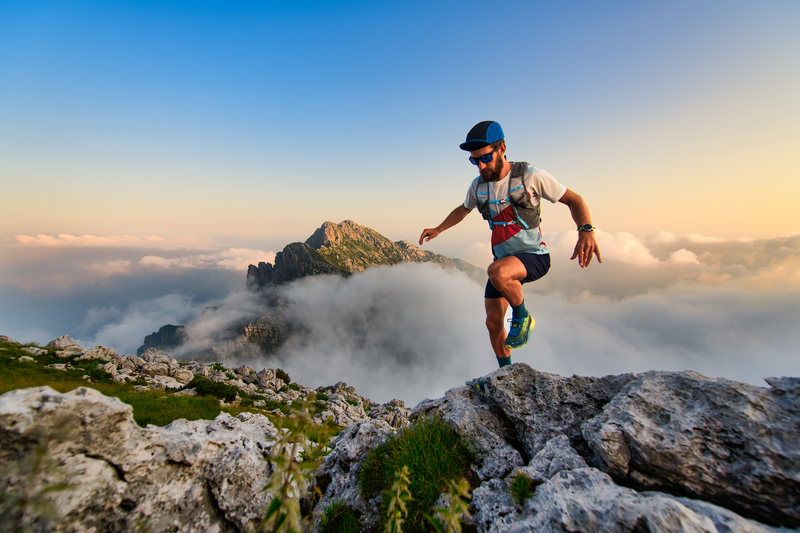
94% of researchers rate our articles as excellent or good
Learn more about the work of our research integrity team to safeguard the quality of each article we publish.
Find out more
ORIGINAL RESEARCH article
Front. Mamm. Sci. , 18 December 2023
Sec. Life History and Conservation
Volume 2 - 2023 | https://doi.org/10.3389/fmamm.2023.1243883
Most research on mammalian resting and sleep behavior is conducted under controlled laboratory or zoo settings, with limited studies taking place in their natural habitats. To analyze these behaviors within a natural setting, actigraphy has been identified as a valuable method. This study sought to, firstly, measure inactivity and sleep in free-roaming Angolan giraffe (Giraffa giraffa angolensis) and, secondly, present their nocturnal behavioral rhythmicity. Three giraffe were fitted with an accelerometer-GPS device attached to an ossicone in November 2018 and behavioral data were collected daily between December 2018 and April 2019 to record their cranial movement. As giraffe show behavior specific head movements, sleep events could be detected as well as active and inactive behavior patterns. In this study, we analyzed how long giraffe were inactive during the night, how many sleep events they showed and for how long they were in the sleep position during the night. Giraffe were inactive for 490.8 ± 43.7 minutes during the night (from 19:00 to 7:00 local time), and a total of 857 sleep events were recorded with an average of 2.0 ± 1.0 events per night and total length of sleep per night averaged 8.6 ± 7.9 minutes. Further, results show a clear biphasic sleep profile during the night with peaks of inactivity and sleep events during the hours after sunset (21:00–23:00) and during the early morning hours before sunrise (3:00–5:00). We found individual differences for the number or sleep events, the total time sleeping as well as for inactivity. Interestingly, differences between months only were detected for inactivity. No differences were found for the number of sleep events nor for the total sleep time per night between months. For the first time, this study sheds light on the nocturnal sleep behavior and biphasic inactivity rhythm of giraffe in their natural habitat, providing additional support for previous findings indicating brief sleep episodes in giraffe.
Biodiversity conservation has gained significant attention in modern society, fueled by concerns about a potential sixth mass extinction. Biologists warn that we might be on the brink of another mass extinction event, given the known species losses in recent centuries and millennia (Barnosky et al., 2011). Besides plants and invertebrates, 18% of vertebrates and 22% of mammals that have been assessed are at risk (IUCN, 2020). Some of these threatened species include giraffe (Giraffa spp.) as the combined wild population has declined dramatically by ~30% across their range in Africa over the last 35 years (Brown et al., 2021). As a result of this decline, the International Union for Conservation of Nature (IUCN) Red List classified giraffe as “vulnerable” (Muller et al., 2018; O’Connor et al., 2019; Brown et al., 2021). More specifically, three of the known four species of giraffe are threatened with low population numbers and declining range (Brown et al., 2021). As for many other mammals, these population declines vary from habitat loss and fragmentation to illegal hunting and climate change (Muller et al., 2018).
To tackle this critical issue and protect biodiversity, a comprehensive understanding of the ecology and biological requirements of individual species is first necessary (Fehlmann et al., 2017). This knowledge is important as it enables the development of effective conservation measures. In mammals, it becomes evident that they must adapt to a myriad of internal and external stimuli, which can significantly influence their behavioral patterns. How animals cope with these stimuli varies greatly between species, between individuals of the same species, and even within the same animal over time (Coppens et al., 2010; Breed and Moore, 2016; Lea et al., 2020). Through behavioral monitoring and the analysis of how these coping strategies manifest in specific behavioral patterns, we can enhance our understanding of such complex structures and functions of animal behavior. Direct observations of animals have been used since the early 20th century, however, several methods to collect behavioral data in natural settings have emerged in recent decades (Dominoni et al., 2017; Hughey et al., 2018; Lahoz-Monfort and Magrath, 2021). Contemporary technologies facilitate automated, efficient, and extensive data recording, even under low-light conditions, in remote locations, or across challenging terrains. Remote sensing technologies, such as camera traps, thermal imaging or night-vision systems have significantly contributed to species population assessments, our understanding of species ecology, and their behavior (Rattenborg et al., 2017).
The use of accelerometer data loggers, either independently or in combination with other remote sensing technologies like GPS has become prevalent (Neumann et al., 2015; Lahoz-Monfort and Magrath, 2021). Tri-axial accelerometers can measure acceleration in three orthogonal axes, providing information on an animal’s omnidirectional dynamic movement and posture. When used alongside GPS loggers, they can offer detailed insights into behavior patterns in both space and time (Katzner and Arlettaz, 2020; Williams et al., 2020). Further, accelerometer data can be collected over longer periods, even in inaccessible areas and without disturbing the animals by direct observers (Veasey et al., 1996). In particular, the use of accelerometers has shown to be especially effective for long-term studies, providing detailed information on behavioral patterns and rhythmicity in mammals (Shepard et al., 2008; Brown et al., 2013), fish, (Kadar et al., 2019) and reptiles (Arkwright et al., 2020). Using accelerometers, recent studies on ungulates examined species-specific behavior patterns to answer questions of natural needs. For instance, the coping strategies of blue wildebeest (Connochaetes taurinus) and gemsbok (Oryx gazella gazella) were investigated with regards to differing water dependencies (Boyers et al., 2019). McGowan and colleagues (McGowan et al., 2022) tested two different accelerometer devices for categorization accuracy to determine fine-scale behaviors in cheetahs.
Building on efforts to date, accelerometer data is now regularly used to determine behavioral rhythms and activity budgets of a species. Due to recording options over 24 hours with a high sampling rate and even during darkness, it is a valuable tool to study nocturnal behavior. This is particularly useful when studying inactivity and sleep behavior in diurnal species, such as most ungulates (Bennie et al., 2014; Wu et al., 2018). Although sleep is essential for survival and occurs in all vertebrates, surprisingly little is known about its function and even less about sleep behavior under natural conditions (Siegel, 2005; Anafi et al., 2019). In this context, Aulsebrook et al. (2016) highlighted this gap and the need for a comprehensive understanding of the functions and evolutionary origins of sleep. According to Siegel’s (2008) definition, sleep is a rapidly reversible state characterized by reduced responsiveness, motor activity, and metabolism. In mammals, this state can be categorized into rapid eye movement (REM) and non-REM states (Manger and Siegel, 2020). Although the exact function of sleep remains unclear, some theories propose that it prevents species from being active at inappropriate times and serves as an energy-saving state (Siegel, 2005; Capellini et al., 2010; Acerbi and Nunn, 2011). Notably, carnivorous and herbivorous mammals exhibit different sleep behavior patterns, with herbivores sleeping significantly less per day than carnivores, and sleep time in herbivores correlating inversely with body mass (Siegel, 2005; Gonfalone and Jha, 2015). Additionally, an animal’s digestive physiology, along with body mass, has a crucial impact on body posture (Pucora et al., 2019). In this context, the sleep behavior of ruminants, characterized by short REM sleep phases (Campbell and Tobler, 1984; Guebert et al., 2023), is particularly intriguing, especially for species like giraffe. Giraffe typically display polyphasic sleep behaviors with various short sleep events cyclically alternating with foraging, moving, and suckling events during the night (Tobler and Schwierin, 1996; Sicks, 2012; Razal and Miller, 2017; Burger et al., 2020a; Zoelzer et al., 2020). These cyclic behavioral sequences settle into a 24-hour rhythm generated by endogenous biological clocks (Mistlberger and Rusak, 2005). Circadian rhythms, usually synchronized by environmental stimuli or “zeitgebers,” are predominantly influenced by light (Aschoff, 1998; Reppert and Waever, 2002; Mistlberger and Rusak, 2005), but non-photonic stimuli such as environmental conditions, food availability, or social cues can also impact circadian rhythms in giraffe (Bashaw, 2011; Razal and Miller, 2017; Takagi et al., 2019).
Recent studies on sleep behavior in wild ungulates using accelerometer data showed how targeted analysis of species-specific activity budgets and sleep behavior can provide important information about their natural behavior and ecology, which in turn can contribute to their future conservation (e.g. in free-roaming Arabian oryx, Oryx leucoryx, Davimes et al., 2018; and in blue wildebeest, Malungo et al., 2021). In Angolan giraffe (Giraffa giraffa angolensis), accelerometers and GPS satellite units were used to determine their locomotion behavior in a natural habitat and especially its movement rhythmicity over a 24-hour cycle depending on sunlight and moon phases (Hart et al., 2020a). Although this study characterized the activity of Angolan giraffe over 24 hours, no detailed information on sleep behavior could have been provided due to a low sampling rate. Burger et al. (2021) firstly obtained data on this by observing giraffe directly and determining the number and length of sleep events. However, this attempt came with several difficulties, above all, it was impossible to follow the giraffe after their active phase around midnight due to inaccessible terrain. As a result, sleep behavior in free-ranging giraffe has only been analyzed until midnight. Nevertheless, information on sleep behavior and nocturnal rhythmicity of captive giraffe is apparent. Several zoo studies confirm giraffe to be short sleepers and to show a biphasic inactivity and sleep profile during the night (Tobler and Schwierin, 1996; Sicks, 2012; Burger et al., 2020a).
The main objective of this study was to analyze the nocturnal behavior of Angolan giraffe using accelerometers. More specifically, our aims were firstly to identify active, inactive and REM sleep behavior patterns in free-ranging giraffe using accelerometer data. Secondly, to verify whether nocturnal biphasic behavioral rhythms, which have already been described in giraffe in zoos (Burger et al., 2020a), also occur in free-ranging giraffe.
Okapuka Ranch in the Khomas Region, Namibia, is a private farm located 30 km north of the capital Windhoek. The farm is characterized by typical arid savannah landscapes and mixed woodland–grassland ecosystems. The climate is sub-tropical arid, tempered by altitude >1,450 m, with a hot-rainy season from December to March and a dry season from May to October, within which there is a cool period from May to August. Beside Angolan giraffe, a wide diversity of antelope is found on the ranch, including greater kudu (Tragelaphus strepsiceros), common waterbuck (Kobus ellipsiprymnus), impala (Aepyceros melampus), black wildebeest (C. gnou), blue wildebeest, and gemsbok. Additionally, several predators are on the property including cheetah (Acinonyx jubatus), leopard (Panthera pardus), spotted hyena (Crocuta crocuta) and other smaller predator species.
To analyze nocturnal rhythmicity and sleep behavior of free-roaming giraffe, ossicone mounted devices including an accelerometers and a GPS unit (Supplementary Figure 1) were fitted to three randomly selected young adult Angolan giraffe: one male (G1) and two females (G2 + G3) in November 2018 and data were collected until April 2019. Data were continuously recorded during the whole night from 19:00 to 7:00. However, the analysis was based on the months from December to March, as no more data could be recorded from G3 from 24 March onwards. The capture and tagging procedures were conducted by a registered Namibian wildlife veterinarian, in accordance with best practice protocols previously described by Hart et al. (Hart et al., 2020a; Hart et al., 2020b). In short, each individual giraffe was darted with a potent opioid and then safely secured on the ground with a rope and capture once the drug had taken effect. Once secured, a reversal drug was administered, and the animal was fitted with the unit before being safely released. All research was undertaken under the Namibian National Commission on Research, Science & Technology Certificate No. RCIV00042018, Authorization No. 2018011402.
To investigate the nocturnal activity patterns and sleep profiles of giraffe using accelerometer data, we categorized their behaviors into three main states: being active, inactive, or exhibiting Rapid Eye Movement (REM) sleep posture, according to established ethograms for giraffe (Burger et al., 2020a; Razal and Miller, 2017; Seeber et al., 2012). Analyzing the accelerometer data, giraffe were considered active if their head showed noisy movements as during walking or feeding. In contrast, giraffe were classified inactive when there were only slight to no head movements. This was the case during standing and lying. Behaviors analyzed via video data included walking, feeding, standing, lying, and sleeping. However, similar changes were measured on all three axes during walking and feeding (Supplementary Figure 2). Even lying and standing cannot be distinguished by the accelerometer data. Therefore, the behavior categories of the accelerometer data were characterized as follow: active = walking, feeding; inactive = standing, lying; RSP = specific REM sleep posture (Figure 1; compare Burger et al., 2020b). A giraffe was classified to be in REM sleep posture when lying on the ground, arching its neck backward, and resting its head on the flank or ground (Seeber et al., 2012; Tobler and Schwierin, 1996). This was recently confirmed by correlating body position/behavioral data and polysomnographic data in dromedary camels (El Allali et al., 2022) and in dairy cows (Hänninen et al., 2008; Ternman et al., 2014). In our study, this particular REM sleep posture led to a unique (and persistent) combination in the change of the three axes of the accelerometer during the “inactivity phases” (Figure 1; Supplementary Figure 2). RSP events were evaluated by means of a visual inspection. The following conditions had to be met for classification as an RSP event: 1) RSP events are only possible in the inactivity phases. 2) RSP events are characterized by a change in the Z-axis of more than −0.4 g. 3) RSP events have a minimum length of 2 min, whereby the changes in the Z-axis remain stable during this period. Unfortunately, this evaluation approach means that short-term RSP events (< 2 min) are not considered. Consequently, the number of RSP events is underestimated and the average duration in the study is overestimated. Previous studies show that the average duration of RSP events in free-ranging giraffe is between 1.48 minutes (rainy season) and 2.58 minutes (dry season) (Burger et al., 2020b). Despite this limitation, the collected data provide important insights into the rhythm and temporal occurrence of RSP events. The inactivity phases were analyzed automatically using Microsoft Excel (MS Office 365, Microsoft, USA). For this purpose, the median was determined for all accelerometer axes (X = −0.94, Y = 0.10, Z = 0.24). To be categorized as “inactivity”, the values of the individual axes were allowed to deviate by a maximum of ± 0.12. Furthermore, the standard deviation at this point (three data points: current value and the two data points before and after) was allowed to be a maximum of 0.015. If at least five of these six conditions were met, the data point was categorized as inactive (see Figure 1A).
Figure 1 Accelerometer-based determination of behavioral states in Angolan giraffe, Okapuka Ranch, Namibia. (A) The period from 19:00 to 07:00 (local time) is shown. Typically, phases occurred during the nights in which the changes in the three accelerometer axes were small and thus the head position remained constant (see also Supplementary Figure 2). These phases were therefore analyzed separately as “inactivity phases” and are shown in the upper sub-figure. Within these inactivity phases, characteristic changes occurred in the Z (and Y) axis, which had a comparable amplitude. The X-axis, on the other hand, remained largely unaffected. These characteristic events, which often occur during the inactivity phases, were analyzed in detail. (B) Section of the overall measurement (grey background in sub-figure (A), time period: 21:55 to 23:20 local time). The recorded data points are highlighted by circles. The filled circles and associated numbers indicate the time periods where validation with video footage took place (see sub-figure C). (C) Image sequences at the time points marked in (B). The animal equipped with the device (G2) is highlighted by arrows. In the image sequence 1 to 10, the temporary transition to RSP can be seen, which is simultaneously evident in the change of the Z (and Y) axis. The image sequence 11 to 15 marks a change of place within the group. Here, the animal lying in front of G2 stands up and lies down again behind the giraffe on the left side of the picture. Another animal lies down behind G2. In the image sequence 16 to 25, another RSP is visible. The measurement excursion at data point or image 17 (opposite changes in the Z and Y axes) is not recognizable in the video as a change in body or head position. In image sequence 26 to 30, the animals stand up and move around. This phase is characterized by rapid (minute-by-minute) change in all three axes, where the amplitude can vary in all axes.
Information on the nocturnal movement behavior of free-living giraffe has already been obtained using GPS devices (Hart et al., 2020a). However, detailed studies on the sleeping behavior of free-ranging giraffe covering the entire night have not yet been conducted. Studies using thermal imaging or night vision cameras have the difficulty that observation over an entire night is hardly possible due to nocturnal activity phases of the giraffe with (sometimes) rapid changes in the location of the group. Therefore, in this study, three young adult adult giraffe were fitted with accelerometers to measure the nocturnal behavior of giraffe using accelerometers. The accelerometers (RadioTag-14 unit in an adapted housing and base station for telemetry loggers, Milsar, Poland) measured 60 × 38 × 22 mm. The accelerometer device is equipped with a 300 mAh battery. Since the standard data loggers are adapted to birds by design, the housing design was adapted for use on giraffe to include a concave back, a brown color, a solar panel on the front and two feedthroughs for mounting straps (see Supplementary Figures 1A, B). Sampling rate was at one data point per minute. The used sample rate allowed for continuous recording during the dark phase and solar powered recharging of the battery during the day (Supplementary Figures 1C, D). Originally, the accelerometer data was meant to be at a much higher recording rate (one second sampling rate). During the pilot study in November 2018, however, it unfortunately turned out that with such a high recording rate, the battery was not sufficient to record the entire night. However, as we wanted to investigate the behavioral rhythms over the entire night, we had to decide to reduce the recording rate to one point per minute.
To validate the accelerometer data, video recordings were made in parallel (see also Shepard et al., 2008). The calibration of the accelerometer data was partly based on daytime video recordings (Supplementary Figure 2). However, as the giraffe were mainly observed during the night, further recordings were made using thermal imaging cameras (Figure 1). We used a SEEK CompactPro (with a 320 × 240 pixel temperature sensor and a 32-degree field of view; Seek Thermal Inc., USA) to record the observed behaviors, which in turn were affixed to a tablet (iPad mini 4, Apple, USA) and mounted on a tripod.
Rstudio (version 3.3.0) was used for the statistical tests. To investigate behavioral differences between individuals or months, a Friedmann-test and Bonferoni post-hoc test were applied. The Fast Fourier Transformation (FFT) was used to mathematically verify the biphasic sleep behavior of giraffe. The FFT allows a discrete time signal to be decomposed into its frequency components. In this case, the discrete time signal is the duration of RSP or inactivity per hour. The number of phases for RSP or inactivity can be derived from the resulting frequency components. MathWorks MATLAB 2023a (The MathWorks Inc., Natick, USA) was used to compute the FFT.
The accelerometer analysis showed that the Angolan giraffe were inactive for an average of 490.8 ± 43.7 minutes during the night (from 19:00 to 7:00 local time). Between December 2018 and March 2019, a total of 857 RSP events were recorded in the three giraffe. The number of RSP events varied nightly, ranging from 1 to 8 events per night. On 16–22% of the nights (depending on the individual), no RSP events were observed. Overall, giraffe showed 2.0 ± 1.0 RSP events per night and the total length of RSP per night averaged 8.6 ± 7.9 min.
Regarding RSP and inactivity, significant individual differences were found in this study. The total RSP length per night was significantly lower in G1 (5.7 ± 5.2 min/night, n = 121 nights) than in G2 (9.3 ± 8.6 min/night, n = 121 nights, G1–G2: p<0.001) and G3 (10.1 ± 8.6 min/night, n = 114 nights, G1–G3: p<0.001; see Figure 2A). Significant differences also occurred in the number of RSP per night: G1 1.5 ± 1.2 events/night, G2 2.2 ± 1.9 events/night, G3 2.3 ± 1.7 events/night (G1–G2: p<0.01; G1–G3: p<0.001; Figure 2B) and inactivity per night G1 468. 8 ± 42.5 min/night, G2 501.8 ± 84.2 min/night, G3 498.3 ± 42.0 min/night (G1–G2: p<0.001; G1–G3: p<0.001; Figure 2C). There were no significant differences between G2 and G3 for any of the three parameters.
Figure 2 Individual differences of RSP and inactivity in Angolan giraffe, Okapuka Ranch, Namibia. RSP length (A; in min), number of RSP events (B) and Inactivity (C; in min) per night for G1 to G3. Significant differences are marked by asterisks (* = p < 0.05, ** = p < 0.01, *** = p < 0.001). G1: n = 121 nights. G2: n= 121 nights, G3: n = 114 nights.
However, two giraffe showed significant differences in inactivity between months (Figure 3). In particular, G1 was significantly more inactive in February (485.6 ± 45.2 min) compared to December (458.47 ± 41.8 min) (p < 0.05). For G3, March was the month with the highest level of inactivity. We found significant differences between December (487.78 ± 39.4 min) and March (515.6 ± 39.4 min; p < 0.05) as well as between January (488.0 ± 36.0 min) and March (p < 0.05).
Figure 3 Variability of RSP and inactivity in Angolan giraffe, Okapuka Ranch, Namibia. RSP length (A; in min), number of RSP events (B) and Inactivity (C; in min) per night for G1 to G3 from December 2018 to March 2019. Significant differences only occurred in (C) and they are marked by asterisks (* = p < 0.05). G1: n = 121 nights. G2: n= 121 nights, G3: n = 114 nights.
When analyzing the results, it became apparent that RSP events and inactivity phases occur more frequently in individual sections of the night and that a nocturnal rhythm occurs. For a more detailed analysis, the respective temporal proportions for RSP and inactivity were determined, divided into hourly blocks. Higher proportions of time were observed for RSP between 21:00 and 23:00 (beginning about two hours after sunset) and 3:00 and 5:00 for all animals (Figure 4A). The highest inactivity was also observed for all giraffe in the same time range (Figure 4B). Both the RSP events and the inactivity show a clear biphasic profile during the night. This rhythmicity is also evident when analyzed with a Fast Fourier Transform (FFT), which confirms a biphasic rhythmicity for both RSP events and inactivity within the 12 hours (19:00 to 7:00 local time) for all individuals (Figures 4C, D).
Figure 4 Time course of RSP and inactivity during the night in Angolan giraffe, Okapuka Ranch, Namibia. RSP length (A; in min per hour) and inactivity (B; in min per hour) for G1 (triangle), G2 (circle) and G3 (square). The results of the Fast Fourier Transformation (FFT) for RSP (C) and Inactivity (D) can be see below. The frequency corresponds to the number of phases per 12 hours.
This study presents detailed insights into the nocturnal behavior of wild Angolan giraffe and outlines a biphasic inactivity profile consisting of several short sleep events. The results confirmed that giraffe, as diurnal animals, exhibit polyphasic sleep patterns characterized by few (2.0 ± 1.0) sleep events. Total sleep time in RSP was very short with 8.6 ± 7.9 min. per night. Sleep events occurred exclusively during the two periods of inactivity after and before sunset. In total, giraffe spent (490.8 ± 43.7 min) of the night on inactive behaviors such as standing or lying. Depending on whether a species is diurnal or nocturnal, behavior patterns and activity peaks during the day and the night may vary tremendously. Diurnal species, such as most large herbivores, are more active during the day while mainly sleeping at night (Bennie et al., 2014; Wu et al., 2018). The occurrence of different sleep events per night classifies most herbivores as polyphasic sleepers (Phillips et al., 2010). However, even among diurnal species, large differences can be found in terms of rhythmicity, duration of sleep events and total sleep time during the night (Campbell and Tobler, 1984; Clauss et al., 2021). Gübert and colleagues (Guebert et al., 2023) compared the activity budgets and sleep profiles of 19 ungulates and showed great differences between zebra species, Cetartiodactyla and white rhinos. In detail, zebras showed the lowest proportion of lying per night with 21.4% to 32.2%. In contrast, Cetartiodactyla were lying 79.6% of the night, with Hippotragus reported to be the species lying down the longest (88%). Investigating their sleep behavior, Guebert et al. (2023) found rhinos to spent the most time on REM sleep (18.3%) while zebras only spent 1.5% per night. Note that all species showed 3 to 7 sleep events per night. Interestingly, in all species, sleep events occurred throughout the whole night without a precise rhythm. Although all of these species are polyphasic sleepers, none of the species, not even the okapi which is closely related to the giraffe, showed a biphasic inactivity and sleep profile. In conclusion, this represents a peculiarity in the behavior of giraffe. Our results underpin recent findings on the nocturnal behavior of captive giraffe with similar RSP profiles including low number and shortness of sleep events (Tobler and Schwierin, 1996; Sicks, 2012; Burger et al., 2020b). Sub-adult giraffe living in zoos were only half of the night active (45.5 ± 11.3%). In more detail, giraffe spent 52.3 ± 10.9% lying and 2.0 ± 1.1% on REM sleep (Burger et al., 2020a). Zoo studies further showed that it is mainly biological factors such as age, maternity or health status that have an influence on the characteristics of sleep behavior. It is interesting to note that the average length of sleep events remains the same while only the number of sleep events per night changes across age classes. Juvenile giraffe showed 5.85 ± 3.56 min sleep phases with an average length of 3.37 ± 2.11 min and adult giraffe 3.14 ± 2.55 min with an average length of 2.87 ± 2.36 min per night. However, external influences such as transport from one zoo to another or changing housing conditions can also lead to short-term changes in the sleep profile (Bashaw, 2011; Sicks, 2012). As sleep is a highly sensitive process that is determined and regulated by a variety of internal and external influences, these influencing factors must also be analyzed (Ruckstuhl and Neuhaus, 2002, 2009; Siegel, 2005).
The nocturnal behavioral rhythm observed in giraffe is influenced by light, a strong zeitgeber for behavioral activity and sleep onset (Fonken and Nelson, 2014; Navara and Nelson, 2007; Reppert and Waever, 2002; Raap et al., 2015). Moonlight and sunlight have been shown to play a decisive role in the movement behavior (Hart et al., 2020a) and sleep onset (Burger et al., 2021) of wild Angolan giraffe. Results from studies on captive giraffe also confirm a biphasic sleep profile influenced by (artificial) light (Burger et al., 2020a). As darkness falls (whether the sun goes down or the artificial light source in the zoo is switched off), animals calm down and the first inactivity phase begins. Based on the GPS data analyses conducted by Hart et al. (2020a) and findings from zoo studies, it has been observed that giraffe exhibit a notable activity peak at midnight (Burger et al., 2020a). Additionally, zoo studies have indicated that giraffe predominantly engage in feeding during this activity peak. Due to the low sampling rate in the present study, it regrettably hindered the ability to distinguish between walking and feeding behaviors. Consequently, confirmation is limited to the observation that giraffes displayed an activity peak at midnight. The diel activity of mammals, marked by different rhythm patterns (nocturnal, diurnal, crepuscular, or cathemeral), is closely connected to natural light (Bennie et al., 2014). This ecological phenomenon is intricately linked to predator–prey relationships, suggesting a reciprocal co-evolutionary process (Wu et al., 2018). The spatiotemporal behavioral patterns in mammals, including giraffe, may have an evolutionary component contributing to the “ecology of fear” model (Bleicher, 2017). REM sleep time correlates with the safety of the resting site, proclaims Lima et al. (2005). Most large prey animals, such as giraffe or antelopes, which sleep in relatively unprotected resting sites show less REM sleep (Helm et al., 2017). Referring to Burger et al. (2021), this also applies to giraffe, as they observed sleeping giraffe in specific resting sites characterized by single trees or sparse shrubs allowing for a good view on open areas (Beauchamp, 2015).
In addition to light and predation risk, seasonal changes and weather conditions may impact activity patterns and social behavior, as observed in several ungulates (e.g., Kiffner et al., 2014; Davimes et al., 2018; Boyers et al., 2019; Hart et al., 2021). However, in this study, no significant differences between months were found for the number of RSP events nor for the total length of RSP per night. Interestingly, Burger et al. (2021) described seasonal differences during dry and wet season for the length of RSP events. During dry season, giraffe spent on average 155.2 ± 191.1 sec on RSP and less during wet season with only 85.8 ± 94.9 sec per RSP event (Burger et al., 2021). Note, due to the low sampling rate of one minute, our data of RSP length is not as reliable as the second-by-second direct observation. However, even if the comparison across studies is therefore weak, this study showed no difference between months in the number of RSP or the total length. Differences between months were only found for inactivity. G1 was more active in December (dry and hot) compared to in February (hot and rainy), whilst G3 also showed the lowest inactivity in December and the highest in March. These differences in inactivity levels could also be related to browse quality. Studies on the feeding behavior of different wild giraffe species suggest that browse quality is higher (and, consequently, forage bouts are more frequent) during the wet season, prompting giraffe to locally move and take advantage of new vegetation (Dagg, 2014; Mramba et al., 2017). However, these data only results from diurnal observation. One hypothesis could be that giraffe are more active during the day due to higher energy costs in feeding and that they need to rest more during the night. In consequence, the correlation between seasonal impacts (e.g. light, temperature, humidity, rain, food availability, predation risk, etc.) and nocturnal behavior requires further studies in giraffe, as it has been done already for other species. These and other ecological factors influence the frequency and duration of sleep in large herbivores with a high basal metabolic rate, such as elephants and rhinoceros. Gravett et al. (2017) found that light and sunset played no significant role in sleep onset in African savannah elephant (Loxodonta africana), with environmental conditions such as ambient air temperature and relative humidity having a larger impact. On the other hand, a study on black rhinoceros (Diceros bicornis bicornis) could not confirm a seasonal impact on sleep patterns (Santymire et al., 2012). In ungulates, significant effects of season on the duration of REM sleep have been described for Arabian oryx (Davimes et al., 2018), with oryx showing less and shorter sleep episodes during summer than during winter. Interestingly longer sleep events were more frequent during summer during the day, whereas shorter sleep events were more frequent during winter especially during nighttime (Davimes et al., 2018). In male blue wildebeest (Connochaetes taurinus), inactivity increased after a by season determined period of rut compared to prior to the rut Malungo et al., 2023).
Giraffe fold into a specific body position when entering REM sleep (REM sleep posture = RSP). The animal’s head needs to be rested during REM sleep due to postural muscle atonia (Lima et al., 2005; Zepelin et al., 2005). Accordingly, the body posture lying down and resting its head on the flank or ground can be used to estimate REM sleep in giraffe (Seeber et al., 2012). As shown in recent studies on domestic cows (Bos taurus; Ternman et al., 2014; Fukasawa et al., 2018), dromedary camels (Camelus dromedaries; El Allali et al., 2022) and the common eland (Taurotragus oryx; Zizkova et al., 2013), using REM body posture is used as an indicator for REM sleep behavior. Using accelerometry, these changes in head position result in unique burst patterns which makes RSP events clearly determinable in the actogram and enables for detailed sleep behavior analysis. As highlighted, sleep is a very sensitive phase for a species that is influenced by internal and external stimuli e.g. temperature, light, conspecifics, or predators (Siegel, 2008; Mistlberger and Rusak, 2011; Joiner, 2016). By using accelerometers instead of direct observers and research vehicles, external stimuli can be reduced (Veasey et al., 1996; Rattenborg et al., 2017). Nevertheless, video recordings for calibration purposes remained necessary for a few days. After successful calibration, long-term data recording was possible without human interference or disturbance. This type of automated data collection becomes even more useful when animals live in secluded, remote or in densely vegetated environments as it is the case for wild giraffe (Brown et al., 2013). Aside from remote or inaccessible study areas, behavioral observations are often restricted by light. By using accelerometers, behavior can be recorded at any time of day or night, regardless of the light conditions. Therewith, accelerometers are best suited for continuous 24/7 long-term recordings generating large data sets. Automated analysis is therefore of great interest and importance. Bidder et al. (2014) have successfully used the K-nearest neighbor for some mammalian species, while Brandes et al. (2021) published on the successful use of deep learning algorithms to analyze the behavior of three captive giraffe equipped with two different GPS devices. For captive animals, analyses using accelerometry can also play a pivotal role in assessing animal welfare. As activity budgets can serve as a reference point for comparing actual behavioral changes in an animal (Watters et al., 2009).
Giraffe as a megaherbivore play a crucial role in ecosystem functioning. Unfortunately, they are exposed to several human threats from which they need to be protected. Insight into their nocturnal timing and level of inactivity as well as their sleep behavior across month contributes substantial ecological information for comprehending their natural behavior. Giraffe only show two periods of inactivity during which they fall asleep. Sleep deprivation is a major threat to an animal and less or even no REM sleep over consecutive nights would lead to a reduced level in fitness (Siegel, 2005). In consequence, giraffe need to be protected from anthropogenic threats especially during these two highly sensitive periods of inactivity. Given the prevailing human influence on wildlife today, addressing this impact is critical. The declining giraffe populations, resulting in their vulnerable status on the IUCN Red List (Muller et al., 2018), are primarily attributed to human population growth effects leading to habitat loss and fragmentation, civil unrest, and illegal hunting. Recent studies, exploring the influence of human activity on wildlife, underscore the alarming increase in nocturnality in mammals globally due to human disturbance (Gaynor et al., 2018). Factors such as illegal hunting, hiking, urban development, and agriculture contribute to potential disturbances. The presence of humans in wildlife environments prompts behavioral adaptations in time patterns, social interactions, foraging behavior, and habitat use (Gaynor et al., 2018; Bond et al., 2021). Bond et al. (2023) conducted a study on the impact of human influences on the extinction risk of giraffe. Among others, the authors found reduction in law enforcement leading to more poaching to be the major threat. In light of human–wildlife conflict and the need to counter further declines in giraffe populations caused by human intervention, understanding the natural behavior of these animals and their habitat requirements becomes crucial. By delineating the nocturnal behavior and habitat use of wild giraffe, this study aimed to enhance our understanding of giraffe sleeping behaviors in their natural environment. When combined with findings from other studies, our results can contribute to an increased understanding of giraffe sleep patterns, with resulting conservation implications that, hopefully, will lead to their enhanced protection across Africa.
Our results present the first holistic behavioral sleep analyses for wild Angolan giraffe, classifying them not only as polyphasic but more specific as biphasic sleepers. Sleep events recorded by an accelerometer were identified precisely within the first two periods of inactivity after sunset and during the early morning hours. Despite the methodological difficulties described, data from accelerometers and GPS satellite devices offer important insights into the behavioral ecology of free-ranging animals. Through this study, we have expanded the understanding of wild giraffe’s nocturnal activities, furthering our knowledge to support conservation efforts for this iconic taxa.
The original contributions presented in the study are included in the article/Supplementary Material. Further inquiries can be directed to the corresponding author.
The animal study was approved by Namibian National 147 Commission on Research, Science & Technology Certificate No. RCIV00042018, Authorization No. 148 2018011402. The study was conducted in accordance with the local legislation and institutional requirements.
Conceptualisation and study design: AB and PD. Writing original draft: AB. Data collection: AB, ET, SF, JF and PD. Statistical analysis: AB and PD. Data interpretation and visualisation: AB and PD. Project administration and funding acquisition: PD. Review and editing: AB, PD, SF an JF. All authors contributed to the article and approved the submitted version.
The author(s) declare financial support was received for the research, authorship, and/or publication of this article. AB, ET and PD were supported by Opel-Zoo Foundation Professorship in Zoo Biology from the “von Opel Hessische Zoostiftung”. JF and SF were supported by the Giraffe Conservation Foundation.
The authors are very grateful to Okapuka Ranch and its owners for their generosity and allowing us to conduct this work. The research was conducted in Namibia under the research permit of the Giraffe Conservation Foundation: Namibian National Commission on Research, Science & Technology Certificate No. RCIV00042018, Authorization No. 2018011402. We also thank Dr H.O. Reuter for his expert wildlife veterinarian support, and the Giraffe Conservation Foundation and Ultimate Safaris for their financial contribution.
The authors declare that the research was conducted in the absence of any commercial or financial relationships that could be construed as a potential conflict of interest.
The author AB declared that they were an editorial board member of Frontiers, at the time of submission. This had no impact on the peer review process and the final decision.
All claims expressed in this article are solely those of the authors and do not necessarily represent those of their affiliated organizations, or those of the publisher, the editors and the reviewers. Any product that may be evaluated in this article, or claim that may be made by its manufacturer, is not guaranteed or endorsed by the publisher.
The Supplementary Material for this article can be found online at: https://www.frontiersin.org/articles/10.3389/fmamm.2023.1243883/full#supplementary-material
Supplementary Figure 1 | Adjustment of the data recording rate in the field. (A) Data recording is done via data loggers from Milsar (RadioTag-14 unit in an adapted housing measuring 60x38x22 mm and base station for telemetry loggers, Milsar, Poland). The housing design was adapted for use on giraffe to include a concave back, a solar panel on the front and two feedthroughs for mounting straps (UV-stable). Each stored data point contains the values for data logger identification number (ID), time stamp in UTC format (Universal Time Coordinated), accelerometer X-, Y- and Z-axis, temperature, altitude, speed, battery voltage, solar voltage, and the GPS coordinates in decimal degrees. The memory size of the devices is 1.2 gigabytes each. The data is retrieved via a base station using a UHF signal. Each data logger has its own ID. (B) The ‘Ossi-unit’ incorporating the solar-powered accelerometer device is attached to the ossicone on the inside. The X-axis shows whether the sensor is oriented downwards or upwards due to the head movement, the Y-axis describes the inclination of the head to the left or right and the Z-axis describes the raising and lowering of the head. (C) In a first step, the maximum possible data acquisition rate in the field was determined. At the beginning (data recording setting 1; November 2018) a recording rate of one data point per second was used. Continuous data recording was not possible with these settings, as data recording was interrupted for long periods, particularly at night (no solar charging of the battery). The hibernation threshold of the battery is about 3.65 V (highlighted in grey) and with the high data rate, the battery cannot be sufficiently charged during the day. By changing to data recording setting 2 (recording rate one data point per minute between 19:00 and 7:00 local time and one data point per hour between 8:00 and 18:00 local time; from December 2018 until the end of the recording period) it was possible to record during the night without data gaps. The battery voltage increased continuously during the day and reached a maximum of about 4.1 V. This provided a sufficient buffer to compensate for bad weather phases with less solar radiation (e.g. cloudy weather during the rainy season). The figure shows the period from 12 November to 14 March for G2. The asterisks indicate the time periods that are zoomed out in sub-figure (D). (D) At a data rate of one data point per second (data recordings setting 1, left sub-image), continuous data recording was not possible because data recording was interrupted for long periods, especially at night. The data for the Z-axis show correspondingly larger data gaps. The threshold value at 3.65 V was undershot for a long time and during the sunny phases of the day, the battery charge was insufficient to permanently increase the battery charge level. By switching to data recording setting 2 (right sub-image), a continuous increase in the battery voltage (to a maximum of about 4.1 V) was measured on sunny days during the day. The average battery voltage varied depending on the solar radiation, but it was sufficient to ensure continuous data recording during the entire measurement period (compare C).
Supplementary Figure 2 | Calibration of accelerometer data based on daytime video recordings. (A) Accelerometer data of the X-, Y- and Z-axis at different behaviors (W, Walking; F, Feeding; S, Standing; L, Lying) and head positions (U, Up; N, Neutral; D, Down). During feeding and walking, rapid, larger changes in accelerometer data are observed, whereas during standing and lying (without head movement), the changes in accelerometer data of the X, Y and Z axes are minor. The data was recorded at a rate of one data point per second (data recording setting 1). The white filled circles show the evaluation at a data rate of one data point per minute (to simulate data recording setting 2). (B, C) Example photos of different behaviors from the periods highlighted in grey in A).
Supplementary Figure 3 | Actigraphy devices and animal identification using thermal imaging. The giraffe were mostly observed in a herd of four to 12 animals. The download function of the logger was used to locate the animals for the video recordings with the thermal imaging camera. A connection between the device and the base station was usually established at between two and three kilometres. Here, the GPS coordinates of the animals in the field were read out with the corresponding base station, a USB cable, the software, and a laptop. These coordinates were then driven to in a car. Within a herd, it was possible to trigger a short light signal on the logger via the base station, so that individual animals could be identified and assigned in the thermal image. In the figure, a herd of seven animals is clearly visible in the thermal image (six lying animals in the rear area and one standing animal, marked by arrows). The animal with the logger was identified by a light signal (marked by the light bulb) from the logger as individual pattern recognition was not possible at night. The logger had an automatic radio download function and when the device comes within range of the base station; data is automatically downloaded and stored in the base station. This process was done daily for the first 20 days (when the animals were within range) and about once a week thereafter. Afterwards, the data was downloaded from the base station to the PC using a USB cable.
Acerbi A., Nunn C. L. (2011). Predation and the phasing of sleep: An evolutionary individual-based model. Anim. Behav. 81, 801–811. doi: 10.1016/j.anbehav.2011.01.015
Anafi R. C., Kayser M. S., Raizen D. M. (2019). Exploring phylogeny to find the function of sleep. Nat. Rev. Neurosci. 20, 109–116. doi: 10.1038/s41583-018-0098-9
Arkwright A. C., Archibald E., Fahlman A., Holton M. D., Crespo-Picazo J. L., Cabedo V. M., et al. (2020). Behavioural biomarkers for animal health: A case study using animal-attached technology on Loggerhead Turtles. Front. Ecol. Evol. 7. doi: 10.3389/fevo.2019.00504
Aschoff J. (1998). Circadian parameters as individual characteristics. J. Biol. Rhythms. 13, 123–131. doi: 10.1177/074873098128999970
Aulsebrook A. E., Jones T. M., Rattenborg N. C., Roth T. C., Lesku J. A. (2016). Sleep ecophysiology: Integrating neuroscience and ecology. Trends Ecol. Evol. 31 (8), 590–599. doi: 10.1016/j.tree.2016.05.004
Barnosky A. D., Matzke N., Tomiya S., Wogan G. O. U., Swartz B., Quental T. B., et al. (2011). Has the Earth’s sixth mass extinction already arrived? Nature 471, 51–57. doi: 10.1038/nature09678
Bashaw M. J. (2011). Consistency of captive giraffe behaviour under two different management regimes. Zoo Biol. 30, 371–378. doi: 10.1002/zoo.20338
Bennie J. J., Duffy J. P., Inger R., Gaston K. J. (2014). Biogeography of time partitioning in mammals. Proc. Natl. Acad. Sci. U.S.A. 111 (38), 13727–13732. doi: 10.1073/pnas.1216063110
Bidder O. R., Campbell H. A., Gómez-Laich A., Urgé P., Walker J., Cai Y., et al. (2014). Love thy neighbour: Automatic animal behavioural classification of acceleration data using the K-nearest neighbour algorithm. PloS One 9 (2), e88609. doi: 10.1371/journal.pone.0088609
Bleicher S. S. (2017). The landscape of fear conceptual framework: Definition and review of current applications and misuses. PeerJ 5, e3772. doi: 10.7717/peerj.3772
Bond M. L., Lee D. E., Farine D. R., Ozgul A., König B. (2021). Sociability increases survival of adult female giraffes. Proc. R. Soc B 288, 20202770. doi: 10.1098/rspb.2020.2770
Bond M. L., Lee D. E., Paniw M. (2023). Extinction risks and mitigation for a megaherbivore, the giraffe, in a human-influenced landscape under climate change. Glob Chang Biol. 29, 6693–6712. doi: 10.1111/gcb.16970
Boyers M., Parrini F., Owen-Smith N., Erasmus B. F. N., Hetem R. S. (2019). How free-ranging ungulates with differing water dependencies cope with seasonal variation in temperature and aridity. Conserv. Physiol. 7 (1), coz064. doi: 10.1093/conphys/coz064
Brandes S., Sicks F., Berger A. (2021). Behaviour classification on giraffes (Giraffa camelopardalis) using machine learning algorithms on triaxial acceleration data of two commonly used GPS devices and its possible application for their management and conservation. Sensors 21, 2229. doi: 10.3390/s21062229
Brown B. B., Kulkarni T., Ferguson S., Fennessy S., Muneza A., Stabach J. A., et al. (2021). Conservation status of giraffe: evaluating contemporary distribution and abundance with evolving taxonomic perspectives. Imperiled: Encyclo. Conserv. (3), 471–487. doi: 10.1016/B978-0-12-821139-7.00139-2
Brown D. D., Kays R., Wikelski M., Wilson R., Klimley A. P. (2013). Observing the unwatchable through acceleration logging of animal behaviour. Anim. Biotelemetry 1, 20. doi: 10.1186/2050-3385-1-20
Burger A. L., Fennessy J., Fennessy S., Dierkes P. W. (2020b). Nightly selection of resting sites and group behaviour reveal antipredator strategies in giraffe. Ecol. Evol. 10 (6), 2917–2927. doi: 10.1002/ece3.6106
Burger A. L., Hartig J., Dierkes P. W. (2020a). Shedding light into the dark: Age and light shape nocturnal activity and sleep behaviour of giraffe. Appl. Anim. Behav. Sci. 229, 105012. doi: 10.1016/j.applanim.2020.105012
Burger A. L., Hartig J., Dierkes P. W. (2021). Biological and environmental factors as sources of variation in nocturnal behaviour of giraffe. Zoo Biol. 40 (3), 171–181. doi: 10.1002/zoo.21596
Campbell S. S., Tobler I. (1984). Animal sleep: a review of sleep duration across phylogeny. Neurosci. Biobehav. Rev. 8, 269–300. doi: 10.1016/0149-7634(84)90054-X
Capellini I., Preston B. T., McNamara P., Barton R. A., Nunn C. L. (2010). “Ecological constraints on mammalian sleep architecture,” in Evolution of sleep. Phylogenetic and functional perspectives. Eds. McNamara P., Barton R. A., Nunn &C.L. (Cambridge, UK: Cambridge University Press), 12–33.
Clauss M., Scriba M., Kioko J., Ganzhorn J. U., Kiffner C. (2021). Camera-trap data do not indicate scaling of diel activity and cathemerality with body mass in an East African mammal assemblage. Ecol. Evol. 11 (20), 13846–13861. doi: 10.1002/ece3.8090
Coppens C. M., de Boer S. F., Koolhaas J. M. (2010). Coping styles and behavioural flexibility: Towards underlying mechanisms. Phil. Trans. R. Soc B 365, 4021–4028. doi: 10.1098/rstb.2010.0217
Dagg A. (2014). Giraffe: Biology, behavior and conservation (Cambridge, UK: Cambridge University Press).
Davimes J. G., Alagaili A. N., Bhagwandin A., Bertelsen M. F., Mohammed O. B., Bennett N. C., et al. (2018). Seasonal variations in sleep of free-ranging Arabian oryx (Oryx leucoryx) under natural hyperarid conditions. Sleep 41 (5), zsy038. doi: 10.1093/sleep/zsy038
Dominoni D. M., Åkesson S., Klaassen R., Spoelstra K., Bulla M. (2017). Methods in field chronobiology. Phil. Trans. R. Soc B 372, 20160247. doi: 10.1098/rstb.2016.0247
El Allali K., Beniaich Y., Farsi H., El Mehdi M′hani M., Sobhi Jabal M., Piro M., et al. (2022). Sleep pattern in the dromedary camel: a behavioural and polysomnography study. Sleep 45 (8), zsac101. doi: 10.1093/sleep/zsac101
Fehlmann G., O’Riain M. J., Hopkins P. W., O’Sullivan J., Holton M. D., Shepard E. L. C., et al. (2017). Identification of behaviours from accelerometer data in a wild social primate. Anim. Biotelemetry 5, 6. doi: 10.1186/s40317-017-0121-3
Fonken L. K., Nelson R. J. (2014). The effects of light at night on circadian clocks and metabolism. Endocr. Rev. 35 (4), 648–670. doi: 10.1210/er.2013-1051
Fukasawa M., Komatsu T., Higashiyama Y., Oshibe A. (2018). The use of accelerometer to measure sleeping posture of beef cows. Anim. Sci. J. 89, 488–493. doi: 10.1111/asj.12931
Gaynor K. M., Hojnowski C. E., Carter N. H., Brashares J. S. (2018). The influence of human disturbance on wildlife nocturnality. Science 360, 1232–1235. doi: 10.1126/science.aar7121
Gonfalone A. A., Jha S. K. (2015). The influence of gravity on REM sleep. Open Access Anim. Physiol. 7, 65–72. doi: 10.2147/OAAP.S80731
Gravett N., Bhagwandin A., Sutcliffe R., Landen K., Chase M. J., Lyamin O. I., et al. (2017). Inactivity/ sleep in two wild free-roaming African elephant matriarchs – Does large body size make elephants the shortest mammalian sleepers? PloS One 12 (3), e0171903. doi: 10.1371/journal.pone.0171903
Guebert J., Hahn-Klimroth M., Dierkes P. W. (2023). A large-scale study on the nocturnal behavior of African ungulates in zoos and its influencing factors. Front. Ethol. 2-2023. doi: 10.3389/fetho.2023.1219977
Hänninen L., Mäkelä J. P., Rushen J., de Passillé A. M., Saloniemi H. (2008). Assessing sleep state in calves through electrophysiological and behavioural recordings: A preliminary study. Appl. Anim. Behav. Sci. 111 (3-4), 235–250. doi: 10.1016/j.applanim.2007.06.009
Hart E. E., Fennessy J., Hauenstein S., Ciuti S. (2020a). Intensity of giraffe locomotor activity is shaped by solar and lunar zeitgebers. Behav. Processes 178, 104178. doi: 10.1016/j.beproc.2020.104178
Hart E. E., Fennessy J., Rasmussen H. B., Butler-Brown M., Muneza A. B., Ciuti S. (2020b). Precision and performance of an 180 g solar-powered GPS device for tracking medium to large-bodied terrestrial mammals. Wildl. Biol., wlb.00669. doi: 10.2981/wlb.00669
Hart E. E., Fennessy J., Wells E., Ciuti S. (2021). Seasonal shifts in sociosexual behaviour and reproductive phenology in giraffe. Behav. Ecol. Sociobiol. 75, 15. doi: 10.1007/s00265-020-02954-6
Helm B., Visser M. E., Schwartz W., Kronfeld-Schor N., Gerkema M., Piersma T., et al. (2017). Two sides of a coin: Ecological and chronobiological perspectives of timing in the wild. Philosophical Transactions of the Royal Society of London. Ser. B Biol. Sci. 372, 20160246. doi: 10.1098/rstb.2016.0246
Hughey L. F., Hein A. M., Strandburg-Peshkin A., Jensen F. H. (2018). Challenges and solutions for studying collective animal behaviour in the wild. Phil. Trans. R. Soc B 373, 20170005. doi: 10.1098/rstb.2017.000
IUCN (2020) Table 1a. Available at: https://www.iucnredlist.org/resources/summarystatistics (Accessed 01 Oct 2023).
Joiner W. J. (2016). Unraveling the evolutionary determinants of sleep. Curr. Biol. 26, R1073–R1108. doi: 10.1016/j.cub.2016.08.068
Kadar J., Ladds M., Mourier J., Day J., Brown C. (2019). Acoustic accelerometry reveals diel activity patterns in premigratory Port Jackson sharks. Ecol. Evol. 9, 8933–8944. doi: 10.1002/ece3.5323
Katzner T. E., Arlettaz R. (2020). Evaluating contributions of recent tracking-based animal movement ecology to conservation management. Front. Ecol. Evol. 7. doi: 10.3389/fevo.2019.00519
Kiffner C., Kioko J., Leweri C., Krause S. (2014). Seasonal patterns of mixed species groups in large east African mammals. PloS One 9 (12), e113446. doi: 10.1371/journal.pone.0113446
Lahoz-Monfort J. J., Magrath M. J. L. (2021). A comprehensive overview of technologies for species and habitat monitoring and conservation. BioScience 71) 10, 1038–1062. doi: 10.1093/biosci/biab073
Lea S. E. G., Chow P. K. Y., Leaver L. A., McLaren I. P. L. (2020). Behavioral flexibility: A review, a model, and some exploratory tests. Learn. Behav. 48, 173–187. doi: 10.3758/s13420-020-00421-w
Lima S., Rattenborg N., Lesku J., Amlaner C. (2005). Sleeping under the risk of predation. Anim. Behav. 70, 723–736. doi: 10.1016/j.anbehav.2005.01.008
Malungo I. B., Gravett N., Bhagwandin A., Davimes J. G., Manger P. R. (2021). Sleep in two free-roaming blue wildebeest (Connochaetes taurinus), with observations on the agreement of polysomnographic and actigraphic techniques. IBRO Neurosci. Rep. 10, 142–152. doi: 10.1016/j.ibneur.2021.02.005
Malungo I. B., Gravett N., Ganswindt A., Manger P. R. (2023). Male blue wildebeest increase activity during the rut, but not at the expense of rest. J. Comp. Physiol. B. doi: 10.1007/s00360-023-01493-6
Manger P. R., Siegel J. M. (2020). Do all mammals dream? J. Comp. Neurol. 528(17), 3198–3204. doi: 10.1002/cne.24860
McGowan N. E., Marks N. J., Maule A. G., Schmidt-Künzel A., Marker L. L., Scantlebury D. M. (2022). Categorising cheetah behaviour using tri-axial accelerometer data loggers: a comparison of model resolution and data logger performance. Mov Ecol. 10, 7. doi: 10.1186/s40462-022-00305-w
Mistlberger R. E., Rusak B. (2005). “Circadian rhythms in mammals: Formal properties and environmental influences,” in Principles and practice of sleep medicine, 4th Edition. Eds. Kryger M., Roth T., Dement W. C. (St. Louis, MO: Elsevier Sauders), 321–334.
Mistlberger R. E., Rusak B. (2011). “Circadian rhythms in mammals: Formal properties and environmental influences,” in Principles and Practice of Sleep Medicine. 4th edition. Eds. Kryger M., Roth T., Dement W. C. (Philadelphia, PA: Elsevier), 321–334.
Mramba R. P., Mahenya O., Siyaya A., Mathisen K. M., Andreassen H. P., Skarpe C. (2017). Sexual segregation in foraging giraffe. Acta Oecol. 79, 26–35. doi: 10.1016/j.actao.2016.12.007
Muller Z., Bercovitch F., Brand R., Brown D., Brown M., Bolger D., et al. (2018). “Giraffa camelopardalis (errata version),” in The IUCN Red List of Threatened Species 2016, e.T9194A109326950. (Gland, Switzerland: International Union for Conservation of Nature and Natural Resources). doi: 10.2305/IUCN.UK.2016-3.RLTS.T9194A51140239.en
Navara K. J., Nelson R. J. (2007). The dark side of light at night: physiological, epidemiological, and ecological consequences. J. Pineal Res. 43, 215–224. doi: 10.1111/j.1600-079X.2007.00473.x
Neumann W., Martinuzzi S., Estes A. B., Pidgeon A. M., Dettki H., Erricsson G., et al. (2015). Opportunities for the application of advanced remotely-sensed data in ecological studies of terrestrial animal movement. Mov Ecol. 3, 8. doi: 10.1186/s40462-015-0036-7
O'Connor D., Stacy-Dawes J., Muneza A., Fennessy J., Gobush K., Chase M. J., et al. (2019). Updated geographic range maps for giraffe, Giraffa spp., throughout sub-Saharan Africa, and implications of changing distributions for conservation. Mamm. Rev. 49(4), 285–299.
Phillips A. J. K., Robinson P. A., Kedziora D. J., Abeysuriya R. G. (2010). Mammalian sleep dynamics: how diverse features arise from a common physiological framework. PLoS Comput. Biol. 6(6):e1000826. doi: 10.1371/journal.pcbi.1000826
Pucora E., Schiffmann C., Clauss M. (2019). Resting posture in terrestrial mammalian herbivores. J. Mammal. 100, 552–563. doi: 10.1093/jmammal/gyz044
Raap T., Pinxten R., Eens M. (2015). Light pollution disrupts sleep in free-living animals. Sci. Rep. 5, 13557. doi: 10.1038/srep13557
Rattenborg N., La Iglesia H., Kempenaers B., Lesku J., Meerlo P., Scriba M. (2017). Sleep research goes wild: New methods and approaches to investigate the ecology, evolution and functions of sleep. Philos. Trans. R. Soc Lond. B 372, 20160251. doi: 10.1098/rstb.2016.0251
Razal C., Miller L. (2017). Monitoring the behavioural and adrenal activity of giraffe (Giraffa camelopardalis) to assess welfare during seasonal housing changes. Anim. Behav. Cogn. 4 (2), 154–168. doi: 10.12966/abc.03.05.2017
Reppert S. M., Waever D. R. (2002). Coordination of circadian timing in mammals. Nature 418, 935–941. doi: 10.1038/nature00965
Ruckstuhl K. E., Neuhaus P. (2009). Activity budgets and sociality in a monomorphic ungulate: The African oryx (Oryx gazella). Can. J. Zool. 87, 165–174. doi: 10.1139/Z08-148
Ruckstuhl K. E., Neuhaus P. (2002). Modelling sexual segregation in ungulates: effects of group size, activity budgets and synchrony. Anim. Behav. 64, 909–914. doi: 10.1006/anbe.2002.2015
Santymire R., Meyer J., Freeman E. (2012). Characterizing sleep behaviour of the wild black rhinoceros (Diceros bicornis bicornis). Sleep 35, 1569–1574. doi: 10.5665/sleep.2212
Seeber P. A., Ciofolo I., Ganswindt A. (2012). Behavioral inventory of the giraffe (Giraffa camelopardalis). BMC Res. Notes 5, 650. doi: 10.1186/1756-0500-5-650
Shepard E. L. C., Wilson R. P., Quintana F., Gómez Laich A., Liebsch N., Albareda D. A., et al. (2008). Identification of animal movement patterns using tri-axial accelerometery. Endanger. Species Res. 10, 47–60. doi: 10.3354/esr00084
Sicks F. (2012). Paradoxer Schlaf als Parameter zur Messung der Stressbelastung bei Giraffen (Giraffa camelopardalis). Dissertation (Frankfurt, Germany: Goethe University Frankfurt, Germany). Available at: https://dnb.info/1044276150/34.
Siegel J. (2005). Clues to the functions of mammalian sleep. Nature 437 (27), 1264–1271. doi: 10.1038/nature04285
Siegel J. (2008). Do all animals sleep? Trends Neurosci. 31 (4), 208–213. doi: 10.1016/j.tins.2008.02.001
Takagi N., Saito M., Ito H., Tanaka M., Yamanashi Y. (2019). Sleep-related behaviors in zoo-housed giraffes (Giraffa camelopardalis reticulata): basic characteristics and effects of season and parturition. Zoo Biol. 38, 490–497. doi: 10.1002/zoo.21511
Ternman E., Pastell M., Agenäs S., Strasser C., Winckler C., Nielsen P., et al. (2014). Agreement between different sleep states and behaviour indicators in dairy cows. Appl. Anim. Behav. Sci. 160, 12–18. doi: 10.1016/j.applanim.2014.08.014
Tobler I., Schwierin B. (1996). Behavioural sleep in the giraffe (Giraffa camelopardalis) in a zoological garden. Sleep Res. 5 (1), 21–32. doi: 10.1046/j.1365-2869.1996.00010.x
Veasey J. S., Waran N. K., Young R. J. (1996). On comparing the behaviour of zoo housed animals with wild conspecifics as a welfare indicator, using the giraffe (Giraffa camelopardalis) as a model. Anim. Welf. 5, 139–153. doi: 10.1017/S0962728600018571
Watters J. V., Margulis S. W., Atsalis S. (2009). Behavioural monitoring in zoos and aquariums: A tool for guiding husbandry and directing Research. Zoo Biol. 28, 35–48. doi: 10.1002/zoo.20207
Williams H. J., Taylor L. A., Benhamou S., Bijleveld A. I., Clay T. A., de Grissac S., et al. (2020). Optimizing the use of biologgers for movement ecology research. J. Anim. Ecol. 89, 186–206. doi: 10.1111/1365
Wu Y., Wang H., Wang H., Feng J. (2018). Arms race of temporal partitioning between carnivorous and herbivorous mammals. Sci. Rep. 8, 1713. doi: 10.1038/s41598-018-20098-6
Zepelin H., Siegel J. M., Tobler I. (2005). “Chapter 8 - Mammalian Sleep,” in Principles and Practice of Sleep Medicine. Eds. Kryger M. H., Roth T., Dement W. C. (New York: Elsevier), 91–100.
Zizkova K., Kotrba R., Kocisova A. (2013). Effect of changes in behaviour on the heart rate and its diurnal variation in a male and a female eland (Taurotragus oryx). Agricult. tropica subtropica 46, 29–33. doi: 10.2478/ats-2013-0005
Keywords: field chronobiology, accelerometry, Angolan giraffe, sleep, nocturnal behavior
Citation: Burger-Schulz AL, Thiel E, Fennessy J, Fennessy S and Dierkes PW (2023) Accelerometry reveals nocturnal biphasic sleep behavior in wild giraffe. Front. Mamm. Sci. 2:1243883. doi: 10.3389/fmamm.2023.1243883
Received: 21 June 2023; Accepted: 29 November 2023;
Published: 18 December 2023.
Edited by:
Steve Portugal, University of London, United KingdomReviewed by:
Michael Scantlebury, Queen’s University Belfast, United KingdomCopyright © 2023 Burger-Schulz, Thiel, Fennessy, Fennessy and Dierkes. This is an open-access article distributed under the terms of the Creative Commons Attribution License (CC BY). The use, distribution or reproduction in other forums is permitted, provided the original author(s) and the copyright owner(s) are credited and that the original publication in this journal is cited, in accordance with accepted academic practice. No use, distribution or reproduction is permitted which does not comply with these terms.
*Correspondence: Anna Lena Burger-Schulz, YnVyZ2VyQGVtLnVuaS1mcmFua2Z1cnQuZGU=
Disclaimer: All claims expressed in this article are solely those of the authors and do not necessarily represent those of their affiliated organizations, or those of the publisher, the editors and the reviewers. Any product that may be evaluated in this article or claim that may be made by its manufacturer is not guaranteed or endorsed by the publisher.
Research integrity at Frontiers
Learn more about the work of our research integrity team to safeguard the quality of each article we publish.