- Department of Psychology, California Polytechnic State University Humboldt, Arcata, CA, United States
In two experiments, English words and non-words varying in phonotactic probability were cross-modally compared in an AB matching task. Participants were presented with either visual-only (V) speech (a talker's speaking face) or auditory-only (A) speech (a talker's voice) in the A position. Stimuli in the B position were of the opposing modality (counterbalanced). Experiment 1 employed monosyllabic items, while experiment 2 employed bisyllabic items. Accuracy measures for experiment 1 revealed main effects for phonotactic probability and presentation order (A-V vs. V-A), while experiment 2 revealed main effects for lexicality and presentation order. Reaction time measures for experiment 1 revealed an interaction between probability and lexicality, with a main effect for presentation order. Reaction time measures for experiment 2 revealed two 2-way interactions: probability and lexicality and probability and presentation order, with significant main effects. Overall, the data suggests that (1) cross-modal research can be conducted with various presentation orders, (2) perception is guided by the most predictive components of a stimulus, and (3) more complex stimuli can support the results from experiments using simpler stimuli, but can also uncover new information.
Introduction
Speech perception is a multimodal process (see Rosenblum, 2019 for a review). Under normal circumstances, these streams of complementary information are integrated to facilitate perception. For instance, a visible speaking face increases spoken word recognition under adverse auditory conditions, such as when perceiving speech in a noisy environment (e.g., Sumby and Pollack, 1954; Grant and Seitz, 2000; Bernstein et al., 2004; Kim and Davis, 2004), when listening to someone with an accent (Arnold and Hill, 2001), and when listening to a dense message (Reisberg et al., 1987). Under abnormal circumstances, different speech modalities will also become integrated, such as evidenced by McGurk and MacDonald (1976) seminal study demonstrating the McGurk effect and the research that has followed along this line (see Rosenblum, 2019 for a review). Thus, speech perception is not solely an auditory phenomenon and in fact, there is mounting evidence in neuroscience research that suggests a cross-modal relationship between the senses in human and animal research across a variety of speech and non-speech situations (see Rosenblum et al., 2017).
Investigations of cross-modal speech information have revealed that the visual and auditory sensory modalities carry information about both the linguistic message and indexical properties of the speaker (e.g., Lachs and Pisoni, 2004), though the linguistic research is emphasized here. However, important questions remain and the current study aims to address the following questions: (1) Can cross-modal speech research be conducted with various presentation orders? (2) What component(s) of a speech stimulus guides perception? and (3) should speech researchers be more cautious in overinterpreting the results obtained from relatively simple stimuli and deliberately follow up their research with more complex stimuli?
Cross-modal research
Concerning question one, can cross-modal speech research be conducted with various presentation orders, studies have historically made methodological decisions that have led to a singular presentation order, where visual stimuli serve to prime auditory stimuli, but not the other way around (e.g., Buchwald et al., 2009; Kim et al., 2004; Sanchez et al., 2013). Within this research, insofar as linguistic information is concerned, cross-modal studies have found that when presented with speech information in one modality, that information can be used to prime information presented in a different modality (Buchwald et al., 2009; Kim et al., 2004) and facilitate the perception of a message (e.g., target words) presented in a different modality (e.g., Buchwald et al., 2009; Sanchez et al., 2013). For example, Kim et al. (2004) found that visual speech items could reliably prime auditory speech or text based targets in both a lexical decision task and a word naming task, supporting the idea that auditory and visual speech are processed similarly (e.g., Auer, 2002; Mattys et al., 2002; Rosenblum et al., 2017). However, it should be noted that Kim et al. only found reliable priming for words, but not non-word items. Kim et al. indicate that it is possible that non-word items were not able to be primed due to the fact that the lists presented to participants contained word and non-word items intermixed, which may have led to words competing in non-word trials. It should also be noted that Kim et al. always had visual items as primes and not targets, because lip-reading is such a demanding task often accompanied by many errors.
As an extension of Kim et al. (2004); Buchwald et al. (2009), investigated the role of lexical access on cross-modal priming using monosyllabic words. Due to the influence of lexical frequency and neighborhood density, recognition of words vary in speed and accuracy within auditory-only (e.g., Luce and Pisoni, 1998; Vitevitch and Luce, 1999) and visual-only contexts (e.g., Auer, 2002). In line with the auditory-only (e.g., Luce and Pisoni, 1998; Vitevitch and Luce, 1999) and visual-only research (e.g., Auer, 2002), Buchwald et al. found that high frequency words from sparse neighborhoods (e.g., “easy” words have little competition) were responded to faster and more accurately than low frequency words from dense neighborhoods (e.g., “hard” words compete with each other), suggesting that lexical access may be an important determiner for recognition, however, non-words were not used. Like Kim et al., visual items always served as primes to auditory targets, though the targets were presented in noise that challenged the identification of the target item. In addition, Buchwald et al. found that the visual primes did not have to come from the same speech event as the auditory targets, as accuracy and reaction time did not differ between match and mismatched primes and targets (in the mismatch trials, the prime and target were of different gendered talkers), suggesting that lexical influences and priming effects are not rigidly instance specific (e.g., episodic).
In a related vein, Sanchez et al. (2013) tested whether a word's lexical characteristics would impact word identification from a familiar or unfamiliar talker in a cross-modal experiment. In the training or familiarization phase of Sanchez et al. (2013), a single talker's words were presented auditorily-only, and varied in lexical frequency (high, medium-high, medium-low, and low, see Goldinger, 1998). Later, in a lip-reading (visual-only) task, participants were presented with two talkers, the familiar talker from the training phase and an unfamiliar talker. Both talkers in the lipreading phase uttered old words (i.e., words that had been presented during the voice familiarization phase) and new words. It was found that old words were lipread more accurately than new words, and high frequency words were lipread more accurately than low frequency words (Auer et al., 2000; Auer, 2002; Buchwald et al., 2009). Importantly for cross-modal speech perception, words were more accurately recognized when spoken by the familiar talker than the unfamiliar talker—even though the familiarity of the talker was trained in a different sensory modality. These results are in line with past research (e.g., Buchwald et al., 2009; Rosenblum et al., 2007) suggesting that both talker and lexical knowledge may be carried in auditory and visual modalities. In addition, this study demonstrates that cross-modal matching can reliably occur to visual lipread speech as the target stimulus. However, questions remain whether cross-modal matching can successfully occur without training.
Past research has revealed a lexical influence on cross-modal speech perception. For example, Kim et al. (2004) found that visual words (but not non-words) can facilitate the perception of auditory words. Similarly, Buchwald et al. (2009) showed that neighborhood density of a word presented cross-modally impacts perception with high frequency “easy” words from sparse neighborhoods as easier to perceive than low frequency “hard” words from dense neighborhoods, and that the visual primes and auditory targets do not have to come from the same speech event. In addition, Sanchez et al. (2013) demonstrated that visual lipread speech can successfully serve as target stimuli in a cross-modal experiment and that high frequency words are more accurately identified as compared to low frequency words. Although this seems to suggest that cross-modal research can be conducted with various presentation orders, where visual or auditory stimuli can serve as targets and primes interchangeably, the current research specifically aims to test this notion in an untrained sample.
Speech components guiding perception
Concerning question two, what component(s) of a speech stimulus guides perception, in the present study, we highlight the role of phonotactic probability, which is the likelihood of a particular phoneme or sequence of phonemes occurring at a given location within a word (using real words and non-words), while controlling for neighborhood density and lexical frequency to explore the relationship between audio and visual speech information. Motivation to investigate the role of phonotactic probability and lexicality is drawn from the work of Vitevitch and Luce (1999) as they provide a relevant framework to compare their audio-only experiments and results to our study, which uses the same general methodology and stimuli, but adapted to include auditory and visual speech. In addition, we are able to position the question of the nature of visual speech within a formalized model, specifically Grossberg's adaptive resonance theory (ART) (Grossberg, 2013, 2021).
Vitevitch and Luce (1999) conducted a series of experiments aiming to address the competitive and facilitatory effects of lexical and sublexical processing in spoken word recognition using words and non-words. Phonotactic probability was manipulated because it provides a means to highlight the constituent parts, or the relevant groupings of information when it comes to speech recognition. This is not to say that lexicality or phonotactic probability represent “critical units” of speech. In fact, within ART, the speech unit question is a moot point, as “all possible grain sizes are emergent products of resonant brain states” (Goldinger and Azuma, 2003). The current investigation however finds cause to explore the impact of phonotactic probability to compare and extend the work of Vitevitch and Luce and interpret visual-only speech data within the ART framework, which has not been conducted to the knowledge of the author.
Within ART, sensory (bottom-up) information is perceived and recognized based on the observer's prior learning or knowledge, expectations, and experiences (e.g., top-down processing). In essence, a stimulus in the world is akin to a vibrating tuning fork. The vibrations from the external tuning fork causes the tuning fork(s) in the observer's mind (created from previous experiences/learning) to also vibrate or resonate depending on whether the observer has a similar tuning fork within their mind. Within ART, in general, the most predictive units (e.g., familiar items compared to unfamiliar items) will have the greatest resonance and thus impact perception and recognition (Grossberg, 2021; Goldinger and Azuma, 2003). When a stimulus from the world interfaces with the top-down knowledge of the observer, the stimulus's features (in the form of items/feature clusters) are activated in working memory and subsequently activate list chunks in short-term memory. Chunks can be of varying size depending on the input, and can include, but are not limited to lexical and sublexical units and reflect the prior learning (e.g., prototypes) that emerge from the resonance between the stimulus from the outside world and the observer's mind. Within this framework, a hierarchy between the contents in the chunk is not assumed, nor is it a requirement for a sublexical unit to play a role in the activation of a lexical unit (or vice versa). However, as chunks compete with one another for dominance (via lateral inhibitory links), longer list chunks outcompete smaller list chunks via masking and inhibition. A feedback system begins when a stimulus's features activate list chunks, however, an identical match between the stimulus and the prototypes in the observer's mind is not necessary for resonance to occur. Small mismatches are resolved via competitive and cooperative levels, though resonance is disrupted for large mismatches. The resonance state that emerges between bottom-up and top-down interactions, rather than simply activation of information in one's mind, is considered the percept of the observer and is thus what is acted upon.
Therefore, when presented with a speech token, the token's status as a word compared to a non-word, the typicality of the token's constituent parts (e.g., phonotactic probability), and the complexity of the token (e.g., monosyllabic compared to bisyllabic), are all predicted to impact the resonance state within ART. When considering the token's lexicality, words are predicted to be quicker to achieve a resonance state and thus, faster reaction times are expected when responding to word stimuli compared to non-words, given that legitimate word stimuli are more likely to activate previously stored information in one's mind compared to a non-word, where arguably no previous experience exists.
However, given that identical matches are not necessary for resonance to occur, the similarity of the non-word token to stored instances and its constituent parts in the observer's mind, may yet lead to resonance, though at a slower rate. When considering non-words, the constituent parts, in the current case we specifically mean the phonotactic probability of the token, bears more weight in the activation of the resonance state than for words. For our purposes, and also as used by Vitevitch and Luce (1999), the phonotactics of the tokens are all valid in English (the language used in the respective experiments), though they vary on whether they are highly probable, and thus predictable in English, compared to low probability phonotactics. Thus, for non-words, resonance should still occur within the observer, as the observer arguably has stored instances of the various phonotactics upon which resonance is able to occur, even if they are sometimes infrequently experienced. However, in the non-word case, tokens composed of highly probable phonotactics are predicted to be quicker to achieve a resonance state and result in faster reaction times because there are more instances of these highly probable instances to support resonance to occur, as compared to less probable phonotactics, where resonance relies on fewer instances to support resonance.
However, words may not necessarily be immune to the impacts of its constituent parts, at least when comparing one word to another. In fact, Vitevitch and Luce (1999) found that when comparing words against words, words were responded to differently depending on the particular word's phonotactics. They found that participants responded to words with low phonotactic probability faster than those words with high phonotactic probability, while responding to non-words in a reverse manner, as expected by ART (i.e., faster for high probability non-words compared to low). Vitevitch and Luce (1999, and also see Luce and Pisoni, 1998; Vitevitch and Luce, 1998) suggest that for words, the lack of competition for low phonotactic probability words allows for resonance to occur faster than for high phonotactic probability words, as the many stored instances might have many similar competitors, where the competition slows down the resonance state.
Simple and complex stimuli
Concerning question three, should speech researchers be more cautious in overinterpreting the results obtained from relatively simple stimuli and deliberately follow up their research with more complex stimuli, the current study aims to first examine monosyllabic stimuli and then replicate and extend the research by using bisyllabic stimuli as the complexity and composition of a word may be highlighted when using bisyllabic compound words of various phonotactic probabilities, as compared to monosyllabic words. Use of temporally longer stimuli provides the ability to test whether, in some circumstances, the constituent parts of a word may bear more weight in perception. Longer stimuli require more time to unfold, and thus may lead to processing of sub-lexical components, like phonemes, to inform behavior (Vitevitch and Luce, 1999). However, ART1 contends that larger chunks (e.g., whole words) should outcompete smaller chunks (e.g., phonemes, syllables, smaller words), and should in fact mask and inhibit the smaller chunks, so that the perceptual experience is of the whole word and not a collection of parts (Grossberg, 2021; Goldinger and Azuma, 2003). This would suggest that for real words, the constituent parts of the word should not play a big role on the resonance state and that words composed of high or low phonotactic probabilities should be equivalent. For example, upon the presentation of a word composed of highly probable phonotactics (e.g., “pancake”, both “pan” and “cake” are composed of phonemes that are highly probable), as compared to a word composed of less probable phonotactics (e.g., “logjam”, both “log” and “jam” are low phonotactic probability units), one's perceptual experience is of the whole word (“pancake” and “logjam”). Notwithstanding, when comparing the speed of perception between real words, and thus resonance, it is possible that the constituent parts that inform the whole word may vary in the speed at which resonance occurs. In this case, as Vitevitch and Luce (1999) suggest, high probability components may result in more competition than low probability components and thus, words with high probability components may achieve resonance at a slower rate than words with low probability components. However, differences should emerge in the opposite direction when comparing words to non-words composed of different phonotactic compositions. For example, non-word items composed of highly probable units (e.g., /ɹɑɪɹmɑɪd/, both /ɹɑɪɹ/ and/ mɑɪd/are composed of phonemes that are highly probable) should be faster to perceive than items composed of low probability phonotactics (e.g., /ðΛ∫∫Λd3/, both/ ðΛ∫/ and/ ∫Λd3/ are low phonotactic probability units) and is predicted by ART.
In addition, the nature of the signal (e.g., audio-only, visual-only) may also impact the resonance state. However, thus far, this issue has not been investigated. Yet, there is some indication that at least one difference may stem from the speed at which auditory-only or visual-only information creates the resulting resonance state. It is assumed that auditory-only information would result in a faster resonance state compared to visual-only information. Grossberg (2021, p. 428–429) suggests that humans learn the associative link between speech sounds and speech movements (motor commands) via an imitative map. Although ART does not assume that the underlying speech information is in the form of gestures, as per the Motor Theory (see, e.g., Liberman et al., 1967; Liberman and Mattingly, 1985; For a review, see Galantucci et al., 2006) ART does account for both auditory and visual speech as they are directly coupled and influence and shape each other. Thus, when presenting stimuli comparing audio to visual or visual to audio, it is expected that stimuli in an audio format will achieve resonance faster than visual-only, as it is likely for the observer to have more matches in their mind similar to the stimulus than for visual-only. Although resonance can certainly be achieved with visual-only stimuli, the potential for ambiguity is likely to lead to a slower resonance state. However, the current study is presenting stimuli comparisons of audio to visual and visual to audio, in which case, it is expected that the pattern of results would be similar in nature, though the audio to visual is expected to demonstrate faster reaction times than video to audio.
The current study aims to address the role of lexicality, phonotactic probability, and word complexity (e.g., length) on cross-modal speech perception in a same-different task that varies the presentation order of stimuli. Experiment 1 presents monosyllabic items, while experiment 2 presents bisyllabic items. These experiments are aimed to widen the scope of current speech theories, to include visual based data and will highlight Grossberg's adaptive resonance theory (ART).
Experiment 1
Method
Participants
Participants were 67 native English speakers (52 females) enrolled at Cal Poly Humboldt participating for class credit. All participants (Mean age = 20 years) reported having good hearing and normal or corrected-to-normal vision.
Materials and stimuli
Nine different speakers (five female) were audio-visually recorded uttering the stimulus lists from Vitevitch and Luce (1999) three times and the single best token per speaker was used in the experiment. One female speaker's recordings were used only in the practice trials and were not used in the full experiment. These lists contained both monosyllabic and bisyllabic items. Note that the word items were also used in Vitevitch and Luce (1999), while the non-words were also used in Jusczyk et al. (1994). Experiment 1 used only the monosyllabic items. The monosyllabic items varied on lexicality (140 words, 240 non-words) and phonotactic probability, where half of the items were high on phonotactic probability in English, and the other half were low on phonotactic probability in English.
Two measures of phonotactic probability, segment frequency and biphone frequency, were calculated in Vitevitch and Luce (1999) and are reported here. Segment frequency refers to the frequency with which a given segment occurs in a given position for English words. Biphone frequency is a measure that is correlated with segmental transitional probability (Gaygen, 1998). Biphone frequency refers to the probability of co-occurrence between segments.
Segment and biphone probability were calculated from log-frequency weighted counts of 20,000 words from an online version of Merriam-Webster's (1967) Pocket Dictionary (Vitevitch and Luce, 1999). Thus, words classified as high in phonotactic probability are (1) items that have high segment positional probabilities and (2) have a high probability phonotactic pattern of biphones with high probability initial consonant–vowel and vowel–final consonant sequences. Words classified as low in phonotactic probability have low values on the aforementioned features. Table 1 shows the average segment and biphone probabilities for word and non-word items for high and low probability stimuli lists used in Vitevitch and Luce (1999) and the current study's experiment 1.
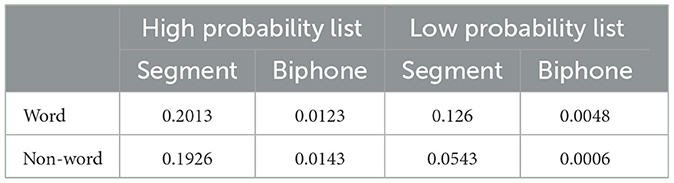
Table 1. Average segment and biphone probabilities for word and non-word items for high and low probability stimuli lists used in Experiment 1 (from Vitevitch and Luce, 1999).
In addition, Vitevitch and Luce (1999) controlled the stimuli for frequency-weighted similarity neighborhoods, isolation points, and word frequency. A neighbor was set at a Levensthein distance of 1. Frequency-weighting of the neighborhood was computed via summed log frequencies of neighbors for words and non-words, following Luce and Pisoni (1998). The mean log-frequency weighted neighborhood density values for high density words was 56 and for low density words was 40. The mean log-frequency weighted neighborhood density values for high density non-words was 45 and for low density non-words was 13. Isolation points for the words were also calculated (Luce, 1986; Marslen-Wilson and Tyler, 1980). High probability/density words had mean isolation points of 2.98 phonemes, and low probability/density words had mean isolation points of 2.93 phonemes (there were no statistical differences in the isolation points for these two groups of words). All non-words had isolation points at the final segment. Finally, frequency of occurrence Kucera and Francis (1967) was also calculated. For words, high probability/density words had an average log word frequency of 2.68 and low probability/density words had an average log word frequency of 2.59 (there was no significant difference in the log word frequency of these two groups of words).
All speakers recorded for this experiment were native to Central California, with a mean age of 23 years. Each speaker was recorded on a Sony Nex-7 E 18-55 mm F3.5-5.6 OSS, which captured the speaker from the top of the head to the shoulders. These recordings were edited to isolate the individual tokens uttered. The audio tracks were removed from the video files and amplitude adjusted to be equivalent using the sound editing software (Audacity 2.1.3 [Computer Software], 2017).
Design
A two presentation order (A-V, V-A) by two phonotactic probability (high, low) by two lexicality (word, non-word) within subjects design was implemented. The two levels of presentation order were presented within-subjects and refer to the order in which stimuli were presented in the matching task: audio-video (AV) or video-audio (VA). The two levels of lexicality refers to the word or non-word status of the stimulus items. The two levels of phonotactic probability are high and low. The operational definition of these two levels differ based on the lexicality of the stimulus item: for words, high phonotactic probability items are found frequently in English while low phonotactic probability have a lower frequency of occurrence in English; for non-words, the phonotactic probability of a non-word refers to the transitional phonotactic probabilities computed based on the phonotactic probabilities of lexical items in English, as per Psychology Software Tools (2012).
Procedure
Participants engaged in the experiment in isolation, in individual experimental rooms at Cal Poly Humboldt. Participants were instructed that they would be presented with recordings of a speaker saying word or word-like items. On each trial, participants were presented with the auditory or visual display of the speaker uttering a stimulus word or non-word. Participants were then presented with the same word or non-word in the other modality.
Participants were asked to decide whether or not the audio recording matches the visual recording or whether the video recording matches the audio recording by responding with their right hand on the keyboard number pad (1 = match, 2 = mismatch). Participants were instructed to respond as quickly as possible. The experimental stimuli were presented using the program E-Prime (Psychology Software Tools, Pittsburgh, PA) with Beyerdynamic (DT 770 Pro) headphones. Each participant was presented with the recordings made of a single speaker, randomly assigned.
Participants engaged in this task over four blocks, where presentation order and lexical type was consistent within a block, and alternated from one block to another. For the first two blocks, presentation orders (A-V or V-A) were the same and then the order switched for the last two blocks, counterbalanced between participants. Lexicality always varied per each block, so that word blocks would follow non-word blocks, and vice versa, counterbalanced between participants. For example, a participant might experience the following order: e.g., V-A word block, V-A non-word block, A-V word block, A-V non-word block. Blocks in which stimuli were words consisted of 70 items, while blocks in which the stimuli were non-words consisted of 140 items. Half of the stimuli in each block matched and varied on both levels of phonotactic probability equally. Mismatching trials also varied on both levels of phonotactic probability. On mismatching trials, foils in position B were of the same phonotactic probability as the item in position A and were paired based on the same initial phoneme and (when possible) the same vowel. Rationale for implementing blocked lists based on lexicality was based on the results obtained from Kim et al. (2004) where non-word items failed to be primed due to the intermixed lists when engaging in a cross-modal task. Thus, the current experiment aims to replicate the methods used in Vitevitch and Luce's (1999) experiment 1, where lists were blocked based on lexicality.
Experiment 1: results and discussion
Reaction times two standard deviations above and below the mean were eliminated from the analyses (Ratcliff, 1993). Using this criteria, 4.74% of the data was removed. All data were analyzed using R (Development Core Team, 2009) and the R packages lme4 (Bates and Maechler, 2009) and languageR (Baayen, 2009; cf. Baayen, 2008). Logistic mixed effects models were fit by hand, using model comparison and included comparison to a control model. All models started with the highest level interaction (a possible 3-way interaction between presentation order, phonotactic probability, and lexicality). Non-significant interactions and main effects (provided they were not part of a larger interaction) were removed until only significant terms were present. The dependent (predictor) variables were accuracy and reaction time (Z-scored). All analyses were conducted on correct responses to “match” trials, following the analyses conducted in Vitevitch and Luce (1999). The fixed effects in our model were our independent variables (i.e., phonotactic probability, lexicality, and presentation order) which were allowed to interact. All analyses had random intercepts of Subject and Item (Baayen et al., 2008; Clark, 1973). The final and reduced model contained a maximal random effect structure (Barr et al., 2013). In all cases, the reduced final models performed better than the control models (e.g., values for AIC and BIC were significantly lower for the reduced final models). All analyses adopted a p-value criterion of p < 0.05.
Accuracy analyses
The reduced final model using accuracy as the dependent variable did not contain any significant interaction terms or a main effect for lexicality and they were subsequently removed from the reduced final model. A significant main effect of phonotactic probability indicated that items of high probability (M = 0.76) were responded to less accurately (β = −0.19, Std. error = 0.07, Z-value = −2.70, p < 2.00E-16) than items of low probability (M = 0.79). The accuracy results will be also considered together with the reaction time results.
In addition, a significant main effect of presentation order indicated that participants were less accurate (M = 0.71) on VA trials (β = −0.89, Std. error = 0.11, Z-value = −10.19, p < 2.00E-16) compared to AV trials (M = 0.84). This result suggests that when the visual stimulus precedes the auditory stimulus, there is greater potential for ambiguity and thus negatively impacts accuracy. This finding is consistent with previous research (e.g., Sanchez et al., 2013) and is in line with what would be predicted by ART.
Reaction time analyses
The reduced final model using reaction time (Z-scored per participant to control for the impact of individual differences on processing speed and variability, as recommended by Faust et al., 1999) as the dependent variable contains one interaction term and all independent variables as main effects. Figure 1 displays a significant two-way interaction between phonotactic probability and lexicality (β = 0.17, Std. error = 0.04, t-value = 4.20, p = 5.23E-05). Follow-up simple contrasts were conducted using the emmeans R package (Lenth, 2021). When considering items with low phonotactic probability, words and non-words significantly differed, with words (M = −0.38) predicting faster reaction times (β = −0.32, Std. error = 0.04, Z-ratio = −7.49, p < 0.0001) than non-words (M = −0.07). Similarly, for items with high phonotactic probability, words and non-words significantly differed, with words (M = −0.37) predicting faster reaction times (β = −0.15, Std. error = 0.04, Z-ratio = −4.20, p = 0.0001) than non-words (M = −0.21). For non-words, high and low phonotactic probability significantly differed, with high probability phonotactics (M = −0.21) predicting faster reaction times (β = −0.15, Std. error = 0.02, Z-ratio = −5.95, p < 0.0001) than low probability phonotactics (M = −0.07). However, for words, high (M = −0.37) and low (M = −0.38) phonotactic probability did not significantly differ (β = 0.02, Std. error = 0.03, Z-ratio = 0.79, p = 0.83).
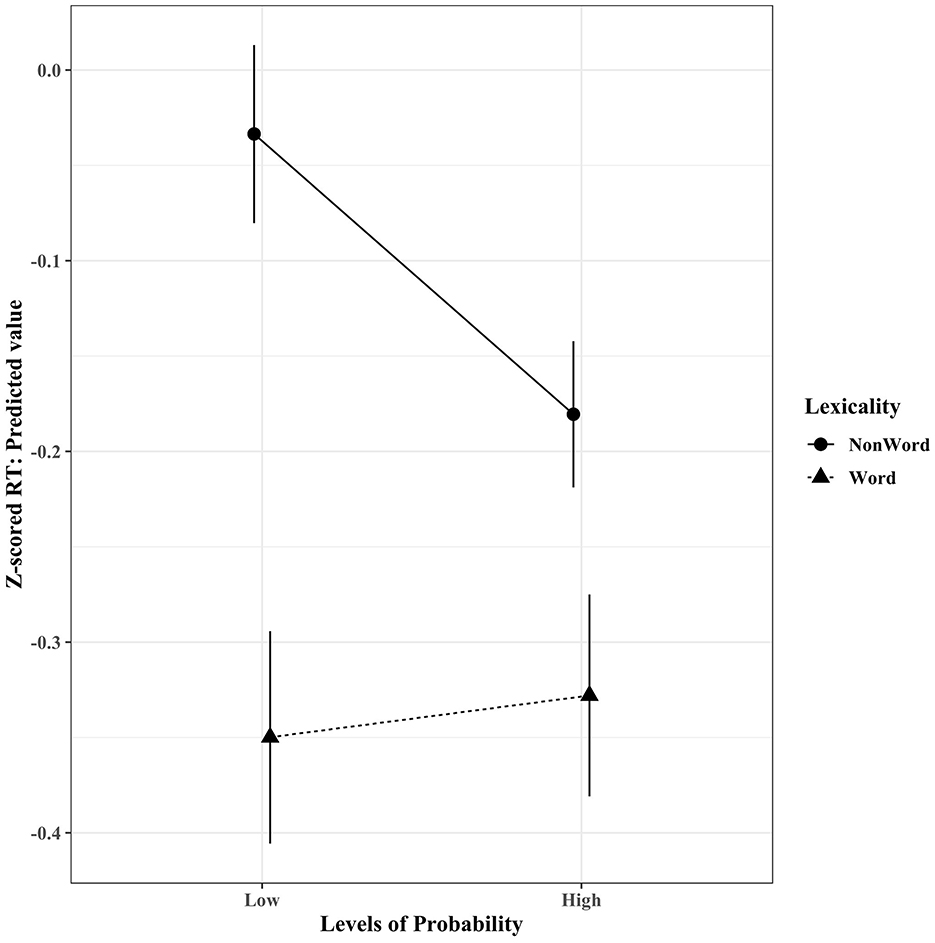
Figure 1. Experiment 1 used monosyllablic tokens. Reaction time data (z-scored) revealed a significant interaction between the variables (phonotactic) probability and lexicality. Error bars indicate 95% confidence interval.
Interestingly, these results are more in line with Vitevitch and Luce's (1999) experiment 2 results than their experiment 1, which the current study aimed to extend by using cross-modal presentations of stimuli. Vitevitch and Luce's experiment 1 presented participants with two different blocks of trials, where each block represented either word items or non-word items counterbalanced between participants. In the current study, we too present different blocks of trials according to different levels of lexicality, but the inclusion of the presentation order variable (e.g., A-V or V-A) resulted in four different blocks of alternating lexicality. Although no significant effects were observed of Vitevitch and Luce's accuracy measure, their reaction time measure resulted in a significant interaction between phonotactic probability and lexicality, where high and low phonetic probability differed for both words and non-words in an opposite direction. Specifically, low phonotactic probability items were responded to faster for words compared to high phonotactic probability items, while low phonotactic probability items were responded to slower for non-words compared to high phonotactic probability items. Due to the blocked trials of lexicality, Vitevitch and Luce argued that participants emphasized different components of the stimuli to make matches based on the stimuli in the given block, where participants could emphasize the word status of the items in the word blocks and emphasize more granular components, like phonotactic probability of the items in the non-word blocks, resulting in opposite effects for different levels of phonotactic probability for word and non-words.
However, in Vitevitch and Luce's (1999) experiment 2, lexicality was not blocked, but instead intermixed. The motivation to test an intermixed list compared to a blocked list was to induce participants to make matches highlighting the smaller components of the stimuli (e.g., phoneme level differences, phonotactic probability) rather than the larger components (e.g., word level) present in the stimuli. For their accuracy measure, a main effect of lexicality was observed, where words were responded to more accurately than non-words. For the reaction time measure, a significant interaction between phonotactic probability and lexicality was observed. Although high and low levels of phonotactic probability items were responded to at a similar speed for words, they varied for non-words; low phonotactic probability items were responded to slower for non-words compared to high phonotactic probability items. This pattern of results mirrors the results of the current study. It is possible that the inclusion of the visual stimulus in the current experiment unintentionally induced participants to make same-different judgements highlighting phoneme level differences rather than the wordness of the stimuli. This could be due to the fact that when a visual stimulus unfolds, each movement of the articulators slowly reveals the identity of the speech component (e.g., phoneme/viseme, syllables, etc.). Notwithstanding, we find that our results are in line with the predictions made by ART; when longer list chunks are present, in this case word items, the smaller constituent parts (e.g., phonemes) are masked, thus no effect for phoneme level differences are found at the word level. However, when longer list chunks are not available, as in the case for non-words, items composed of highly probable phonotactics achieve resonance at a quicker rate than items composed of less probable phonotactics.
In addition to the interaction, significant main effects were observed for phonotactic probability, lexicality, and presentation order. For phonotactic probability, items with highly probable phonotactics (M = −0.28) were responded to faster (β = −0.15, Std. error = 0.02, t-value = −5.95, p = 2.82E-08) than items with low probability phonotactics (M = −0.19). This result is consistent with the prediction made by ART, as typically, the most common items are expected to achieve resonance faster than less common items. However, in light of the accuracy results, this suggests a speed-accuracy trade-off may occur when considering the constituent parts of a stimulus.
For lexicality, word items (M = −0.37) were responded to faster (β = −0.32, Std. error = 0.04, t-value = −7.49, p = 4.62E-11) than non-word items (M = −0.15). The word advantage compared to non-words is in line with the prediction made by ART. Words are expected to result in faster reaction times because they are able to achieve resonance quicker than non-words, given that presented word items have a higher likelihood of existing within a participant's mind, via previous experience, as compared to a non-word.
In addition, for presentation order, V-A trials (M = −0.05) were responded to slower (β = 0.52, Std. error = 0.04, t-value = 13.55, p < 2.00E-16) than A-V trials (M = −0.46). This result is consistent with the accuracy result and again suggests that the ambiguity of the visual stimulus in the “A” position results in significantly slower resonance than when an auditory stimulus is in the “A” position.
Overall, the results of our experiment 1 are consistent with the results obtained in Vitevitch and Luce's (1999) experiment 2, though the current experiment aimed to model Vitevitch and Luce's experiment 1. With respect to ART, the interaction of phonotactic probability and lexicality for reaction time indeed suggests that when larger chunks of information are present, as in the case of words, that those larger chunks dominate the resonance state. However, in non-word instances, the constituent parts of the item are responsible for the resonance state, where highly probable phonemes are more likely to achieve resonance at a faster rate (due to more stored instances) than less probable phonemes.
Experiment 2
The results of experiment 1 represent a simple test of the relationship between phonotactic probability and lexicality in a cross-modal matching task, as the stimuli were composed of monosyllabic items. A stronger test, using more complex stimuli, such as bisyllabic stimuli may present more validation. Experiment 2 was designed to replicate and extend experiment 1 by examining the role of longer, more complex bisyllabic stimuli and the relationship between phonotactic probability and lexicality in a cross-modal matching task.
Method
Participants
94 participants (67 females) English first language speakers enrolled at cal poly humboldt, participated for class credit. All participants (Mean age = 21 years) reported having good hearing and normal or corrected-to-normal vision.
Materials and stimuli
Experiment 2 used the bisyllabic words and non-words from Vitevitch and Luce (1999). The recording procedure was identical in all respects to that used for creating the monosyllabic stimuli in experiment 1. 120 words and 120 non-words were recorded. Four types of bisyllabic items were used: high-high, high-low, low-high, and low-low. The first and second syllable could each independently have a high or low phonotactic probability, as calculated in Vitevitch and Luce.
Design
A 2 presentation order (A-V, V-A) by 4 phonotactic probability (high-high, high-low, low-high, low-low) by 2 lexicality (word, non-word) within subjects design was implemented. The two levels of presentation order reflect the modality order of the stimulus presentations: audio-video (A-V) and video-audio (V-A). The four levels of phonotactic probability in this study are: high-high, high-low, low-high, and low-low. The two levels of lexicality are represented by real English words and non-words that are plausible English words.
Procedure
The procedure for experiment 2 followed the procedure employed in experiment 1, but used bisyllabic items.
Experiment 2: results and discussion
All data were analyzed in the same fashion as the experiment 1 data. Reaction times two standard deviations above and below the mean were eliminated from the analyses (Ratcliff, 1993). Using this criteria, 4.56% of the data was removed.
Accuracy analyses
The reduced final model using accuracy as the dependent variable did not contain any significant interaction terms nor a main effect for phonotactic probability and they were subsequently removed from the reduced final model. A significant main effect of lexicality indicated that words (M = 0.86) were responded to more accurately (β = 0.45, Std. error = 0.08, Z-value = 5.84, p = 5.24E-09) than non-words (M = 0.80). In addition, a significant main effect of presentation order indicated that participants were less accurate (M = 0.77) on V-A trials (β = −1.07, Std. error = 0.09, Z-value = −12.12, p < 2.00E-16) compared to A-V trials (M = 0.89). These results are consistent with our monosyllabic data from experiment 1.
Reaction time analyses
Table 2 displays the summary of the reduced final model using reaction time (Z-scored per participant, as recommended by Faust et al., 1999) as the dependent variable. Two significant two-way interactions and all three independent variables are significant as main effects in the model; given that all independent variables are part of an interaction, the interactions will be emphasized.
Figure 2 displays a significant two-way interaction between phonotactic probability and lexicality. Follow-up simple contrasts were conducted using the emmeans R package (Lenth, 2021). When considering items with low-low phonotactic probability, words and non-words significantly differed, with words (M = −0.44) predicting faster reaction times (β = −0.77, Std. error = 0.05, Z-ratio = −15.36, p < 0.0001) than non-words (M = 0.32). For items with low-high phonotactic probability, words and non-words significantly differed, with words (M = −0.52) predicting faster reaction times (β = −0.61, Std. error = 0.04, Z-ratio = −13.81, p < 0.0001) than non-words (M = 0.09). For items with high-low phonotactic probability, words and non-words significantly differed, with words (M = −0.50) predicting faster reaction times (β = −0.62, Std. error = 0.04, Z-ratio = −14.62, p < 0.0001) than non-words (M = 0.12). For items with high-high phonotactic probability, words and non-words significantly differed, with words (M = −0.52) predicting faster reaction times (β = −0.51, Std. error = 0.04, Z-ratio = −13.08, p < 0.0001) than non-words (M = −0.01). For non-words, items with high-low and low-low phonotactic probabilities significantly differed, with high-low probability phonotactics (M = 0.12) predicting faster reaction times (β = −0.23, Std. error = 0.04, Z-ratio = −6.02, p < 0.0001) than low-low probability phonotactics (M = 0.32). In addition, for non-words, items with high-high and high-low phonotactic probabilities significantly differed, with high-high probability phonotactics (M = −0.12) predicting faster reaction times (β = −0.13, Std. error = 0.04, Z-ratio = −3.11, p = 0.02) than high-low probability phonotactics (M = 0.12). However, for non-words, items with high-low (M = 0.12) and low-high (M = 0.09) phonotactic probabilities did not significantly differ. No significant differences were found between any level of phonotactic probability for word items.
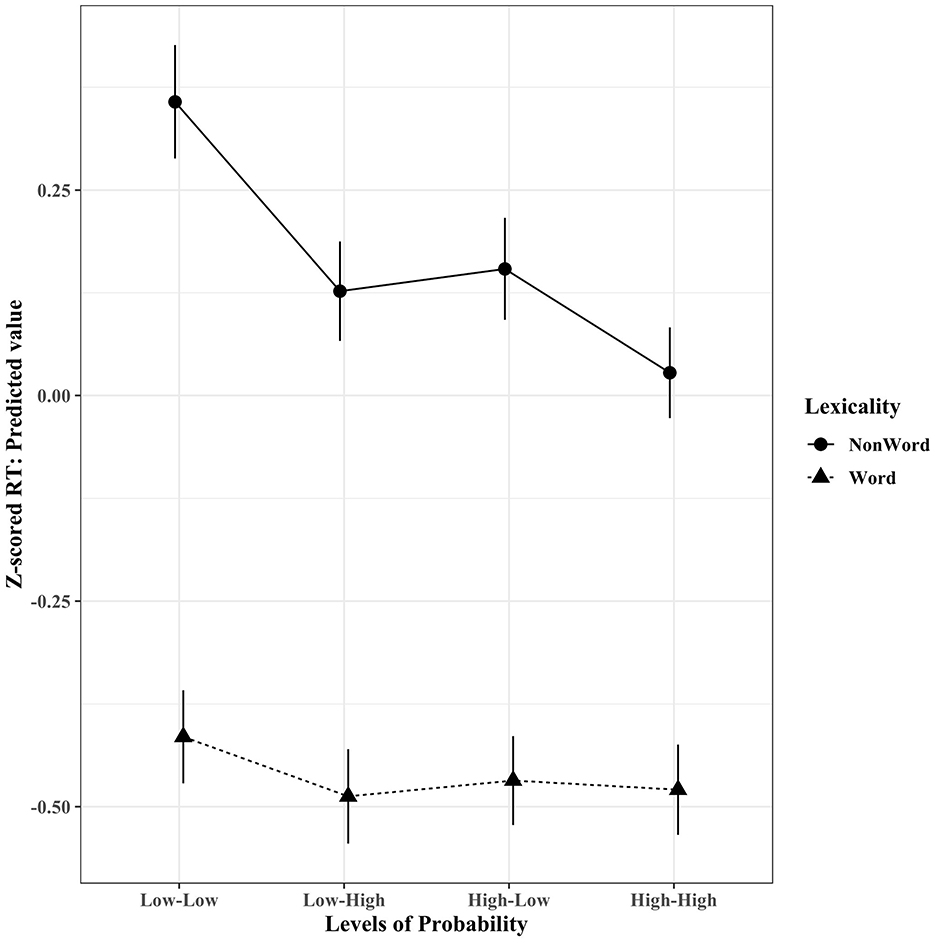
Figure 2. Experiment 2 used bisyllabic tokens. Reaction time data (z-scored) revealed a significant interaction between the variables (phonotactic) probability and lexicality. Error bars indicate 95% confidence interval.
The bisyllabic interaction between phonotactic probability and lexicality are in line with the monosyllabic interaction observed in experiment 1. The importance of the constituent parts of the item (i.e., phoneme level differences, phonotactic probability) was only found to be of importance for non-word items. This pattern of results is in line with the predictions made by ART. In ART, in general, larger chunks (e.g., words) are expected to dominate the resonance state and out-compete subcomponents of an item (e.g., phonemes), so that when presented with items like “pancake” and “logjam” the whole word is experienced and not a series of high or low probability pieces. However, for non-words, where the largest chunk available reflects the phonotactics a person has accumulated over time, highly probable phonotactics are expected and were found to achieve resonance quicker than less probable phonotactics. This is demonstrated in the results by all non-word items with a highly probable phonotactic component (i.e., low-high, high-low, and high-high) being responded to significantly faster than items composed of only low probability phonotactics (i.e., low-low). In line with Vitevitch and Luce (1999), items with mixed probabilities (i.e., low-high, high-low) were not found to be different from one another. However, high-high was not found to be significantly faster than low-high, though high-high was found to be significantly faster than high-low for non-words.
To account for why high-low items were responded to slower than expected for non-words, it is possible that the way an item begins sets the observer's expectations in a way that can either help or hinder the resonance process. In fact, ART fully acknowledges the impact of expectations and context playing important roles in the resonance process (Grossberg, 2021; Goldinger and Azuma, 2003; Vitevitch and Luce, 1999). In the case of non-words where the constituent parts are emphasized, high probability items are expected to achieve resonance at a faster rate than low probability items because the abundance of relevant traces that match within the mind of the observer are easier to access; conversely, the relatively sparse number of relevant traces available for low probability items are more challenging to access. This prediction was borne out through the data from experiment 1, which used monosyllabic stimuli. Now, in the case of experiment 2, which uses bisyllabic stimuli, when an item begins with a high probability component it is possible that high probability components are expected to follow, but when a low probability component follows, one's expectation is violated in a negative way, and the search of relevant memory traces is disrupted, resulting in a slower time to achieve resonance (compared to high-high). In contrast, for low-high phonetic probability cases, one's expectation is violated in a positive way, and the search of relevant memory traces is facilitated, resulting in a faster time to achieve resonance, equal to that observed in a high-high case. Two instances of high phonotactic probability components (i.e., high-high) did not result in an increased speed benefit compared to a single instance of a high phonotactic probability component present in the second half of the stimulus.
Figure 3 displays a significant two-way interaction between phonotactic probability and presentation order. Follow-up simple contrasts were conducted using the emmeans R package (Lenth, 2021). When considering items with low-low phonotactic probability, presentation orders significantly differed, with V-A trials (M = 0.15) predicting slower reaction times (β = 0.43, Std. error = 0.04, Z-ratio = 11.13, p < 0.0001) than A-V trials (M = −0.27). For items with low-high phonotactic probability, presentation orders significantly differed, with V-A trials (M = 0.01) predicting slower reaction times (β = 0.46, Std. error = 0.03, Z-ratio = 13.10, p < 0.0001) than A-V trials (M = –.43). For items with high-low phonotactic probability, presentation orders significantly differed, with V-A trials (M = 0.06) predicting slower reaction times (β = 0.50, Std. error = 0.04, Z-ratio = −12.91, p < 0.0001) than A-V trials (M = −0.43). For items with high-high phonotactic probability, presentation orders significantly differed, with V-A trials (M = 0.01) predicting slower reaction times (β = 0.52, Std. error = 0.04, Z-ratio = 13.40, p < 0.0001) than A-V trials (M = −0.50). For A-V trials, items with low-high and low-low phonotactic probabilities significantly differed, with low-high probability phonotactics (M = −0.43) predicting faster reaction times (β = −0.16, Std. error = 0.03, Z-ratio = −5.54, p < 0.0001) than low-low probability phonotactics (M = −0.27). However, for A-V trials, items with high-low (M = −0.43) and low-high (M = −0.43) phonotactic probabilities and high-low (M = −0.43) and high-high (M = −0.50) phonotactic probabilities did not significantly differ. In addition, for V-A trials, items with low-high (M = 0.01) and low-low (M = 0.15) phonotactic probabilities significantly differed, with low-high probability phonotactics predicting faster reaction times (β = −0.14, Std. error = 0.03, Z-ratio = −4.42, p < 0.0001) than low-low probability phonotactics. However, for V-A trials, items with high-low (M = 0.06) probability phonotactics did not significantly differ from any phonotactic level, and low-high (M =.01) and high-high (M =.01) phonotactic probabilities did not significantly differ.
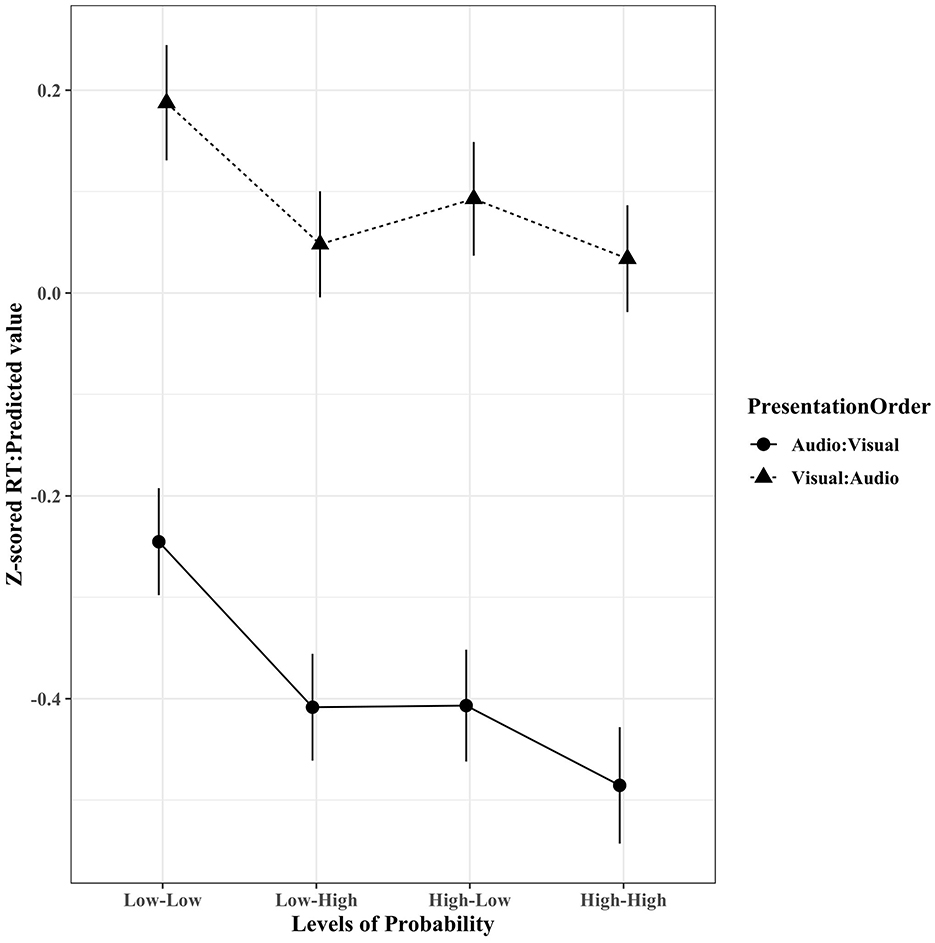
Figure 3. Experiment 2 used bisyllabic tokens. Reaction time data (z-scored) revealed a significant interaction between the variables (phonotactic) probability and presentation order. Error bars indicate 95% confidence interval.
Concerning the interaction between phonotactic probability and presentation order, it is possible that the use of bisyllabic stimuli allowed for different expectations to play a role in the results. Although overall comparisons made from audio-only to visual-only (A-V) trials were significantly faster than comparisons made from visual-only to auditory only (V-A) trials, variations occurred when accounting for phonotactic probability. For A-V trials, low-low probability items were responded to significantly slower than all other levels of phonotactic probability, and all other levels of phonotactic probability were found to be equivalent to each other. However, for V-A trials, a different pattern emerged that may be attributed to the initial visual stimulus creating a situation where observers processed the visual stimulus in a way that emphasizes the component parts (e.g., phoneme/viseme, syllables, etc.). Although low-low was significantly slower than low-high and high-high, and low-high was not found to be different from high-high, as in the phonotactic probability by lexicality interaction, here, high-low again seems to behave in a way that is contrary to expectation. In the present case, high-low was not found to be different from any other level of phonotactic probability. Again, it is possible that the violation of expectation from high to low creates a negative disruption, leading to a slower resonance state.
General discussion
The present study aimed to address three main questions by extending Vitevitch and Luce's (1999) unimodal study with a cross-modal implementation: (1) Can cross-modal speech research be conducted with various presentation orders? (2) What component(s) of a speech stimulus guides perception? and (3) should speech researchers be more cautious in overinterpreting the results obtained from relatively simple stimuli and deliberately follow up their research with more complex stimuli? With respect to the first question, the data from both experiments suggest yes. The results from experiments 1 and 2 suggest that cross-modal matches can occur successfully in both A-V and V-A directions. Although previous cross-modal studies have been reluctant to use a visual target (e.g., Buchwald et al., 2009; Kim et al., 2004), the current investigation is in line with the work of Sanchez et al. (2013), in supporting the idea that meaningful cross-modal research can occur using a visual target. When presented with a visual-only stimulus first, a perceiver may be able to call upon tacit knowledge of the ways that phonemes are typically sequenced (e.g., phonotactic probability) in their language to reduce the number of possible interpretations of that visual-only stimulus, greatly increasing the chance of correctly determining whether the second (audio-only) stimulus matches or not. This interpretation is borne out by the overall pattern of results across experiments and by recent neurophysiological evidence suggesting that late-stage integration processes in the posterior superior temporal sulcus may serve to constrain lexical competition during audiovisual spoken word recognition (Peelle and Sommers, 2015). In addition, this proposal is consistent with studies that have demonstrated that the same processes of perceptual similarity involved in the recognition of auditory words also play a role in visual-only spoken word recognition (e.g., Feld and Sommers, 2011; Strand and Sommers, 2011) and that perceptions of audio-only and visual-only words lead to similar changes in speech productions of observers (Miller et al., 2010, 2013; Sanchez, 2011).
With respect to question two, the results from our study are in line with the predictions made by ART: resonance occurs based on the most predictive units available and that no unit is special. In both experiments 1 and 2, we find that when word sized units are available, they will be used in perception, and when word sized units are not available, then smaller components (i.e., phonemes) will be used in perception. When the smaller units differ, where some are highly probable compared to less probable, the more probable (e.g., predictive) the unit, the quicker resonance is achieved.
With respect to question three, the results of the current study suggest that more complex stimuli should be used to support studies using simpler stimuli, but also to potentially reveal more. The stimulus complexity increased from experiment 1 to experiment 2 by employing a bisyllabic items (instead of monosyllabic items) while varying lexicality and phonotactic predictability and served as a replication and extension of experiment 1. As in experiment 1, experiment 2 also finds an interaction between phonotactic probability and lexicality. Specifically, the perception of non-words were subject to differences based on phonotactic probability, where items with higher probability components were responded to faster than items with low probability components, while word items were not perceived differently regardless of the phonotactic probability inherent in the words. However, in experiment 2, the bisyllabic nature of the stimuli revealed that indeed, items composed of low-low phonotactic probability are responded to the slowest, but that items composed of high-high probabilities was not necessarily the fastest, and that items with high-low probabilities might result in a processing disadvantage compared to high-high.
In addition, in experiment 2, we find a significant interaction between phonotactic probability and presentation order, which was not observed for experiment 1. The use of bisyllabic stimuli revealed that for words and non-words, items composed of low-low phonotactics were generally responded to at a slower rate than all other levels of probability, though in the V-A condition, items composed of high-low phonotactic probabilities were not found to be different from any other level. This suggests that again, high-low probabilities may result in a processing disadvantage.
However, it should be noted that there are limitations to the current study that provide opportunities for future research. An overwhelming number of studies, including this one, uses English language stimuli and English first language participants and may not be reflective of non-English speakers as noted by Blasi et al. (2022). Thus, we recommend future studies employ a diverse set of language stimuli and participants. In addition, the stimuli presented to participants reflected single tokens selected from a given set of speakers which may not reflect the variability one experiences in the natural world. In fact, Magnotti et al. (2020) finds that participants presented with a single talker's visual recording of an utterance and combined with the auditory recording of several different acoustic utterances in a McGurk experiment demonstrated a surprising range of successful McGurk integration rates that cautions researchers in overgeneralizing research based on single tokens. Moreover, experimental design choices were made for the current study due to past research that has found that cross-modal priming is hindered when words and non-words are intermixed (Kim et al., 2004). This led to the current study's experimental procedure to be designed on Vitevitch and Luce's (1999) experiment 1 which employed a same-different matching task where speech tokens varied by lexicality (word or non-words) and phonotactic probability (high or low). Words and non-words were presented in different blocked lists where half of the stimuli matched with equal values of high and low probability items (as in the current study). As such, a design with a mixed list presentation of words and non-words, as per Vitevitch and Luce's experiment 2 was not pursued, nor was a lexical decision task employed (experiments 3 and 5 from Vitevitch and Luce) due to inherently requiring a mixed list as well (as participants would need to respond to words and non-words equally in a block). In addition, a shadowing task (experiments 4 and 6 from Vitevitch and Luce) was also not employed; to date shadowing tasks on visual only stimuli have currently only been conducted on real words where participants are provided with support in the lipreading task by being presented with two possible words (e.g., “cabbage” and “camel”) that precedes a video of a speaker silently uttering one of the two possible words (Miller et al., 2010, 2013; Sanchez, 2011).
Notwithstanding, our study supports the validity of using different presentation orders in cross-modal research which may encourage researchers to address novel ways to conduct cross-modal research. In addition, the results of our study are in line with Grossberg's adaptive resonance theory (ART) framework, as the most predictive components of the stimuli guided perception. Finally, this study suggests that more complex stimuli can support the results from experiments using simpler stimuli, but can also uncover new information.
Data availability statement
The raw data supporting the conclusions of this article will be made available by the authors, without undue reservation.
Ethics statement
The studies involving humans were approved by Institutional Review Board at Cal Poly HumboldtIRB protocol# IRB 16-010. The studies were conducted in accordance with the local legislation and institutional requirements. The participants provided their written informed consent to participate in this study.
Author contributions
KS: Writing – original draft, Writing – review & editing.
Funding
The author(s) declare that no financial support was received for the research, authorship, and/or publication of this article.
Acknowledgments
I would like to thank Dr. Lorin Lachs for his support, feedback, and guidance in this project.
Conflict of interest
The author declares that the research was conducted in the absence of any commercial or financial relationships that could be construed as a potential conflict of interest.
Publisher's note
All claims expressed in this article are solely those of the authors and do not necessarily represent those of their affiliated organizations, or those of the publisher, the editors and the reviewers. Any product that may be evaluated in this article, or claim that may be made by its manufacturer, is not guaranteed or endorsed by the publisher.
Footnotes
1. ^Although this study positions itself with respect to ART as an explanatory model, other models of speech include, but are not limited to (see Dahan and Magnuson, 2006 for a review): the Cohort model (Gaskell and Marslen-Wilson, 1997), the TRACE model (McClelland and Elman, 1986), and the Neighborhood Activation Model (NAM; Luce and Pisoni, 1998). In their review of these theories of spoken word recognition, Dahan and Magnuson indicate that the ART framework holds promise for progressing our knowledge of spoken word recognition in a way that the others do not.
References
Arnold, P., and Hill, F. (2001). Bisensory augmentation: a speechreading advantage when speech is clearly audible and intact. Br J. Psychol. 92, 339–355. doi: 10.1348/000712601162220
Audacity 2.1.3 [Computer Software]. (2017). Pittsburgh: Carnegie Mellon University. Available at: http://audacity.sourceforge.net/
Auer, E. T. (2002). The influence of the lexicon on speech read word recognition: contrasting segmental and lexical distinctiveness. Psychonomic Bull. Rev. 9, 341–347. doi: 10.3758/BF03196291
Auer, E. T., Bernstein, L. E., and Tucker, P. E. (2000). Is subjective word familiarity a meter of ambient language? A natural experiment on the effects of perceptual experience. Mem. Cognit. 28, 789–797. doi: 10.3758/BF03198414
Baayen, R. H. (2008). Analyzing Linguistic Data: A Practical Introduction to Statistics Using R. Cambridge: Cambridge University Press. doi: 10.1017/CBO9780511801686
Baayen, R. H. (2009). Language R: Data sets and functions with “Analyzing Linguistic Data: A Practical Introduction To Statistics”. R Package Version 0.955. doi: 10.32614/CRAN.package.languageR
Baayen, R. H., Davidson, D. J., and Bates, D. M. (2008). Mixed-effects modeling with crossed random effects for subjects and items. J. Mem. Lang. 59, 390–412. doi: 10.1016/j.jml.2007.12.005
Barr, D. J., Levy, R., Scheepers, C., and Tily, H. J. (2013). Random effects structure for confirmatory hypothesis testing: Keep it maximal. J. Mem. Lang. 68, 255–278. doi: 10.1016/j.jml.2012.11.001
Bates, D., and Maechler, M. (2009). lme4: Linear Mixed-Effects Models Using S4 Classes. [Software] (R Package Version). doi: 10.32614/CRAN.package.lme4
Bernstein, L. E., Auer, E. T., and Takayanagi, S. (2004). Auditory speech detection in noise enhanced by lip-reading. Speech Commun. 44, 5–18. doi: 10.1016/j.specom.2004.10.011
Blasi, D. E., Henrich, J., Adamou, E., Kemmerer, D., and Majid, A. (2022). Over-reliance on English hinders cognitive science. Trends Cognit. Sci. 26, 1153–1170. doi: 10.1016/j.tics.2022.09.015
Buchwald, A. B., Winters, S. J., and Pisoni, D. B. (2009). Visual speech primes open-set recognition of spoken words. Lang. Cogn. Proc. 24, 580–610. doi: 10.1080/01690960802536357
Clark, H. H. (1973). The language-as-fixed-effect fallacy: a critique of language statistics in psychological research. J. Verbal Learn. Verbal Behav. 12, 335–359. doi: 10.1016/S0022-5371(73)80014-3
Dahan, D., and Magnuson, J. S. (2006). “Spoken word recognition”, in Handbook of Psycholinguistics (Academic Press), 249–283. doi: 10.1016/B978-012369374-7/50009-2
Development Core Team, R. (2009). R: A Language and Environment for Statistical Computing. Vienna: R. Foundation for Statistical Computing.
Faust, M. E., Balota, D. A., Spieler, D. H., and Ferraro, F. R. (1999). Individual differences in information-processing rate and amount: implications for group differences in response latency. Psychol. Bull. 125:777. doi: 10.1037/0033-2909.125.6.777
Feld, J., and Sommers, M. (2011). There goes the neighborhood: lipreading and the structure of the mental lexicon. Ear Hear. 53, 220–228. doi: 10.1016/j.specom.2010.09.003
Galantucci, B., Fowler, C. A., and Turvey, M. T. (2006). The motor theory of speech perception reviewed. Psychonomic Bull. Rev. 13, 361–377. doi: 10.3758/BF03193857
Gaskell, M. G., and Marslen-Wilson, W. D. (1997). Integrating form and meaning: a distributed model of speech perception. Lang. Cognit. Processes 12, 613–656. doi: 10.1080/016909697386646
Gaygen, D. E. (1998). The effects of probabilistic phonotactics on the segmentation of continuous speech. (dissertation). University at Buffalo, Buffalo, NY. doi: 10.1121/1.423694
Goldinger, S. D. (1998). Echoes of echoes? An episodic theory of lexical access. Psychol. Rev. 105, 251–279. doi: 10.1037/0033-295X.105.2.251
Goldinger, S. D., and Azuma, T. (2003). Puzzle-solving science: the quixotic quest for units in speech perception. J. Phonetics 31, 305–320. doi: 10.1016/S0095-4470(03)00030-5
Grant, K. W., and Seitz, P. (2000). The use of visible speech cues for improving auditory detection of spoken sentences. J. Acoust. Soc. Am. 108, 1197–1208. doi: 10.1121/1.1288668
Grossberg, S. (2013). Adaptive resonance theory: how a brain learns to consciously attend, learn, and recognize a changing world. Neural Networks 37, 1–47. doi: 10.1016/j.neunet.2012.09.017
Grossberg, S. (2021). Conscious Mind, Resonant Brain: How Each Brain Makes a Mind. New York, NY: Oxford University Press. doi: 10.1093/oso/9780190070557.001.0001
Jusczyk, P. W., Luce, P. A., and Charles-Luce, J. (1994). Infants sensitivity of phonotactic patterns in the native language. J. Mem. Lang. 33, 630–645. doi: 10.1006/jmla.1994.1030
Kim, J., and Davis, C. (2004). Integrating the audio-visual speech detection advantage. Speech Commun. 44, 19–30. doi: 10.1016/j.specom.2004.09.008
Kim, J., Davis, C., and Krins, P. (2004). Amodal processing of visual speech as revealed by priming. Cognition 93, B39–B47. doi: 10.1016/j.cognition.2003.11.003
Kucera, H., and Francis, W. N. (1967). Computational Analysis of Present-Day American English. Providence, RI: Brown University Press.
Lachs, L., and Pisoni, D. B. (2004). Crossmodal source identification in speech perception. Ecol. Psychol. 16, 159–187. doi: 10.1207/s15326969eco1603_1
Lenth, R. V. (2021). Emmeans: Estimated Marginal Means, aka Least-Squares Means. R package version 1.6.1. Available at: https://CRAN.R-project.org/package=emmeans
Liberman, A. M., Cooper, F. S., Shankweiler, D. P., and Studdert-Kennedy, M. (1967). Perception of the speech code. Psychol. Rev. 74:431. doi: 10.1037/h0020279
Liberman, A. M., and Mattingly, I. G. (1985). The motor theory of speech perception revised. Cognition 21, 1–36. doi: 10.1016/0010-0277(85)90021-6
Luce, P. A. (1986). A computational analysis of uniqueness points in auditory word recognition. Percept. Psychophysics 39, 155–158. doi: 10.3758/BF03212485
Luce, P. A., and Pisoni, D. B. (1998). Recognizing spoken words: the neighborhood activation model. Ear Hear. 19, 1–36. doi: 10.1097/00003446-199802000-00001
Magnotti, J. F., Dzeda, K. B., Wegner-Clemens, K., Rennig, J., and Beauchamp, M. S. (2020). Weak observer-level correlation and strong stimulus-level correlation between the McGurk effect and audiovisual speech-in-noise: a causal inference explanation. Cortex 133, 371–383. doi: 10.1016/j.cortex.2020.10.002
Marslen-Wilson, W. D., and Tyler, L. K. (1980). The temporal structure of spoken language understanding. Cognition 8, 1–71. doi: 10.1016/0010-0277(80)90015-3
Mattys, S. L., Bernstein, L. E., and Auer, E. T. (2002). Stimulus-based lexical distinctiveness as a general word-recognition mechanism. Percept. Psychophysics 64, 667–679. doi: 10.3758/BF03194734
McClelland, J. L., and Elman, J. L. (1986). The TRACE model of speech perception. Cognit. Psychol. 18, 1–86. doi: 10.1016/0010-0285(86)90015-0
McGurk, H., and MacDonald, J. (1976). Hearing lips and seeing voices. Nature 264, 746–748. doi: 10.1038/264746a0
Merriam-Webster (1967). The New Merriam-Webster's Pocket Dictionary. New York, NY: Pocket Cardinal, a division of Simon and Schuster.
Miller, R. M., Sanchez, K., and Rosenblum, L. D. (2010). Alignment to visual speech information. Attention Percept. Psychophysics 72, 1614–1625. doi: 10.3758/APP.72.6.1614
Miller, R. M., Sanchez, K., and Rosenblum, L. D. (2013). Is speech alignment to talkers or tasks? Attention Percept. Psychophysics 75, 1817–1826. doi: 10.3758/s13414-013-0517-y
Peelle, J. E., and Sommers, M. S. (2015). Prediction and constraint in audiovisual speech perception. Cortex 68, 169–181. doi: 10.1016/j.cortex.2015.03.006
Psychology Software Tools, Inc.. (2012). [E-Prime 2.0]. Available at: http://www.pstnet.com
Ratcliff, R. (1993). Methods for dealing with reaction time outliers. Psychol. Bull. 114, 510–532. doi: 10.1037/0033-2909.114.3.510
Reisberg, D., McLean, J., and Goldfield, A. (1987). “Easy to hear but hard to understand: A lip-reading advantage with intact auditory stimuli,” in Hearing by Eye: The Psychology of Lip Reading, eds. B. Dodd and R. Campbell (Hillsdale, New Jersey: Lawrence Erlbaum Associates).
Rosenblum, L. (2019). Audiovisual Speech Perception and the McGurk Effect. Oxford Research Encyclopedia, Linguistics. Oxford University Press. doi: 10.1093/acrefore/9780199384655.013.420
Rosenblum, L. D., Dias, J. W., and Dorsi, J. (2017). The supramodal brain: implications for auditory perception. J. Cognit. Psychol. 29, 65–87. doi: 10.1080/20445911.2016.1181691
Rosenblum, L. D., Miller, R. M., and Sanchez, K. (2007). Lipread me now, hear me better later: crossmodal transfer of talker familiarity effects. Psychol. Sci. 18, 392–396. doi: 10.1111/j.1467-9280.2007.01911.x
Sanchez, K. (2011). Do you hear what I see? The voice and face of a talker similarly influence the speech of multiple listeners. (dissertation). University of California, Riverside.
Sanchez, K., Dias, J., and Rosenblum, L. D. (2013). Experience with a talker can transfer across modalities to facilitate lipreading. Attention Percept. Psychophysics 75, 1359–1365. doi: 10.3758/s13414-013-0534-x
Strand, J. F., and Sommers, M. S. (2011). Sizing up the competition: quantifying the influence of the mental lexicon on auditory and visual spoken word recognition. J. Acoust. Soc. Am. 130:1663. doi: 10.1121/1.3613930
Sumby, W. H., and Pollack, I. (1954). Visual contribution to speech intelligibility in noise. J. Acoust. Soc. Am. 26, 212–215.doi: 10.1121/1.1907309
Vitevitch, M., and Luce, P. (1999). Probabilistic phonotactics and neighborhood activation in spoken word recognition. J. Mem. Lang. 40, 374–408. doi: 10.1006/jmla.1998.2618
Keywords: audio-visual speech perception, multisensory perception, cross-modal speech, psycholinguistics, language processing
Citation: Sanchez K (2025) Cross-modal matching of monosyllabic and bisyllabic items varying in phonotactic probability and lexicality. Front. Lang. Sci. 4:1488399. doi: 10.3389/flang.2025.1488399
Received: 29 August 2024; Accepted: 13 January 2025;
Published: 12 February 2025.
Edited by:
Alejandro Javier Wainselboim, CONICET Mendoza, ArgentinaReviewed by:
Yifei He, University of Marburg, GermanyClaudia Marzi, Antionio Zampolli Institute of Computational Linguistics National Research Council, Italy
Copyright © 2025 Sanchez. This is an open-access article distributed under the terms of the Creative Commons Attribution License (CC BY). The use, distribution or reproduction in other forums is permitted, provided the original author(s) and the copyright owner(s) are credited and that the original publication in this journal is cited, in accordance with accepted academic practice. No use, distribution or reproduction is permitted which does not comply with these terms.
*Correspondence: Kauyumari Sanchez, a2F1eXVtYXJpLnNhbmNoZXpAaHVtYm9sZHQuZWR1