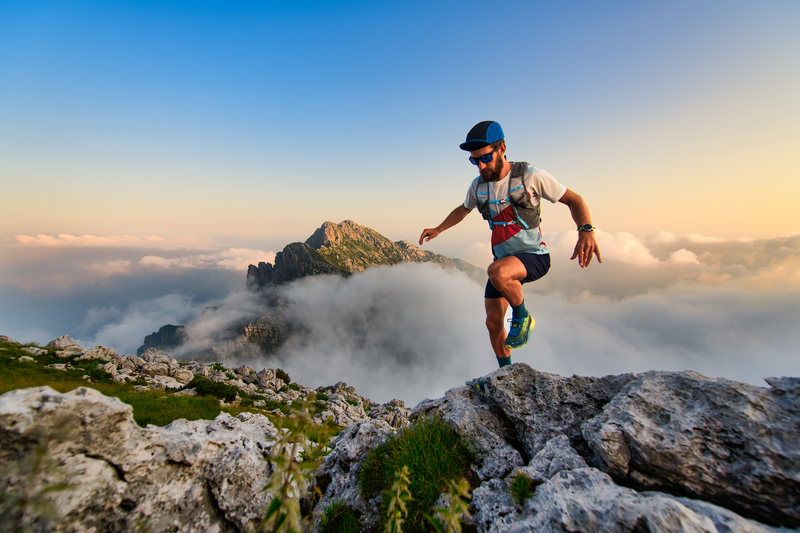
94% of researchers rate our articles as excellent or good
Learn more about the work of our research integrity team to safeguard the quality of each article we publish.
Find out more
ORIGINAL RESEARCH article
Front. Immunol.
Sec. T Cell Biology
Volume 16 - 2025 | doi: 10.3389/fimmu.2025.1536302
The final, formatted version of the article will be published soon.
You have multiple emails registered with Frontiers:
Please enter your email address:
If you already have an account, please login
You don't have a Frontiers account ? You can register here
The functional programs of CD4 + helper (Th) T cell clones play a central role in shaping immune responses to different challenges. While advances in scRNA-Seq have significantly improved our understanding of Th cell diversity, the relationship between scRNA-Seq clusters and traditionally characterized Th subsets remains ambiguous. In this study, we introduce TCR-Track, a method leveraging immune repertoire data to map phenotypically sorted Th subsets onto scRNA-Seq profiles. This approach accurately positions Th1, Th1-17, Th17, Th22, Th2a, Th2, Tfh, and Treg subsets, outperforming mapping based on CITE-Seq. Remarkably, the mapping is tightly focused on specific scRNA-Seq clusters despite a four-year interval between subset sorting and the effector CD4 + scRNA-Seq experiment. These findings highlight the intrinsic program stability of Th clones circulating in peripheral blood. Repertoire overlap analysis at the scRNA-Seq level confirms that circulating Th1, Th2, Th2a, Th17, Th22, and Treg subsets are clonally independent. However, a significant clonal overlap between Th1 and cytotoxic CD4 + T cell clusters suggests that cytotoxic CD4 + T cells differentiate from Th1 clones. Additionally, our study resolves a longstanding ambiguity: we demonstrate that, while CCR10 + Th cells align with a specific Th22 scRNA-Seq cluster, CCR10⁻CCR6⁺CXCR3⁻CCR4⁺ cells, typically classified as Th17, represent a mixture of bona fide Th17 cells and clonally unrelated CCR10 low Th22 cells. The clear distinction between Th17 and Th22 subsets should influence vaccine and T cell-based therapies development. Furthermore, we show that SARS-CoV-2 infection induces systemic IFN type 1 activation of naive helper T cells. An increased proportion of effector IFN-induced Th cells is associated with a moderate course of the disease but remains low in critical COVID-19 cases. Using integrated scRNA-Seq, TCR-Track, and CITE-Seq data from 122 donors, we provide a comprehensive Th scRNA-Seq reference that should facilitate further investigation of Th subsets in fundamental and clinical studies.
Keywords: Helper T cell subsets, ScRNA-seq, scTCR-seq, immune repertoires, T cell memory, Th22, Th17, cytotoxic CD4+ T cells
Received: 28 Nov 2024; Accepted: 21 Feb 2025.
Copyright: © 2025 Chudakov, Lukyanov, Kriukova, Ladell, Shagina, Staroverov, Minasian, Fedosova, Shelyakin, Suchalko, Komkov, Blagodatskikh, Miners, Britanova, Franke and Price. This is an open-access article distributed under the terms of the Creative Commons Attribution License (CC BY). The use, distribution or reproduction in other forums is permitted, provided the original author(s) or licensor are credited and that the original publication in this journal is cited, in accordance with accepted academic practice. No use, distribution or reproduction is permitted which does not comply with these terms.
* Correspondence:
Dmitry M Chudakov, Masaryk University, Brno, Czechia
Disclaimer: All claims expressed in this article are solely those of the authors and do not necessarily represent those of their affiliated organizations, or those of the publisher, the editors and the reviewers. Any product that may be evaluated in this article or claim that may be made by its manufacturer is not guaranteed or endorsed by the publisher.
Research integrity at Frontiers
Learn more about the work of our research integrity team to safeguard the quality of each article we publish.