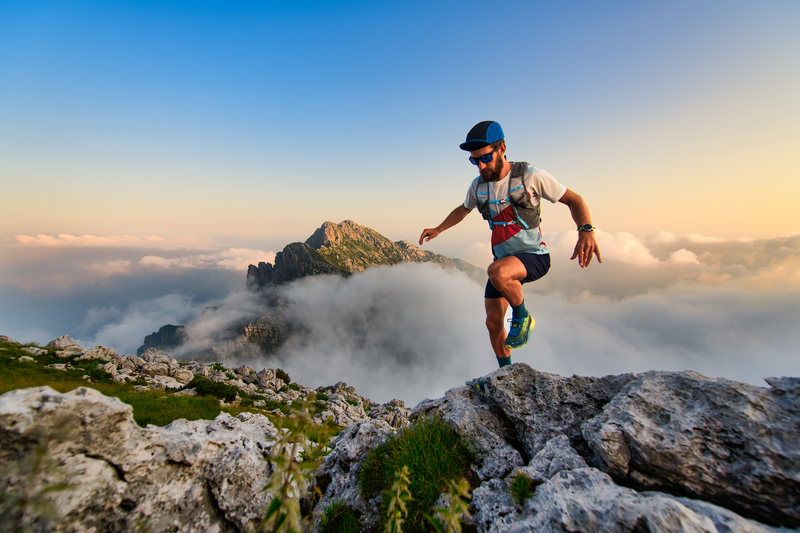
95% of researchers rate our articles as excellent or good
Learn more about the work of our research integrity team to safeguard the quality of each article we publish.
Find out more
ORIGINAL RESEARCH article
Front. Immunol. , 10 January 2025
Sec. Inflammation
Volume 15 - 2024 | https://doi.org/10.3389/fimmu.2024.1539465
This article is part of the Research Topic Harnessing Big Data for Precision Medicine: Revolutionizing Diagnosis and Treatment Strategies View all 36 articles
Background: Multidrug-resistant Klebsiella pneumoniae (MDR-KP) infections pose a significant global healthcare challenge, particularly due to the high mortality risk associated with septic shock. This study aimed to develop and validate a machine learning-based model to predict the risk of MDR-KP-associated septic shock, enabling early risk stratification and targeted interventions.
Methods: A retrospective analysis was conducted on 1,385 patients with MDR-KP infections admitted between January 2019 and June 2024. The cohort was randomly divided into a training set (n = 969) and a validation set (n = 416). Feature selection was performed using LASSO regression and the Boruta algorithm. Seven machine learning algorithms were evaluated, with logistic regression chosen for its optimal balance between performance and robustness against overfitting.
Results: The overall incidence of MDR-KP-associated septic shock was 16.32% (226/1,385). The predictive model identified seven key risk factors: procalcitonin (PCT), sepsis, acute kidney injury, intra-abdominal infection, use of vasoactive medications, ventilator weaning failure, and mechanical ventilation. The logistic regression model demonstrated excellent predictive performance, with an area under the receiver operating characteristic curve (AUC) of 0.906 in the training set and 0.865 in the validation set. Calibration was robust, with Hosmer-Lemeshow test results of P = 0.065 (training) and P = 0.069 (validation). Decision curve analysis indicated substantial clinical net benefit.
Conclusion: This study presents a validated, high-performing predictive model for MDR-KP-associated septic shock, offering a valuable tool for early clinical decision-making. Prospective, multi-center studies are recommended to further evaluate its clinical applicability and effectiveness in diverse settings.
Multidrug-resistant Klebsiella pneumoniae (MDR-KP) infections have become a critical global health threat, escalating in both incidence and mortality, and imposing substantial burdens on healthcare systems worldwide (1). In particular, healthcare infrastructures in resource-limited settings face increasing strain due to the complexity and severity of MDR-KP infections (2). Prolonged hospitalizations, the need for advanced diagnostics, and the administration of high-cost, broad-spectrum antibiotics can significantly increase direct healthcare expenditures. These pressures not only intensify the economic burden on healthcare institutions but also limit the optimal allocation of scarce resources, ultimately hindering patient care and system resilience (3). The surge in MDR-KP cases is primarily attributed to widespread antimicrobial use, which presents significant therapeutic dilemmas in contemporary clinical practice (4). Notably, MDR-KP infections are associated with mortality rates ranging from 40% to 50%, with those involving bloodstream infections experiencing even higher mortality rates up to 70% (5). Septic shock represents one of the most severe complications of MDR-KP infection, often leading to multiple organ dysfunction and increased mortality (6).
The high morbidity and mortality of MDR-KP infections directly correlates with their complex antimicrobial resistance mechanisms. MDR-KP demonstrates adaptability primarily through the production of β-lactamase enzymes, including ESBLs and carbapenemases, leading to broad-spectrum resistance (7). Additional resistance mechanisms include altered outer membrane protein expression and enhanced efflux pump activity (8). These combined resistance mechanisms often render conventional antibiotics ineffective, compromising infection control and increasing the risk of septic shock.
Current MDR-KP research focuses on three main areas: epidemiology, resistance mechanisms, and treatment optimization. Key risk factors for MDR-KP infections include prolonged hospitalization, invasive procedures, and immunocompromised status (9). Treatment research has explored various strategies, including antimicrobial combinations, new antibiotic development, and alternative approaches such as bacteriophage therapy (10). However, there remains a significant gap in developing predictive models for MDR-KP-associated septic shock risk. Early identification and intervention in high-risk cases significantly improve patient outcomes (11). This highlights the need for accurate predictive models to guide clinical decisions and improve prognosis. Although general sepsis prediction tools like qSOFA provide a quick bedside assessment for sepsis and related complications (12), these generic tools may not adequately capture the unique pathophysiology of MDR-KP infections, limiting their accuracy in predicting MDR-KP-specific septic shock risk.
Machine learning has increasingly been applied in medicine for disease risk prediction and prognosis (13–16). These methods excel at analyzing complex clinical data, identifying hidden risk factors and patterns while improving predictive accuracy (17). In infectious diseases, machine learning has shown success in sepsis prediction (18) and antimicrobial resistance forecasting (19). In contrast to traditional statistical approaches, machine learning methods are particularly suitable for this study due to their ability to handle high-dimensional, complex clinical data and uncover intricate nonlinear relationships among variables without stringent parametric assumptions. While traditional statistical models (e.g., logistic regression, Cox proportional hazards models) typically depend on predefined assumptions about data distributions and variable interactions, machine learning approaches autonomously identify and weigh critical predictors, effectively manage collinearity and nonlinearities, and adapt to evolving data patterns. This flexibility and robustness can substantially enhance predictive accuracy and stability, enabling a more comprehensive understanding of multifactorial conditions such as MDR-KP-associated septic shock. However, current MDR-KP prediction models primarily rely on conventional statistics, underutilizing the potential of Big Data analytics and Artificial Intelligence (AI). Current predictive modeling research has several important methodological limitations. First, studies often use limited sample populations, which may affect model stability and generalizability. Second, many models incorporate an insufficient range of predictive variables, potentially missing key risk factors. Additionally, model validation often lacks comprehensive calibration metrics and robust clinical utility assessment. These limitations may reduce the models’ practical utility in real clinical settings.
In this study, we aim to develop a machine learning-based predictive model for MDR-KP-associated septic shock risk, integrating clinical and laboratory parameters. The model incorporates two key improvements: large-scale sampling for model stability and comprehensive risk factors, including demographics, comorbidities, laboratory data, and treatment variables. Model validation employs multiple metrics, including discrimination, calibration, and clinical decision curve analysis, to assess both predictive accuracy and clinical utility.
This investigation received ethical authorization from the Ethics Committee of the Affiliated Huai’an No. 1 People’s Hospital of Nanjing Medical University (approval number: KY-2024-355-01). Informed consent was waived due to the retrospective design. Data anonymization and confidentiality were maintained according to the Declaration of Helsinki.
We conducted a retrospective analysis of clinical data from 1,475 patients infected with MDR-KP who were admitted to The Affiliated Huai’an No.1 People’s Hospital of Nanjing Medical University from January 2019 to June 2024. A total of 1,385 patients met the stringent inclusion criteria and were subsequently analyzed statistically. The criteria for patient inclusion required individuals to be adults aged 18 or older, to have a confirmed MDR-KP infection, and to possess comprehensive clinical documentation, including demographic data (such as gender and age) and laboratory parameters. Exclusion criteria were defined to omit patients with psychiatric or cognitive disorders, those hospitalized for less than 24 hours, patients with concurrent malignancies, cases where MDR-KP was isolated outside the designated timeframe (prior to admission, within 48 hours following admission, or more than 72 hours after discharge), or individuals suffering from non-MDR-KP-induced septic shock.
We developed a machine learning-based predictive model to determine the risk of Septic Shock in patients with Multidrug-resistant Klebsiella pneumoniae (MDR-KP). This retrospective study analyzed data from 1,385 confirmed cases of MDR-KP infections, defining MDR-KP as strains of K. pneumoniae resistant to at least one agent in three or more antimicrobial categories (20). Septic shock was characterized following the Sepsis-3 criteria (21). Clinical data, extracted from electronic health records, encompassed a wide range of variables, including demographics, laboratory indices, treatments, comorbidities, infection sites, metrics of organ dysfunction, and complications. The dataset was randomly split into training (70%) and test (30%) sets.
Feature selection combined Boruta algorithm and LASSO regression (22–24). Multiple machine learning models were evaluated: Logistic Regression (LR), Decision Trees (DT), Random Forests (RF), eXtreme Gradient Boosting (XGBoost), Support Vector Machines (SVM), K-Nearest Neighbors (KNN), and Light Gradient Boosting Machine (LightGBM), using 10-fold cross-validation (25–27). Model performance was assessed through area under the curve (AUC), accuracy, sensitivity, specificity, predictive values, F1 score, calibration curves, and clinical impact analysis. A nomogram was developed to visualize feature importance and prediction mechanisms. The workflow is illustrated in Figure 1.
A total of 99 clinical predictors across seven major categories were analyzed. These included demographic factors (gender, age); laboratory parameters (SO2, pH, HCO3-, BE, PO2, PCO2, AG, WBC count, HGB, RBC count, HCT, RDW-SD, RDW-CV, MCV, MCHC, MCH, PLT count, PDW, MPV, neutrophils [percentage and count], monocytes [percentage and count], lymphocytes [percentage and count], hs-CRP, PCT, Urea, creatinine, UA, CYSC, Serum CO2, TBIL, DBIL, AST, ALT, ALP, γ-GGT, TP, ALB, AFU, ADA, CHE, PA, HBDH, TG, CHOL, CK, LDH, Na, Ca, P, K, Mg, TT, PT, Fibrinogen, D-dimer, NT-proBNP); treatment interventions (surgery, endotracheal intubation, mechanical ventilation, ventilator weaning failure, vasoactive medications, CRRT use, ECMO use, anticoagulant use, tracheostomy); comorbidities (hypertension, diabetes mellitus, diabetes-related complications, hyperlipidemia, hyperlactatemia, coronary heart disease, atrial fibrillation, chronic renal insufficiency, heart failure, anemia); infection sites (liver abscess, biliary tract infection, intra-abdominal infection, pneumonia, urinary tract infection, intracranial infection); acute organ injury (acute liver injury, acute kidney injury, ARDS, MODS, altered mental status, stroke, metabolic encephalopathy, hepatic encephalopathy, ventricular fibrillation, cardiac arrest); and complications (coagulopathy, DIC, sepsis). Demographic and comorbidity data were collected before MDR-KP infection onset. Laboratory parameters were based on initial post-admission results.
Septic shock diagnosis followed the Third International Consensus Definitions for Sepsis and Septic Shock (Sepsis-3) criteria (21). Diagnostic requirements included: 1) suspected or documented infection; 2) persistent hypotension requiring vasopressors to maintain mean arterial pressure ≥65 mmHg; and 3) serum lactate level >2 mmol/L despite adequate volume resuscitation. Alternative causes of hypotension were excluded. While noted, additional features such as altered mental status, tachypnea, decreased urine output, and poor peripheral circulation were not mandatory for diagnosis.
To refine our predictive model, we utilized the Least Absolute Shrinkage and Selection Operator (LASSO) regression (28), leveraging the ‘glmnet’ package in R with settings adjusted for binomial distribution (α = 1) (29, 30). This method determined optimal lambda values via cross-validation—lambda.min for the minimal error and lambda.1se to ensure parsimony—thereby retaining variables with non-zero coefficients which helped mitigate multicollinearity and overfitting in our high-dimensional dataset (31, 32). Additionally, we implemented the Boruta algorithm, a Random Forest-based feature selection method (33, 34). This algorithm assesses feature importance by creating ‘shadow attributes’ for each original feature, iteratively comparing their importance through 500 iterations or until stability is achieved (35, 36). Important features were identified as those consistently outperforming their shadow counterparts, with results processed using the ‘attStats’ and custom ‘adjustdata’ functions to ensure robust selection and minimize false negatives (37, 38).
Several machine learning algorithms were used to predict MDR-KP-associated septic shock risk. Logistic Regression (LR) was applied with L2 regularization to model the binary outcomes, optimizing hyperparameters like regularization factor and iteration limits for balance and precision (39–41). The Decision Tree model utilized the CART algorithm, setting parameters to maintain a balance between complexity and interpretability (37, 42, 43). The Random Forest algorithm was configured with parameters to capture non-linear associations and enhance generalizability (44, 45). For robust pattern recognition, the Extreme Gradient Boosting (XGBoost) model was optimized with specific parameters for tree depth, learning rate, and regularization (46, 47). The Support Vector Machine (SVM) algorithm employed a Radial Basis Function (RBF) kernel, focusing on optimizing the decision boundary in high-dimensional space (24, 48, 49). K-Nearest Neighbors (KNN) used a simple majority voting mechanism with uniform weighting, and the Light Gradient Boosting Machine (LightGBM) was optimized for computational efficiency and effective pattern recognition, making extensive use of gradient boosting technology (50–52). Each model’s configuration aimed to effectively identify risk factors and predict outcomes accurately within our dataset.
In this study, we employed decision curve analysis (DCA) to evaluate the clinical utility of our predictive model (22, 53). The fundamental principle of DCA is to compare the net benefit derived from “intervening based on the model’s predictions” with that of two extreme strategies “treating all patients” or “treating none” across a range of clinically relevant threshold probabilities. By doing so, DCA provides insights into the model’s incremental value in real-world decision-making scenarios. More specifically, DCA integrates the predicted probability of a patient developing MDR-KP-associated septic shock with the clinician’s predetermined threshold probability for initiating intervention (e.g., 10% or 20%). For instance, if a physician chooses to escalate care, such as intensifying monitoring, adjusting antimicrobial regimens, or providing early hemodynamic support only when a patient’s risk surpasses a certain threshold, DCA graphically illustrates whether using the model at that threshold yields additional clinical benefit compared to not using it.
Statistical analyses were performed using SPSS 25.0 (IBM Corp., NY, USA) and R 4.3.2 (R Foundation, Austria). Normally distributed continuous variables were expressed as mean ± standard deviation and compared using independent t-tests, while non-normal variables were presented as median (interquartile range) with Mann-Whitney U tests. Categorical variables were analyzed using chi-square or Fisher’s exact tests as appropriate. Statistical significance was set at P < 0.05 (54, 55).
Among the 1,475 patients with MDR-KP infections admitted to the First Affiliated Hospital of Nanjing Medical University Huai’an from January 2019 and June 2024, 1,385 patients were eventually eligible and included in the statistical analysis (Figure 1). A total of 1,385 patients with MDR-KP infections were divided into a training set consisting of 969 patients and a validation set consisting of 416 patients, following a ratio of 7:3. Statistical analysis revealed no significant differences between the two groups.
The total incidence of MDR-KP-related septic shock was 16.32% (226/1,385), with comparable rates in the training [16.82% (163/969)] and validation [15.14% (63/416)] sets. Significant differences (P<0.05) between groups in the training set were observed across multiple parameters (Table 1). Laboratory indices showed differences in HCO3-, BE, WBC, RDW (SD and CV), blood cell counts (PLT, neutrophils, monocytes, lymphocytes), monocytes percentage, PCT, renal markers (urea, creatinine, UA, CYSC), liver function tests (TBIL, DBIL, AST, TP, ALB, AFU, CHE), metabolic parameters (PA, α-HBDH, CHOL, LDH), heart failure indicators (NT-proBNP), electrolytes (Ca2+, K+), and coagulation markers (PT, D-dimer). Clinical parameters including age, along with interventions such as surgery, endotracheal intubation, mechanical ventilation, ventilator weaning status, vasoactive medications, CRRT, and anticoagulation also differed significantly. Additionally, notable differences were found in comorbidities and complications, encompassing diabetes-related complications, hyperlipidemia, hyperlactatemia, intra-abdominal infection, pneumonia, acute liver/kidney injury, ARDS, MODS, stroke, cardiac arrest, coagulopathy, DIC, and sepsis.
Feature selection employed both Boruta algorithm and LASSO regression analysis. The Boruta algorithm identified 34 significant predictors, including laboratory parameters (cellular components, biochemical markers, and coagulation indices) and clinical factors (organ dysfunction indicators and interventions) (Figure 2A). LASSO regression yielded eight key predictive variables: mechanical ventilation, ventilator weaning failure, vasoactive medications, TG, intra-abdominal infection, acute kidney injury, PCT, and sepsis (Figure 2B). The intersection of these methodologies produced seven consistent predictors: mechanical ventilation, ventilator weaning failure, vasoactive medications, intra-abdominal infection, acute kidney injury, PCT, and sepsis. These variables were incorporated into the final predictive model (Figure 2C).
Figure 2. Predictor screening results. (A) Boruta; (B) Factor screening based on the LASSO regression model, with the left dashed line indicating the best lambda value for the evaluation metrics (lambda. min) and the right dashed line indicating the lambda value for the model where the evaluation metrics are in the range of the best value by one standard error (lambda.1se); (C) Boruta combined LASSO. LASSO, Least Absolute Shrinkage and Selection Operator.
Seven machine learning algorithms [Logistic Regression (LR), Decision Tree (DT), Random Forest (RF), XGBoost, SVM, KNN, and LightGBM] were implemented to predict MDR-KP-related septic shock using the seven key variables identified through combined LASSO and Boruta feature selection. Model optimization employed repeated 5-fold cross-validation with AUC-based parameter tuning. Performance evaluation incorporated multiple metrics: AUC, accuracy, sensitivity, specificity, PPV, NPV, and F1 score (Figure 3, Table 2). In the training cohort, KNN demonstrated superior performance (AUC: 0.997, accuracy: 0.986, sensitivity: 1.000, specificity: 0.983, PPV: 0.921, NPV: 1.000, F1: 0.959). However, in the test cohort, the Logistic Regression model was selected as optimal to minimize overfitting risk (AUC: 0.865, accuracy: 0.724, sensitivity: 0.810, specificity: 0.708, PPV: 0.331, NPV: 0.954, F1: 0.470).
Figure 3. The performance and comparison of seven different predictive models. (A) The training set ROC curve; (B) The test set ROC curve; (C) Forest plot of AUC values in the training set; (D) Forest plot of AUC values in the test set.
Multivariate and univariate logistic analyses identified seven significant predictors of MDR-KP-related septic shock: mechanical ventilation, ventilator weaning failure, intra-abdominal infection, acute kidney injury, vasoactive medications, PCT, and sepsis. The multivariate logistic regression coefficients and odds ratios are presented in Table 3. The predictive model was expressed as: Logit (P) = -3.634 + 0.029 (PCT) + 2.422 (Sepsis) + 0.965 (Acute kidney injury) + 1.512 (Intra-abdominal infection) + 1.297 (Vasoactive medications) + 1.418 (Ventilator weaning failure) + 0.738 (Mechanical ventilation). These variables were subsequently incorporated into a nomogram for visual prediction of MDR-KP-related septic shock probability (Figure 4).
Table 3. Univariate and Multivariate analysis of risk factors for MDR-KP-induced septic shock inpatients.
Figure 4. Nomogram used for predicting MDR-KP-induced septic shock. Logistic regression algorithm was used to establish nomogram. The final score is calculated as the sum of the individual scores of each of the ten variables included in the nomogram.
Comprehensive validation of the model’s predictive performance revealed robust capabilities across multiple assessment metrics. The model demonstrated exceptional discrimination in the training set with an AUC of 0.906 (Figure 3A), supported by strong calibration (Hosmer-Lemeshow test P = 0.065; Figure 5A). This performance was consistently maintained in the test set, achieving an AUC of 0.865 (Figure 3B) with adequate calibration (Hosmer-Lemeshow test P=0.069; Figure 5B). The model’s practical utility was confirmed through decision curve analysis, demonstrating superior net benefits across threshold probabilities of 0.01-0.92 in the training set (Figure 5C) and 0.01-0.75 in the test set (Figure 5D). Detailed confusion matrix analysis further validated the model’s performance, with the training set achieving 86.5% sensitivity and 77.9% specificity (628 true negatives, 141 true positives, 178 false positives, 22 false negatives; Figure 5E). Similar robust performance was observed in the test set, with 81.0% sensitivity and 70.8% specificity (250 true negatives, 51 true positives, 103 false positives, 12 false negatives; Figure 5F). Clinical impact curves (CIC) (Figures 5G, H) provided additional validation of the model’s clinical utility across varying cost-benefit ratios. Particularly noteworthy was the strong alignment between predicted high-risk cases and actual MDR-KP-related septic shock occurrences at threshold probabilities exceeding 40%, substantiating the model’s practical clinical value.
Figure 5. Comprehensive evaluation of the logistic regression model. (A) Calibration curve for the training set; (B) Calibration curve for the test set; (C) Decision curve analysis for the training set; (D) Decision curve analysis for the test set; (E) Confounding matrix for the training set; (F) Confounding matrix for the test set; (G) Clinical impact curve for the training set; (H) Clinical impact curve for the test set.
In the training set, the nomogram demonstrated superior discriminative ability (AUC = 0.906), and DeLong tests showed significant differences when compared with each individual predictor (all P < 0.001): intra-abdominal infection (AUC = 0.555), sepsis (AUC = 0.757), PCT (AUC = 0.690), vasoactive medications (AUC = 0.679), mechanical ventilation (AUC = 0.694), ventilator weaning failure (AUC = 0.594), and acute kidney injury (AUC = 0.686) (Figure 6A). Similarly, in the validation set, the nomogram achieved better predictive performance (AUC = 0.865), and DeLong tests confirmed significant differences compared to all individual predictors (all P < 0.001): intra-abdominal infection (AUC = 0.761), sepsis (AUC = 0.722), PCT (AUC = 0.676), vasoactive medications (AUC = 0.671), mechanical ventilation (AUC = 0.643), ventilator weaning failure (AUC = 0.625), and acute kidney injury (AUC = 0.611) (Figure 6B). Decision curve analyses confirmed the nomogram's enhanced clinical utility in both training (Figure 6C) and validation sets (Figure 6D), demonstrating consistently superior net benefit across varying threshold probabilities compared to single-factor models..
Figure 6. Comparison of the models in the entire study cohort. (A) Receiver operating characteristic curves of various models for the training set; (B) Receiver operating characteristic curves of various models the test set; (C) Decision curve analysis of various models for the training set; (D) Decision curve analysis of various models for the test set.
This study successfully developed a robust machine learning model to predict the risk of septic shock in patients with multidrug-resistant Klebsiella pneumoniae (MDR-KP) infections. Employing a combined approach of LASSO regression and Boruta algorithm, we identified seven key predictors: PCT levels, sepsis, acute kidney injury, intra-abdominal infection, use of vasoactive medications, ventilator weaning failure, and mechanical ventilation. Logistic regression was the optimal method for model development, showing excellent performance in both training and validation phases.
Our predictive model aligns with and expands upon the findings of Cano et al. (56), who identified similar risk factors for MDR-KP infections but did not specifically explore outcomes related to septic shock. Conversely, our results provide a nuanced understanding of septic shock progression in these infections, diverging from Giannella et al. (57), who reported limited predictive utility of certain clinical parameters in similar contexts. This disparity may reflect differences in patient demographics, healthcare settings, and regional MDR-KP strains (58).
The clinical utility of our model is evidenced by its ability to facilitate early identification of high-risk patients, supporting timely and targeted interventions. This predictive capability allows for the stratification of patients into high-risk and low-risk categories based on their individual characteristics. Furthermore, incorporating patient stratification into clinical practice facilitates more precise and personalized care pathways. For example, high-risk patients identified by the model can receive personalized antimicrobial treatment plans tailored to their specific risk profiles, whereas low-risk patients can follow standardized treatment protocols, thereby minimizing unnecessary exposure to broad-spectrum antibiotics and reducing potential side effects. This approach aligns with the principles of precision medicine, which involves customizing treatment strategies according to each patient’s unique characteristics, thereby improving overall clinical outcomes and reducing healthcare costs. This approach is corroborated by Gutiérrez-Gutiérrez et al. (59), who highlighted the benefits of early intervention in managing MDR bacterial infections. Moreover, the model enhances antimicrobial stewardship by enabling precise risk stratification, which could potentially reduce unnecessary use of broad-spectrum antibiotics, aligning with recommendations by Gomez-Simmonds et al. (60). Our findings underscore the importance of specific monitoring parameters such as PCT and markers of acute kidney injury, which are crucial for early intervention and surveillance in MDR-KP-infected patients. These insights contribute significantly to the clinical management of these infections and are supported by robust model validation metrics including AUC, calibration, and decision curve analysis.
While the study underscores the value of the predictive model, the limitations should be acknowledged. One key limitation is the single-center and retrospective design, which may restrict the generalizability of the findings (61). Importantly, variations in MDR-KP strains and healthcare environments across different regions and institutions could influence the model’s applicability. Hence, we acknowledge that the applicability of the model to different populations and healthcare settings, especially those with distinct antimicrobial resistance patterns or patient demographics, may be limited by our current single-center design. To address this, we plan to conduct multi-center validation studies involving diverse cohorts and varying resistance profiles. Such efforts will allow us to confirm the model’s performance across heterogeneous environments, enhance its robustness, and improve its adaptability to a broader range of clinical scenarios. Furthermore, model performance might vary with regional differences in antimicrobial resistance patterns and treatment protocols, a limitation acknowledged in similar studies (62). Future research should focus on multi-center validation as recommended by Zarkotou et al. (63), to assess the model’s applicability in different healthcare settings. Prospective studies, following the methodological approach of Kontopoulou et al. (64), are also needed to confirm the model’s reliability and overcome retrospective biases. Integrating genomic data on MDR-KP strains could further refine the model’s accuracy and uncover strain-specific risk factors, while evaluating the model across diverse patient subgroups, as demonstrated in the stratification methodology by Papadimitriou-Olivgeris et al. (65), will ensure its effectiveness across varied clinical scenarios. Once high-risk patients are identified by the model, several key clinical interventions are recommended. These measures include strengthening infection control practices and promptly optimizing antimicrobial therapy. Prior to the finalization of microbiological results, it may be necessary to consult with infectious disease specialists to rapidly escalate or adjust empiric antibiotic regimens to swiftly control the source of infection. Early initiation of enhanced organ function monitoring, particularly for renal and circulatory support, should be guided by known risk factors such as procalcitonin (PCT) levels and acute kidney injury. Furthermore, high-risk patients may benefit from early admission to the intensive care unit (ICU), individualized mechanical ventilation strategies, careful evaluation of ventilator weaning readiness, and, if necessary, the early initiation of renal replacement therapy or vasoactive support to stabilize hemodynamics. Prompt imaging, surgical consultation, or drainage procedures for intra-abdominal infections can mitigate the progression to septic shock.
In light of the increasingly complex landscape of multidrug resistance, there is a growing need for predictive models capable of handling the multifaceted interplay among diverse resistance mechanisms, host factors, and evolving treatment strategies. Future models should consider incorporating a broader and more dynamic set of clinical and laboratory parameters, alongside advanced machine learning or deep learning frameworks, to capture non-linear and time-dependent patterns. Such enhanced models are expected to provide more accurate risk stratification and improve clinical decision-making, ultimately guiding precision therapy and patient management in the face of complex and rapidly changing MDR scenarios. Our predictive model not only deepens the understanding of MDR-KP-related septic shock but also offers a vital clinical tool for early risk assessment and management, potentially reducing mortality as noted by Hauck et al. (66). The methodologies developed here may also serve as a blueprint for constructing similar predictive models for other antimicrobial-resistant infections, advancing the field of precision medicine in infectious diseases.
This study presents a machine learning-based model that integrates a wide array of clinical and laboratory parameters to assess septic shock risk in patients infected with MDR-KP. By leveraging advanced predictive analytics, the model offers a precise tool for early risk identification, which is expected to improve clinical outcomes, optimize resource allocation, and support targeted treatment strategies. Future work will focus on validating the model across multiple centers with diverse patient populations and varying antimicrobial resistance patterns to ensure its generalizability and enhance its clinical impact. These methodologies not only enhance our current understanding but also pave the way for future innovations in managing antimicrobial-resistant infections.
The original contributions presented in the study are included in the article/Supplementary Material. Further inquiries can be directed to the corresponding authors.
The studies involving humans were approved by Ethics Committee of Huaian No.1 People’s Hospital. The studies were conducted in accordance with the local legislation and institutional requirements. Written informed consent for participation was not required from the participants or the participants’ legal guardians/next of kin in accordance with the national legislation and institutional requirements.
SP: Data curation, Formal analysis, Methodology, Software, Visualization, Writing – original draft. TS: Data curation, Formal analysis, Investigation, Methodology, Visualization, Writing – original draft. JJ: Data curation, Investigation, Methodology, Resources, Software, Writing – original draft. KW: Investigation, Methodology, Software, Writing – original draft. KJ: Methodology, Software, Writing – original draft. YY: Conceptualization, Methodology, Supervision, Validation, Writing – review & editing. CL: Conceptualization, Funding acquisition, Investigation, Project administration, Resources, Supervision, Validation, Writing – review & editing.
The author(s) declare that no financial support was received for the research, authorship, and/or publication of this article.
We extend our profound appreciation to all study participants and the dedicated staff at the Affiliated Huai’an No. 1 People’s Hospital of Nanjing Medical University for their instrumental role in data acquisition and management. Special acknowledgment is due to the manuscript reviewers and editors, whose insightful critiques and editorial expertise substantially enhanced both the scientific rigor and clarity of this work.
The authors declare that the research was conducted in the absence of any commercial or financial relationships that could be construed as a potential conflict of interest.
The author(s) declare that no Generative AI was used in the creation of this manuscript.
All claims expressed in this article are solely those of the authors and do not necessarily represent those of their affiliated organizations, or those of the publisher, the editors and the reviewers. Any product that may be evaluated in this article, or claim that may be made by its manufacturer, is not guaranteed or endorsed by the publisher.
The Supplementary Material for this article can be found online at: https://www.frontiersin.org/articles/10.3389/fimmu.2024.1539465/full#supplementary-material
1. Ranjbar R, Alam M. Antimicrobial resistance collaborators (2022). global burden of bacterial antimicrobial resistance in 2019: a systematic analysis. Evid Based Nurs. (2023) 27:ebnurs-2022-103540. doi: 10.1136/ebnurs-2022-103540
2. Serra-Burriel M, Keys M, Campillo-Artero C, Agodi A, Barchitta M, Gikas A, et al. Impact of multi-drug resistant bacteria on economic and clinical outcomes of healthcare-associated infections in adults: Systematic review and meta-analysis. PloS One. (2020) 15:e0227139. doi: 10.1371/journal.pone.0227139
3. Tacconelli E, Cataldo MA, Dancer SJ, De Angelis G, Falcone M, Frank U, et al. ESCMID guidelines for the management of the infection control measures to reduce transmission of multidrug-resistant Gram-negative bacteria in hospitalized patients. Clin Microbiol Infect. (2014) 20 Suppl 1:1–55. doi: 10.1111/1469-0691.12427
4. Fostervold A, Raffelsberger N, Hetland MAK, Bakksjø R, Bernhoff E, Samuelsen Ø, et al. Risk of death in Klebsiella pneumoniae bloodstream infections is associated with specific phylogenetic lineages. J Infect. (2024) 88:106155. doi: 10.1016/j.jinf.2024.106155
5. Liu KS, Tong YS, Lee MT, Lin HY, Lu MC. Risk factors of 30-day all-cause mortality in patients with carbapenem-resistant klebsiella pneumoniae bloodstream infection. J Pers Med. (2021) 11(7):616. doi: 10.3390/jpm11070616
6. Qian Y, Bi Y, Liu S, Li X, Dong S, Ju M. Predictors of mortality in patients with carbapenem-resistant Klebsiella pneumoniae infection: a meta-analysis and a systematic review. Ann Palliat Med. (2021) 10:7340–50. doi: 10.21037/apm-21-338
7. El-Kady RAE, Elbaiomy MA, Elnagar RM. Molecular mechanisms mediating ceftazidime/avibactam resistance amongst carbapenem-resistant klebsiella pneumoniae isolates from cancer patients. Infect Drug Resist. (2022) 15:5929–40. doi: 10.2147/IDR.S384972
8. Muhsin EA, Sajid Al-Jubori S, Abdulhemid Said L. Prevalence of efflux pump and porin-related antimicrobial resistance in clinical klebsiella pneumoniae in baghdad, Iraq. Arch Razi Inst. (2022) 77:785–98. doi: 10.22092/ARI.2022.356976.1952
9. Liu C, Liu L, Jin MM, Hu YB, Cai X, Wan L, et al. Molecular epidemiology and risk factors of carbapenem-resistant klebsiella pneumoniae bloodstream infections in wuhan, China. Curr Med Sci. (2022) 42:68–76. doi: 10.1007/s11596-021-2480-5
10. Bai XR, Wang ZZ, Li WC, Wang YG, Lou R, Qu X, et al. Clinical efficacy and safety of tigecycline based on therapeutic drug monitoring for carbapenem-resistant Gram-negative bacterium pneumonia in intensive care units. BMC Infect Dis. (2023) 23:830. doi: 10.1186/s12879-023-08815-7
11. Kushner P, Khunti K, Cebrián A, Deed G. Early identification and management of chronic kidney disease: A narrative review of the crucial role of primary care practitioners. Adv Ther. (2024) 41:3757–70. doi: 10.1007/s12325-024-02957-z
12. Pong JZ, Fook-Chong S, Koh ZX, Samsudin MI, Tagami T, Chiew CJ, et al. Combining heart rate variability with disease severity score variables for mortality risk stratification in septic patients presenting at the emergency department. Int J Environ Res Public Health. (2019) 16(10):1725. doi: 10.3390/ijerph16101725
13. Bhagawati M, Paul S, Mantella L, Johri AM, Laird JR, Singh IM, et al. : Deep learning approach for cardiovascular disease risk stratification and survival analysis on a Canadian cohort. Int J Cardiovasc Imaging. (2024) 40:1283–303. doi: 10.1007/s10554-024-03100-3
14. Zhang X, Zhang P, Cong A, Feng Y, Chi H, Xia Z, et al. Unraveling molecular networks in thymic epithelial tumors: deciphering the unique signatures. Front Immunol. (2023) 14:1264325. doi: 10.3389/fimmu.2023.1264325
15. Zhang H, Xia T, Xia Z, Zhou H, Li Z, Wang W, et al. KIF18A inactivates hepatic stellate cells and alleviates liver fibrosis through the TTC3/Akt/mTOR pathway. Cell Mol Life Sci. (2024) 81:96. doi: 10.1007/s00018-024-05114-5
16. Xia Z, Chen S, He M, Li B, Deng Y, Yi L, et al. Editorial: Targeting metabolism to activate T cells and enhance the efficacy of checkpoint blockade immunotherapy in solid tumors. Front Immunol. (2023) 14:1247178. doi: 10.3389/fimmu.2023.1247178
17. Jin W, Yang Q, Chi H, Wei K, Zhang P, Zhao G, et al. Ensemble deep learning enhanced with self-attention for predicting immunotherapeutic responses to cancers. Front Immunol. (2022) 13:1025330. doi: 10.3389/fimmu.2022.1025330
18. Peiffer-Smadja N, Rawson TM, Ahmad R, Buchard A, Georgiou P, Lescure FX, et al. Machine learning for clinical decision support in infectious diseases: a narrative review of current applications. Clin Microbiol Infect. (2020) 26:584–95. doi: 10.1016/j.cmi.2019.09.009
19. Tzelves L, Lazarou L, Feretzakis G, Kalles D, Mourmouris P, Loupelis E, et al. : Using machine learning techniques to predict antimicrobial resistance in stone disease patients. World J Urol. (2022) 40:1731–6. doi: 10.1007/s00345-022-04043-x
20. Magiorakos AP, Srinivasan A, Carey RB, Carmeli Y, Falagas ME, Giske CG, et al. : Multidrug-resistant, extensively drug-resistant and pandrug-resistant bacteria: an international expert proposal for interim standard definitions for acquired resistance. Clin Microbiol Infect. (2012) 18:268–81. doi: 10.1111/j.1469-0691.2011.03570.x
21. Singer M, Deutschman CS, Seymour CW, Shankar-Hari M, Annane D, Bauer M, et al. : the third international consensus definitions for sepsis and septic shock (Sepsis-3). Jama. (2016) 315:801–10. doi: 10.1001/jama.2016.0287
22. Chi H, Jiang P, Xu K, Zhao Y, Song B, Peng G, et al. A novel anoikis-related gene signature predicts prognosis in patients with head and neck squamous cell carcinoma and reveals immune infiltration. Front Genet. (2022) 13:984273. doi: 10.3389/fgene.2022.984273
23. Liu Y, Cheng L, Song X, Li C, Zhang J, Wang L. A TP53-associated immune prognostic signature for the prediction of the overall survival and therapeutic responses in pancreatic cancer. Math Biosci Eng. (2022) 19:191–208. doi: 10.3934/mbe.2022010
24. Jiang S, Yang X, Lin Y, Liu Y, Tran LJ, Zhang J, et al. Unveiling Anoikis-related genes: A breakthrough in the prognosis of bladder cancer. J Gene Med. (2024) 26:e3651. doi: 10.1002/jgm.v26.1
25. Li C, Liu T, Liu Y, Zhang J, Zuo D. Prognostic value of tumour microenvironment-related genes by TCGA database in rectal cancer. J Cell Mol Med. (2021) 25:5811–22. doi: 10.1111/jcmm.v25.12
26. Wang Y, Ma L, He J, Gu H, Zhu H. Identification of cancer stem cell-related genes through single cells and machine learning for predicting prostate cancer prognosis and immunotherapy. Front Immunol. (2024) 15:1464698. doi: 10.3389/fimmu.2024.1464698
27. Zhu C, Sun Z, Wang J, Meng X, Ma Z, Guo R, et al. : Exploring oncogenes for renal clear cell carcinoma based on G protein-coupled receptor-associated genes. Discovery Oncol. (2023) 14:182. doi: 10.1007/s12672-023-00795-z
28. Frost HR, Amos CI. Gene set selection via LASSO penalized regression (SLPR). Nucleic Acids Res. (2017) 45:e114. doi: 10.1093/nar/gkx291
29. Zuo D, Zhang J, Liu T, Li C, Ning G. Claudin-1 is a valuable prognostic biomarker in colorectal cancer: A meta-analysis. Gastroenterol Res Pract. (2020) 2020:4258035. doi: 10.1155/2020/4258035
30. Sun Z, Wang J, Fan Z, Yang Y, Meng X, Ma Z, et al. : Investigating the prognostic role of lncRNAs associated with disulfidptosis-related genes in clear cell renal cell carcinoma. J Gene Med. (2024) 26:e3608. doi: 10.1002/jgm.v26.1
31. Lee S, Gornitz N, Xing EP, Heckerman D, Lippert C. Ensembles of lasso screening rules. IEEE Trans Pattern Anal Mach Intell. (2018) 40:2841–52. doi: 10.1109/TPAMI.34
32. Sauerbrei W, Perperoglou A, Schmid M, Abrahamowicz M, Becher H, Binder H, et al. State of the art in selection of variables and functional forms in multivariable analysis-outstanding issues. Diagn Progn Res. (2020) 4:3. doi: 10.1186/s41512-020-00074-3
33. Degenhardt F, Seifert S, Szymczak S. Evaluation of variable selection methods for random forests and omics data sets. Brief Bioinform. (2019) 20:492–503. doi: 10.1093/bib/bbx124
34. Pang ZQ, Wang JS, Wang JF, Wang YX, Ji B, Xu YD, et al. : JAM3: A prognostic biomarker for bladder cancer via epithelial-mesenchymal transition regulation. Biomol BioMed. (2024) 24:897–911. doi: 10.17305/bb.2024.9979
35. Speiser JL, Miller ME, Tooze J, Ip E. A comparison of random forest variable selection methods for classification prediction modeling. Expert Syst Appl. (2019) 134:93–101. doi: 10.1016/j.eswa.2019.05.028
36. Zuo D, Li C, Liu T, Yue M, Zhang J, Ning G. Construction and validation of a metabolic risk model predicting prognosis of colon cancer. Sci Rep. (2021) 11:6837. doi: 10.1038/s41598-021-86286-z
37. Nembrini S, König IR, Wright MN. The revival of the Gini importance? Bioinformatics. (2018) 34(21):3711–8. doi: 10.1093/bioinformatics/bty373
38. Wang J, Zuo Z, Yu Z, Chen Z, Tran LJ, Zhang J, et al. Collaborating single-cell and bulk RNA sequencing for comprehensive characterization of the intratumor heterogeneity and prognostic model development for bladder cancer. Aging (Albany NY). (2023) 15:12104–19. doi: 10.18632/aging.205166
39. Steyerberg EW, Vergouwe Y. Towards better clinical prediction models: seven steps for development and an ABCD for validation. Eur Heart J. (2014) 35:1925–31. doi: 10.1093/eurheartj/ehu207
40. Riley RD, Ensor J, Snell KIE, Harrell FE, Martin GP, Reitsma JB, et al. Calculating the sample size required for developing a clinical prediction model. Bmj. (2020) 368:m441. doi: 10.1136/bmj.m441
41. Wang Y, Wang J, Liu Y, Wang X, Ren M. Multidimensional pan-cancer analysis of HSPA5 and its validation in the prognostic value of bladder cancer. Heliyon. (2024) 10:e27184. doi: 10.1016/j.heliyon.2024.e27184
42. Strobi C, Malley J, Tutz G. An introduction to recursive partitioning: rationale, application, and characteristics of classification and regression trees, bagging, and random forests. psychol Methods. (2009) 14:323–48. doi: 10.1037/a0016973
43. Li C, Wirth U, Schardey J, Ehrlich-Treuenstatt VV, Bazhin AV, Werner J, et al. An immune-related gene prognostic index for predicting prognosis in patients with colorectal cancer. Front Immunol. (2023) 14:1156488. doi: 10.3389/fimmu.2023.1156488
44. Couronné R, Probst P, Boulesteix AL. Random forest versus logistic regression: a large-scale benchmark experiment. BMC Bioinf. (2018) 19:270. doi: 10.1186/s12859-018-2264-5
45. Zhang C, Sun D, Li C, Liu Y, Zhou Y, Zhang J. Development of cancer-associated fibroblasts subtype and prognostic model in gastric cancer and the landscape of tumor microenvironment. Int J Biochem Cell Biol. (2022) 152:106309. doi: 10.1016/j.biocel.2022.106309
46. Sandell FL, Holzweber T, Street NR, Dohm JC, Himmelbauer H. Genomic basis of seed colour in quinoa inferred from variant patterns using extreme gradient boosting. Plant Biotechnol J. (2024) 22:1312–24. doi: 10.1111/pbi.14267
47. Liu T, Li C, Zhang J, Hu H, Li C. Unveiling efferocytosis-related signatures through the integration of single-cell analysis and machine learning: a predictive framework for prognosis and immunotherapy response in hepatocellular carcinoma. Front Immunol. (2023) 14:1237350. doi: 10.3389/fimmu.2023.1237350
48. Zhou J, Ji N, Wang G, Zhang Y, Song H, Yuan Y, et al. Metabolic detection of Malignant brain gliomas through plasma lipidomic analysis and support vector machine-based machine learning. EBioMedicine. (2022) 81:104097. doi: 10.1016/j.ebiom.2022.104097
49. Zhou W, Yun Z, Wang T, Li C, Zhang J. BTF3-mediated regulation of BMI1 promotes colorectal cancer through influencing epithelial-mesenchymal transition and stem cell-like traits. Int J Biol Macromol. (2021) 187:800–10. doi: 10.1016/j.ijbiomac.2021.07.106
50. Cottrell S, Hozumi Y, Wei GW. K-nearest-neighbors induced topological PCA for single cell RNA-sequence data analysis. Comput Biol Med. (2024) 175:108497. doi: 10.1016/j.compbiomed.2024.108497
51. Yan J, Xu Y, Cheng Q, Jiang S, Wang Q, Xiao Y, et al. LightGBM: accelerated genomically designed crop breeding through ensemble learning. Genome Biol. (2021) 22:271. doi: 10.1186/s13059-021-02492-y
52. Wang Y, Wang J, He J, Ji B, Pang Z, Wang J, et al. Comprehensive analysis of PRPF19 immune infiltrates, DNA methylation, senescence-associated secretory phenotype and ceRNA network in bladder cancer. Front Immunol. (2023) 14:1289198. doi: 10.3389/fimmu.2023.1289198
53. Wang Y, He J, Zhao Q, Bo J, Zhou Y, Sun H, et al. Evaluating the predictive value of angiogenesis-related genes for prognosis and immunotherapy response in prostate adenocarcinoma using machine learning and experimental approaches. Front Immunol. (2024) 15:1416914. doi: 10.3389/fimmu.2024.1416914
54. Xiao J, Lin H, Liu B, Xia Z, Zhang J, Jin J. Decreased S1P and SPHK2 are involved in pancreatic acinar cell injury. biomark Med. (2019) 13:627–37. doi: 10.2217/bmm-2018-0404
55. Zhai X, Zhang H, Xia Z, Liu M, Du G, Jiang Z, et al. : Oxytocin alleviates liver fibrosis via hepatic macrophages. JHEP Rep. (2024) 6:101032. doi: 10.1016/j.jhepr.2024.101032
56. Cano A, Gutiérrez-Gutiérrez B, Machuca I, Gracia-Ahufinger I, Pérez-Nadales E, Causse M, et al. Risks of Infection and Mortality Among Patients Colonized With Klebsiella pneumoniae Carbapenemase-Producing K. pneumoniae: Validation of Scores and Proposal for Management. Clin Infect diseases: an Off Publ Infect Dis Soc America. (2018) 66:1204–10. doi: 10.1093/cid/cix991
57. Giannella M, Trecarichi EM, De Rosa FG, Bono VD, Bassetti M, Lewis RE, et al. Risk factors for carbapenem-resistant Klebsiella pneumoniae bloodstream infection among rectal carriers: a prospective observational multicentre study. Clin Microbiol infection: Off Publ Eur Soc Clin Microbiol Infect Dis. (2014) 20:1357–62. doi: 10.1111/1469-0691.12747
58. Karaiskos I, Giamarellou H. Multidrug-resistant and extensively drug-resistant Gram-negative pathogens: current and emerging therapeutic approaches. Expert Opin Pharmacother. (2014) 15:1351–70. doi: 10.1517/14656566.2014.914172
59. Gutiérrez-Gutiérrez B, Salamanca E, Cueto M, Hsueh PR, Viale P, Paño-Pardo JR, et al. Effect of appropriate combination therapy on mortality of patients with bloodstream infections due to carbapenemase-producing Enterobacteriaceae (INCREMENT): a retrospective cohort study. Lancet Infect Dis. (2017) 17:726–34. doi: 10.1016/S1473-3099(17)30228-1
60. Gomez-Simmonds A, Nelson B, Eiras DP, Loo A, Jenkins SG, Whittier S, et al. Combination regimens for treatment of carbapenem-resistant klebsiella pneumoniae bloodstream infections. Antimicrob Agents Chemother. (2016) 60:3601–7. doi: 10.1128/AAC.03007-15
61. Tacconelli E, Carrara E, Savoldi A, Harbarth S, Mendelson M, Monnet DL, et al. : Discovery, research, and development of new antibiotics: the WHO priority list of antibiotic-resistant bacteria and tuberculosis. Lancet Infect Dis. (2018) 18:318–27. doi: 10.1016/S1473-3099(17)30753-3
62. Tumbarello M, Trecarichi EM, De Rosa FG, Giannella M, Giacobbe DR, Bassetti M, et al. : Infections caused by KPC-producing Klebsiella pneumoniae: differences in therapy and mortality in a multicentre study. J Antimicrob Chemother. (2015) 70:2133–43. doi: 10.1093/jac/dkv086
63. Zarkotou O, Pournaras S, Tselioti P, Dragoumanos V, Pitiriga V, Ranellou K, et al. Predictors of mortality in patients with bloodstream infections caused by KPC-producing Klebsiella pneumoniae and impact of appropriate antimicrobial treatment. Clin Microbiol Infect. (2011) 17:1798–803. doi: 10.1111/j.1469-0691.2011.03514.x
64. Kontopoulou K, Iosifidis E, Antoniadou E, Tasioudis P, Petinaki E, Malli E, et al. The clinical significance of carbapenem-resistant Klebsiella pneumoniae rectal colonization in critically ill patients: from colonization to bloodstream infection. J Med Microbiol. (2019) 68:326–35. doi: 10.1099/jmm.0.000921
65. Papadimitriou-Olivgeris M, Marangos M, Fligou F, Christofidou M, Bartzavali C, Anastassiou ED, et al. Risk factors for KPC-producing Klebsiella pneumoniae enteric colonization upon ICU admission. J Antimicrob Chemother. (2012) 67:2976–81. doi: 10.1093/jac/dks316
Keywords: multidrug-resistant Klebsiella pneumoniae, septic shock, machine learning, predictive model, risk factors
Citation: Pan S, Shi T, Ji J, Wang K, Jiang K, Yu Y and Li C (2025) Developing and validating a machine learning model to predict multidrug-resistant Klebsiella pneumoniae-related septic shock. Front. Immunol. 15:1539465. doi: 10.3389/fimmu.2024.1539465
Received: 04 December 2024; Accepted: 23 December 2024;
Published: 10 January 2025.
Edited by:
Dan Liu, Wuhan University, ChinaReviewed by:
Dongyue Yu, Nankai University, ChinaCopyright © 2025 Pan, Shi, Ji, Wang, Jiang, Yu and Li. This is an open-access article distributed under the terms of the Creative Commons Attribution License (CC BY). The use, distribution or reproduction in other forums is permitted, provided the original author(s) and the copyright owner(s) are credited and that the original publication in this journal is cited, in accordance with accepted academic practice. No use, distribution or reproduction is permitted which does not comply with these terms.
*Correspondence: Chang Li, aGF5eWxpY2hhbmdAMTYzLmNvbQ==; Yabin Yu, eXV5YWJpbmJpbkAxMjYuY29t
†These authors have contributed equally to this work
Disclaimer: All claims expressed in this article are solely those of the authors and do not necessarily represent those of their affiliated organizations, or those of the publisher, the editors and the reviewers. Any product that may be evaluated in this article or claim that may be made by its manufacturer is not guaranteed or endorsed by the publisher.
Research integrity at Frontiers
Learn more about the work of our research integrity team to safeguard the quality of each article we publish.