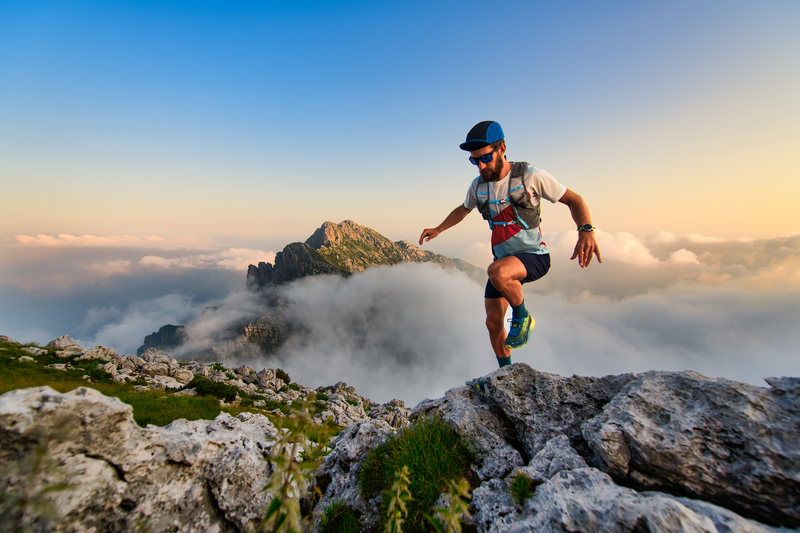
94% of researchers rate our articles as excellent or good
Learn more about the work of our research integrity team to safeguard the quality of each article we publish.
Find out more
ORIGINAL RESEARCH article
Front. Immunol.
Sec. Cancer Immunity and Immunotherapy
Volume 16 - 2025 | doi: 10.3389/fimmu.2025.1567102
This article is part of the Research Topic Neural influences on tumor immunity: Exploring neuroimmunology in cancer View all articles
The final, formatted version of the article will be published soon.
You have multiple emails registered with Frontiers:
Please enter your email address:
If you already have an account, please login
You don't have a Frontiers account ? You can register here
Background: Colorectal cancer (CRC) is one of the malignant tumours in the digestive tract, which affects the health of people to a large extent. LDL receptor-related protein 1B (LRP1B) might serve as a crucial role in tumorigenesis and disease progression.We conducted a comparative analysis of differential gene expression, mutation patterns, drug sensitivity and cellular phenotypes among different subgroup of LRP1B expression level. Cellular and molecular experiments were further conducted to confirm the results of our analysis.Results: LRP1B was implicated as a tumortumour suppressor gene in our analysis. The results of experiments verified that the expression of LRP1B was decreased in CRC, and knocking down it was associated with poor prognosis. The molecular mechanism study revealed that LRP1B took negative acts on Hedgehog (Hh) signalingsignalling pathway to impact the cell cycle and apoptosis programs. Single cell analysis showed that T cells, B cells, epithelial cells and myeloid cells were significantly differentially infiltrated in high and low expression of LRP1B groups. Immune cell infiltration and drug sensitivities analysis demonstrated LRP1B played a markedly important role in immunotherapy or targeted therapy, implicating that restoring the function of LRP1B would be a prospective treatment target in CRC.Our results demonstrated that LRP1B may function as a tumortumour suppressor factor in CRC, which has significance in mutation, therapy and immune infiltration. Knockdown of LRP1B can activate the Hh pathway in tumor cells to inhibit several malignant biological behaviorsbehaviours.
Keywords: colorectal cancer (CRC), LRP1B, hedgehog (Hh) signaling pathway, Immune Cell Infiltration, Tumor Microenvironment
Received: 26 Jan 2025; Accepted: 21 Feb 2025.
Copyright: © 2025 Chong, Liu, Zhong, Sang, Kang, Zhu, Cui, Liu, Wang, Chen, Jing and Li. This is an open-access article distributed under the terms of the Creative Commons Attribution License (CC BY). The use, distribution or reproduction in other forums is permitted, provided the original author(s) or licensor are credited and that the original publication in this journal is cited, in accordance with accepted academic practice. No use, distribution or reproduction is permitted which does not comply with these terms.
* Correspondence:
Wei Chong, Shandong Provincial Hospital, Jinan, China
Disclaimer: All claims expressed in this article are solely those of the authors and do not necessarily represent those of their affiliated organizations, or those of the publisher, the editors and the reviewers. Any product that may be evaluated in this article or claim that may be made by its manufacturer is not guaranteed or endorsed by the publisher.
Research integrity at Frontiers
Learn more about the work of our research integrity team to safeguard the quality of each article we publish.