- 1Department of Radiation Oncology, Shandong Cancer Hospital and Institute, Shandong First Medical University and Shandong Academy of Medical Sciences, Jinan, Shandong, China
- 2Department of Pathology, Shandong Cancer Hospital and Institute, Shandong First Medical University and Shandong Academy of Medical Sciences, Jinan, Shandong, China
- 3The Second School of Clinical Medicine, Zhejiang Chinese Medical University, Hangzhou, Zhejiang, China
- 4Department of Radiology, Zhejiang Cancer Hospital, Hangzhou Institute of Medicine (HIM), Chinese Academy of Sciences, Hangzhou, Zhejiang, China
Background: This study aimed to develop a multi-modality model by incorporating pretreatment computed tomography (CT) radiomics and pathomics features along with clinical variables to predict pathologic complete response (pCR) to neoadjuvant chemoimmunotherapy in patients with locally advanced esophageal cancer (EC).
Method: A total of 223 EC patients who underwent neoadjuvant chemoimmunotherapy followed by surgical intervention between August 2021 and December 2023 were included in this study. Radiomics features were extracted from contrast-enhanced CT images using PyrRadiomics, while pathomics features were derived from whole-slide images (WSIs) of pathological specimens using a fine-tuned deep learning model (ResNet-50). After feature selection, three single-modality prediction models and a combined multi-modality model integrating two radiomics features, 11 pathomics features, and two clinicopathological features were constructed using the support vector machine (SVM) algorithm. The performance of the models were evaluated using receiver operating characteristic (ROC) analysis, calibration plots, and decision curve analysis (DCA). Shapley values were also utilized to explain the prediction model.
Results: The predictive capability of the multi-modality model in predicting pCR yielded an area under the curve (AUC) of 0.89 (95% confidence interval [CI], 0.75-1.00), outperforming the radiomics model (AUC 0.70 [95% CI 0.54-0.85]), pathomics model (AUC 0.77 [95% CI 0.53-1.00]), and clinical model (AUC 0.63 [95% CI 0.46-0.80]). Additionally, both the calibration plot and DCA curves support the clinical utility of the integrated multi-modality model.
Conclusions: The combined multi-modality model we propose can better predict the pCR status of esophageal cancer and help inform clinical treatment decisions.
Introduction
Esophageal cancer (EC) is one of the major types of cancer worldwide, with surgical therapy being the primary treatment modality. However, outcomes are often suboptimal due to early postoperative recurrence (1, 2). Large-scale randomized clinical trials have shown that neoadjuvant chemoimmunotherapy followed by surgery is more effective than surgery alone for locally advanced EC. Preliminary findings suggest that esophagectomy following neoadjuvant chemoimmunotherapy achieves satisfactory efficacy and manageable safety, with pathologic complete response (pCR) rates ranging from 16.7% to 50.0% (3–5). Patients achieving pCR exhibit a more favorable long-term prognosis compared to those without pCR and may benefit from wait-and-see strategies (6–8). Nevertheless, a subset of patients does not respond to neoadjuvant chemoimmunotherapy, potentially incurring high drug costs and immunotherapy-related adverse events (irAEs). Therefore, accurately predicting the response to neoadjuvant chemoimmunotherapy before surgery is of great clinical significance as it could identify patients who are likely to benefit and inform appropriate and personalized treatment plans.
Radiomics, a promising method that extracts high-throughput quantitative data from medical images, has been successfully applied in precision diagnosis, treatment response prediction, and prognosis assessment for various types of malignancies (9, 10). Several radiomics- based models have been proposed to predict pCR in EC patients undergoing neoadjuvant chemoradiotherapy (11, 12).However, limited research has explored the utilization of radiomics in assessing the response to neoadjuvant chemoimmunotherapy, and there is a need to improve the prediction accuracy of existing models (13). An optimal strategy that incorporates additional dimensions is essential for enhancing the performance of prediction models.
With the advent of digital pathology, quantitative analyses based on artificial intelligence have increased in the field of EC, demonstrating strong performance in early diagnosis, treatment response assessment, and survival prediction (14–16). Unlike radiomics features, digital pathology provides insights into molecular characteristics or genetic patterns, potentially complementing tumor heterogeneity and improving the predictive accuracy of current models. However, to our knowledge, there is a lack of academic research integrating pretreatment CT-derived radiomics features with pathomics features derived from whole-slide imaging (WSI) for predicting pCR in EC.
In this retrospective study, we aimed to develop and compare a multi-modality machine learning model by integrating pretreatment CT radiomics, pathomics features, and clinical variables to predict pCR in esophageal cancer prior to the initiation of therapy.
Materials and methods
Patients
This retrospective study was approved by the Ethics Committee of Shandong Cancer Hospital and Institute and the requirement for written informed consent was waived. A specific flowchart of patient selection is displayed in Figure 1, and an overview of the entire architecture of the study is provided in Figure 2.
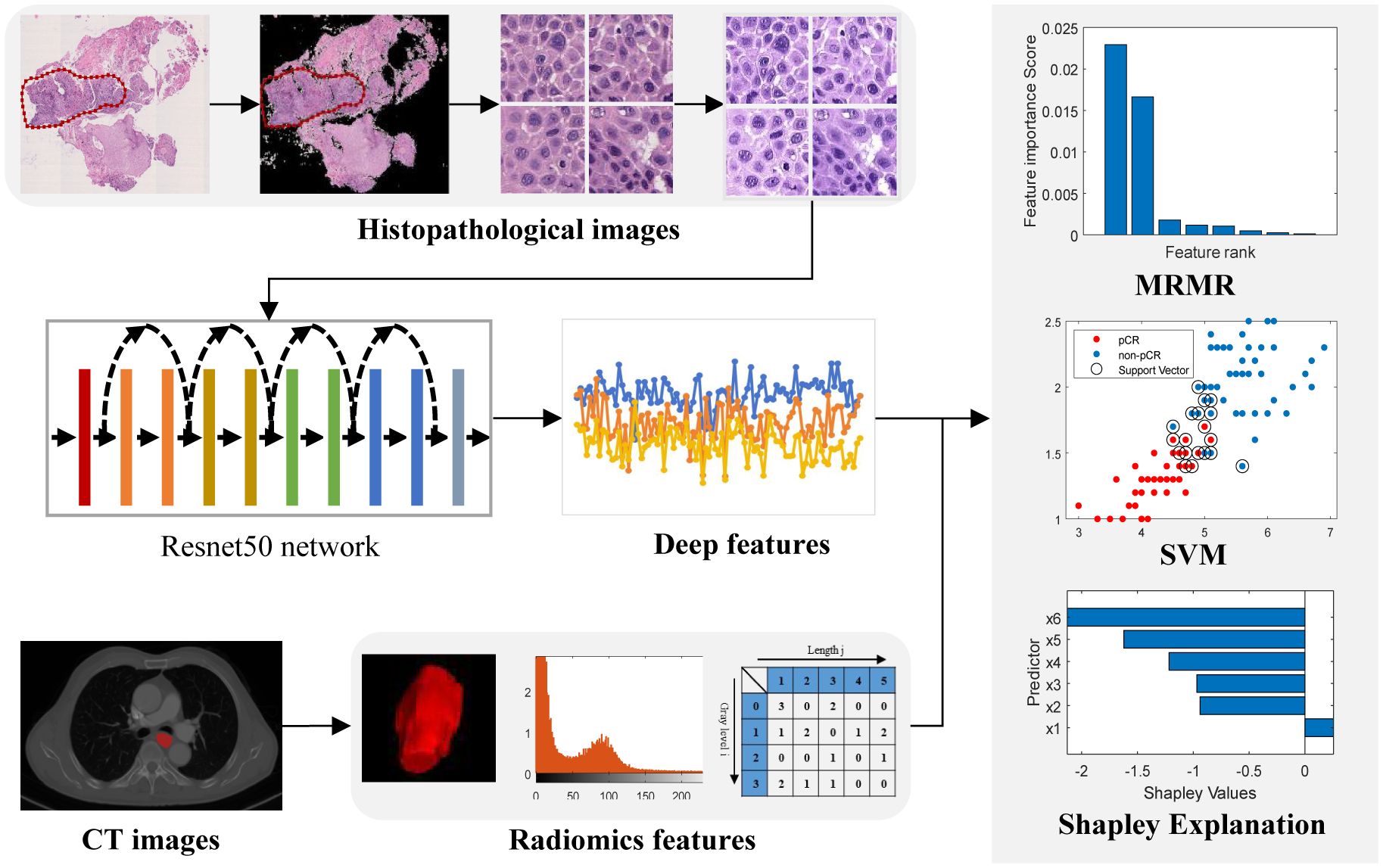
Figure 2. Workflow of the study. Images of pretreatment CT images and biopsy hematoxylin and eosin-stained slides were retrospectively retrieved and segmented for feature extraction. A two-sided sample t-test or U-test and the maximum relevance–minimum redundancy (MRMR) algorithm was used to select features. The top features with scores larger than zero were retained to develop a classifier model by a Support Vector Machine (SVM) method.
The inclusion criteria were as follows: (1) pathological biopsy confirming EC with no distant metastasis; (2) age between 18 and 75 years. The exclusion criteria were: (1) treatment with chemotherapy, radiotherapy, or other anticancer therapies prior to baseline CT scans; (2) synchronous tumors or a history of other malignancies; and (3) no surgical treatment following chemoimmunotherapy.
A total of 223 patients with histologically proven EC who underwent neoadjuvant chemoimmunotherapy and surgical treatment between August 2021 and December 2023 at Shandong Cancer Hospital and Institute were recruited for this study. Among these, 214 patients with pretreatment CT images were enrolled in the radiomics cohort to build a radiomics model. Additionally, 89 patients with WSI were used to construct the pathomics model. To further evaluate the ability of pathomics features to predict pCR when combined with CT-based radiomics and clinicopathological features, a combined multi-modality model was developed in 80 patients with both pretreatment CT and WSI. Patients were randomly allocated to the training and testing sets in a 3:1 ratio.
Evaluation of tumor response
The pathological response was assessed by examining the percentage of residual viable tumor cells in the resected specimens, based on the immune-mediated pathological response criteria (irPRC) (17, 18). Surgical specimens were evaluated by two pathologists who were blinded to clinical information and CT images (Figure 3). pCR was defined as the absence of viable tumor cells in both the primary tumor and the lymph nodes (Figure 3A).
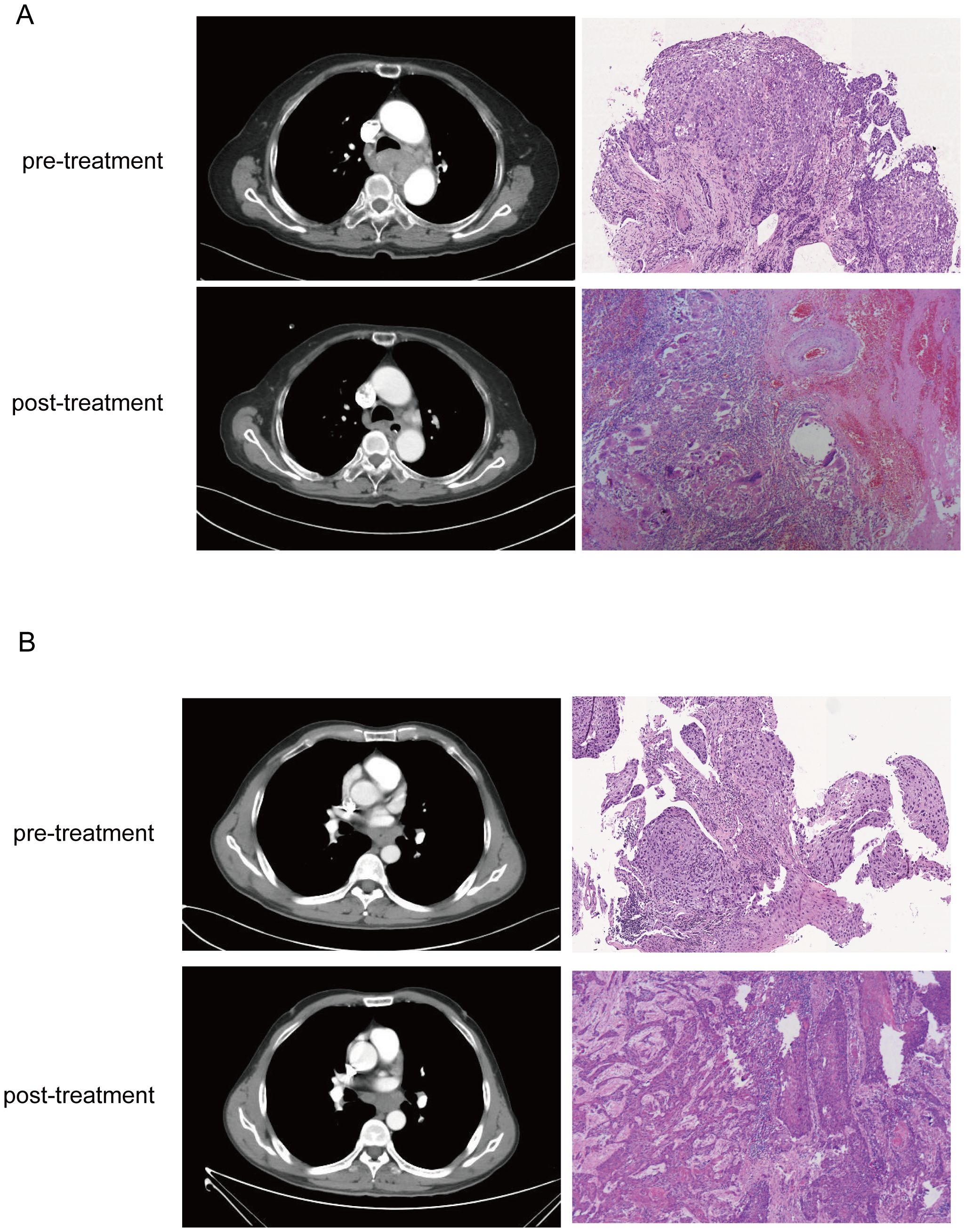
Figure 3. Corresponding CT images and WSIs from pCR (A) and non-pCR (B) before and after neoadjuvant treatment. In the CT image, it is seen that the tumor in the pCR have completely dissipated in the post-treatment image, and stromal tissue with no visible tumor cells was presented in the pathological images. But CT and histology images from non-pCR shows residual tumor cells but reduced compared to baseline.
CT images and WSI acquisition
Contrast-enhanced CT scans were performed using a SIEMENS CT scanner before treatment. The scanning parameters were as follows: slice thickness of 5.0 mm, tube voltage of 120 kV, and tube current of 220 mA. An iodinated contrast agent (300 mg/mL) at a dose of 1.5 ml/kg of body mass was injected rapidly at a flow rate of 2 mL/s through the patient’s elbow vein using a high-pressure syringe. To maintain data consistency, only arterial phase CT images were collected. All acquired CT images were extracted from the institutional picture archiving and communication system (PACS) and saved in DICOM format.
H&E-stained slides from formalin-fixed paraffin-embedded biopsy tissues were used for pathological diagnosis. WSIs for analysis were scanned using a Panoramic SCAN II scanner at 40× objective magnification. All images were obtained in NDPI format for subsequent region of interest (ROI) annotation and pathological feature extraction.
Image segmentation and feature extraction
Two experienced radiologists with 5 and 8 years of experience, who were blinded to the clinical and/or pathological data, manually delineated the regions of tumor on CT images with the ITK-SNAP software (version 3.6). The region of interest (ROI) was delineated to encompass the primary tumor volume in all slices. Before feature extraction, the CT image intensities were normalized to a scale of 2048 and re-binned by a width of 16 to avoid the influence of the sparse matrix on the calculation of texture features. And then the images of all patients were resampled to the same voxel size of 0.8 mm×0.8 mm×5 mm using the ‘sitkBSpline’ interpolator, in order to minimize the differences of images among patients. In total, 851 features, including shape, first-order, texture, and wavelet features, were computed and extracted using PyRadiomics (19) in Python 3.6.
For digital pathology image analysis, ASAP (version 2.1), an open-source software, was employed to annotate the tumor regions within the WSI. The original WSIs were converted from RGB to gray and a threshold of 220 was used to segment the tissue regions on the gray WSIs. Subsequently, the tissues in the tumor regions were subdivided into non-overlapping 224×224 tiles at a resolution of 0.5 μm/pixel (20× magnification). Tiles with less than 50% tissue were excluded from the analysis. Color normalization was performed on each tile to normalize the color variations caused by different staining and scanning of pathology images based on the Vahadane Method (20). The deep features of each tile were extracted using a pretrained ResNet50 backbone. The tiles were input into the ResNet50 backbone and the 2048 outputs at the fully connected layer were treated as 2048 deep features for each patch. For each deep feature, the median value of all tiles for one patient was treated as its feature value, resulting in 2048 deep features for each patient.
Feature selection and model construction
Feature selection was performed separately for CT radiomics and pathomics features in the training set. The features were first normalized using z-scores and tested for normality. A two-sided sample t-test or U-test was applied to identify features showing significant differences between the pCR and non-pCR groups. Finally, features with p<0.1 were selected and further ranked using a maximum relevance-minimum redundancy (MRMR) algorithm (21, 22). Top features with scores greater than zero were retained for the classifier model. In addition to radiomics and pathomics features, critical clinical characteristics, including age, sex, smoking history, histology, treatment cycles, T-stage, N-stage, and clinical stage, were incorporated to select the feature set for constructing the multimodal prediction model.
Three single-modality prediction models—radiomics, pathomics, and clinical models—were constructed using a Support Vector Machine (SVM) approach. The SVM model, employing a radial basis function (RBF) kernel, was optimized through five-fold cross-validation using data from the training set. Subsequently, we developed and validated a multimodal model that integrated all selected radiomics features with pathomics features and significant clinical factors. The performance of these models in predicting pCR was assessed in both the training and testing sets using receiver operating characteristic (ROC) curve analysis and area under the curve (AUC). Youden’s index was used to determine the optimal threshold for converting model output scores into predicted classes. The accuracy, sensitivity, specificity, positive predictive value (PPV), and negative predictive value (NPV) were calculated. Calibration curves were used to evaluate the goodness-of-fit of the models, and decision curve analysis (DCA) was performed to quantify the net benefit to patients at different threshold probabilities, thus assessing the clinical utility of the predictive models developed in this study.
To further evaluate the contribution of the combined features to the multi-modality model prediction, the SHAP value was calculated to decompose the SVM model decision into individual feature influences for each sample. SHAP facilitates the visualization of feature importance within complex machine learning-based models, elucidating how individual features impact the likelihood of a particular output, either positively or negatively.
Statistical analysis
Python3.7 was used for feature extraction, feature selection, machine learning model training, model evaluation, and plotting. SPSS (version 26.0) was used for the t-test, chi-square test, and Fisher’s exact test. The ROC curve was plotted for model performance evaluation. AUC was calculated, and bootstrapping was utilized for calculating the 95% confidence interval (95% CI). Continuous variables are presented as mean ± standard deviation, and categorical variables are presented as counts (%). Student’s t-test was used to compare continuous variables, and the chi-square test or Fisher’s exact test was used to compare categorical variables. Statistical significance was set at p<0.05.
Results
Patient population characteristics
The baseline clinical characteristics and pCR distribution of the patients in each cohort are summarized in Tables 1 and 2, respectively. Patients achieving pCR accounted for 28% (60/214) and 28.1% (25/89) in the radiomics and pathomics groups, respectively. There were no statistically significant differences in baseline characteristics between patients with and without pCR, except for sex (p=0.015) in the pathomics cohort.
Feature selection and interpretation
In our study, 851 radiomics features were extracted from ROIs on CT images, and 2048 deep learning features (pathomics features) were extracted from pathological section images. We performed an independent feature-selection strategy for each feature set within the training cohort. Following dimensionality reduction, 10 radiomics features, 20 pathomics features, and 15 combined features significantly associated with pCR were retained. The selected features of these model are listed in Table 3 and Figure 4A.
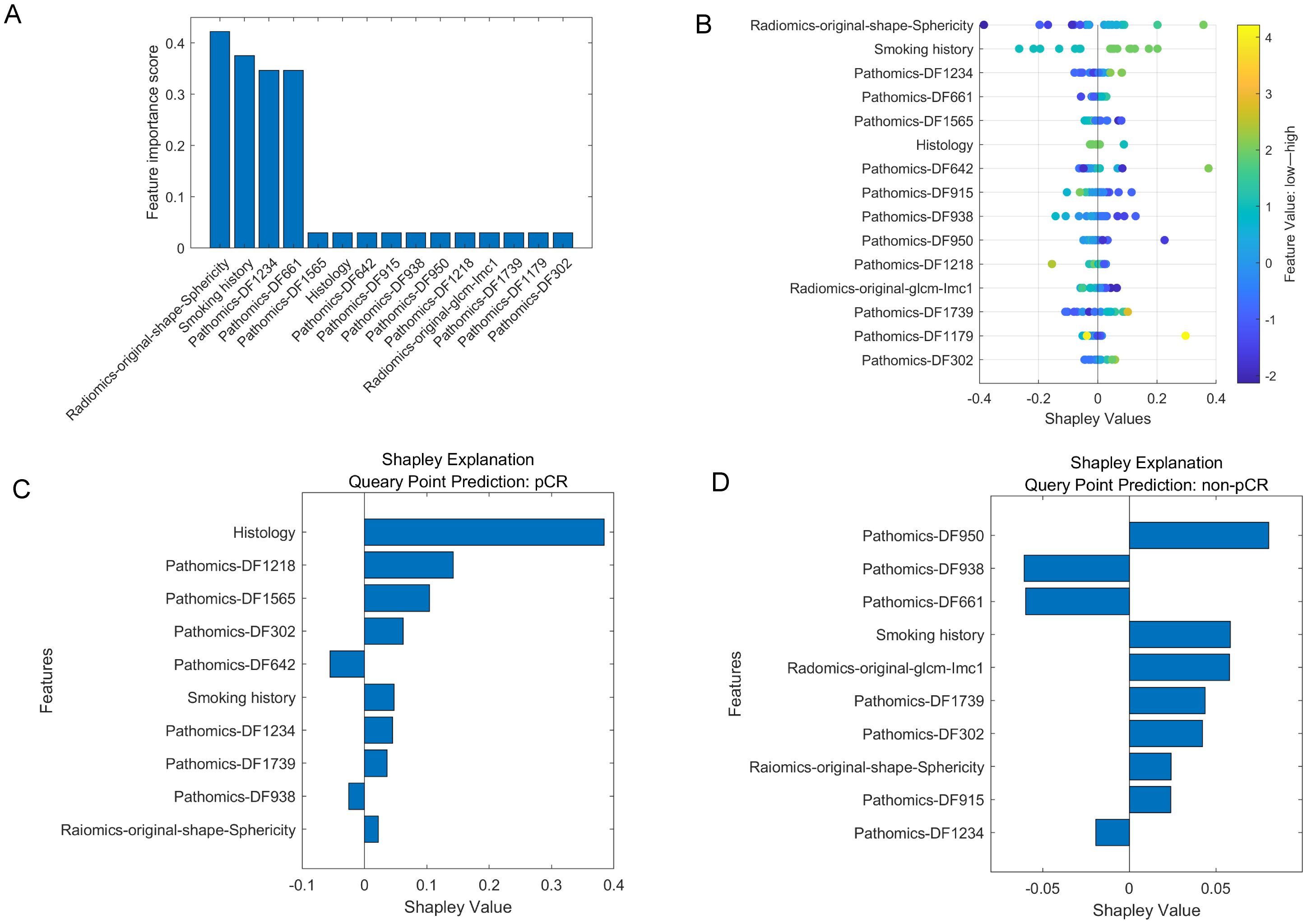
Figure 4. The selected features in multi-modality model. (A). The importance scores of the top 15 features chosen by the MRMR. (B). SHAP values of the multi-modality model for overall patient cohort. (C, D). SHAP values of the multi-modality model for cases with pCR and non-pCR, respectively. A SHAP value larger than zero indicates a positive impact, while a SHAP value smaller than zero indicates a negative impact. The features with absolute SHAP values smaller than 0.01 were not shown in this figure.
To further evaluate the contribution of the combined features to the multi-modality model prediction, the SHAP value was calculated to decompose the SVM model decision into individual feature influences for each sample. Figure 4B shows the SHAP values of the multi-modality model for overall patient cohort, as well as Figure 4C, D show the impact of the important features on the model output in one pCR case and one non-pCR case, respectively. For the pCR case, the “Histology” was the feature that contributed the most to the model output, while four pathomics features also showed great impact. For the non-pCR case, three pathomics features corresponded to an increased likelihood of non-pCR.
Performance comparison of the prediction models
By integrating clinical, radiomics, and pathomics features in the training set, a combined multi-modality prediction model was developed using the nonlinear SVM method. The distributions of combined scores are illustrated in Figure 5, with an increase in combined score, and more participants achieving pCR were identified in the training and testing cohorts.
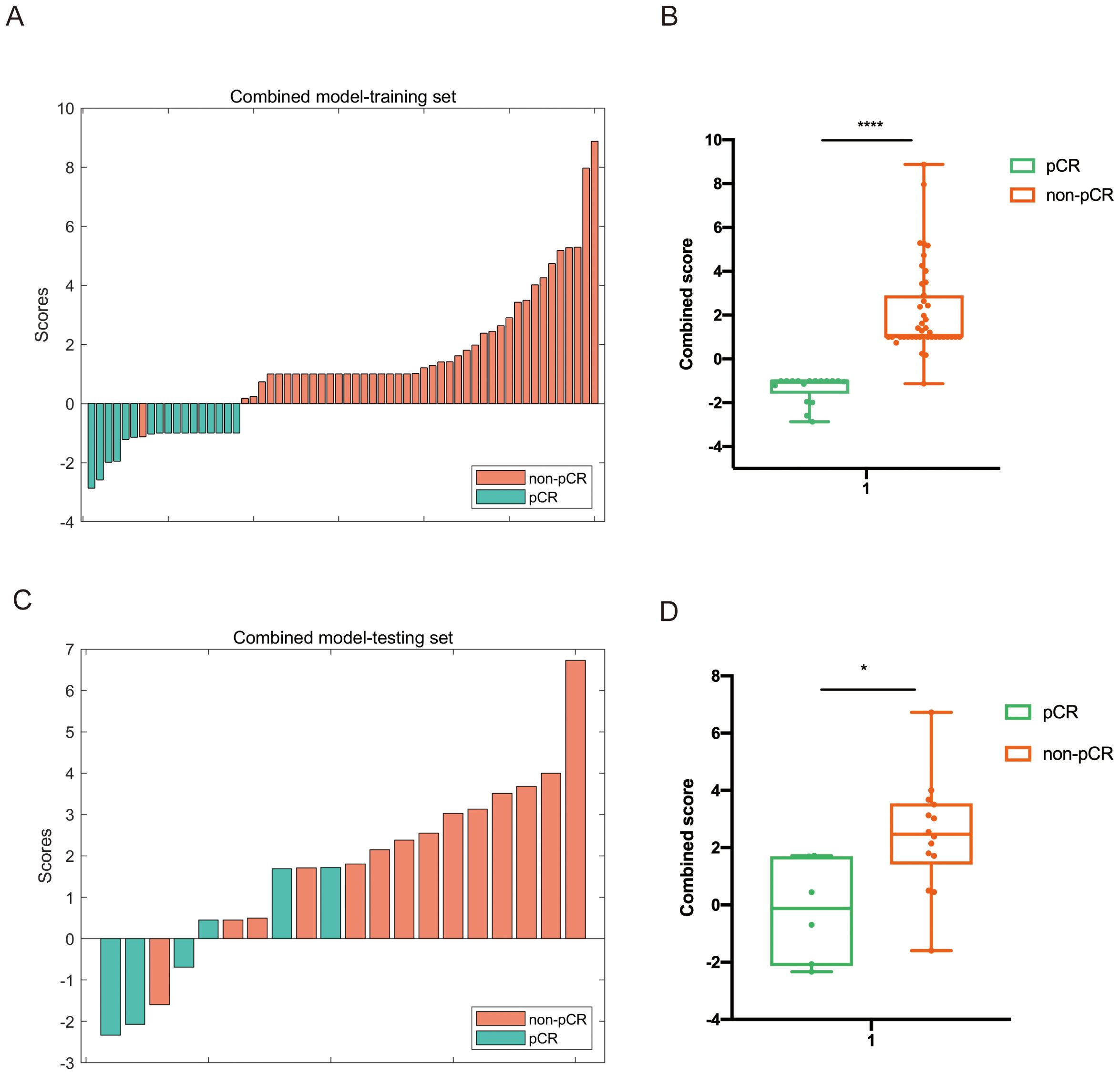
Figure 5. Distribution of the combined score between the pCR and non-pCR groups in the multi-modality model in both training (A, B) and testing set (C, D).
Table 4 and Figure 6 present a comparison of the different SVM models. The combined model accurately predicted pCR in the training (AUC=0.99 [0.96-1.00]) and testing (AUC=0.89 [0.75-1.00]) sets. The specificity of the multi-modality was markedly high in the testing set at 94.28%, while the sensitivity was moderate (66.7%). The NPV of the combined model was 86.7% in the testing set, whereas the PPV was approximately 80%. Additionally, the single radiomics model displayed a marginally lower AUC (0.70 [0.54-0.85]) than multi-modality model, whereas the clinical model had a much lower AUC of 0.63 (0.46-0.80). The pathomics model yielded a higher AUC (0.77 [0.53-1.0]) than the other single-modality models but was still slightly lower than that of the multi-modality model (Figures 6A, B).
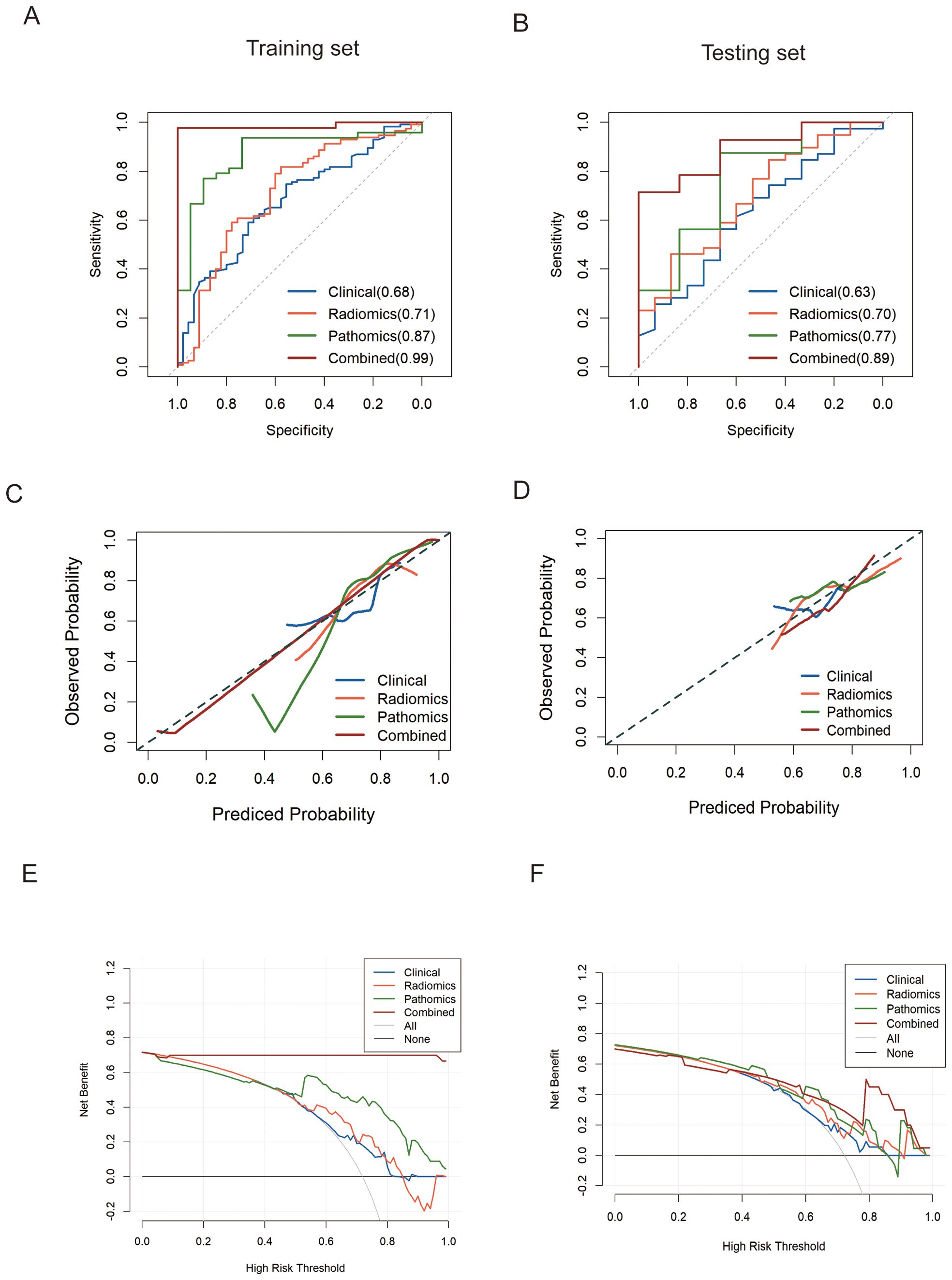
Figure 6. ROC analysis of predict models for predicting pCR in the training set (A) and validation set (B), respectively. (C, D). Calibration curves of models in training and testing set on discriminating pCR versus non-pCR. (E, F). Decision curve analysis (DCA) was performed on four models for classifying pCR versus non-pCR in the training and testing cohorts.
Clinical usefulness of the multi-modality model
We evaluated the clinical usefulness of the combined multi-modality model for pCR recognition via DCA in the training and testing cohorts, revealing that the multi-modality model conferred better net benefits than the CT radiomics and pathomics models (Figure 6E, F). The calibration curves also revealed that the prediction probability of the multi-modality model was in good agreement with the actual outcomes of neoadjuvant chemoimmunotherapy for esophageal cancer in both the training and testing groups (Figures 6C, D).
Discussion
We developed a combined predictive model using multimodal pretreatment CT radiomics and WSI pathomics features, in addition to clinical variables, to predict the response to neoadjuvant chemoimmunotherapy in esophageal cancer prior to treatment initiation. The integrative, multi-modality model showed significant improvement in assessing the response to neoadjuvant chemoimmunotherapy compared with the single-modality model in terms of the AUC (0.89 [95% CI: 0.75,1.00]). To our knowledge, this is the first study to combine pathomics and radiomics in the field of esophageal cancer.
Individualized treatment for patients with esophageal cancer undergoing neoadjuvant therapy has been a research priority over the past decade. Neoadjuvant chemoimmunotherapy has shown remarkable advances in the management of esophageal cancer, resulting in a high rate of pCR and improved long-term prognosis (8, 23, 24). However, pCR can only be confirmed postoperatively. Patients who are sensitive to neoadjuvant chemoimmunotherapy might have good prognoses and could benefit from watch-and-wait strategies (25). Conversely, for patients who do not respond to neoadjuvant chemoimmunotherapy, earlier surgical intervention can be considered to avoid unnecessary immunotherapy-associated toxicity and morbidity. Recently, several studies have explored the predictive effectiveness of genetic (26, 27), microbiome (28), and tumor microenvironment biomarkers (3, 29) in EC patients following neoadjuvant chemoimmunotherapy. However, their clinical application remains limited due to high costs and invasiveness. Therefore, the development of a preoperative and accurate approach to predict pCR prior to treatment is meaningful.
Radiomics is a rapidly developing research field, and many studies have demonstrated the role of radiomics biomarkers in predicting pCR to neoadjuvant chemoradiotherapy in esophageal cancer (11, 30, 31). A meta-analysis by Zhao et al. (32) highlighted the clinical value of pretreatment imaging-based radiomics in predicting pCR, achieving a pooled AUC of 0.84 (95%CI: 0.81–0.87) in patients who underwent neoadjuvant chemoradiotherapy. However, the role of radiomics in predicting pCR following neoadjuvant chemoimmunotherapy for esophageal cancer remains limited. In our study, the AUC value of the prediction model based on radiomics features was only 0.70 in the testing set, which was lower than that reported by Li et al. (33). The superior results in Li et al.’s study was mainly attributed to post-treatment CT, which provided direct information on tumor regression or residual after treatment. However, their model could not provide an earlier estimation of treatment response to guide the administration of neoadjuvant chemoimmunotherapy and required patients to undergo several examinations. Thus, the predictive performance of the single-modality model based solely on radiomics features remains unsatisfactory. Radiomics-derived data should be combined with other features to achieve a more powerful predictive value (34).
In contrast to radiomics, which captures the spatial macrostructure of tumors, histopathology contains valuable microstructural information about the tumor cell, extracellular matrix, and tissue morphology, which may be overlooked in radiomics analyses. With the development of artificial intelligence and digital pathology, we can extract quantitative features from diagnostic slides that cannot be recognized by the naked eye (35–38). Pathomics-based analyses of esophageal cancer specimens have proven effective in lesion detection, cancer diagnosis, and prognostic evaluation (14, 15). In terms of predicting the efficacy of neoadjuvant therapy, Bhargava (39) and Tian (40) et al. demonstrated that the pathological features from biopsy slides are predictive of pCR in breast cancer with an AUCs of 0.71-0.73 in the validation cohorts. Consistent with these studies, our pathomics model based on deep learning also demonstrated good performance in predicting pCR in esophageal cancer (AUC of 0.87 on the training set and 0.77 on the testing set). Biopsies can provide insights into specific subregions or areas with unique characteristics of the tumor tissue, such as cellular composition and morphological features, under a microscope. By fusing biopsy data with imaging, complementary information from both microscopic and macroscopic levels representative of tumor heterogeneity can be provided. The superior performance of our combined multi-modality model is likely due to the integration of heterogeneous radiomics and pathomics features, suggesting that the comprehensive capture of microstructural and macrostructural features can effectively predict pCR in esophageal cancer.
In clinical practice, the combined multi-modality model has the potential to assist clinicians in accurately predicting the efficacy of neoadjuvant chemoimmunotherapy before its administration, which is critical for developing patient treatment plans and optimizing overall patient management. For patients predicted to achieve a pCR, it may be beneficial to administer intensive neoadjuvant chemoimmunotherapy and adopt a watch-and-wait strategy to improve survival and quality of life. Conversely, for patients unlikely to achieve a pCR, neoadjuvant treatment might be unnecessary due to its associated excessive treatment-related toxicity.
Despite the innovative nature of our study, several limitations exist. First, the study’s retrospective design, along with the small sample size and absence of external validation. A total of 223 patients were included in our study; however, combined pathological and radiological analysis was only conducted in 80 patients due to the exclusion of a significant number of patients who did not undergo biopsy at our institution. Multicenter and large-sample prospective studies are warranted to further validate the preliminary results. Another significant factor that hampers the effectiveness of the proposed prediction model is the imbalance in the distribution of baseline characteristics, such as sex, between patients who achieved a pCR and those who did not within the pathomics cohort. Specifically, the relatively small number of females in our study may influence the outcomes, as previous literature has indicated that sex is associated with the extent of benefit derived from immunotherapy (41). Our future research will include more female patients to ensure the balance of baseline characteristics between patients with and without pCR. Additionally, previous studies (4) have shown that adenocarcinoma (ADC) and squamous cell carcinoma (SCC) have different response to neoadjuvant chemoimmunotherapy and may lead to different prediction performances. The model constructed by merging the two histopathological types may lead to a decline in prediction efficiency. The lack of adequate patients with ADC (less than 10%) also placed a higher weight on the SCC group, which may bias the pooled estimates toward SCC group when generating the overall results. Therefore, larger data sets should be used in future research, and the two histological subtypes should be hierarchically modeled and verified. Finally, all ROIs are manually delineated from the arterial-phase CT images, which is time-consuming and laborious. It is necessary to collect multiphase CT data and develop a user-friendly tool to encourage the use of radiomic measures in daily clinical practice.
In conclusion, the current study demonstrated that a model integrating pretreatment CT, WSI, and clinical variables significantly enhances the prediction of pCR in patients undergoing neoadjuvant chemoimmunotherapy, compared to single-modality models. The improved performance of our multi-modality model highlights its potential for advancing precision decision-making and personalizing treatment strategies for patients with esophageal cancer.
Data availability statement
The original contributions presented in the study are included in the article/supplementary material. Further inquiries can be directed to the corresponding authors.
Ethics statement
The studies involving humans were approved by Ethics Committee of Shandong Cancer Hospital. The studies were conducted in accordance with the local legislation and institutional requirements. The ethics committee/institutional review board waived the requirement of written informed consent for participation from the participants or the participants’ legal guardians/next of kin because the requirement for informed consent was waived owing to the retrospective nature of the study.
Author contributions
YQ: Data curation, Formal analysis, Writing – original draft. YH: Data curation, Writing – original draft, Methodology, Software. CL: Data curation, Methodology, Software, Writing – original draft, Project administration. GS: Project administration, Software, Validation, Writing – original draft. LS: Project administration, Software, Methodology, Writing – review & editing. HZ: Writing – review & editing, Conceptualization, Supervision.
Funding
The author(s) declare financial support was received for the research, authorship, and/or publication of this article. This work was supported by the Natural Science Foundation of Shandong Province (grant numbers: ZR2023QH388) and the Medical Health Science and Technology Project of Zhejiang Province (grant number: 2022KY652).
Conflict of interest
The authors declare that the research was conducted in the absence of any commercial or financial relationships that could be construed as a potential conflict of interest.
Generative AI statement
The author(s) declare that no Generative AI was used in the creation of this manuscript.
Publisher’s note
All claims expressed in this article are solely those of the authors and do not necessarily represent those of their affiliated organizations, or those of the publisher, the editors and the reviewers. Any product that may be evaluated in this article, or claim that may be made by its manufacturer, is not guaranteed or endorsed by the publisher.
Abbreviations
EC, Esophageal cancer; pCR, pathological complete response; WSI, Whole-slide image; SVM, Support vector machine; ROC, Receiver operating characteristic; AUC, Area under curve; PPV, Positive predictive value; NPV, Negative predictive value; DCA, Decision curve analyses; ROI, Region of interest; MRMR, Maximum relevance-minimum redundancy.
References
1. Sung H, Ferlay J, Siegel RL, Laversanne M, Soerjomataram I, Jemal A, et al. Global cancer statistics 2020: GLOBOCAN estimates of incidence and mortality worldwide for 36 cancers in 185 countries. CA Cancer J Clin. (2021) 71:209–49. doi: 10.3322/caac.21660
2. Sohda M, Yoshida T, Nakazawa N, Ubukata Y, Kuriyama K, Hara K, et al. Comparative study on recurrence pattern and treatment method after radical esophagectomy for esophageal cancer. J Med Invest. (2021) 68:129–35. doi: 10.2152/jmi.68.129
3. Yang W, Xing X, Yeung SJ, Wang S, Chen W, Bao Y, et al. Neoadjuvant programmed cell death 1 blockade combined with chemotherapy for resectable esophageal squamous cell carcinoma. J Immunother Cancer. (2022) 10:e003497. doi: 10.1136/jitc-2021-003497
4. Ge F, Huo Z, Cai X, Hu Q, Chen W, Lin G, et al. Evaluation of clinical and safety outcomes of neoadjuvant immunotherapy combined with chemotherapy for patients with resectable esophageal cancer: A systematic review and meta-analysis. JAMA Netw Open. (2022) 5:e2239778. doi: 10.1001/jamanetworkopen.2022.39778
5. Li Q, Liu T, Ding Z. Neoadjuvant immunotherapy for resectable esophageal cancer: A review. Front Immunol. (2022) 13:1051841. doi: 10.3389/fimmu.2022.1051841
6. Donahue JM, Nichols FC, Li Z, Schomas DA, Allen MS, Cassivi SD, et al. Complete pathologic response after neoadjuvant chemoradiotherapy for esophageal cancer is associated with enhanced survival. Ann Thorac Surg. (2009) 87:392–8; discussion 398-9. doi: 10.1016/j.athoracsur.2008.11.001
7. Lin JW, Hsu CP, Yeh HL, Chuang CY, Lin CH. The impact of pathological complete response after neoadjuvant chemoradiotherapy in locally advanced squamous cell carcinoma of esophagus. J Chin Med Assoc. (2018) 81:18–24. doi: 10.1016/j.jcma.2017.08.007
8. Wang H, Jiang Z, Wang Q, Wu T, Guo F, Xu Z, et al. Pathological response and prognostic factors of neoadjuvant PD-1 blockade combined with chemotherapy in resectable oesophageal squamous cell carcinoma. Eur J Cancer. (2023) 186:196–210. doi: 10.1016/j.ejca.2023.03.008
9. Bera K, Braman N, Gupta A, Velcheti V, Madabhushi A. Predicting cancer outcomes with radiomics and artificial intelligence in radiology. Nat Rev Clin Oncol. (2022) 19:132–46. doi: 10.1038/s41571-021-00560-7
10. Yang M, Li X, Cai C, Liu C, Ma M, Qu W, et al. (18)F]FDG PET-CT radiomics signature to predict pathological complete response to neoadjuvant chemoimmunotherapy in non-small cell lung cancer: a multicenter study. Eur Radiol. (2024) 34:4352–63. doi: 10.1007/s00330-023-10503-8
11. Wang J, Zhu X, Zeng J, Liu C, Shen W, Sun X, et al. Using clinical and radiomic feature-based machine learning models to predict pathological complete response in patients with esophageal squamous cell carcinoma receiving neoadjuvant chemoradiation. Eur Radiol. (2023) 33:8554–63. doi: 10.1007/s00330-023-09884-7
12. Liu Y, Wang Y, Wang X, Xue L, Zhang H, Ma Z, et al. MR radiomics predicts pathological complete response of esophageal squamous cell carcinoma after neoadjuvant chemoradiotherapy: a multicenter study. Cancer Imaging. (2024) 24:16. doi: 10.1186/s40644-024-00659-x
13. Zhu Y, Yao W, Xu BC, Lei YY, Guo QK, Liu LZ, et al. Predicting response to immunotherapy plus chemotherapy in patients with esophageal squamous cell carcinoma using non-invasive Radiomic biomarkers. BMC Cancer. (2021) 21:1167. doi: 10.1186/s12885-021-08899-x
14. Li B, Qin W, Yang L, Li H, Jiang C, Yao Y, et al. From pixels to patient care: deep learning-enabled pathomics signature offers precise outcome predictions for immunotherapy in esophageal squamous cell cancer. J Transl Med. (2024) 22:195. doi: 10.1186/s12967-024-04997-z
15. Botros M, de Boer OJ, Cardenas B, Bekkers EJ, Jansen M, van der Wel MJ , et al. Deep learning for histopathological assessment of esophageal adenocarcinoma precursor lesions. Mod Pathol. (2024) 37:100531. doi: 10.1016/j.modpat.2024.100531
16. Chen Y, Gao R, Jing D, Shi L, Kuang F, Jing R. Classification and prediction of chemoradiotherapy response and survival from esophageal carcinoma histopathology images. Spectrochim Acta A Mol Biomol Spectrosc. (2024) 312:124030. doi: 10.1016/j.saa.2024.124030
17. Cottrell TR, Thompson ED, Forde PM, Stein JE, Duffield AS, Anagnostou V, et al. Pathologic features of response to neoadjuvant anti-PD-1 in resected non-small-cell lung carcinoma: a proposal for quantitative immune-related pathologic response criteria (irPRC). Ann Oncol. (2018) 29:1853–60. doi: 10.1093/annonc/mdy218
18. Stein JE, Lipson EJ, Cottrell TR, Forde PM, Anders RA, Cimino-Mathews A, et al. Pan-tumor pathologic scoring of response to PD-(L)1 blockade. Clin Cancer Res. (2020) 26:545–51. doi: 10.1158/1078-0432.CCR-19-2379
19. van Griethuysen JJM, Fedorov A, Parmar C, Hosny A, Aucoin N, Narayan V, et al. Computational radiomics system to decode the radiographic phenotype. Cancer Res. (2017) 77:e104–7. doi: 10.1158/0008-5472.CAN-17-0339
20. Vahadane A, Peng T, Sethi A, Albarqouni S, Wang L, Baust M, et al. Structure-preserving color normalization and sparse stain separation for histological images. IEEE Trans Med Imaging. (2016) 35:1962–71. doi: 10.1109/TMI.2016.2529665
21. Jiang W, Song Y, Sun Z, Qiu J, Shi L. Dosimetric factors and radiomics features within different regions of interest in planning CT images for improving the prediction of radiation pneumonitis. Int J Radiat Oncol Biol Phys. (2021) 110:1161–70. doi: 10.1016/j.ijrobp.2021.01.049
22. Meng X, Xia W, Xie P, Zhang R, Li W, Wang M, et al. Preoperative radiomic signature based on multiparametric magnetic resonance imaging for noninvasive evaluation of biological characteristics in rectal cancer. Eur Radiol. (2019) 29:3200–9. doi: 10.1007/s00330-018-5763-x
23. Lv H, Huang C, Li J, Zhang F, Gai C, Liu Z, et al. The survival outcomes of neoadjuvant sintilimab combined with chemotherapy in patients with locally advanced esophageal squamous cell carcinoma. Front Immunol. (2022) 13:1100750. doi: 10.3389/fimmu.2022.1100750
24. Su F, Yang X, Yin J, Shen Y, Tan L. Validity of using pathological response as a surrogate for overall survival in neoadjuvant studies for esophageal cancer: A systematic review and meta-analysis. Ann Surg Oncol. (2023) 30:7461–71. doi: 10.1245/s10434-023-13778-9
25. Chen N, Ajani JA, Wu A. Nonoperative management of gastrointestinal Malignancies in era of neoadjuvant treatment. Chin J Cancer Res. (2023) 35:44–57. doi: 10.21147/j.issn.1000-9604.2023.01.05
26. Zhang H, Wen H, Zhu Q, Zhang Y, Xu F, Ma T, et al. Genomic profiling and associated B cell lineages delineate the efficacy of neoadjuvant anti-PD-1-based therapy in oesophageal squamous cell carcinoma. EBioMedicine. (2024) 100:104971. doi: 10.1016/j.ebiom.2024.104971
27. Ji G, Yang Q, Wang S, Yan X, Ou Q, Gong L, et al. Single-cell profiling of response to neoadjuvant chemo-immunotherapy in surgically resectable esophageal squamous cell carcinoma. Genome Med. (2024) 16:49. doi: 10.1186/s13073-024-01320-9
28. Wu H, Leng X, Liu Q, Mao T, Jiang T, Liu Y, et al. Intratumoral microbiota composition regulates chemoimmunotherapy response in esophageal squamous cell carcinoma. Cancer Res. (2023) 83:3131–44. doi: 10.1158/0008-5472.CAN-22-2593
29. Wang X, Ling X, Wang C, Zhang J, Yang Y, Jiang H, et al. Response to neoadjuvant immune checkpoint inhibitors and chemotherapy in Chinese patients with esophageal squamous cell carcinoma: the role of tumor immune microenvironment. Cancer Immunol Immunother. (2023) 72:1619–31. doi: 10.1007/s00262-022-03354-7
30. Zhang M, Lu Y, Sun H, Hou C, Zhou Z, Liu X, et al. CT-based deep learning radiomics and hematological biomarkers in the assessment of pathological complete response to neoadjuvant chemoradiotherapy in patients with esophageal squamous cell carcinoma: A two-center study. Transl Oncol. (2024) 39:101804. doi: 10.1016/j.tranon.2023.101804
31. Wang X, Gong G, Sun Q, Meng X. Prediction of pCR based on clinical-radiomic model in patients with locally advanced ESCC treated with neoadjuvant immunotherapy plus chemoradiotherapy. Front Oncol. (2024) 14:1350914. doi: 10.3389/fonc.2024.1350914
32. Yang Z, Gong J, Li J, Sun H, Pan Y, Zhao L. The gap before real clinical application of imaging-based machine-learning and radiomic models for chemoradiation outcome prediction in esophageal cancer: a systematic review and meta-analysis. Int J Surg. (2023) 109:2451–66. doi: 10.1097/JS9.0000000000000441
33. Li K, Li Y, Wang Z, Huang C, Sun S, Liu X, et al. Delta-radiomics based on CT predicts pathologic complete response in ESCC treated with neoadjuvant immunochemotherapy and surgery. Front Oncol. (2023) 13:1131883. doi: 10.3389/fonc.2023.1131883
34. Lambin P, Leijenaar RTH, Deist TM, Peerlings J, de Jong EEC, van Timmeren J, et al. Radiomics: the bridge between medical imaging and personalized medicine. Nat Rev Clin Oncol. (2017) 14:749–62. doi: 10.1038/nrclinonc.2017.141
35. Niazi MKK, Parwani AV, Gurcan MN. Digital pathology and artificial intelligence. Lancet Oncol. (2019) 20:E253–61. doi: 10.1016/S1470-2045(19)30154-8
36. Bera K, Schalper KA, Rimm DL, Velcheti V, Madabhushi A. Artificial intelligence in digital pathology - new tools for diagnosis and precision oncology. Nat Rev Clin Oncol. (2019) 16:703–15. doi: 10.1038/s41571-019-0252-y
37. Kather JN, Pearson AT, Halama N, Jäger D, Krause J, Loosen SH, et al. Deep learning can predict microsatellite instability directly from histology in gastrointestinal cancer. Nat Med. (2019) 25:1054. doi: 10.1038/s41591-019-0462-y
38. Coudray N, Ocampo PS, Sakellaropoulos T, Narula N, Snuderl M, Fenyö D, et al. Classification and mutation prediction from non-small cell lung cancer histopathology images using deep learning. Nat Med. (2018) 24:1559. doi: 10.1038/s41591-018-0177-5
39. Zeng H, Qiu S, Zhuang S, Wei X, Wu J, Zhang R, et al. Deep learning-based predictive model for pathological complete response to neoadjuvant chemotherapy in breast cancer from biopsy pathological images: a multicenter study. Front Physiol. (2024) 15:1279982. doi: 10.3389/fphys.2024.1279982
40. Li B, Li F, Liu Z, Xu F, Ye G, Li W, et al. Deep learning with biopsy whole slide images for pretreatment prediction of pathological complete response to neoadjuvant chemotherapy in breast cancer:A multicenter study. Breast. (2022) 66:183–90. doi: 10.1016/j.breast.2022.10.004
Keywords: pathological complete response, radiomics, pathomics, support vector machine, esophageal cancer (EC)
Citation: Qi Y, Hu Y, Lin C, Song G, Shi L and Zhu H (2025) A preoperative predictive model based on multi-modal features to predict pathological complete response after neoadjuvant chemoimmunotherapy in esophageal cancer patients. Front. Immunol. 16:1530279. doi: 10.3389/fimmu.2025.1530279
Received: 18 November 2024; Accepted: 07 January 2025;
Published: 27 January 2025.
Edited by:
Qiang Wen, Stanford University, United StatesReviewed by:
Wencheng Zhang, Tianjin Medical University Cancer Institute and Hospital, ChinaWen-Yang Liu, Chinese Academy of Medical Sciences and Peking Union Medical College, China
Copyright © 2025 Qi, Hu, Lin, Song, Shi and Zhu. This is an open-access article distributed under the terms of the Creative Commons Attribution License (CC BY). The use, distribution or reproduction in other forums is permitted, provided the original author(s) and the copyright owner(s) are credited and that the original publication in this journal is cited, in accordance with accepted academic practice. No use, distribution or reproduction is permitted which does not comply with these terms.
*Correspondence: Liting Shi, c2hpbHRAempjYy5vcmcuY24=; Hui Zhu, ZHJ6aHVoQDEyNi5jb20=