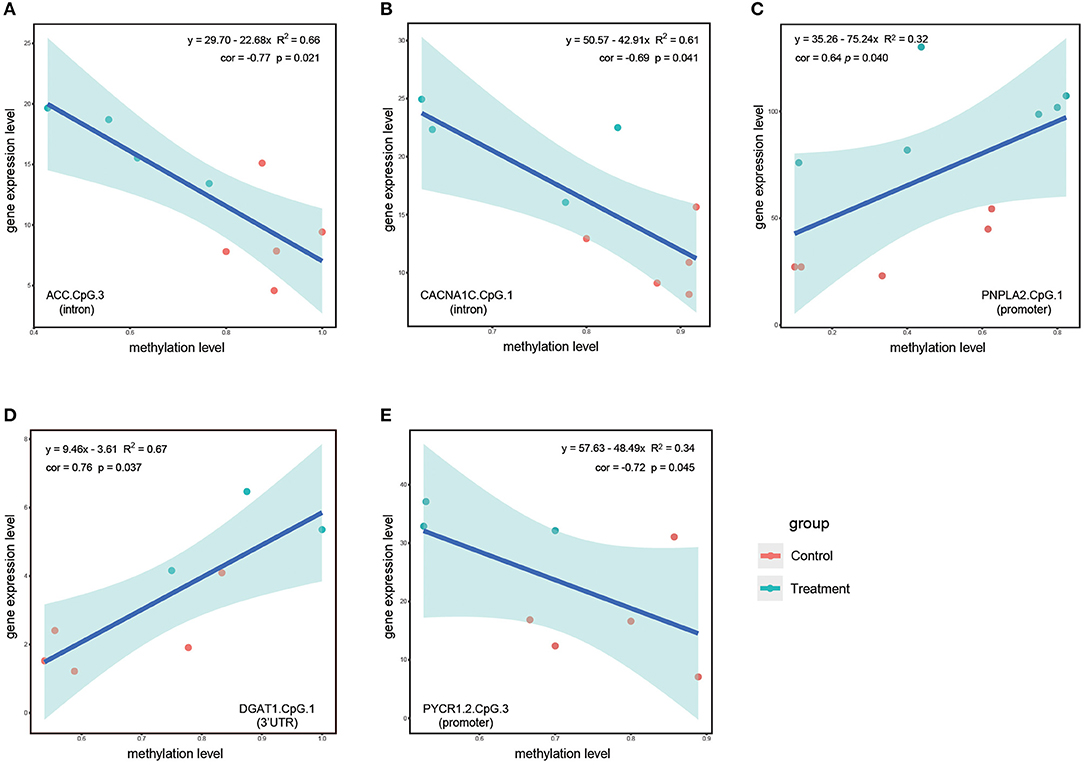
Figure 6. Linear regression analysis based on DNA methylation levels of significantly correlated stress-responsive sites and corresponding gene expression level. (A,B) Represent linear regression analysis based on DNA methylation levels of ACC.CpG.3 and CACNA1C.CpG.1 and corresponding gene expression levels at 24 h during the S1 stage. (C) Represent linear regression analysis based on DNA methylation levels of PNPLA2.CpG.1 and corresponding gene expression levels at 48 h during the S1 stage. (D,E) Represent linear regression analysis based on DNA methylation levels of DGAT1.CpG.1 and PYCR1.2.CpG.1 and corresponding gene expression levels at 48 h during the S2 stage. Genomic features in the parenthesis denote the exact genomic feature of each CpG sites. Red dots denote samples from the control group and turquoise dots denote samples from the treatment groups. The pale turquoise shadow represents a 95% confidence region.
During the S2 stage, we did not detect any significantly correlated sites at 24 h; however, after hyperosmotic stress treatment for a longer time period (48 h), the DNA methylation levels of DGAT1.CpG1 and PYCR1.2.CpG3 were significantly correlated with the transcriptional levels of their corresponding genes (Figures 6D,E). For DGAT1.CpG1 located within 3'UTR of DGAT1, DNA methylation levels of the sites and expression levels of its corresponding gene were upregulated by recurring hyperosmotic stress treatment for 48 h, suggesting a positive correlation between methylation level and gene transcription level (Figure 6D). PYCR1.2.CpG3 was located within promoter region and was in close proximity to transcription start site. In contrast to DAGT1.CpG1, the expression level of PYCR1.2 was inversely correlated with the methylation level of PYCR1.2.CpG3. The methylation level of PYCR1.2.CpG3 was significantly downregulated, whereas the transcriptional level of PYCR1.2 was upregulated, suggesting that downmethylation of PYCR1.2.CpG3 could potentially enhance the proline anabolism under recurring hyperosmotic stress (Figure 6E).
Discussion
Various acute and recurring environmental stresses during range expansions largely threaten the survival of invasive species. Thereby, rapid response to recurring environmental stresses is pivotal to invasion success and may underlie subsequent long-term adaptation. In this study, we investigated the dynamic variation of both the DNA methylation and transcription patterns of key genes involved in response to recurring osmotic stresses, explored the regulatory roles of DNA methylation modification in alteration of gene transcription, and finally tested whether DNA methylation could mediate the formation of stress memory in the invasion process.
Feature-Dependent Distribution of mCpGs
The evolutionary transition of DNA methylation patterns from invertebrates to vertebrates has long been the focus of evolutionary biology. Generally, vertebrate genomes are overall globally and heavily methylated, with CpG sites within promoters and enhancers of housekeeping genes remain unmethylated (Okamura et al., 2010; Keller et al., 2016), whereas invertebrate genomes tend to be sparsely methylated, with methylated regions mostly located within gene bodies and interspersed with unmethylated regions (Keller et al., 2016; Xu et al., 2019). As a result, promoter methylation within invertebrate genomes has been overlooked for a long period of time. Recently, several studies revealed the ubiquitous existence of promoter methylation in invertebrate genomes and further suggested that promoter methylation within invertebrate genomes might be of functional significance (Saint-Carlier and Riviere, 2015; Keller et al., 2016; Xu et al., 2019). In this study, we detected mCpGs within both the promoter and gene body regions, an observation consistent with previous studies (Suzuki et al., 2007; Okamura et al., 2010; Saint-Carlier and Riviere, 2015). Except for SHMT2, CpGs were methylated within either promoter regions or gene bodies, suggesting the feature-dependent distribution of mCpGs within the C. robusta genome. Additionally, promoter regions had a higher proportion of stress-responsive sites (8/15, 53.3%) in comparison with gene bodies (18/53, 34.0%), implying the crucial roles of promoter methylation in response to environmental stresses in invertebrate genomes.
Resilience of Gene Transcription
Akin to ecological resilience, transcriptional resilience has been proposed to depict the recovery of gene expression after a relatively long period of stresses (Seneca and Palumbi, 2015). Seneca and Palumbi (2015) revealed that genes experiencing fast transcriptional recovery were associated with low bleaching in corals and, thus, might contribute to the physiological health and response ability of coral colonies under thermal stresses. For seagrass Zostera marina, thermal stress induced disparate transcription patterns in southern and northern populations that historically inhabited different thermal environments (Franssen et al., 2011). However, the transcriptional pattern of the southern population immediately recovered to that of the ambient condition, whereas the transcriptional level of the northern population failed to recover, suggesting that the resilience of transcriptome could facilitate thermal adaptation of populations that historically inhabited a warmer marine environment (Franssen et al., 2011). In this study, we found that after 48 h of hyperosmotic treatment, the transcriptional pattern during the S1 stage tended to recover to that of the ambient condition in comparison with hyperosmotic treatment for 24 h. Such recovery of gene expression might re-establish the baseline of gene expression and contribute to the maintenance of transcriptional homeostasis and normal cellular function (Seneca and Palumbi, 2015), enhancing the chance of successful invasions.
Methylation of CpG Sites Within Introns of ACC and CACNA1C Inversely Regulated Gene Transcription
The methylation levels of two sites, ACC.CpG3 and CACNA1C.CpG1 located in the introns, were significantly negatively correlated with the expression levels of these 2 genes. Specifically, the DNA methylation levels were significantly downregulated by the hyperosmotic treatment at 24 h; in contrast, the corresponding gene expression levels were upregulated (Figures 6A,B). Introns were proposed as gene regulators, as they had cis-regulatory elements essential for gene expression (Stemmler et al., 2005; Rose, 2019). Unoki and Nakamura (2003) reported that the methylation of intron 1 of EGR2 gene conferred the enhancer-like activity and, thus, might be related to the high level of gene expression. In Arabidopsis, methylation of a large intron in IBM1 orthologs was indispensable for the accumulation of IBM1-L transcript that encodes H3K9 demethylase (Rigal et al., 2012). However, contrasting results were obtained in several species such as European sea bass, where methylation levels of the first intron exhibited a clear inverse relationship with gene expression levels (Anastasiadi et al., 2018). In several diseases, the methylation of intronic CpGs was also validated to be involved in downregulation or silencing of disease-related genes (Zhang et al., 2010; Ozaki et al., 2017; Nam et al., 2020). Thereby, we proposed that hyperosmotic stress induced alterations in DNA methylation status of ACC.CpG3 and CACNA1C.CpG1 and such alterations might interfere with potential gene regulatory elements in proximity to these two sites, resulting in the upregulation of ACC and CACNA1C.
The expression patterns of ACC under hyperosmotic stress differed among species. In red-eared slider turtle, the expression level of ACC was downregulated when exposed to elevated salinities (Hong et al., 2019). In contrast, the upregulation of ACC under hyperosmotic stress was observed in multiple species such as Chanos chanos and Dunaliella salina (Hu et al., 2015; Panahi et al., 2019). In addition, ACC was reported to respond to a wide range of abiotic stresses (Kumar et al., 2017; Wu et al., 2020; Liu et al., 2021). All these results suggest the important and fundamental function of ACC. ACC encodes acetyl-CoA carboxylase, an enzyme that catalyzes the initial rate-limiting step of fatty acid biosynthesis (Ho et al., 2017). Thus, the expression of ACC was indispensable for the synthesis of fatty acid and energy mobilization (Chen et al., 2019). Osmotic stresses could shift fatty acid composition by generating more long-chain and monosaturated fatty acids and some of these fatty acids are susceptible to peroxidation (Reglero et al., 2009; Qureshi et al., 2013). In this study, ACC was upregulated at 24 h during the S1 stage, suggesting an increase in energy demands required for osmoregulation and subsequently enhanced lipid metabolism to meet such demands.
CACNA1C encodes the calcium voltage-gated channel subunit alpha1 C (L-type), which forms the pore of voltage-dependent calcium channel and facilitates the influx of Ca2+ into cells. Calcium ion has been demonstrated to regulate biological processes such as cell motility, membrane lipid peroxidation, cell signaling, muscle contraction, lipid metabolism, gene transcription, and many others (Allen and Beck, 1986; Kader and Lindberg, 2010; Bootman, 2012; Campo et al., 2014; Frouin et al., 2018). The multifunctional nature of calcium ion suggests its potential roles in stress resistance or tolerance. In this study, we observed upregulation of CACNA1C at 24 h during the S1 stage, suggesting the enhanced influx of calcium ions into cells. Increase in the intracellular Ca2+ concentration would regulate downstream biological processes such as cell signaling, muscle contraction, and lipid metabolism, eventually facilitating the rapid response of C. robusta to hyperosmotic stresses.
Methylation of CpG Site Within 3'UTR of DGAT1 Positively Regulated Gene Transcription
In this study, the DNA methylation level of DGAT1.CpG1 was positively correlated with the expression level of DGAT1 at 48 h during the S2 stage. Interestingly, DGAT1.CpG1 was located within the 3'UTR of DGAT1, suggesting that methylation of 3'UTR might be functionally related to its gene transcription. During the evolutionary course of animals, the number of protein coding genes remained relatively consistent between species; however, 3'UTR of messenger RNAs (mRNAs) boosted both in length and number, regulating mRNA translation, stability, localization, and protein–protein interactions (Mayr, 2016, 2019). An empirical study revealed that hypermethylation of 4 CpGs within 3'UTR of the TrkB-T1 gene decreased the activity of luciferase in reporter cells, suggesting that the methylation of 3'UTR was negatively correlated with the TrkB-T1 transcriptional level (Maussion et al., 2014). Studies using omics approaches identified both the repressing and inducing roles of 3'UTR on gene expression in cancers (Beltrami et al., 2017; McGuire et al., 2019). Similarly to introns, 3'UTR was also proposed to possess cis-regulatory elements that affect the transcription, stability, or localization of cognate transcripts. Lau et al. (2010) observed a switch from shorter to longer 3'UTR of the BDNF gene during the activation of hippocampal neurons and longer 3'UTR enabled flexible regulation of transcripts through binding different mRNA-associated proteins. Thereby, we proposed that recurring hyperosmotic stresses could induce alterations in DNA methylation status of DGAT1.CpG1 and such alterations might interfere with potential gene regulatory elements in proximity to the site, resulting in the upregulation of DGAT1.
DGAT1 encodes the diacylglycerol O-acyltransferase 1, which catalyzes the synthesis of triglyceride using diacylglycerol and fatty acyl-CoA as substrates (Liu et al., 2009). DGAT1 is widely expressed in various tissues, with a significantly higher expression level in tissues including skeleton muscle, adipose tissue, and heart (Liu et al., 2009). Overexpression of DGAT1 was verified to increase intramuscular fat deposition (Thaller et al., 2003). Besides its well-known function in hydrolyzing triglyceride into fatty acids, DGAT1 also enables the reversible conversion from hydrolyzed fatty acids into triglyceride, protecting endoplasmic reticulum (ER) from lipotoxic stresses, and related adverse effects (Chitraju et al., 2017). In addition, DGAT1-deficient mice had increased insulin sensitivity, suggesting the potential role of DGAT1 in modulating glucose metabolism (Chen, 2006). In this study, the upregulation of DGAT1 at 48 h during the S2 stage suggests the enhanced triglyceride synthesis that might participate in the protection of ER from lipotoxic stresses and regulation of glucose metabolism. Considering the intertwining role of DGAT1 in various biological processes, the regulatory role of DGAT1 under salinity stresses might be far more complicated than we have realized so far.
Dual Functions of Promoter Methylation in Regulation of Gene Expression
Deoxyribonucleic acid methylation within promoters is well-known for its role in repressing gene transcription (Smith et al., 2020). In a “standard” model, promoter methylation represses gene transcription via either direct interference with the binding of transcription factors or indirect recruitment of chromatin remodeling complexes (Smith et al., 2020). However, such a model has been increasingly challenged, as hypermethylation within promoters has been reported to be entangled with gene activation (Olson and Roberts, 2014; Smith et al., 2020). FOXA2 is a transcription factor that regulates pancreatic gene expression and endoderm development. Smith et al. (2020) demonstrated that the transcription of FOXA2 could be negatively regulated by a CpG island within FOXA2 promoter, hence the methylation of such a CpG island subsequently abolished the repressive effect on gene transcription, suggesting an alternative role of promoter hypermethylation in gene activation. In oysters, promoter regions with a high proportion of methylated sites were discovered in highly expressed genes, suggesting the positive relationship between promoter methylation and gene expression (Olson and Roberts, 2014).
In this study, we detected 2 CpG sites within promoter regions (PNPLA2.CpG1 and PYCR1.2.CpG3) that could serve as potential repressors/facilitators of gene expression. At 48 h during the S1 stage, both the DNA methylation level of PNPLA2.CpG1 and transcription of this gene were upregulated, suggesting that the methylation of PNPLA2.CpG1 could potentially serve as facilitator of gene expression. PNPLA2 encodes patatin-like phospholipase domain containing protein 2, which is the rate-determining enzyme that catalyzes the initial step of triglyceride lipolysis (Janssen et al., 2013). In the human genome, PNPLA2 normally expresses at a low level across tissues, but can be induced by environmental signals (Wilson et al., 2006). In a pufferfish, high salinity stresses induced a significant decline in triglyceride concentration, suggesting that the response to high salinity stresses can be an energy-consuming process and genes responsible for triglyceride catabolism can be upregulated to meet the energy demands (Wen et al., 2021). In line with the aforementioned evidence, the upregulation of PNPLA2 during the S1 stage in this study might satisfy the increased energy consumption under hyperosmotic stresses.
In contrast to PNPLA2.CpG1, the DNA methylation level of PYCR1.2.CpG3 was negatively correlated with its gene expression level. Hence, PYCR1.2.CpG3 might serve as a potential repressor of gene expression. PYCR1.2 is responsible for encoding pyrroline-5-carboxylate reductase 1, an enzyme catalyzing the rate-limiting step of proline synthesis from pyrroline-5-carboxylate (Xiao et al., 2020). Proline has been testified to play essential roles in various biological processes such as osmotic adjustment, redox homeostasis, radical scavenging, and cell signaling (Servet et al., 2012; Patriarca et al., 2021). As a compatible solute, proline enables living cells to adjust osmotic homeostasis and cell volume without overaccumulation of inorganic osmolytes, thus protecting organisms from fluctuations of osmotic pressure. In oysters challenged with hyperosmotic stresses, both the PYCR1 transcription and accumulation of proline were enhanced, suggesting that active transcription of PYCR1 enabled higher accumulation of proline to respond to hyperosmotic stresses (Meng et al., 2013). In consistence with oysters, we detected significant upregulation of PYCR1.2, suggesting the enhanced proline biosynthesis to balance the intracellular and extracellular osmotic potential and counteract cell volume shrinkage under recurring hyperosmotic stresses (Chen and Jiang, 2010; Wiesenthal et al., 2019).
Deoxyribonucleic Acid Methylation and Gene Transcription Facilitated the Formation of Stress Memory
Stress memory was initially discovered in plant species that suffered from frequent drought stresses and those species can remember past adverse environmental cues and alter their responses during subsequent stresses (Ding et al., 2013). Analogous to plant species, invasive species are inevitably and constantly challenged by recurring environmental stresses during the invasion process. Avramova (2015) suggested that different response pathways might be activated during single and repetitive stresses at the molecular level. In this study, we identified stress-responsive sites that mutually responded during both the S1 and S2 stages as well as stage-specific sites that merely responded during either the S1 or S2 stage. Stress-responsive sites that mutually responded during the S1 stage and the S2 stage might play essential and fundamental roles, whereas stage-specific stress-responsive sites might participate in the distinct regulatory mechanisms involved during the S1 and S2 stages.
We identified two early-responding sites and four tardy-responding sites that facilitated the formation of stress memory under recurring hyperosmotic stresses. The two early-responding sites, located within gene bodies of TAUT2 and MOGAT2, might participate in the dynamic regulation of taurine transport and synthesis of diacylglycerol during the S1 stage (Yang and Nickels, 2015; Wen et al., 2018). The four tardy-responding sites were located within promoter regions of PYCR1.2 and PNPLA2 as well as gene bodies of CACNA1S and PNPLA8, suggesting that the regulation of biological processes such as proline anabolism and hydrolysis of triglycerides might be postponed during subsequent recurring stresses (Janssen et al., 2013; Kim et al., 2016; Xiao et al., 2020).
At the transcriptional level, we identified eight memory genes, including three tardy-responding genes and five early-responding genes. For the three tardy-responding genes (NKA subunit β1β2, AQP8.1, and AQP8.2), we observed a postponed downregulation of these candidate genes under recurring hyperosmotic stresses. NKA subunit β1β2 is a molecular chaperone that is crucial for maintaining the structure and regulating the transport properties of NKA subunit α (Geering, 2008). The postponed downregulation of NKA subunit β1β2 during the S2 stage might contribute to a more stable structural stability of NKA subunit α under recurring hyperosmotic stresses. AQPs are well-known for its function as bidirectional transporters of water molecules (Madeira et al., 2016). The transcriptional behaviors of AQPs under hyperosmotic stresses varied between species. For example, the transcription of AQP8 was enhanced in response to salinity acclimation in Anguilla anguilla and Oreochromis mossambicus, whereas contrasting evidence also accumulated in species such as Oreochromis niloticus (Cutler et al., 2009; Ronkin et al., 2015). Such disparate expression profiles were possibly related to the contrasting adaptive environments among species (Ronkin et al., 2015). In this study, we observed postponed downregulation of AQP81 and AQP82 during the S2 stage. Such postponed alterations in transcription could contribute to the postponed regulation of water channels under recurring hyperosmotic stresses. In addition to tardy-responding genes, five early-responding genes that specifically regulated during the S1 stage might stimulate the degradation of glycine and synthesis of fatty acid and taurine and mediate the transport of Na+, K+, Ca2+, and Cl−. Collectively, the memorial behavior of the 6 CpG sites and the transcriptional memory of the eight genes might work in concert, facilitating the formation of stress memory and improving the performance of C. robusta under repetitive stresses. Despite that all the results in this study are obtained in the invasive species, C. robusta, stress memory might also be involved in the plastic response to environmental challenges in a wide range of taxa such as intertidal organisms that experience recurrent environmental stresses daily. Thus, the findings in this study offer new insights into the molecular mechanisms of plastic response widely existing in marine organisms.
Conclusion
In this study, we selected 30 genes potentially involved in rapid response to hyperosmotic stresses to study the regulatory roles of DNA methylation on corresponding gene transcription and further investigated their roles in the formation of stress memory. Among all the 68 CpG sites, significant correlation between DNA methylation and gene expression was detected at only 5 CpG sites, thus challenging the generally accepted regulatory role of DNA methylation on gene expression. To further test this regulatory relationship, genome-wide investigations are needed on more stress response genes and the potentially causal relationship between these two mechanisms should also be experimentally tested in the future study.
At the DNA methylation level, we identified two types of memory sites including two early-responding sites and four tardy-responding sites. At the transcriptional level, we identified two types of memory genes including three tardy-responding memory genes and five early-responding memory genes. The memory of these methylated sites and transcriptional memory of these genes might work in concert to facilitate the formation of stress memory, improving the performance of invasive species under acute and recurring hyperosmotic stresses.
Data Availability Statement
The raw whole genome bisulfite-sequencing data and raw RNA sequencing data were deposited in the National Center for Biotechnology Informaion (NCBI) Sequence Read Archive (SRA) database under the accession number PRJNA775866.
Author Contributions
AZ, RF, and XH conceived the study and designed the experiments. RF conducted the experiment, collected the samples, conducted the rest of the experiments, performed the data analysis, visualized the results, and wrote the manuscript. ZC assisted with data analysis. All authors interpreted the results, contributed to manuscript revisions, and approved the submitted version of the manuscript.
Funding
This study was supported by the National Natural Science Foundation of China under grant numbers 32061143012, 32101352, and 31772449.
Conflict of Interest
The authors declare that the research was conducted in the absence of any commercial or financial relationships that could be construed as a potential conflict of interest.
Publisher's Note
All claims expressed in this article are solely those of the authors and do not necessarily represent those of their affiliated organizations, or those of the publisher, the editors and the reviewers. Any product that may be evaluated in this article, or claim that may be made by its manufacturer, is not guaranteed or endorsed by the publisher.
Acknowledgments
We thank Zunchun Zhou and Bei Jiang for their assistance in the ascidian sample collection and providing experimental sites for acclimation and treatments. We also thank Zhan Laboratory members, YC, Xi Li, and ZC, for their assistance in collection of tissue samples during the animal treatment.
Supplementary Material
The Supplementary Material for this article can be found online at: https://www.frontiersin.org/articles/10.3389/fmars.2021.800745/full#supplementary-material
Supplementary Figure 1. Histogram of CpG methylation levels under ambient condition. The y-axis represents the number of methylated CpG sites and the x-axis represents ranges of methylation levels of each bin. Numbers above bars represent the number of methylated sites within each bin.
Supplementary Figure 2. Principal component analysis based on methylation levels of all the sites and transcriptional levels of all the candidate genes. (A–E) Represent PCA based on methylation levels of all the CpG sites. (F–J) Represent PCA based on transcriptional levels of all the candidate genes. Red, blue, and purple dots indicate samples from the control group, the hyperosmotic treatment groups, and the recovery group, respectively. Each ellipse represents a 95% confidence region.
Supplementary Table 1. Site methylation level.
Abbreviations
mCpGs, methylated CpG sites; Igf2, insulin-like growth factor 2; CTAB, cetyltrimethylammonium bromide; NCBI, national center for biotechnology information; HISAT2, hierarchical indexing for spliced alignment of transcripts 2; CHG, CHG trinucleotide, where H stands for A, T or C; CHH, CHH trinucleotide, where H stands for A, T or C; PNPLA8, patatin like phospholipase domain containing 8; PLA2G6, phospholipase A2 group VI; NKCC, Na-K-Cl cotransporter; KCNN2, potassium calcium-activated channel subfamily N member 2; NHE, sodium-hydrogen exchanger; CACNA1S, calcium voltage-gated channel subunit alpha1 S (L-type); NCC, sodium-chloride transporter; SHMT2, Serine hydroxymethyltransferase 2; DGAT1, diacylglycerol O-acyltransferase 1; GLDC, glycine decarboxylase; CSAD1, cysteinesulfinic acid decarboxylase; MOGAT2, monoacylglycerol O-acyltransferase 2; NKA, Na+-K+-ATPase; ACC, Acetyl-CoA carboxylase; CACNA1I, calcium voltage-gated channel subunit alpha1 I (T-type); CACNA1C, calcium voltage-gated channel subunit alpha1 C (L-type); UTR, untranslated region; EGR2, early growth response protein 2; IBM1, increase in BONSAI methylation 1; BDNF, brain-derived neurotrophic factor; FOXA2, forkhead box A2.
References
Allen, D. O., and Beck, R. R. (1986). Role of calcium ion in hormone-stimulated lipolysis. Biochem. Pharmacol. 35, 767–772. doi: 10.1016/0006-2952(86)90244-3
Anastasiadi, D., Esteve-Codina, A., and Piferrer, F. (2018). Consistent inverse correlation between DNA methylation of the first intron and gene expression across tissues and species. Epigenet. Chromatin. 11, 1–17. doi: 10.1186/s13072-018-0205-1
Avramova, Z. (2015). Transcriptional ‘memory’ of a stress: transient chromatin and memory (epigenetic) marks at stress-response genes. Plant J. 83, 149–159. doi: 10.1111/tpj.12832
Beltrami, C. M., dos Reis, M. B., Barros-Filho, M. C., Marchi, F. A., Kuasne, H., Pinto, C. A. L., et al. (2017). Integrated data analysis reveals potential drivers and pathways disrupted by DNA methylation in papillary thyroid carcinomas. Clin. Epigenet. 9, 1–11. doi: 10.1186/s13148-017-0346-2
Bolger, A. M., Lohse, M., and Usadel, B. (2014). Trimmomatic: a flexible trimmer for illumina sequence data. Bioinformatics 30, 2114–2120. doi: 10.1093/bioinformatics/btu170
Bootman, M. D. (2012). Calcium signaling. CSH Perspect. Biol. 4:a011171. doi: 10.1101/cshperspect.a011171
Briski, E., Bailey, S. A., Casas-Monroy, O., DiBacco, C., Kaczmarska, I., Lawrence, J. E., et al. (2013). Taxon- and vector-specific variation in species richness and abundance during the transport stage of biological invasions. Limnol. Oceanogr. 58, 1361–1372. doi: 10.4319/lo.2013.58.4.1361
Campo, S., Baldrich, P., Messeguer, J., Lalanne, E., Coca, M., and San Segundo, B. (2014). Overexpression of a calcium-dependent protein kinase confers salt and drought tolerance in rice by preventing membrane lipid peroxidation. Plant Physiol. 165, 688–704. doi: 10.1104/pp.113.230268
Caputi, L., Andreakis, N., Mastrototaro, F., Cirino, P., Vassillo, M., and Sordino, P. (2007). Cryptic speciation in a model invertebrate chordate. Proc. Natl. Acad. Sci. U. S. A. 104, 9364–9369. doi: 10.1073/pnas.0610158104
Chen, D., Yuan, X., Liang, L. M., Liu, K., Ye, H. Y., Liu, Z., et al. (2019). Overexpression of acetyl-CoA carboxylase increases fatty acid production in the green alga Chlamydomonas reinhardtii. Biotechnol. Lett. 41, 1133–1145. doi: 10.1007/s10529-019-02715-0
Chen, H., and Jiang, J. G. (2010). Osmotic adjustment and plant adaptation to environmental changes related to drought and salinity. Environ. Rev. 18, 309–319. doi: 10.1139/A10-014
Chen, H. C. (2006). Enhancing energy and glucose metabolism by disrupting triglyceride synthesis: lessons from mice lacking DGAT1. Nutr. Metab. 3, 1–4. doi: 10.1186/1743-7075-3-10
Chen, Y. Y., Shenkar, N., Ni, P., Lin, Y. P., Li, S. G., and Zhan, A. B. (2018). Rapid microevolution during recent range expansion to harsh environments. BMC Evol. Biol. 18, 1–13. doi: 10.1186/s12862-018-1311-1
Chitraju, C., Mejhert, N., Haas, J. T., Diaz-Ramirez, L. G., Grueter, C. A., Imbriglio, J. E., et al. (2017). Triglyceride synthesis by DGAT1 protects adipocytes from lipid-induced ER stress during lipolysis. Cell Metab. 26, 407–418. doi: 10.1016/j.cmet.2017.07.012
Cutler, C. P., Philips, C., Hazon, N., and Cramb, G. (2009). Aquaporin 8 (AQP8) intestinal mRNA expression increases in response to salinity acclimation in yellow and silver European eels (Anguilla anguilla). Comp. Biochem. Physiol. A 153:S78. doi: 10.1016/j.cbpa.2009.04.047
Ding, Y., Fromm, M., and Avramova, Z. (2012). Multiple exposures to drought ‘train’ transcriptional responses in Arabidopsis. Nat. Commun. 3, 1–9. doi: 10.1038/ncomms1732
Ding, Y., Liu, N., Virlouvet, L., Riethoven, J. J., Fromm, M., and Avramova, Z. (2013). Four distinct types of dehydration stress memory genes in Arabidopsis thaliana. BMC Plant Biol. 13, 1–11. doi: 10.1186/1471-2229-13-229
Epelbaum, A., Herborg, L. M., Therriault, T. W., and Pearce, C. M. (2009). Temperature and salinity effects on growth, survival, reproduction, and potential distribution of two non-indigenous Botryllid ascidians in British Columbia. J. Exp. Biol. Ecol. 369, 43–52. doi: 10.1016/j.jembe.2008.10.028
Foret, S., Kucharski, R., Pellegrini, M., Feng, S., Jacobsen, S. E., Robinson, G. E., et al. (2012). DNA methylation dynamics, metabolic fluxes, gene splicing, and alternative phenotypes in honey bees. Proc. Natl. Acad. Sci. U. S. A. 109, 4968–4973. doi: 10.1073/pnas.1202392109
Franssen, S. U., Gu, J., Bergmann, N., Winters, G., Klostermeier, U. C., Rosenstiel, P., et al. (2011). Transcriptomic resilience to global warming in the seagrass Zostera marina, a marine foundation species. Proc Natl. Acad. Sci. U. S. A. 108, 19276–19281. doi: 10.1073/pnas.1107680108
Frouin, J., Languillaume, A., Mas, J., Mieulet, D., Boisnard, A., Labeyrie, A., et al. (2018). Tolerance to mild salinity stress in japonica rice: a genome-wide association mapping study highlights calcium signaling and metabolism genes. PLoS ONE 13:e0190964. doi: 10.1371/journal.pone.0190964
Fu, R. Y., Huang, X. N., and Zhan, A. B. (2021). Identification of DNA (de) methylation-related genes and their transcriptional response to environmental challenges in an invasive model ascidian. Gene 768:145331. doi: 10.1016/j.gene.2020.145331
Geering, K. (2008). Functional roles of Na, K-ATPase subunits. Curr. Opin. Nephrol. Hy. 17, 526–532. doi: 10.1097/MNH.0b013e3283036cbf
Georgoulis, I., Feidantsis, K., Giantsis, I. A., Kakale, A., Bock, C., Pörtner, H. O., et al. (2021). Heat hardening enhances mitochondrial potential for respiration and oxidative defence capacity in the mantle of thermally stressed Mytilus galloprovincialis. Sci. Rep. 11, 1–18. doi: 10.1038/s41598-021-96617-9
Gollasch, S., and David, M. (2021). Abiotic and biological differences in ballast water uptake and discharge samples. Mar. Pollut. Bull. 164:112046. doi: 10.1016/j.marpolbul.2021.112046
Halpern, K. B., Vana, T., and Walker, M. D. (2014). Paradoxical role of DNA methylation in activation of FoxA2 gene expression during endoderm development. J. Biol. Chem. 289, 23882–23892. doi: 10.1074/jbc.M114.573469
Heckwolf, M. J., Meyer, B. S., Häsler, R., Höppner, M. P., Eizaguirre, C., and Reusch, T. B. (2020). Two different epigenetic information channels in wild three-spined sticklebacks are involved in salinity adaptation. Sci. Adv. 6:eaaz1138. doi: 10.1126/sciadv.aaz1138
Ho, S. H., Nakanishi, A., Kato, Y., Yamasaki, H., Chang, J. S., Misawa, N., et al. (2017). Dynamic metabolic profiling together with transcription analysis reveals salinity-induced starch-to-lipid biosynthesis in alga Chlamydomonas sp. JSC4. Sci. Rep. 7, 1–11. doi: 10.1038/srep45471
Hong, M. L., Li, N., Li, J. Y., Li, W. H., Liang, L. Y., Li, Q., et al. (2019). Adenosine monophosphate-activated protein kinase signaling regulates lipid metabolism in response to salinity stress in the red-eared slider turtle Trachemys scripta elegans. Front. Physiol. 10:962. doi: 10.3389/fphys.2019.00962
Hu, J., Askary, A. M., Thurman, T. J., Spiller, D. A., Palmer, T. M., Pringle, R. M., et al. (2019). The epigenetic signature of colonizing new environments in Anolis lizards. Mol. Biol. Ebol. 36, 2165–2170. doi: 10.1093/molbev/msz133
Hu, Y. C., Kang, C. K., Tang, C. H., and Lee, T. H. (2015). Transcriptomic analysis of metabolic pathways in milkfish that respond to salinity and temperature changes. PLoS ONE 10:e0134959. doi: 10.1371/journal.pone.0134959
Huang, X. N., Li, S. G., Ni, P., Gao, Y. C., Jiang, B., Zhou, Z. C., et al. (2017). Rapid response to changing environments during biological invasions: DNA methylation perspectives. Mol. Ecol. 26, 6621–6633. doi: 10.1111/mec.14382
Huang, X. N., and Zhan, A. B. (2021). Highly dynamic transcriptional reprogramming and shorter isoform shifts under acute stresses during biological invasions. RNA Biol. 18, 340–353. doi: 10.1080/15476286.2020.1805904
Ibrahim, A. M., and El-Naggar, M. M. (2012). Ballast water review: impacts, treatments and management. Middle-East J. Sci. Res. 12, 976–984. doi: 10.5829/idosi.mejsr.2012.12.7.7131
Janssen, M. C., Van Engelen, B., Kapusta, L., Lammens, M., Van Dijk, M., Fischer, J., et al. (2013). Symptomatic lipid storage in carriers for the PNPLA2 gene. Eur. J. Hum. Genet. 21, 807–815. doi: 10.1038/ejhg.2012.256
Kader, M. A., and Lindberg, S. (2010). Cytosolic calcium and pH signaling in plants under salinity stress. Plant Signal. Behav. 5, 233–238. doi: 10.4161/psb.5.3.10740
Keller, T. E., Han, P., and Yi, S. V. (2016). Evolutionary transition of promoter and gene body DNA methylation across invertebrate-vertebrate boundary. Mol. Biol. Ebol. 33, 1019–1028. doi: 10.1093/molbev/msv345
Kim, D., Paggi, J. M., Park, C., Bennett, C., and Salzberg, S. L. (2019). Graph-based genome alignment and genotyping with HISAT2 and HISAT-genotype. Nat. Biotechnol. 37, 907–915. doi: 10.1038/s41587-019-0201-4
Kim, K. Y., Jang, H. J., Yang, Y. R., Park, K. I., Seo, J., Shin, I. W., et al. (2016). SREBP-2/PNPLA8 axis improves non-alcoholic fatty liver disease through activation of autophagy. Sci. Rep. 6, 1–14. doi: 10.1038/srep35732
Kou, S. Y., Gu, Q. Y., Duan, L., Liu, G. J., Yuan, P. R., Li, H. H., et al. (2021). Genome-wide bisulphite sequencing uncovered the contribution of DNA methylation to rice short-term drought memory formation. J. Plant Growth Regul. 3, 1–15. doi: 10.1007/s00344-021-10483-3
Kovaka, S., Zimin, A. V., Pertea, G. M., Razaghi, R., Salzberg, S. L., and Pertea, M. (2019). Transcriptome assembly from long-read RNA-seq alignments with StringTie2. Genome Biol. 20, 1–13. doi: 10.1186/s13059-019-1910-1
Krueger, F., and Andrews, S. R. (2011). Bismark: a flexible aligner and methylation caller for Bisulfite-Seq applications. Bioinformatics 27, 1571–1572. doi: 10.1093/bioinformatics/btr167
Kumar, R., Biswas, K., Singh, P. K., Singh, P. K., Elumalai, S., Shukla, P., et al. (2017). Lipid production and molecular dynamics simulation for regulation of accD gene in cyanobacteria under different N and P regimes. Biotechnol. Biofuels 10, 1–14. doi: 10.1186/s13068-017-0776-2
Lau, A. G., Irier, H. A., Gu, J., Tian, D., Ku, L., Liu, G., et al. (2010). Distinct 3′ UTRs differentially regulate activity-dependent translation of brain-derived neurotrophic factor (BDNF). Proc. Natl. Acad. Sci. U. S. A. 107, 15945–15950. doi: 10.1073/pnas.1002929107
Li, H., Yang, X. Y., Wang, Q. F., Chen, J. M., and Shi, T. (2021). Distinct methylome patterns contribute to ecotypic differentiation in the growth of the storage organ of a flowering plant (sacred lotus). Mol. Ecol. 30, 2831–2845. doi: 10.1111/mec.15933
Li, H. X., Huang, X. N., and Zhan, A. B. (2020). Stress memory of recurrent environmental challenges in marine invasive species: Ciona robusta as a case study. Front. Physiol. 11:94. doi: 10.3389/fphys.2020.00094
Li, Y., Liew, Y. J., Cui, G., Cziesielski, M. J., Zahran, N., Michell, C. T., et al. (2018). DNA methylation regulates transcriptional homeostasis of algal endosymbiosis in the coral model Aiptasia. Sci. Adv. 4:eaat2142. doi: 10.1126/sciadv.aat2142
Liew, Y. J., Howells, E. J., Wang, X., Michell, C. T., Burt, J. A., Idaghdour, Y., et al. (2020). Intergenerational epigenetic inheritance in reef-building corals. Nat. Clim. Change 10, 254–259. doi: 10.1038/s41558-019-0687-2
Liu, L., Shi, X. J., Bharadwaj, K. G., Ikeda, S., Yamashita, H., Yagyu, H., et al. (2009). DGAT1 expression increases heart triglyceride content but ameliorates lipotoxicity. J. Biol. Chem. 284, 36312–36323. doi: 10.1074/jbc.M109.049817
Liu, Y., Wen, H. S., Qi, X., Zhang, X. Y., Zhang, K. Q., Fan, H. Y., et al. (2019). Genome-wide identification of the Na+/H+ exchanger gene family in Lateolabrax maculatus and its involvement in salinity regulation. Comp. Biochem. Physiol. D 29, 286–298. doi: 10.1016/j.cbd.2019.01.001
Liu, Z. F., Ma, A. J., Yuan, C. H., Zhao, T. T., Chang, H. W., and Zhang, J. S. (2021). Transcriptome analysis of liver lipid metabolism disorders of the turbot Scophthalmus maximus in response to low salinity stress. Aquaculture 534:736273. doi: 10.1016/j.aquaculture.2020.736273
Love, M. I., Huber, W., and Anders, S. (2014). Moderated estimation of fold change and dispersion for RNA-seq data with DESeq2. Genome Biol. 15, 1–21. doi: 10.1186/s13059-014-0550-8
Madeira, A., Moura, T. F., and Soveral, G. (2016). Detecting aquaporin function and regulation. Front. Chem. 4:3. doi: 10.3389/fchem.2016.00003
Maussion, G., Yang, J., Suderman, M., Diallo, A., Nagy, C., Arnovitz, M., et al. (2014). Functional DNA methylation in a transcript specific 3′ UTR region of TrkB associates with suicide. Epigenetics 9, 1061–1070. doi: 10.4161/epi.29068
Mayr, C. (2016). Evolution and biological roles of alternative 3′ UTRs. Trends Cell Biol. 26, 227–237. doi: 10.1016/j.tcb.2015.10.012
Mayr, C. (2019). What are 3′ UTRs doing?. CSH Perspect. Biol. 11:a034728. doi: 10.1101/cshperspect.a034728
McGuire, M. H., Herbrich, S. M., Dasari, S. K., Wu, S. Y., Wang, Y., Rupaimoole, R., et al. (2019). Pan-cancer genomic analysis links 3'UTR DNA methylation with increased gene expression in T cells. EBioMedicine 43, 127–137. doi: 10.1016/j.ebiom.2019.04.045
Meng, J., Zhu, Q. H., Zhang, L. L., Li, C. Y., Li, L., She, Z. C., et al. (2013). Genome and transcriptome analyses provide insight into the euryhaline adaptation mechanism of Crassostrea gigas. PLoS ONE 8:e58563. doi: 10.1371/journal.pone.0058563
Murrell, A., Heeson, S., Bowden, L., Constância, M., Dean, W., Kelsey, G., et al. (2001). An intragenic methylated region in the imprinted Igf2 gene augments transcription. EMBO Rep. 2, 1101–1106. doi: 10.1093/embo-reports/kve248
Nam, A. R., Lee, K. H., Hwang, H. J., Schabort, J. J., An, J. H., Won, S. H., et al. (2020). Alternative methylation of intron motifs is associated with cancer-related gene expression in both canine mammary tumor and human breast cancer. Clin. Epigenet. 12, 1–15. doi: 10.1186/s13148-020-00888-4
Neri, F., Rapelli, S., Krepelova, A., Incarnato, D., Parlato, C., Basile, G., et al. (2017). Intragenic DNA methylation prevents spurious transcription initiation. Nature 543, 72–77. doi: 10.1038/nature21373
Okamura, K., Matsumoto, K. A., and Nakai, K. (2010). Gradual transition from mosaic to global DNA methylation patterns during deuterostome evolution. BMC Bioinformat. 11:S2. doi: 10.1186/1471-2105-11-S7-S2
Olson, C. E., and Roberts, S. B. (2014). Genome-wide profiling of DNA methylation and gene expression in Crassostrea gigas male gametes. Front. Physiol. 5:224. doi: 10.3389/fphys.2014.00224
Ozaki, Y., Yoshino, Y., Yamazaki, K., Sao, T., Mori, Y., Ochi, S., et al. (2017). DNA methylation changes at TREM2 intron 1 and TREM2 mRNA expression in patients with Alzheimer's disease. J. Psychiatr. Res. 92, 74–80. doi: 10.1016/j.jpsychires.2017.04.003
Panahi, B., Frahadian, M., Dums, J. T., and Hejazi, M. A. (2019). Integration of cross species RNA-seq meta-analysis and machine-learning models identifies the most important salt stress–responsive pathways in microalga Dunaliella. Front. Genet. 10:752. doi: 10.3389/fgene.2019.00752
Patriarca, E. J., Cermola, F., D'Aniello, C., Fico, A., Guardiola, O., De Cesare, D., et al. (2021). The multifaceted roles of proline in cell behavior. Front. Cell Dev. Biol. 9:728576. doi: 10.3389/fcell.2021.728576
Pu, C. L., and Zhan, A. B. (2017). Epigenetic divergence of key genes associated with water temperature and salinity in a highly invasive model ascidian. Biol. Invasions 19, 2015–2028. doi: 10.1007/s10530-017-1409-1
Qureshi, M. I., Abdin, M. Z., Ahmad, J., and Iqbal, M. (2013). Effect of long-term salinity on cellular antioxidants, compatible solute and fatty acid profile of Sweet Annie (Artemisia annua L.). Phytochemistry 95, 215–223. doi: 10.1016/j.phytochem.2013.06.026
Reglero, M. M., Taggart, M. A., Monsalve-Gonzalez, L., and Mateo, R. (2009). Heavy metal exposure in large game from a lead mining area: effects on oxidative stress and fatty acid composition in liver. Environ. Pollut. 157, 1388–1395. doi: 10.1016/j.envpol.2008.11.036
Rigal, M., Kevei, Z., Pélissier, T., and Mathieu, O. (2012). DNA methylation in an intron of the IBM1 histone demethylase gene stabilizes chromatin modification patterns. EMBO J. 31, 2981–2993. doi: 10.1038/emboj.2012.141
Ronkin, D., Seroussi, E., Nitzan, T., Doron-Faigenboim, A., and Cnaani, A. (2015). Intestinal transcriptome analysis revealed differential salinity adaptation between two tilapiine species. Comp. Biochem. Physiol. D 13, 35–43. doi: 10.1016/j.cbd.2015.01.003
Rose, A. B. (2019). Introns as gene regulators: a brick on the accelerator. Front. Genet. 9:672. doi: 10.3389/fgene.2018.00672
Saint-Carlier, E., and Riviere, G. (2015). Regulation of Hox orthologues in the oyster Crassostrea gigas evidences a functional role for promoter DNA methylation in an invertebrate. FEBS Lett. 589, 1459–1466. doi: 10.1016/j.febslet.2015.04.043
Seneca, F. O., and Palumbi, S. R. (2015). The role of transcriptome resilience in resistance of corals to bleaching. Mol. Ecol. 24, 1467–1484. doi: 10.1111/mec.13125
Servet, C., Ghelis, T., Richard, L., Zilberstein, A., and Savoure, A. (2012). Proline dehydrogenase: a key enzyme in controlling cellular homeostasis. Front Biosci. 17, 607–620. doi: 10.2741/3947
Shenkar, N., Shmuel, Y., and Huchon, D. (2017). The invasive ascidian Ciona robusta recorded from a Red Sea marina. Mar. Biodiv. 48, 2211–2214. doi: 10.1007/s12526-017-0699-y
Smith, J., Sen, S., Weeks, R. J., Eccles, M. R., and Chatterjee, A. (2020). Promoter DNA hypermethylation and paradoxical gene activation. Trends Cancer 6, 392–406. doi: 10.1016/j.trecan.2020.02.007
Stemmler, M. P., Hecht, A., and Kemler, R. (2005). E-cadherin intron 2 contains cis-regulatory elements essential for gene expression. Development 132, 965–976. doi: 10.1242/dev.01662
Sun, R. Z., Liu, J., Wang, Y. Y., and Deng, X. (2021). DNA methylation-mediated modulation of rapid desiccation tolerance acquisition and dehydration stress memory in the resurrection plant Boea hygrometrica. PLoS Genet. 17:e1009549. doi: 10.1371/journal.pgen.1009549
Suzuki, M. M., Kerr, A. R., De Sousa, D., and Bird, A. (2007). CpG methylation is targeted to transcription units in an invertebrate genome. Genome Res. 17, 625–631. doi: 10.1101/gr.6163007
Thaller, G., Kühn, C., Winter, A., Ewald, G., Bellmann, O., Wegner, J., et al. (2003). DGAT1, a new positional and functional candidate gene for intramuscular fat deposition in cattle. Anim. Genet. 34, 354–357. doi: 10.1046/j.1365-2052.2003.01011.x
Towle, D. W., Henry, R. P., and Terwilliger, N. B. (2011). Microarray-detected changes in gene expression in gills of green crabs (Carcinus maenas) upon dilution of environmental salinity. Comp. Biochem. Physiol. D 6, 115–125. doi: 10.1016/j.cbd.2010.11.001
Unoki, M., and Nakamura, Y. (2003). Methylation at CpG islands in intron 1 of EGR2 confers enhancer-like activity. FEBS Lett. 554, 67–72. doi: 10.1016/S0014-5793(03)01092-5
Wen, C. Y., Li, F. N., Zhang, L. Y., Duan, Y. H., Guo, Q. P., Wang, W. L., et al. (2018). Taurine is involved in energy metabolism in muscle, adipose tissue, and liver. Mol. Nutr. Food Res. 63:e1800536. doi: 10.1002/mnfr.201800536
Wen, X., Chu, P., Xu, J. J., Wei, X. Z., Fu, D. Y., Wang, T., et al. (2021). Combined effects of low temperature and salinity on the immune response, antioxidant capacity and lipid metabolism in the pufferfish (Takifugu fasciatus). Aquaculture 531:735866. doi: 10.1016/j.aquaculture.2020.735866
Wiesenthal, A. A., Müller, C., Harder, K., and Hildebrandt, J. P. (2019). Alanine, proline and urea are major organic osmolytes in the snail Theodoxus fluviatilis under hyperosmotic stress. J. Exp. Biol. 222:jeb193557. doi: 10.1242/jeb.193557
Wilson, P. A., Gardner, S. D., Lambie, N. M., Commans, S. A., and Crowther, D. J. (2006). Characterization of the human patatin-like phospholipase familys. J. Lipid Res. 47, 1940–1949. doi: 10.1194/jlr.M600185-JLR200
Wu, D. L., Liu, Z. Q., Yu, P., Huang, Y. H., Cai, M. Q., Zhang, M., et al. (2020). Cold stress regulates lipid metabolism via AMPK signalling in Cherax quadricarinatus. J. Therm. Biol. 92:102693. doi: 10.1016/j.jtherbio.2020.102693
Xiao, S. Y., Li, S. Z., Yuan, Z. Y., and Zhou, L. Y. (2020). Pyrroline-5-carboxylate reductase 1 (PYCR1) upregulation contributes to gastric cancer progression and indicates poor survival outcome. Ann. Transl. Med. 8:4402. doi: 10.21037/atm-19-4402
Xie, H. J., Han, Y. H., Li, X. Y., Dai, W. M., Song, X. L., Olsen, K. M., et al. (2020). Climate-dependent variation in cold tolerance of weedy rice and rice mediated by OsICE1 promoter methylation. Mol. Ecol. 29, 121–137. doi: 10.1111/mec.15305
Xie, H. J., Sun, Y. L., Cheng, B., Xue, S. M., Cheng, D., Liu, L. L., et al. (2019). Variation in ICE1 methylation primarily determines phenotypic variation in freezing tolerance in Arabidopsis Thaliana. Plant Cell Physiol. 60, 152–165. doi: 10.1093/pcp/pcy197
Xu, X. C., Li, G. Q., Li, C. R., Zhang, J., Wang, Q., Simmons, D. K., et al. (2019). Evolutionary transition between invertebrates and vertebrates via methylation reprogramming in embryogenesis. Natl. Sci. Rev. 6, 993–1003. doi: 10.1093/nsr/nwz064
Yang, M. H., and Nickels, J. T. (2015). MOGAT2: a new therapeutic target for metabolic syndrome. Diseases 3, 176–192. doi: 10.3390/diseases3030176
Zhan, A. B., Ni, P., Xiong, W., Chen, Y. Y., Lin, Y. P., Huang, X. N., et al. (2017). “Biological invasions in aquatic ecosystems in China,” in Biological Invasions and Its Management in China, eds F. H. Wan, M. X. Jiang, and A. B. Zhan (Dordrecht: Springer), 67–96. doi: 10.1007/978-94-024-0948-2_4
Keywords: biological invasion, DNA methylation, gene transcription, rapid response, stress memory
Citation: Fu R, Huang X, Chen Y, Chen Z and Zhan A (2021) Interactive Regulations of Dynamic Methylation and Transcriptional Responses to Recurring Environmental Stresses During Biological Invasions. Front. Mar. Sci. 8:800745. doi: 10.3389/fmars.2021.800745
Received: 25 October 2021; Accepted: 19 November 2021;
Published: 21 December 2021.
Edited by:
Taewoo Ryu, Okinawa Institute of Science and Technology Graduate University, JapanReviewed by:
Yi Liao, University of California, Irvine, United StatesBenoît Chénais, Le Mans Université, France
Copyright © 2021 Fu, Huang, Chen, Chen and Zhan. This is an open-access article distributed under the terms of the Creative Commons Attribution License (CC BY). The use, distribution or reproduction in other forums is permitted, provided the original author(s) and the copyright owner(s) are credited and that the original publication in this journal is cited, in accordance with accepted academic practice. No use, distribution or reproduction is permitted which does not comply with these terms.
*Correspondence: Aibin Zhan, emhhbmFpYmluQGhvdG1haWwuY29t; YXpoYW5AcmNlZXMuYWMuY24=