- 1Department of Oncology, The Fifth Affiliated Hospital of Zhengzhou University, Zhengzhou, China
- 2Marshall B. J. Medical Research Center, Zhengzhou University, Zhengzhou, Henan, China
- 3Department of Gastrointestinal & Thyroid Surgery, The Fifth Affiliated Hospital of Zhengzhou University, Zhengzhou, China
Liver cancer remains one of the most formidable challenges in modern medicine, characterized by its high incidence and mortality rate. Emerging evidence underscores the critical roles of the immune microenvironment in tumor initiation, development, prognosis, and therapeutic responsiveness. However, the composition of the immune microenvironment of liver cancer (LC-IME) and its association with clinicopathological significance remain unelucidated. In this review, we present the recent developments related to the use of artificial intelligence (AI) for studying the immune microenvironment of liver cancer, focusing on the deciphering of complex high-throughput data. Additionally, we discussed the current challenges of data harmonization and algorithm interpretability for studying LC-IME.
1 Introduction
Liver cancer poses huge health challenges due to escalating global incidence, notably in transitional regions like East and Southeast Asia. It currently ranks 6th in cancer incidence and 3rd in mortality, surpassed only by lung and colorectal cancers (1). Surgery provides relatively satisfactory outcomes when detected at an early stage, liver transplantation at early-stage liver cancer patients achieved a 5-year survival of about 70-80.0%. Surgical resection or tumor ablation can reach a 5-year survival rate of 50% to 70% (2–5). For patients with locally advanced liver cancer, Trans-arterial Chemoembolization (TACE), either in combination with other treatments or as a standalone therapy, yields a 5-year survival rate of 20% to 40% (4, 6).
Systemic therapy has witnessed significant breakthroughs in targeted therapy and immune therapy in the past two decades, which have not only improved survival in advanced patients but also made some of them suitable for surgical removal. Even so, liver cancer remains one of the worst-prognosed diseases due to late diagnosis, drug resistance, and frequent recurrence and metastasis (7). The chances of survival of the patients with liver cancer at late stage are low due to the lack of effective drugs, meaning that patients typically live for only 6 to 20 months after diagnosis (8). This underscores the urgent need for effective treatments (9).
Liver cancer has several subtypes, including hepatocellular carcinoma (HCC), bile-duct cancer, hepatoblastoma, and various liver sarcomas and carcinomas. Among them, HCC is the most common worldwide, whereas, in some Asian countries, bile-duct cancer is more common than HCC. This regional variation may result from different risk factors, such as hepatitis B virus, hepatitis C virus, fungi, aflatoxin, alcohol, poor diet, and parasitic flatworm (10, 11). It is still unclear that why some people can live with liver disease for many years, whereas others develop fatal cancer. Increasing evidence suggests the alterations of the liver immune microenvironment play a key role during cancer transformation and drug resistance. However, the heterogeneity and intricate molecular dynamics impede a deep understanding of the immune microenvironment of liver cancer.
In this review, we first provide a brief overview of AI and describe its common applications in cancer research. We also illustrated the immunological characteristics of the liver and its pathological alterations during cancer development. Subsequently, we explored the latest applications of AI and current challenges within the context of LC-IME.
2 Applications of AI in cancer research
2.1 AI and machine learning
AI technology involves the development of systems capable of executing tasks typically requiring human intelligence, such as reasoning, learning, and problem-solving. It is designed to replicate cognitive processes like perception, language processing, and decision-making, these systems draw from a diverse range of disciplines, including computer science, mathematics, psychology, and linguistics. AI technology has penetrated all aspects of human activities (12–15). In the cancer research field, AI is characterized by the use of machine learning and deep learning algorithms (16), which are important in processing and analyzing large-scale datasets (17–19).
Machine learning (ML), an integral part of artificial intelligence, encapsulates the autonomous identification of patterns and formulations within vast datasets (20). By discerning and extracting significant features from the data, ML can make accurate predictions and decisions .It fundamentally extracts patterns and rules from the data and apply them to new data. The workflow of ML comprises the acquisition, pre-processing, feature extraction of the data, model training, and evaluation optimization application of the obtained model. During model training, parameters are adjusted to minimize the discrepancy between predicted and actual outcomes, known as ‘error’ or ‘loss,’ which is quantified to direct the optimization process towards enhanced accuracy. Based on the model training approaches, there are four different types of ML: supervised (21), unsupervised (22, 23), semi-supervised (24), and reinforcement learning (25) (Figure 1).
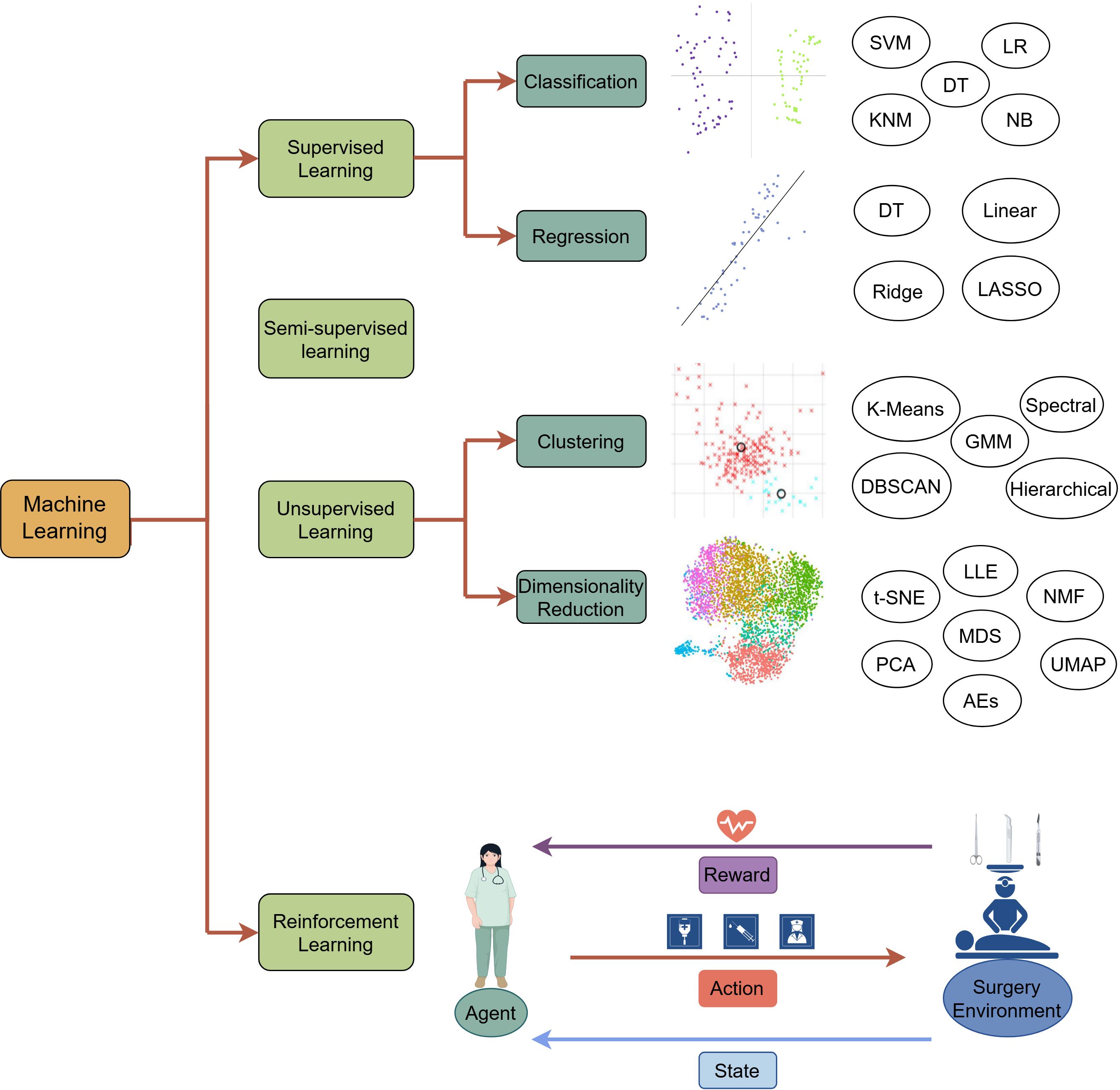
Figure 1. The overview of machine learning paradigms. Supervised learning: Trains models on a labeled dataset, where the training dataset consists of input data and corresponding output labels, allowing the model to be able to make accurate predictions on classification and regression tasks. Semi-supervised learning: Trains the model with a small amount of labeled data and applies the model trained to annotate unlabeled data. Unsupervised learning: Discovers hidden patterns, structures, or subgroups in the unlabeled data through clustering and dimensionality reduction. It uses datasets without clear notice of the dependent (response) variable. Unsupervised means that the machine or computer should learn patterns from the data without referring to any specific response. Unsupervised learning aims to explore the data structure and generate a hypothesis rather than to test any hypothesis by statistical methods or to construct prediction or classification models on the basis of a set of conditions and a specified response. Algorithms for unsupervised learning can be subdivided into two categories: (1) clustering algorithms and (2) dimensionality reduction. Reinforcement learning: Identifies a sequence of actions to increase the probability of achieving a predetermined goal. A RL problem is solved through a trial-and-error learning process. A RL agent interacts with an environment to maximize the cumulative reward resulting from its actions. Generally, RL problems are modeled and solved using a Markov Decision Process (MDP), guided by Bellman’s equation. There are four components: (1) a state that represents the environment at each time step; (2) an action the agent takes at each time step that influences the next state; (3) a transition probability that provides an estimate for reaching different subsequent states, which reflects the environment in which an agent interacts; and (4) a reward function, which is the observed feedback given a state-action pair. LR, Logistic Regression; DT, Decision Tree; NB, Naïve Bayes; SVM, Support Vector Machine; NN, K-nearest Neighbor; Ridge, Ridge Regression; Linear, Linear Regression; LASSO, Least Absolute Shrinkage and Selection Operator; GMM, Gaussian Mixture Model; DBSCAN, Density-Based Spatial Clustering of Applications with Noise; PCA, Principal Component Analysis; MDS, Multidimensional Scaling; NMF, Non-negative Matrix Factorization; LLE, Locally Linear embedding; t-SNE, t-Distributed Stochastic Neighbor Embedding Algorithm; UMAP, Uniform Manifold Approximation and Projection; AEs, Autoencoders.
Deep learning (DL), a subfield of machine learning, employs artificial neural networks (26) to represent important information from massive amounts of data. DL comprises an input layer, multiple hidden layers, and an output layer, each of which receives the output of the previous layer as input and performs nonlinear transformations that progressively distill raw data into meaningful feature abstractions. There are several popular DL architectures: multilayer perceptron (MLP), convolutional neural networks (CNNs), recurrent neural networks (RNNs), auto-encoders (AEs), generative adversarial networks (GANs) and transformer (Figure 2). These architectures can be used according to the specificity of the data.
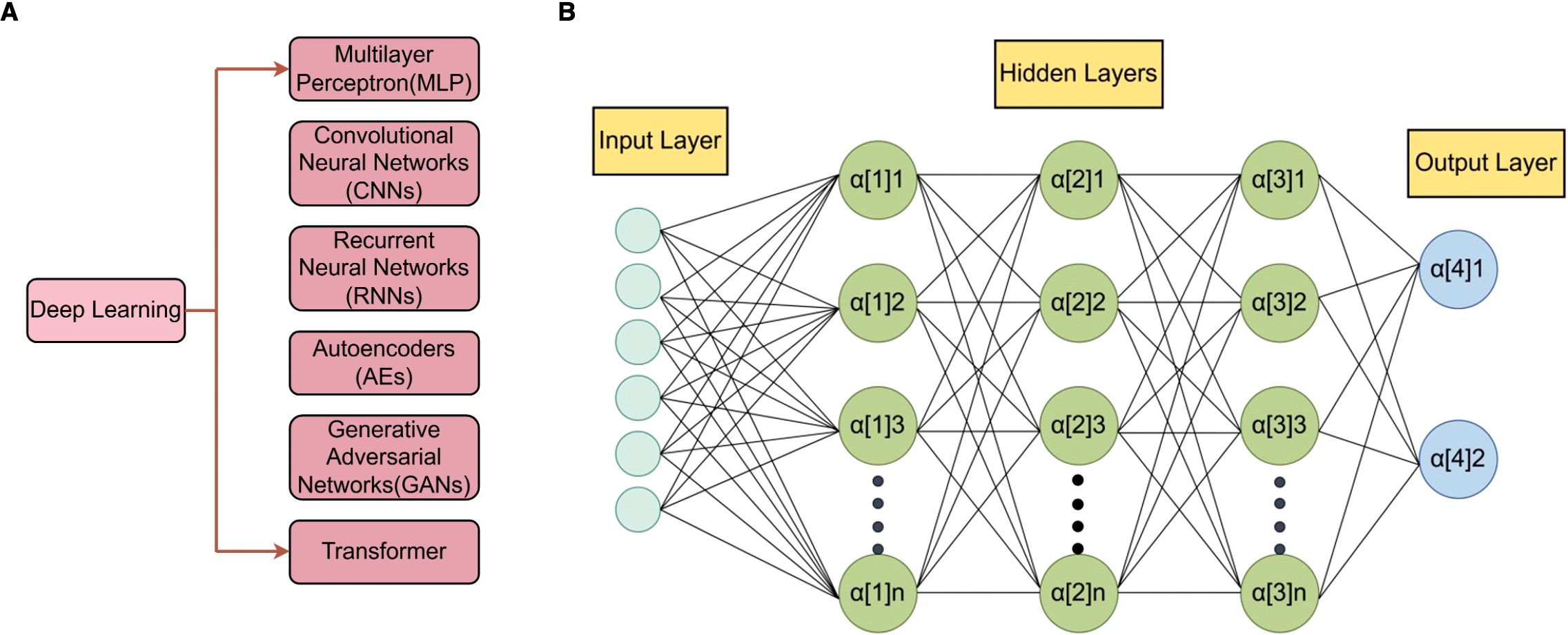
Figure 2. The overview of deep learning paradigms. (A), Deep learning is a subfield of machine learning. It employs artificial neural networks for representation learning from massive amounts of data. A deep neural network consists of an input layer, multiple hidden layers, and an output layer, each of which receives the output of the previous layer of neurons as input and performs nonlinear transformation processing, thereby gradually transforming the raw data into meaningful feature representations. (B), The composition of multilayer perceptron (MLP) is shown as an example of deep learning. The input layer receives data, where each neuron corresponds to a feature of the input data. The hidden layers perform computations and transformations on the input data through weighted sums and non-linear activation functions. These processed signals are then conveyed to the output layer, which generates the final output of the network.
2.2 Application of AI in cancer research
Early cancer detection: By facilitating cancer detection at the precancer stage, AI allows for early interventions that significantly prolong the overall survival time of the patients. For example, Klein et al. used a blood-based multi-cancer early detection (MCED) test and applied cell-free DNA sequencing, combined with machine learning, which predicted the origin of cancer signals with high specificity and accuracy in a variety of cancers (27). Similarly, Stark et al. constructed machine learning models using Gail model inputs and personal health data. These models exhibit strong performance in predicting breast cancer risk and can be used as non-invasive tools to increase early detection and prevention of breast cancer (28). Additionally, to develop a machine learning model to predict the risk of lymph node metastasis in renal carcinoma, Feng et al. filtered clinical features through LASSO and univariate and multivariate logistic regression analyses and then used statistically significant risk factors to build the XGB model. It could distinguish about 89% of LNM patients when the threshold probability was set to 54.6%, suggesting a promising application prospect in the clinic (29).
Machine learning and deep learning emerge as potent tools to identify biomarkers from intricate datasets (30). For example, Halner et al. established a random forest-based machine learning pipeline, “Decancer,” to analyze liquid biopsies. Decanter enhanced the sensitivity for detecting stage I cancer from 48% to 90% regardless of cancer type. Promisingly, DEcancer’s performance using a 14-43 protein panel is comparable to 1,000 original proteins (31). To identify metabolomic biomarkers for the diagnosis and prognosis of gastric cancer, Chen et al. used the LASSO regression algorithm to build a 10-metabolite GC diagnostic model, which is validated in an external test set with a sensitivity of 0.905. This model exhibited superior performance to traditional models that utilized clinical parameters and identified two distinct biomarker panels, enabling early diagnosis and prognosis of cancer (32). Tayob et al. developed the parametric empirical Bayes algorithm and the Bayesian screening algorithm to improve the early detection of cancer, which improved sensitivity to cancer biomarkers (33). Furthermore, Konstantinos et al. tested the miRNA expression profiles of Gastrointestinal stromal tumors (GISTs) and applied machine learning to identify the miRNAs associated with the risk of GIST development. They found that several miRNAs, with hsa-miR218-5p as the best, may strongly affect the prognosis of GISTs and can serve as predictors for their development (34). In short, the application of AI and machine learning in oncology clinics has improved diagnostic time and clinical outcomes in various cancers.
Medical imaging: Imaging is at the forefront of clinical care. The integration of AI into image interpretation helps radiologists streamline workflow and improve patient care (35). Within imaging, convolutional neural networks (CNNs) and Deep Learning (DL) are exceptionally useful in computer vision and enable machines to see and interpret visual data (36, 37). Al-Masni et al. developed the ROI-based Convolutional Neural Network “You Only Look Once (YOLO)” to accurately detect and classify the masses in mammograms. It achieves an overall accuracy of 96.33% in detecting the mass location and 85.52% in distinguishing between benign and malignant lesions (38). Zhao et al. built the deep-learning-based, fully automated lymph node detection and segmentation (auto-LNDS) model based on multiparametric magnetic resonance imaging (mpMRI). The auto-LNDS achieved a sensitivity, PPV, and FP/vol of 80.0%, 73.5%, and 8.6 in internal testing and 62.6%, 64.5%, and 8.2 in external testing, respectively, significantly better than the performance of junior radiologists, therefore holding great potential for facilitating N-staging in clinical practice (39). Jin et al. developed a CNN-based algorithm to Improve the accuracy in Optical Diagnosis of Colorectal Polyps. It increased the accuracy of novice endoscopists to 85.6% and significantly reduced the skill-level dependence of endoscopists and costs (40).
Pathological identification: As the gold standard for confirming cancer, pathological identification holds paramount significance in diagnosis, prognosis, and therapeutic strategies. However, the heterogeneity of tumors poses a big challenge to precise diagnosis (41, 42). AI has transformed the landscape of cancer pathology by empowering it with enhanced diagnostic accuracy and streamlined decision-making frameworks, leveraging sophisticated histology image analysis (43). For example, to achieve an AI-based pathological prediction of the origins of unknown cancers. Lu et al. build the Tumor Origin Assessment via Deep Learning (TOAD), a deep-learning-based algorithm that provides a differential diagnosis for the origin of the primary tumor based on routinely acquired histology slides (44). In addition, Lee et al. presented a graph deep neural-network model to analyze the whole-slide images (45). This model considers histopathological features from the tumor microenvironment. in gigapixel-sized WSIs in a semi-supervised manner and was trained to provide interpretable prognostic biomarkers in patients with kidney, breast, lung, and uterine cancers.
Treatment: The outcomes of cancer treatment are affected by several key factors, such as the patient`s health status, cancer subtype, and stage. Additionally, molecular cancer research has recently revealed the contribution of genetic mutations to patients` responses to a specific treatment. The complex interplay of the above factors in the real world poses a significant challenge for oncologists in selecting the appropriate treatment regimen for a specific patient.
In this scenario, AI is inherently a powerful approach to the integration and aggregation of intricate and multi-dimensional datasets and providing comprehensive data support for decision-making (46, 47). For example, Luo et al. proposed a collaborative filtering method with machine learning. It can identify the most suitable compounds for patients without genetic data, making it feasible to predict drug sensitivity and achieve personalized drug selection in a cost-effective way (48). Abajian et al. used Supervised Machine Learning with both Logistic Regression (LR) and Random Forest (RF) algorithms to explore the treatment response to transarterial chemoembolization for hepatocellular carcinoma. Both LR and RF models achieved an overall accuracy of 78% and identified cirrhosis status and relative tumor signal intensity (>27.0) as the two strongest predictors of treatment response (49). Kong et al. introduced a NetBio-based machine learning, which accurately predicted the treatment responses to Immune checkpoint inhibitors (ICIs) in three different cancer types-melanoma, gastric cancer, and bladder cancer. This model demonstrated superior performance in comparison with conventional ICI treatment biomarkers, such as the expression profiles of ICI targets (50).
Prognosis and management: Understanding the progression and survival time of patients is essential for cancer management. Oncologists used to predict patients’ prognoses based on their experience of understanding patients’ clinical profiles (age, health status) and tumor characteristics (subtype, stage, and grade). Nevertheless, this strategy is inherently limited and has a low predictive capability due to individual variation. AI has exhibited great promise to deal with these constraints and achieves accurate prognosis prediction for individual patients (51). Qiu et al. developed an XGBoost model to help physicians make clinical decisions. It employed clinicopathological information and predicted the risk of distant metastasis in patients with rectal cancer (52). Based on LASSO regression and Pearson correlation coefficients, Cai et al. identified metastasis-associated genes from different cancer tissues and then used them to build a CNN-based model, “Multi-Dimensional Convolutional Neural Network (MDCNN).” It achieved satisfactory prediction accuracy in bone metastasis, lung metastasis, and liver metastasis (53). The combination of AI and the Internet of Things (IoT) technology enables telemedicine and intelligent monitoring functions, allowing patients to receive scenario-based remote management (54).
Drug Discovery: Drug discovery and development of anti-cancer drugs is the goal of translational medicine. However, this work is quite a costly and time-consuming operation. Additionally, although the advances in muti-omics and clinical trials provide quite meaningful information, their complexity also imposes a huge obstacle. AI and computer-aided drug design, along with modern experimental technical knowledge, has energized data mining for faster drug design and development in the pharmaceutical industry (55). For example, AlphaFold2, a deep neural network algorithm, demonstrates high accuracy in predicting the three-dimensional structures of proteins, particularly when sequences of multiple homologs are available. It helps us understand protein function changes underlying carcinogenesis and improve our approaches to counter them (56). Meanwhile, low-cost cancer drug repurposing can be achieved by deep learning approaches, which aid the modeling of existing drugs for discovering novel drug targets. For example, Zhou et al. designed a prediction approach called an ensemble of multiple drug repositioning approaches (EMUDRA). Using EMUDRA, they predicted and experimentally validated the antibiotic rifabutin as an anti-cancer drug for triple-negative breast cancer (57).
3 Liver immune microenvironment and its alterations in cancer
The liver is not only an important metabolic organ but also possesses significant immune functions, and it contains a vast array of immune cells (Table 1). Several factors contribute to the unique immune functions of the liver. Firstly, the liver is a hematopoietic organ during embryonic development. Secondly, the flow of portal venous blood carries components from the gastrointestinal tract and spleen (58). Thirdly, the liver participates in mucosal immunity through the biliary system. Due to the liver’s direct exposure to many antigens from the gastrointestinal tract, it has developed a unique immune tolerance, which is manifested as intrinsic tolerance mechanisms in both innate and adaptive immune responses. Therefore, the liver can protect itself from autoimmune damage caused by the extensive presentation of gastrointestinal antigens (59, 60). However, in the context of liver injury and disease, various liver cells participate in complex pro-inflammatory responses, which may lead to hepatocyte death and further disease progression (61).
Innate immune system: In the liver, the innate immune system forms the first line of defense against pathogens, present at birth and lasts throughout life. Immunity against various pathogens or malignant cells is provided by different types of immune cells (62). These cells include neutrophils, natural killer cells, Kupffer cells, monocytes, dendritic cells, and natural killer T cells (NKT) (63). Neutrophils, the most abundant group of circulating white blood cells, constitute the first line of defense in acute inflammation by phagocytosing and killing invading microorganisms (64). Natural killer cells Identify and kill cells infected with viruses and tumor cells (65). They don’t require secondary activation for their cytolytic activity. Instead, they induce apoptosis in tumor cells by activating FasL or TRAIL (66, 67). In case of their inactivation or restricted infiltration to the liver, tumor cells grow rapidly (68, 69). Kupffer cells are resident macrophages in the liver and constantly in contact with antigens from the gastrointestinal tract (70–72). Bleriot et al. identified two distinct populations of Kupffer cells, which share core molecular characteristics but express different genes and proteins (73). Additionally, the liver also recruits a large number of monocytes from peripheral blood and converts them into macrophages in the liver microenvironment (monocyte-derived macrophages). Different subtypes of macrophages can be distinguished with the specific expression of cell markers, such as CD11b, CCR2, and F4/80 (74–76). M1 macrophages mainly express CD16 and CD32, etc., and also produce TNFα, nitric oxide (NO), and reactive oxygen intermediates (ROI) to play antitumor roles, while M2 macrophages express several surface molecules such as CD163, Dectin-1, etc., and release interleukins (IL-4 and IL-13) and glucocorticoids, mainly perform the immunosuppressive pro-tumor activity (77, 78). Dendritic cells, also known as antigen-presenting cells, identify affected cells or pathogens and present them to other immune cells, thus maintaining immune tolerance and regulating liver-specific immune responses (79). NKT cells are unconventional T cells that are activated by glycolipid antigens (80, 81). They have both NK cell surface markers and antigen receptor characteristics of T cells and serve as a bridge between innate and adaptive immunity (82). The NKT cells that are located in hepatic sinusoids provide intravascular immune surveillance (83) where they may mediate proinflammatory effects through type I NKT cell subsets or exhibit immunosuppressive functions via type II NKT cells (84). In short, these immune cells coordinate with each other to accomplish the innate immune response in three steps: early inflammation, amplification of the inflammatory signal, and resolution.
Adaptive immune system: Several subtypes of T cells abundantly exist in healthy liver, including CD4+ helper T (Th) cells, CD8+ cytotoxic T cells, and regulatory T cells (Tregs) (85). CD4+ T cells are crucial for preventing tumorigenesis by facilitating the elimination of malignant cells (86–88). They typically act as initiators of antitumor responses and correlate with favorable responses to immunotherapy. CD8+ cytotoxic T cells serve as the primary effector cells of the cellular immune system, which recognize presented antigens and kill infected or malignant cells (89). Additionally, a population of CD8+ tissue-resident memory (TRM) cells exist in the liver, functioning as local immune sentinels (90, 91). Tregs are a subset of CD4+ T cells with immunosuppressive properties. These cells are crucial for maintaining homeostasis and immune tolerance (92, 93). Accumulation of Tregs has been implicated in facilitating immune evasion and hepatocarcinogenesis (92, 93).
B cells are a group of specialized cells that produce specific antibodies, participate in antigen presentation, and regulate the activities of other immune cells (94).
The development of liver cancer is highly related to infection and inflammation, which foster the unique Immunosuppressive microenvironment of liver cancer. It is characterized by blunted anti-tumor immunity, an enrichment of tumor-promoting immunosuppressive cell types, and impaired innate and adaptive immunity (95–99). Recently, immune checkpoint inhibitors (ICIs) have demonstrated promising clinical benefits in HCC, thus emphasizing the importance of immunotherapy (100). Apart from ICIs, immunotherapy also encompasses adoptive cell therapy, oncolytic virotherapy, and cancer vaccine therapy. These approaches can improve T-cell function and enhance cellular immunity, thereby leading to the elimination of LC-IME and the inhibition of tumor growth. To guide the application of immunotherapy, more efforts are needed to gain a deeper understanding of LC-IME.
4 The integration of AI and the immune microenvironment of liver cancer
In liver cancer, the intricate heterogeneity, consisting of diverse immune and stromal cells, significantly contributes to metastasis, relapse, and drug resistance (101–103). The exploration of the tumor immune microenvironment and complex cellular interactions can provide crucial insights for developing more effective, tailored immune-oncology therapies. However, the sheer volume and complexity of data from single-cell RNA sequencing (scRNA-seq) and multi-omics pose challenges for direct clinical application. In addressing these challenges, artificial intelligence (AI) is increasingly recognized as a potent tool that enhances our understanding of these large-scale datasets.
4.1 The integration of AI and omics data
scRNA-seq analysis: scRNA-seq allows researchers to conduct in-depth analysis of molecular characteristics, such as gene expression and epigenetic modifications within individual cells, generating vast amounts of genetic information data (104). The analysis of these data is crucial for revealing cellular heterogeneity and functional characteristics (105). With the intervention of AI, rapid processing and interpretation of massive scRNA-seq data can be achieved with enhanced data accuracy. AI algorithms can automatically identify and filter out noise, retaining the true biological differences between cells and thereby improving data reliability (106). Additionally, batch effects, a common issue in scRNA-seq analysis, can be caused by various factors such as experimental samples, platforms, and library construction methods. AI technologies can effectively eliminate these batch effects while preserving biological differences by projecting high-dimensional data into a low-dimensional cellular embedding space through an asymmetric autoencoder structure (107). In fact, these methods not only improve the accuracy of data integration but also enable online data integration and comparative analysis of new data with existing data.
By use of different machine learning approaches, cell type identification models are developed to recognize cell types and subtypes (108). These models can extract key biological insights to predict the changes in gene expression levels or even dynamic changes in gene interaction networks. For example, scRobust, a self-supervised learning strategy built on the transformer architecture, has demonstrated effectiveness in cell-type annotation and drug tolerance detection (109). A deep learning model “Enformer Celltyping” predicts epigenetic signals across cell types. It overcomes the limitations of existing machine learning approaches, which are confined to the cell types they were trained on (110). Here, we summarized cell-type identification models in Table 2.
Multi-omics analysis: Complex and dynamic networks of molecules in LC-IME make a single layer of “omics” unable to provide deep insights into the underlying mechanisms. Recent technological advancement in high-throughput measurement of genome (111, 112), epigenome (113, 114), metabolome (115), transcriptome (116), and proteome (117) allows comprehensive multi-omic studies. Multi-omics approaches are pivotal in identifying new therapeutic targets (118) and predicting patients’ responses to treatments (119). The data from different omics can be cross-fused and mutually verified, providing a more reliable, comprehensive, and systematic perspective (120, 121). Through the integrated analysis of omics data, in-depth biological data that cannot be obtained by a single omics technology can be uncovered (122, 123). However, the advantage of multi-omics data integration comes with the extra complexity deriving from inherently diverse types of omics datasets, which may pose a challenge to integrateing the omics data in a biologically meaningful manner (124). The experimental data generated across diverse laboratories often cannot be seamlessly amalgamated due to inherent constraints. Additionally, the inherent heterogeneity of multi-omic datasets, stemming from technical, biological, chemical, and physical sources, poses significant challenges for interpretation (125).
With the continuous development of AI technologies, the integration of AI and multi-omics has emerged as one powerful solution to these challenges (126–128). AI has remarkable capabilities in deciphering complex patterns and extracting meaningful insights from large and intricate datasets (129–131). This enables researchers to more systematically analyze the complexity of biological systems (132), reveal the interactions and regulatory mechanisms between different molecular layers, and more accurately identify disease-related molecular markers and potential drug targets (133, 134). This subsequently contributes to the development of personalized medicine and precise treatment plans, improving therapeutic effects and reducing side effects (16, 135, 136).
Data-based Integration. This methodology has proven effective in several studies. Zhang Team merged information from single-nucleotide polymorphisms (SNPs) and transcriptomic profiles into a single matrix, which uses a Bayesian integrative model to facilitate the investigation of their interplay and enable the prediction of quantitative phenotypes (137). To predict remission rates and survival outcomes in ovarian cancer, Mankoo and colleagues integrated the data of copy number alteration, DNA methylation, microRNA, and gene expression and performed a multivariate Cox-LASSO analysis (138). Shen et al. proposed the iCluster framework for glioblastoma subtyping. This framework harmoniously and integrated, with a common set of latent variables, three distinct omics data of copy number alteration, gene expression, and DNA methylation (139).
Model-based Integration. In a model-centric integration framework, distinct models tailored to individual data perspectives are initially formulated, subsequently converging through a fusion process of their respective outputs. For example, the ATHENA tool (140–142), which is designed for investigating heritable and environmental network associations, integrates different omics data of copy number alterations, DNA methylation, miRNA, and gene expression to uncover correlations with clinical endpoints. This integration involves constructing foundational models and neural networks per omics type, ultimately leading to the construction of an integrated model (137). Wang’s team used Similarity Network Fusion (SNF) for cancer subtyping. It begins by creating patient similarity matrices based on DNA methylation and the expression of mRNA expression or miRNA and moves to an iterative nonlinear integration, where the three foundational similarity matrices converge into a unified matrix (143). To predict drug resistance in HIV protease mutants, Dr. Ghici and Potter devised an ensemble-based strategy. It sets up the basic predictive models with structural characteristics of the HIV protease-drug inhibitor complex and DNA sequence variations, respectively, and then orchestrates a majority voting system to enhance the accuracy of drug resistance prediction (144).
4.2 Current achievements of AI-guided scRNA-seq for cellular identification in LC-IME
4.2.1 Neutrophil
Neutrophils play a key role during the initiation of innate immunity and the shaping of adaptive immunity. Several subtypes of Tumor-associated neutrophils (TANs) exist with different functions and markers: the antitumor N1, the protumor N2, and polymorphonuclear myeloid-derived suppressor cells (PMN-MDSCs) (145, 146). Tumor cells or other stromal cells in LC-IME educate TANs polarization towards pro-tumor phenotype through the secretion of cytokines or chemokines, such as GM-CSF, IL-6, TGF-β, and E2 PGE2 (147). Furthermore, the elevated neutrophil-lymphocyte ratio is associated with advanced cancer stage, aggressive tumor characteristics, as well as recurrence after resection but varies with etiology (148). Neutrophil extracellular traps (NETs), a unique structure produced during neutrophil death, have been shown to promote HCC metastasis by provoking inflammatory responses (149–152). However, due to the existence of high heterogeneity, some subsets of neutrophils have pro-tumor effects, while others appear to have anti-tumor effects, the overall influence of neutrophils on cancer therapy remains obscure (153–155). Zhang's team performed scRNA-seq analysis and stratified patients into five subtypes, including immune activation, immune suppression mediated by myeloid or stromal cells, immune exclusion, and immune residence phenotypes, which were spatially organized and associated with chemokine networks and genomic features. Notably, the abundance of tumor-associated neutrophils (TANs), particularly prominent within the myeloid-cell-dominated subtype, emerged as a harbinger of an adverse clinical prognosis. Depletion of TANs in mouse models significantly attenuated tumor progression, thereby shedding a promising light on therapeutic targets for innovative immunotherapeutic strategies (156). Neutrophils also showed resistance to anti-PDL-1 therapy in HCC via T-cell exhaustion (156). Interestingly, due to shorter lifespan and less abundance of RNAs, Neutrophils are difficult to identify by single-cell sequencing. However, application of optimized workflows (such as no enrichment strategy) (156) or capture methods (such as the BD Rhapsody platform) (157) made it possible to identify them. Still, some neutrophils with unique transcriptomic and functional features are identified in HCC by scRNA sequencing. Neutrophils expressing MMP8, CD74, SPP1, etc in HCC are considered tumor-associated neutrophils. Importantly, Particularly, CD10+ ALPL+ neutrophils hinder anti-PD-1 therapy by permanently destroying the T-cell (153). Suggesting that identifying and targeting neutrophils in HCC is essential for successful clinical outcomes.
4.2.2 Macrophages
Tumor-associated macrophages (TAMs) are one of the most abundant innate immune cells and are observed at all stages of tumor progression in the LC-IME (95). According to the difference of functions in tumor progression, there are the classical M1 subtype and the alternative M2 subtype. The M1 phenotype is induced by pro-inflammatory cytokines such as IL-1β, IL-6, IL-12, and tumor necrosis factor-α (TNF-α), whereas the M2 phenotype is polarized by immunomodulatory molecules such as IL-4, IL-10, macrophage colony-stimulating factor (M-CSF), and transforming growth factor-β (TGF-β) (158). TAMs promote liver cancer progression through various approaches, including angiogenesis, cancer cell proliferation, immunosuppression, extracellular matrix remodeling, and drug resistance to therapeutic agents (159). TAMs express inhibitory immune checkpoint proteins, such as PD-1, PD-L1, and TIM-3, secret the immunosuppressive cytokine IL-6, and recruit Tregs (160–162). Furthermore, TAMs are important bridges between tumor cells and other immune effector cells. M2 TAMs secrete IGF-1 and CCL20 to recruit Tregs and impair CD8+ T cell function (163). FasL+CD11b+F4/80+ monocyte-derived macrophages interact with the activated antigen-specific Fas+CD8+ T cells and make them undergo apoptosis. The elimination of these hepatic macrophages significantly increased the survival of hepatic T cells (164). Moreover, Osteopontin (OPN), a pro-metastatic gene, promotes macrophage infiltration and PD-L1 expression in HCC by activating CSF1/CSF1R pathway (165). Conversely, upon appropriate stimulation, macrophages exhibit remarkable anti-tumor capabilities, such as phagocytosis of cancer cells and cytotoxic tumor eradication (159). Therefore, Macrophage-targeting strategies have the potential to synergize with current therapeutic tools to improve the outcomes of patients with liver cancer. Single-cell sequencing has identified several new subtypes of macrophages in HCC. For instance, there are two major types of macrophages: C1QA+ and THBS1+ macrophages (166). Among them, THBS1+ macrophages are myeloid-derived suppressor cells (MDSC)-like cells. However, C1QA+ are considered TAM-like macrophages, which have properties of both M1 and M2 macrophages and highly express APOE, GPNMB, and SLC40A1 (98) and are associated with poor prognosis of liver cancer. The accumulation of LAIR1+ and TIM3+ TAM macrophages reduced the infiltration of CD8+T cells and was associated with poor prognosis of HCC patients (167). Similarly, the abundance of the APOC1+ macrophages was comparatively higher in HCC tissues, inhibiting APOC1 improves the effects of anti-PD-1 therapy by reshaping M2 macrophages into the M1 macrophages (168). Considering the key role of macrophages (TAM) in cancer development, chemical inhibitors are being trialed, such as the combination of CCR2/CCR5 antagonists (targeting macrophages) with Nivolumab is currently in phase II clinical trials (NCT04123379). The complex functions of TAMs have sparked great interest in developing new therapeutic strategies targeting macrophages.
4.2.3 Dendritic cells
Dendritic cells (DCs), functioning as antigen-presenting cells (APCs), interact with diverse immune cells and form a vital mediator between innate and adaptive immunity. There are two types of DCs, including Conventional DCs (cDCs) and plasmacytoid DCs (pDCs). The primary responsibility of cDCs (either cDC1 or cDC2) is antigen presentation, whereas pDCs are specialized for antiviral and antitumor immunity via the secretion of type I interferons (169),
In immunosuppressive tumor microenvironment, DC cells can be functionally reshaped and lose their antitumor functions. Tregs suppress the expression of HLA-DR and impair the antigen-presenting function of cDC2 cells (170, 171). DC cells often play an immunosuppressive role, and the enrichment of tumor-infiltrating pDCs was correlated with Tregs infiltration as well as poor prognosis in patients with HCC (172, 173). As the immune response to immunotherapy largely depends on DC cells, many strategies have been evaluated for stimulating DC cells in HCC patients, such as DC vaccines (174), nanodrugs (175, 176), and DC-derived exosomes (177), some of which have been demonstrated to activate tumor-specific immunity. Advanced scRNA-seq has identified the heterogeneous nature of dendritic cells (DC) in HCC, thus revealing diversity in their functions. These heterogeneous mature DCs, including CCR7+ LAMP3+ DCs, can migrate from tumors to lymph nodes, interfering with T cell function, including exhausted T cells (TEX) and Tregs cells (98). They are also found in lung cancer because they also express immune regulatory markers (Cd274, Pdcd1lg2, and Cd200) and maturation markers (Cd40, Ccr7, and Il12b); thus, they are named as mature dendritic cells enriched in immune regulatory molecules (mregDCs) (178). In the context of ICI treatment, a cellular triad composed of mregDCs, CXCL13+ helper T (Th) cells, and PD-1hi progenitor CD8+ T cells is significantly enriched in the HCC microenvironment. Communication between mregDCs and CXCL13+ Th cells within these cellular triads helps in the differentiation of progenitor CD8+ T cells into effector antitumor CD8+ T cells (179). Similarly, CXCR3+ CD8+ effector memory T (TEM) cells and HLA-DR+ cDC1 recruited to determine the responsiveness of HCC to ICI (180).
4.2.4 T cells
CD8+T cells exhibit an exhausted phenotype and are incapable of halting tumor progression in HCC, and the enrichment of exhausted CD8+T is negatively correlated with the response to immunotherapy and prognosis in patients in patients with HCC (181). It has been demonstrated that dysfunction of CD8+ T cells occurs within a few hours after they encounter tumor antigens, even before undergoing cell division T (182). This rapid divergence of T cell fate prior to cell division provides us a clue for timely application of immunotherapy. Additionally, tissue-resident memory CD8+ T (TRM) cells are also enriched in tumors, especially in HBV-related HCC (183). A high TRM proportion is associated with better outcomes following ICI therapy (181, 184).
Under the co-stimulation of activated APCs and different cytokines, Naïve CD4+ T cells proliferate and differentiate into different subsets, including Th cells (specifically, Th1, Th2, and Th17), follicular helper T (Tfh) cells, and Treg cells (185). Among them, Th1 cells secret IFN-γ and IL-2 and promote the anti-tumor effect of CD8+ T cells (186). Furthermore, Th1 cells facilitate dendritic cell (DC) maturation through the CD40-CD40L signaling axis (187). On the other hand, Th17 cells are abundant in HCC and are associated with unfavorable clinical outcomes (188). Moreover, Th17 cells contribute to resistance against PD-L1 therapy by upregulating PD-L1 expression in HCC cells through the secretion of IL-17A (189). Treg cells are significantly increased in HCC and are correlated with dysfunction of CD8 T-cells, reduced clinical benefits of anti-PD-L1 plus anti-VEGFR, and poor survival (190–192). Given the crucial role of Tregs in maintaining immune homeostasis and preventing auto-immune diseases, there is a pressing need for innovative approaches that precisely target tumor-infiltrating Tregs and spare the physiological function of Tregs. Currently, little is known about the roles of Th2 and Tfh cells in HCC, which require further investigation. Both exhausted CD8+T and Treg cells are characterized by upregulated expression of a series of inhibitory receptors, such as PD-1 and CTLA-4 (193, 194). In pre-tumoral HCC tissues, monocytes express higher levels of CD93, which inhibit the infiltration of CD8 T cells (195). Thus, targeting CD93-expressing monocytes can help increase the activation and infiltration of the CD8 T cells. Multi-omics is commonly used to identify the T-cells in tumors, but standard AI-based systems for efficiently detecting T-cells in liver TME are not available. Questions regarding advanced AI intervention in the detection of T cells in solid tumors, especially liver cancer, remain unclear.
4.2.5 B cells
Tumor-infiltrating B cells play pivotal roles in tumor immunity, including antigen presentation, antibody production, and other functions (196). Their presence is notably a favorable marker for HCC prognosis (94, 197). Additionally, the presence of intra-tumoral tertiary lymphoid structures (TLS) is correlated with a reduced risk of early recurrence in HCC patients after surgical resection (198). Within TLS, abundant B cells transform into plasma cells and produce IgG antibodies that effectively combat tumors (199). However, there are some subtypes of B cells that play pro-tumor roles in HCC. IgA-producing B cells enhanced the expression of PD-L1 and exert an inhibitory influence on T-cell activation (200). Furthermore, regulatory B cells (Bregs), which are characterized by IL-10 secretion, not only dampen T-cell responsiveness but also contribute to HCC progression via the CD40/CD154 signaling axis (201). Given the intricate and diverse functions exhibited by various B-cell subsets in HCC, further research endeavors are required to unlock their full potential in therapeutic strategies. As aforementioned, single-cell sequencing is commonly used to identify the cellular composition of tumors. The level of B cells in liver tumors was detected using conventional sc-seq (202), but AI-guided sc-seq is not generally applied.
4.2.6 NK cells and other innate lymphoid cells
Innate lymphoid cells (ILCs) are a highly heterogeneous family, which comprise NK cells, ILCs also include ILC1s, ILC2s, and ILC3s. In the human liver, NK cells constitute a prominent subtype of lymphocyte, accounting for about 50% of the total intrahepatic lymphocytes (203). These NK cells can be categorically split into two distinct subsets: cytotoxic NK cells marked by CD56dimCD16high expression and immunoregulatory NK cells characterized by CD56brightCD16low expression (204). Cytokines, such as IL-10 and TGF-β, induce the exhaustion phenotype of CD11b-/CD27-NK cells through the upregulation of NKG2A and CD96, respectively. The blockade of IL-10 or TGF-β pathway can reverse the dysfunction of NK cells (205, 206). Furthermore, a significant reduction of NAD+ in NK cells causes their dysfunction. Supplementation with NMN, a NAD+ precursor, restores the anti-tumor effects of NK cells (207). Due to its potent cytotoxicity against tumors without dependence on secondary activation, various innovative NK cell-based therapeutic strategies have been explored in HCC (208). For example, bispecific antibodies are designed to bridge the gap between NK cells and tumor cells (209–211). These antibodies possess dual specificity, enabling them to simultaneously bind to a tumor-associated antigen on HCC cells and an activating receptor on NK cells. This interaction triggers potent antibody-dependent cellular cytotoxicity (ADCC), NK cells are activated and directed toward the tumor, leading to their direct killing via the release of cytotoxic granules containing perforin and granzymes. Furthermore, adoptive cell transfer (ACT) therapies have emerged as a promising avenue for NK cell-based treatments. NK cells are either expanded ex vivo or subsequently modified to enhance their tumor-targeting and killing capabilities. These modifications can be achieved through the engineering of chimeric antigen receptors (CARs) into NK cells (212) or the activating killing-ability of NK cells with cytokines (213).
Not like the cytolytic NK cells, ILC1s, ILC2s, and ILC3s function through cytokine secretion. Interestingly, the secretion behaviors of ILC1s, ILC2s, and ILC3s mirror the functions and characteristics of CD4+ helper T cell subsets. The three ILC subtypes secrete IFN-γ/TNF-α, IL-4/IL-5/IL-13, and IL-17/IL-22, which are signatures of characteristics of Th1, Th2, and Th17 cells, respectively (204, 214). Currently, their roles in liver cancer are still controversial. For example, ICOS+ILC2a cells were enriched in HCC and associated with poor prognosis (215). However, in another study, a high ILC2/ILC1 ratio is associated with enhanced anti-tumor immune responses and better prognosis (216). Further studies are needed to define the contribution of these cytokine-secretion ILCs in HCC.
5 Challenges and Future Prospects
In the past several years, a drastic rise in data digitization has been seen in many sectors, including the medical sector. However, it comes with challenges, especially in acquiring and scrutinizing suitable data to solve various complex problems. LC-IME is highly heterogeneous and complex, and so far, no AI system has been constructed to identify various cell types and signaling pathways (217, 218). The cellular composition of the LC-IME is usually determined by conventional single-cell sequencing; AI intervention can improve the overall outcome. With enhanced automation, AI has the power to handle large-scale data because AI-guided tools can learn from input data and independently draw conclusions according to the given objectives. On the other hand, conventional methods require stepwise monitoring and human input for manual analysis and drawing conclusions. However, several limitations still exist for freely applying AI in LC-IME. First, there is a long way to go in building highly accurate AI algorithms and models that are both explainable and trustworthy. Further integration of constraints into the models, based on biological domain knowledge in a principled manner, is necessary to improve both the accuracy and interpretability of models being applied to LC-IME. Second, the reliability of most studies is limited by small sample sizes. Future rigorous, large-scale longitudinal studies on LC-IME are needed for feature decomposition and to reduce the large number of variables. Third, research growth in this area is hindered by the lack of international guidelines or models that specify where AI is more likely to be useful in monitoring the integration of large data. There is a need for transparent, accessible, and curated data sharing. Moreover, interdisciplinary approaches, supplemented by rigorous co-production and co-design processes alongside individuals with liver cancer, are key to progress in this area. These research directions are expected to drive the in-depth application of AI technology in LC-IME field, thereby providing new solutions for precision medicine of liver cancer and significantly improving treatment outcomes and quality of life for patients.
6 Conclusion
With the rapid development of high-throughput sequencing technology and computer science, the amount of large omics data has increased exponentially, the advantages of multi-omics analysis have gradually emerged, and the application of artificial intelligence has become more and more extensive. Overall, this review has highlighted the potential, current applications, and implementation framework for integrating AI in the discovery and validation of biomarkers in HCC. Finally, we briefly explained the current challenges of multi-omics analysis and artificial intelligence in order to provide new research ideas for the medical industry and to promote the development and application of precision medicine.
Author contributions
XC: Writing – original draft, Writing – review & editing, Conceptualization. IB: Writing – review & editing. ML: Writing – review & editing. GL: Methodology, Visualization, Writing – review & editing. JX: Writing – review & editing. PZ: Supervision, Writing – review & editing.
Funding
The author(s) declare financial support was received for the research, authorship, and/or publication of this article. This project was supported by Research Fund for the Doctoral program of the Fifth Affiliated Hospital of Zhengzhou University (pb2024kyqdj03), Supporting Program for Young Talent Innovation Teams of Zhengzhou University (32320688), Henan Medical Key Technologies R & D Program (LHGJ20220570).
Acknowledgments
We are deeply grateful to Xinfeng Liang for her crucial management and coordination in the planning and execution of the research activity. Her efforts have ensured the smooth progress and successful outcome of our endeavors.
Conflict of interest
The authors declare that the research was conducted in the absence of any commercial or financial relationships that could be construed as a potential conflict of interest.
Generative AI statement
The author(s) declare that no Generative AI was used in the creation of this manuscript.
Publisher’s note
All claims expressed in this article are solely those of the authors and do not necessarily represent those of their affiliated organizations, or those of the publisher, the editors and the reviewers. Any product that may be evaluated in this article, or claim that may be made by its manufacturer, is not guaranteed or endorsed by the publisher.
Abbreviations
CSF1/CSF1R, Colony Stimulating Factor 1/Colony Stimulating Factor 1 Receptor; PD-L1, programmed death-ligand 1; TIM-3, T cell immunoglobulin and mucin domain-3; IGF-1, insulin-like growth factor-1; CCL20, C-C motif ligand 20; IFN-γ, interferon-γ; NAD+, nicotinamide adenine dinucleotide; NMN, nicotinamide mononucleotide; ICOS, T-cell co-stimulator; GM-CSF, colony-stimulating factor; PGE2, prostaglandin E2; TOX, thymocyte selection associated high mobility group box; CTLA-4, cytotoxic T-lymphocyte antigen 4; LAG3, lymphocyte activation gene 3.
References
1. Bray F, Laversanne M, Sung H, Ferlay J, Siegel RL, Soerjomataram I, et al. Global cancer statistics 2022: GLOBOCAN estimates of incidence and mortality worldwide for 36 cancers in 185 countries. CA Cancer J Clin. (2024) 74:229–63. doi: 10.3322/caac.21834
2. Jianyong L, Lunan Y, Dajiang L, Wentao W, Liver Surgery G. Comparison of open liver resection and RFA for the treatment of solitary 3-5-cmhepatocellular carcinoma: a retrospective study. BMC Surg. (2019) 19:195. doi: 10.1186/s12893-019-0663-9
3. Sugawara Y, Hibi T. Surgical treatment of hepatocellular carcinoma. Biosci Trends. (2021) 15:138–41. doi: 10.5582/bst.2021.01094
4. Cassinotto C, Nogue E, Durand Q, Panaro F, Assenat E, Dohan A, et al. Life expectancy of patients with hepatocellular carcinoma according to the upfront treatment: A nationwide analysis. Diagn Interv Imaging. (2023) 104:192–9. doi: 10.1016/j.diii.2023.01.002
5. Lee MW, Kang D, Lim HK, Cho J, Sinn DH, Kang TW, et al. Updated 10-year outcomes of percutaneous radiofrequency ablation as first-line therapy for single hepatocellular carcinoma < 3 cm: emphasis on association of local tumor progression and overall survival. Eur Radiol. (2020) 30:2391–400. doi: 10.1007/s00330-019-06575-0
6. National Clinical Research Center for Infectious D, Society of Hepatology BMA, Translational Medicine Branch CAoG, Geriatrics. Expert consensus on precise diagnosis and management of primary hepatocellular carcinoma in advanced stage (2023 version). Zhonghua Gan Zang Bing Za Zhi. (2023) 31:910–20. doi: 10.3760/cma.j.cn501113-20230717-00006
7. Brown ZJ, Greten TF, Heinrich B. Adjuvant treatment of hepatocellular carcinoma: prospect of immunotherapy. Hepatology. (2019) 70:1437–42. doi: 10.1002/hep.30633
11. Llovet JM, Kelley RK, Villanueva A, Singal AG, Pikarsky E, Roayaie S, et al. Hepatocellular carcinoma. Nat Rev Dis Primers. (2021) 7:6. doi: 10.1038/s41572-020-00240-3
12. Pashkov VM, Harkusha AO, Soloviov OS. The impact of the introduction of artificial intelligence technologies on the current human rights and freedoms concept. Pol Merkur Lekarski. (2023) 51:646–53. doi: 10.36740/Merkur
13. Hou Y, Dong Q, Wang D, Liu J. Introduction to ‘Artificial intelligence in failure analysis of transportation infrastructure and materials’. Philos Trans A Math Phys Eng Sci. (2023) 381:20220177. doi: 10.1098/rsta.2022.0177
14. Bundy A, Chater N, Muggleton S. Introduction to ‘Cognitive artificial intelligence’. Philos Trans A Math Phys Eng Sci. (2023) 381:20220051. doi: 10.1098/rsta.2022.0051
15. Xiao F, Ke J. Pricing, management and decision-making of financial markets with artificial intelligence: introduction to the issue. Financ Innov. (2021) 7:85. doi: 10.1186/s40854-021-00302-9
16. Li L, Sun M, Wang J, Wan S. Multi-omics based artificial intelligence for cancer research. Adv Cancer Res. (2024) 163:303–56. doi: 10.1016/bs.acr.2024.06.005
17. Wang F, Preininger A. AI in health: state of the art, challenges, and future directions. Yearb Med Inform. (2019) 28:16–26. doi: 10.1055/s-0039-1677908
18. Mintz Y, Brodie R. Introduction to artificial intelligence in medicine. Minim Invasive Ther Allied Technol. (2019) 28:73–81. doi: 10.1080/13645706.2019.1575882
19. Sung JJY. Introduction to artificial intelligence in medicine. Singapore Med J. (2024) 65:132. doi: 10.4103/singaporemedj.SMJ-2024-060
20. Sarker IH. Machine learning: algorithms, real-world applications and research directions. SN Comput Sci. (2021) 2:160. doi: 10.1007/s42979-021-00592-x
21. Jiang T, Gradus JL, Rosellini AJ. Supervised machine learning: A brief primer. Behav Ther. (2020) 51:675–87. doi: 10.1016/j.beth.2020.05.002
22. Eckhardt CM, Madjarova SJ, Williams RJ, Ollivier M, Karlsson J, Pareek A, et al. Unsupervised machine learning methods and emerging applications in healthcare. Knee Surg Sports Traumatol Arthrosc. (2023) 31:376–81. doi: 10.1007/s00167-022-07233-7
23. Valkenborg D, Rousseau AJ, Geubbelmans M, Burzykowski T. Unsupervised learning. Am J Orthod Dentofacial Orthop. (2023) 163:877–82. doi: 10.1016/j.ajodo.2023.04.001
24. Reddy YCAP, Viswanath P, Eswara Reddy B. Semi-supervised learning: A brief review. Int J Eng Technol. (2018) 7:81. doi: 10.14419/ijet.v7i1.8.9977
25. Datta S, Li Y, Ruppert MM, Ren Y, Shickel B, Ozrazgat-Baslanti T, et al. Reinforcement learning in surgery. Surgery. (2021) 170:329–32. doi: 10.1016/j.surg.2020.11.040
27. Klein EA, Richards D, Cohn A, Tummala M, Lapham R, Cosgrove D, et al. Clinical validation of a targeted methylation-based multi-cancer early detection test using an independent validation set. Ann Oncol. (2021) 32:1167–77. doi: 10.1016/j.annonc.2021.05.806
28. Stark GF, Hart GR, Nartowt BJ, Deng J. Predicting breast cancer risk using personal health data and machine learning models. PloS One. (2019) 14:e0226765. doi: 10.1371/journal.pone.0226765
29. Feng X, Hong T, Liu W, Xu C, Li W, Yang B, et al. Development and validation of a machine learning model to predict the risk of lymph node metastasis in renal carcinoma. Front Endocrinol (Lausanne). (2022) 13:1054358. doi: 10.3389/fendo.2022.1054358
30. Rodland KD, Webb-Robertson BJ, Srivastava S. Introduction to the special issue on Applications of Artificial Intelligence in Biomarker Research. Cancer biomark. (2022) 33:171–2. doi: 10.3233/CBM-229001
31. Halner A, Hankey L, Liang Z, Pozzetti F, Szulc D, Mi E, et al. DEcancer: Machine learning framework tailored to liquid biopsy based cancer detection and biomarker signature selection. iScience. (2023) 26:106610. doi: 10.1016/j.isci.2023.106610
32. Chen Y, Wang B, Zhao Y, Shao X, Wang M, Ma F, et al. Metabolomic machine learning predictor for diagnosis and prognosis of gastric cancer. Nat Commun. (2024) 15:1657. doi: 10.1038/s41467-024-46043-y
33. Tayob N, Feng Z. Personalized statistical learning algorithms to improve the early detection of cancer using longitudinal biomarkers. Cancer biomark. (2022) 33:199–210. doi: 10.3233/CBM-210307
34. Stefanou IK, Dovrolis N, Gazouli M, Theodorou D, Zografos GK, Toutouzas KG. miRNAs expression pattern and machine learning models elucidate risk for gastric GIST. Cancer biomark. (2022) 33:237–47. doi: 10.3233/CBM-210173
35. Starikov A, Al’Aref SJ, Singh G, Min JK. Artificial intelligence in clinical imaging: An introduction. Clin Imaging. (2018) 49:vii–ix. doi: 10.1016/j.clinimag.2018.04.001
36. Lee JG, Jun S, Cho YW, Lee H, Kim GB, Seo JB, et al. Deep learning in medical imaging: general overview. Korean J Radiol. (2017) 18:570–84. doi: 10.3348/kjr.2017.18.4.570
37. Kourounis G, Elmahmudi AA, Thomson B, Hunter J, Ugail H, Wilson C. Computer image analysis with artificial intelligence: a practical introduction to convolutional neural networks for medical professionals. Postgrad Med J. (2023) 99:1287–94. doi: 10.1093/postmj/qgad095
38. Al-Masni MA, Al-Antari MA, Park JM, Gi G, Kim TY, Rivera P, et al. Detection and classification of the breast abnormalities in digital mammograms via regional Convolutional Neural Network. Annu Int Conf IEEE Eng Med Biol Soc. (2017) 2017:1230–3. doi: 10.1109/EMBC.2017.8037053
39. Zhao X, Xie P, Wang M, Li W, Pickhardt PJ, Xia W, et al. Deep learning-based fully automated detection and segmentation of lymph nodes on multiparametric-mri for rectal cancer: A multicentre study. EBioMedicine. (2020) 56:102780. doi: 10.1016/j.ebiom.2020.102780
40. Jin EH, Lee D, Bae JH, Kang HY, Kwak MS, Seo JY, et al. Improved accuracy in optical diagnosis of colorectal polyps using convolutional neural networks with visual explanations. Gastroenterology. (2020) 158:2169–79 e8. doi: 10.1053/j.gastro.2020.02.036
41. Ramon YCS, Sese M, Capdevila C, Aasen T, De Mattos-Arruda L, Diaz-Cano SJ, et al. Clinical implications of intratumor heterogeneity: challenges and opportunities. J Mol Med (Berl). (2020) 98:161–77. doi: 10.1007/s00109-020-01874-2
42. Marusyk A, Janiszewska M, Polyak K. Intratumor heterogeneity: the rosetta stone of therapy resistance. Cancer Cell. (2020) 37:471–84. doi: 10.1016/j.ccell.2020.03.007
43. Shmatko A, Ghaffari Laleh N, Gerstung M, Kather JN. Artificial intelligence in histopathology: enhancing cancer research and clinical oncology. Nat Cancer. (2022) 3:1026–38. doi: 10.1038/s43018-022-00436-4
44. Lu MY, Chen TY, Williamson DFK, Zhao M, Shady M, Lipkova J, et al. AI-based pathology predicts origins for cancers of unknown primary. Nature. (2021) 594:106–10. doi: 10.1038/s41586-021-03512-4
45. Lee Y, Park JH, Oh S, Shin K, Sun J, Jung M, et al. Derivation of prognostic contextual histopathological features from whole-slide images of tumours via graph deep learning. Nat BioMed Eng. (2022). doi: 10.1038/s41551-022-00923-0
46. Pei Q, Luo Y, Chen Y, Li J, Xie D, Ye T. Artificial intelligence in clinical applications for lung cancer: diagnosis, treatment and prognosis. Clin Chem Lab Med. (2022) 60:1974–83. doi: 10.1515/cclm-2022-0291
47. Mitsala A, Tsalikidis C, Pitiakoudis M, Simopoulos C, Tsaroucha AK. Artificial intelligence in colorectal cancer screening, diagnosis and treatment. A New Era Curr Oncol. (2021) 28:1581–607. doi: 10.3390/curroncol28030149
48. Luo S, Xu J, Jiang Z, Liu L, Wu Q, Leung EL, et al. Artificial intelligence-based collaborative filtering method with ensemble learning for personalized lung cancer medicine without genetic sequencing. Pharmacol Res. (2020) 160:105037. doi: 10.1016/j.phrs.2020.105037
49. Abajian A, Murali N, Savic LJ, Laage-Gaupp FM, Nezami N, Duncan JS, et al. Predicting treatment response to intra-arterial therapies for hepatocellular carcinoma with the use of supervised machine learning-an artificial intelligence concept. J Vasc Interv Radiol. (2018) 29:850–7 e1. doi: 10.1016/j.jvir.2018.01.769
50. Kong J, Ha D, Lee J, Kim I, Park M, Im SH, et al. Network-based machine learning approach to predict immunotherapy response in cancer patients. Nat Commun. (2022) 13:3703. doi: 10.1038/s41467-022-31535-6
51. Levy EI, Taussky P, Cohen JE, Kan P. Introduction. Neurosurgical management of stroke, organization of stroke management, and artificial intelligence applications. Neurosurg Focus. (2021) 51:E1. doi: 10.3171/2021.4.FOCUS21264
52. Qiu B, Shen Z, Wu S, Qin X, Yang D, Wang Q. A machine learning-based model for predicting distant metastasis in patients with rectal cancer. Front Oncol. (2023) 13:1235121. doi: 10.3389/fonc.2023.1235121
53. Cai WL, Cheng M, Wang Y, Xu PH, Yang X, Sun ZW, et al. Prediction and related genes of cancer distant metastasis based on deep learning. Comput Biol Med. (2024) 168:107664. doi: 10.1016/j.compbiomed.2023.107664
54. Lu ZX, Qian P, Bi D, Ye ZW, He X, Zhao YH, et al. Application of AI and ioT in clinical medicine: summary and challenges. Curr Med Sci. (2021) 41:1134–50. doi: 10.1007/s11596-021-2486-z
55. Sarkar C, Das B, Rawat VS, Wahlang JB, Nongpiur A, Tiewsoh I, et al. Artificial intelligence and machine learning technology driven modern drug discovery and development. Int J Mol Sci. (2023) 24:2026. doi: 10.3390/ijms24032026
56. Qiu X, Li H, Ver Steeg G, Godzik A. Advances in AI for protein structure prediction: implications for cancer drug discovery and development. Biomolecules. (2024) 14:339. doi: 10.3390/biom14030339
57. Zhou X, Wang M, Katsyv I, Irie H, Zhang B. EMUDRA: Ensemble of Multiple Drug Repositioning Approaches to improve prediction accuracy. Bioinformatics. (2018) 34:3151–9. doi: 10.1093/bioinformatics/bty325
58. Jenne CN, Kubes P. Immune surveillance by the liver. Nat Immunol. (2013) 14:996–1006. doi: 10.1038/ni.2691
59. Zheng M, Tian Z. Liver-mediated adaptive immune tolerance. Front Immunol. (2019) 10:2525. doi: 10.3389/fimmu.2019.02525
60. Schramm C, Oo YH, Lohse AW. Tolerance and autoimmunity in the liver. Semin Immunopathol. (2022) 44:393–5. doi: 10.1007/s00281-022-00952-6
61. Koo SY, Park EJ, Lee CW. Immunological distinctions between nonalcoholic steatohepatitis and hepatocellular carcinoma. Exp Mol Med. (2020) 52:1209–19. doi: 10.1038/s12276-020-0480-3
62. Yang Zhou J. Innate immunity and early liver inflammation. Front Immunol. (2023) 14:1175147. doi: 10.3389/fimmu.2023.1175147
63. Meyer M, Schwarzler J, Jukic A, Tilg H. Innate immunity and MASLD. Biomolecules. (2024) 14:476. doi: 10.3390/biom14040476
64. Tang J, Yan Z, Feng Q, Yu L, Wang H. The roles of neutrophils in the pathogenesis of liver diseases. Front Immunol. (2021) 12:625472. doi: 10.3389/fimmu.2021.625472
65. Kahraman A, Schlattjan M, Kocabayoglu P, Yildiz-Meziletoglu S, Schlensak M, Fingas CD, et al. Major histocompatibility complex class I-related chains A and B (MIC A/B): a novel role in nonalcoholic steatohepatitis. Hepatology. (2010) 51:92–102. doi: 10.1002/hep.23253
66. Backes CS, Friedmann KS, Mang S, Knorck A, Hoth M, Kummerow C. Natural killer cells induce distinct modes of cancer cell death: Discrimination, quantification, and modulation of apoptosis, necrosis, and mixed forms. J Biol Chem. (2018) 293:16348–63. doi: 10.1074/jbc.RA118.004549
67. Zhang Y, Wu Y, Shen W, Wang B, Yuan X. Crosstalk between NK cells and hepatic stellate cells in liver fibrosis (Review). Mol Med Rep. (2022) 25:208. doi: 10.1186/s10020-021-00415-y
68. Kumar S. Natural killer cell cytotoxicity and its regulation by inhibitory receptors. Immunology. (2018) 154:383–93. doi: 10.1111/imm.2018.154.issue-3
69. Doherty DG. Immunity, tolerance and autoimmunity in the liver: A comprehensive review. J Autoimmun. (2016) 66:60–75. doi: 10.1016/j.jaut.2015.08.020
70. Racanelli V, Rehermann B. The liver as an immunological organ. Hepatology. (2006) 43:S54–62. doi: 10.1002/hep.21060
71. Nakamoto N, Kanai T. Role of toll-like receptors in immune activation and tolerance in the liver. Front Immunol. (2014) 5:221. doi: 10.3389/fimmu.2014.00221
72. Tacke F. Targeting hepatic macrophages to treat liver diseases. J Hepatol. (2017) 66:1300–12. doi: 10.1016/j.jhep.2017.02.026
73. Bleriot C, Barreby E, Dunsmore G, Ballaire R, Chakarov S, Ficht X, et al. A subset of Kupffer cells regulates metabolism through the expression of CD36. Immunity. (2021) 54:2101–16 e6. doi: 10.1016/j.immuni.2021.08.006
74. Holt MP, Cheng L, Ju C. Identification and characterization of infiltrating macrophages in acetaminophen-induced liver injury. J Leukoc Biol. (2008) 84:1410–21. doi: 10.1189/jlb.0308173
75. Obstfeld AE, Sugaru E, Thearle M, Francisco AM, Gayet C, Ginsberg HN, et al. C-C chemokine receptor 2 (CCR2) regulates the hepatic recruitment of myeloid cells that promote obesity-induced hepatic steatosis. Diabetes. (2010) 59:916–25. doi: 10.2337/db09-1403
76. Stienstra R, Saudale F, Duval C, Keshtkar S, Groener JE, van Rooijen N, et al. Kupffer cells promote hepatic steatosis via interleukin-1beta-dependent suppression of peroxisome proliferator-activated receptor alpha activity. Hepatology. (2010) 51:511–22. doi: 10.1002/hep.23337
77. Strizova Z, Benesova I, Bartolini R, Novysedlak R, Cecrdlova E, Foley LK, et al. M1/M2 macrophages and their overlaps - myth or reality? Clin Sci (Lond). (2023) 137:1067–93. doi: 10.1042/CS20220531
78. Liu J, Geng X, Hou J, Wu G. New insights into M1/M2 macrophages: key modulators in cancer progression. Cancer Cell Int. (2021) 21:389. doi: 10.1186/s12935-021-02089-2
79. Crispe IN. Liver antigen-presenting cells. J Hepatol. (2011) 54:357–65. doi: 10.1016/j.jhep.2010.10.005
80. Poddighe D, Maulenkul T, Zhubanova G, Akhmaldtinova L, Dossybayeva K. Natural killer T (NKT) cells in autoimmune hepatitis: current evidence from basic and clinical research. Cells. (2023) 12:2854. doi: 10.3390/cells12242854
81. Gan J, Mao XR, Zheng SJ, Li JF. Invariant natural killer T cells: Not to be ignored in liver disease. J Dig Dis. (2021) 22:136–42. doi: 10.1111/1751-2980.12968
82. Arrese M, Cabrera D, Kalergis AM, Feldstein AE. Innate immunity and inflammation in NAFLD/NASH. Dig Dis Sci. (2016) 61:1294–303. doi: 10.1007/s10620-016-4049-x
83. Geissmann F, Cameron TO, Sidobre S, Manlongat N, Kronenberg M, Briskin MJ, et al. Intravascular immune surveillance by CXCR6+ NKT cells patrolling liver sinusoids. PloS Biol. (2005) 3:e113. doi: 10.1371/journal.pbio.0030113
84. Kumar V. NKT-cell subsets: promoters and protectors in inflammatory liver disease. J Hepatol. (2013) 59:618–20. doi: 10.1016/j.jhep.2013.02.032
85. Ramadori P, Kam S, Heikenwalder M. T cells: Friends and foes in NASH pathogenesis and hepatocarcinogenesis. Hepatology. (2022) 75:1038–49. doi: 10.1002/hep.32336
86. Rakhra K, Bachireddy P, Zabuawala T, Zeiser R, Xu L, Kopelman A, et al. CD4(+) T cells contribute to the remodeling of the microenvironment required for sustained tumor regression upon oncogene inactivation. Cancer Cell. (2010) 18:485–98. doi: 10.1016/j.ccr.2010.10.002
87. Kang TW, Yevsa T, Woller N, Hoenicke L, Wuestefeld T, Dauch D, et al. Senescence surveillance of pre-malignant hepatocytes limits liver cancer development. Nature. (2011) 479:547–51. doi: 10.1038/nature10599
88. Heinrich B, Brown ZJ, Diggs LP, Vormehr M, Ma C, Subramanyam V, et al. Steatohepatitis impairs T-cell-directed immunotherapies against liver tumors in mice. Gastroenterology. (2021) 160:331–45 e6. doi: 10.1053/j.gastro.2020.09.031
89. Van Herck MA, Weyler J, Kwanten WJ, Dirinck EL, De Winter BY, Francque SM, et al. The differential roles of T cells in non-alcoholic fatty liver disease and obesity. Front Immunol. (2019) 10:82. doi: 10.3389/fimmu.2019.00082
90. Hirsova P, Bamidele AO, Wang H, Povero D, Revelo XS. Emerging roles of T cells in the pathogenesis of nonalcoholic steatohepatitis and hepatocellular carcinoma. Front Endocrinol (Lausanne). (2021) 12:760860. doi: 10.3389/fendo.2021.760860
91. MacParland SA, Liu JC, Ma XZ, Innes BT, Bartczak AM, Gage BK, et al. Single cell RNA sequencing of human liver reveals distinct intrahepatic macrophage populations. Nat Commun. (2018) 9:4383. doi: 10.1038/s41467-018-06318-7
92. Togashi Y, Shitara K, Nishikawa H. Regulatory T cells in cancer immunosuppression - implications for anticancer therapy. Nat Rev Clin Oncol. (2019) 16:356–71. doi: 10.1038/s41571-019-0175-7
93. Wang H, Zhang H, Wang Y, Brown ZJ, Xia Y, Huang Z, et al. Regulatory T-cell and neutrophil extracellular trap interaction contributes to carcinogenesis in non-alcoholic steatohepatitis. J Hepatol. (2021) 75:1271–83. doi: 10.1016/j.jhep.2021.07.032
94. Garnelo M, Tan A, Her Z, Yeong J, Lim CJ, Chen J, et al. Interaction between tumour-infiltrating B cells and T cells controls the progression of hepatocellular carcinoma. Gut. (2017) 66:342–51. doi: 10.1136/gutjnl-2015-310814
95. Cheng K, Cai N, Zhu J, Yang X, Liang H, Zhang W. Tumor-associated macrophages in liver cancer: From mechanisms to therapy. Cancer Commun (Lond). (2022) 42:1112–40. doi: 10.1002/cac2.v42.11
96. Sangro B, Sarobe P, Hervas-Stubbs S, Melero I. Advances in immunotherapy for hepatocellular carcinoma. Nat Rev Gastroenterol Hepatol. (2021) 18:525–43. doi: 10.1038/s41575-021-00438-0
97. Zheng C, Zheng L, Yoo JK, Guo H, Zhang Y, Guo X, et al. Landscape of infiltrating T cells in liver cancer revealed by single-cell sequencing. Cell. (2017) 169:1342–56 e16. doi: 10.1016/j.cell.2017.05.035
98. Zhang Q, He Y, Luo N, Patel SJ, Han Y, Gao R, et al. Landscape and dynamics of single immune cells in hepatocellular carcinoma. Cell. (2019) 179:829–45 e20. doi: 10.1016/j.cell.2019.10.003
99. Llovet JM, Castet F, Heikenwalder M, Maini MK, Mazzaferro V, Pinato DJ, et al. Immunotherapies for hepatocellular carcinoma. Nat Rev Clin Oncol. (2022) 19:151–72. doi: 10.1038/s41571-021-00573-2
100. Harkus U, Wankell M, Palamuthusingam P, McFarlane C, Hebbard L. Immune checkpoint inhibitors in HCC: Cellular, molecular and systemic data. Semin Cancer Biol. (2022) 86:799–815. doi: 10.1016/j.semcancer.2022.01.005
101. Binnewies M, Roberts EW, Kersten K, Chan V, Fearon DF, Merad M, et al. Understanding the tumor immune microenvironment (TIME) for effective therapy. Nat Med. (2018) 24:541–50. doi: 10.1038/s41591-018-0014-x
102. Thorsson V, Gibbs DL, Brown SD, Wolf D, Bortone DS, Ou Yang TH, et al. The immune landscape of cancer. Immunity. (2018) 48:812–30 e14. doi: 10.1016/j.immuni.2018.03.023
103. Li X, Ramadori P, Pfister D, Seehawer M, Zender L, Heikenwalder M. The immunological and metabolic landscape in primary and metastatic liver cancer. Nat Rev Cancer. (2021) 21:541–57. doi: 10.1038/s41568-021-00383-9
104. Cheng C, Chen W, Jin H, Chen X. A review of single-cell RNA-seq annotation, integration, and cell-cell communication. Cells. (2023) 12:1970. doi: 10.3390/cells12151970
105. Lu J, Sheng Y, Qian W, Pan M, Zhao X, Ge Q. scRNA-seq data analysis method to improve analysis performance. IET Nanobiotechnol. (2023) 17:246–56. doi: 10.1049/nbt2.12115
106. Brendel M, Su C, Bai Z, Zhang H, Elemento O, Wang F. Application of deep learning on single-cell RNA sequencing data analysis: A review. Genomics Proteomics Bioinf. (2022) 20:814–35. doi: 10.1016/j.gpb.2022.11.011
107. Ryu Y, Han GH, Jung E, Hwang D. Integration of single-cell RNA-seq datasets: A review of computational methods. Mol Cells. (2023) 46:106–19. doi: 10.14348/molcells.2023.0009
108. Madadi Y, Monavarfeshani A, Chen H, Stamer WD, Williams RW, Yousefi S. Artificial intelligence models for cell type and subtype identification based on single-cell RNA sequencing data in vision science. IEEE/ACM Trans Comput Biol Bioinform. (2023) 20:2837–52. doi: 10.1109/TCBB.2023.3284795
109. Park S, Lee H. Robust self-supervised learning strategy to tackle the inherent sparsity in single-cell RNA-seq data. Brief Bioinform. (2024) 25:bbae586. doi: 10.1093/bib/bbae586
110. Murphy AE, Beardall W, Rei M, Phuycharoen M, Skene NG. Predicting cell type-specific epigenomic profiles accounting for distal genetic effects. Nat Commun. (2024) 15:9951. doi: 10.1038/s41467-024-54441-5
111. Consortium GT. Human genomics. The Genotype-Tissue Expression (GTEx) pilot analysis: multitissue gene regulation in humans. Science. (2015) 348:648–60. doi: 10.1126/science.1262110
112. Wellcome Trust Case Control C. Genome-wide association study of 14,000 cases of seven common diseases and 3,000 shared controls. Nature. (2007) 447:661–78. doi: 10.1038/nature05911
113. Roadmap Epigenomics C, Kundaje A, Meuleman W, Ernst J, Bilenky M, Yen A, et al. Integrative analysis of 111 reference human epigenomes. Nature. (2015) 518:317–30. doi: 10.1038/nature14248
114. Ziller MJ, Gu H, Muller F, Donaghey J, Tsai LT, Kohlbacher O, et al. Charting a dynamic DNA methylation landscape of the human genome. Nature. (2013) 500:477–81. doi: 10.1038/nature12433
115. Shin SY, Fauman EB, Petersen AK, Krumsiek J, Santos R, Huang J, et al. An atlas of genetic influences on human blood metabolites. Nat Genet. (2014) 46:543–50. doi: 10.1038/ng.2982
116. Trapnell C, Roberts A, Goff L, Pertea G, Kim D, Kelley DR, et al. Differential gene and transcript expression analysis of RNA-seq experiments with TopHat and Cufflinks. Nat Protoc. (2012) 7:562–78. doi: 10.1038/nprot.2012.016
117. Kim MS, Pinto SM, Getnet D, Nirujogi RS, Manda SS, Chaerkady R, et al. A draft map of the human proteome. Nature. (2014) 509:575–81. doi: 10.1038/nature13302
118. Ivanisevic T, Sewduth RN. Multi-omics integration for the design of novel therapies and the identification of novel biomarkers. Proteomes. (2023) 11:34. doi: 10.3390/proteomes11040034
119. Lapuente-Santana O, van Genderen M, Hilbers PAJ, Finotello F, Eduati F. Interpretable systems biomarkers predict response to immune-checkpoint inhibitors. Patterns (N Y). (2021) 2:100293. doi: 10.1016/j.patter.2021.100293
120. Hasin Y, Seldin M, Lusis A. Multi-omics approaches to disease. Genome Biol. (2017) 18:83. doi: 10.1186/s13059-017-1215-1
121. Scarpa JR, Elemento O. Multi-omic molecular profiling and network biology for precision anaesthesiology: a narrative review. Br J Anaesth. (2023) 131:26–36. doi: 10.1016/j.bja.2023.03.006
122. Sun YV, Hu YJ. Integrative analysis of multi-omics data for discovery and functional studies of complex human diseases. Adv Genet. (2016) 93:147–90. doi: 10.1016/bs.adgen.2015.11.004
123. Subramanian I, Verma S, Kumar S, Jere A, Anamika K. Multi-omics data integration, interpretation, and its application. Bioinform Biol Insights. (2020) 14:1177932219899051. doi: 10.1177/1177932219899051
124. Rautenstrauch P, Vlot AHC, Saran S, Ohler U. Intricacies of single-cell multi-omics data integration. Trends Genet. (2022) 38:128–39. doi: 10.1016/j.tig.2021.08.012
125. Das T, Andrieux G, Ahmed M, Chakraborty S. Integration of online omics-data resources for cancer research. Front Genet. (2020) 11:578345. doi: 10.3389/fgene.2020.578345
126. Acosta JN, Falcone GJ, Rajpurkar P, Topol EJ. Multimodal biomedical AI. Nat Med. (2022) 28:1773–84. doi: 10.1038/s41591-022-01981-2
127. Cai Z, Poulos RC, Liu J, Zhong Q. Machine learning for multi-omics data integration in cancer. iScience. (2022) 25:103798. doi: 10.1016/j.isci.2022.103798
128. Wu X, Li W, Tu H. Big data and artificial intelligence in cancer research. Trends Cancer. (2024) 10:147–60. doi: 10.1016/j.trecan.2023.10.006
129. Haas R, Zelezniak A, Iacovacci J, Kamrad S, Townsend S, Ralser M. Designing and interpreting ‘multi-omic’ experiments that may change our understanding of biology. Curr Opin Syst Biol. (2017) 6:37–45. doi: 10.1016/j.coisb.2017.08.009
130. Wang XY, Qu HZ, Fang XD. Omics big data and medical artificial intelligence. Yi Chuan. (2021) 43:930–7. doi: 10.16288/j.yczz.21-215
131. Xu Y, Liu X, Cao X, Huang C, Liu E, Qian S, et al. Artificial intelligence: A powerful paradigm for scientific research. Innovation (Camb). (2021) 2:100179. doi: 10.1016/j.xinn.2021.100179
132. Glass DR, Tsai AG, Oliveria JP, Hartmann FJ, Kimmey SC, Calderon AA, et al. An integrated multi-omic single-cell atlas of human B cell identity. Immunity. (2020) 53:217–32 e5. doi: 10.1016/j.immuni.2020.06.013
133. Mann M, Kumar C, Zeng WF, Strauss MT. Artificial intelligence for proteomics and biomarker discovery. Cell Syst. (2021) 12:759–70. doi: 10.1016/j.cels.2021.06.006
134. Adeoye J, Su YX. Artificial intelligence in salivary biomarker discovery and validation for oral diseases. Oral Dis. (2024) 30:23–37. doi: 10.1111/odi.14641
135. He X, Liu X, Zuo F, Shi H, Jing J. Artificial intelligence-based multi-omics analysis fuels cancer precision medicine. Semin Cancer Biol. (2023) 88:187–200. doi: 10.1016/j.semcancer.2022.12.009
136. Swanson K, Wu E, Zhang A, Alizadeh AA, Zou J. From patterns to patients: Advances in clinical machine learning for cancer diagnosis, prognosis, and treatment. Cell. (2023) 186:1772–91. doi: 10.1016/j.cell.2023.01.035
137. Fridley BL, Lund S, Jenkins GD, Wang L. A Bayesian integrative genomic model for pathway analysis of complex traits. Genet Epidemiol. (2012) 36:352–9. doi: 10.1002/gepi.2012.36.issue-4
138. Mankoo PK, Shen R, Schultz N, Levine DA, Sander C. Time to recurrence and survival in serous ovarian tumors predicted from integrated genomic profiles. PloS One. (2011) 6:e24709. doi: 10.1371/journal.pone.0024709
139. Shen R, Mo Q, Schultz N, Seshan VE, Olshen AB, Huse J, et al. Integrative subtype discovery in glioblastoma using iCluster. PloS One. (2012) 7:e35236. doi: 10.1371/journal.pone.0035236
140. Holzinger ER, Dudek SM, Frase AT, Pendergrass SA, Ritchie MD. ATHENA: the analysis tool for heritable and environmental network associations. Bioinformatics. (2014) 30:698–705. doi: 10.1093/bioinformatics/btt572
141. Kim S, Yeganova L, Comeau DC, Wilbur WJ, Lu Z. PubMed Phrases, an open set of coherent phrases for searching biomedical literature. Sci Data. (2018) 5:180104. doi: 10.1038/sdata.2018.104
142. Turner SD, Dudek SM, Ritchie MD. ATHENA: A knowledge-based hybrid backpropagation-grammatical evolution neural network algorithm for discovering epistasis among quantitative trait Loci. BioData Min. (2010) 3:5. doi: 10.1186/1756-0381-3-5
143. Wang B, Mezlini AM, Demir F, Fiume M, Tu Z, Brudno M, et al. Similarity network fusion for aggregating data types on a genomic scale. Nat Methods. (2014) 11:333–7. doi: 10.1038/nmeth.2810
144. Draghici S, Potter RB. Predicting HIV drug resistance with neural networks. Bioinformatics. (2003) 19:98–107. doi: 10.1093/bioinformatics/19.1.98
145. Zhou SL, Yin D, Hu ZQ, Luo CB, Zhou ZJ, Xin HY, et al. A positive feedback loop between cancer stem-like cells and tumor-associated neutrophils controls hepatocellular carcinoma progression. Hepatology. (2019) 70:1214–30. doi: 10.1002/hep.30630
146. Yu SJ, Ma C, Heinrich B, Brown ZJ, Sandhu M, Zhang Q, et al. Targeting the crosstalk between cytokine-induced killer cells and myeloid-derived suppressor cells in hepatocellular carcinoma. J Hepatol. (2019) 70:449–57. doi: 10.1016/j.jhep.2018.10.040
147. Geh D, Leslie J, Rumney R, Reeves HL, Bird TG, Mann DA. Neutrophils as potential therapeutic targets in hepatocellular carcinoma. Nat Rev Gastroenterol Hepatol. (2022) 19:257–73. doi: 10.1038/s41575-021-00568-5
148. Wong L, Bozhilov K, Hernandez B, Kwee S, Chan O, Ellis L, et al. Underlying liver disease and advanced stage liver cancer are associated with elevated neutrophil-lymphocyte ratio. Clin Mol Hepatol. (2019) 25:305–16. doi: 10.3350/cmh.2019.0004
149. Yang L, Liu Q, Zhang X, Liu X, Zhou B, Chen J, et al. DNA of neutrophil extracellular traps promotes cancer metastasis via CCDC25. Nature. (2020) 583:133–8. doi: 10.1038/s41586-020-2394-6
150. Xiao Y, Cong M, Li J, He D, Wu Q, Tian P, et al. Cathepsin C promotes breast cancer lung metastasis by modulating neutrophil infiltration and neutrophil extracellular trap formation. Cancer Cell. (2021) 39:423–37 e7. doi: 10.1016/j.ccell.2020.12.012
151. van der Windt DJ, Sud V, Zhang H, Varley PR, Goswami J, Yazdani HO, et al. Neutrophil extracellular traps promote inflammation and development of hepatocellular carcinoma in nonalcoholic steatohepatitis. Hepatology. (2018) 68:1347–60. doi: 10.1002/hep.29914
152. Yang LY, Luo Q, Lu L, Zhu WW, Sun HT, Wei R, et al. Increased neutrophil extracellular traps promote metastasis potential of hepatocellular carcinoma via provoking tumorous inflammatory response. J Hematol Oncol. (2020) 13:3. doi: 10.1186/s13045-019-0836-0
153. Meng Y, Ye F, Nie P, Zhao Q, An L, Wang W, et al. Immunosuppressive CD10(+)ALPL(+) neutrophils promote resistance to anti-PD-1 therapy in HCC by mediating irreversible exhaustion of T cells. J Hepatol. (2023) 79:1435–49. doi: 10.1016/j.jhep.2023.08.024
154. Gungabeesoon J, Gort-Freitas NA, Kiss M, Bolli E, Messemaker M, Siwicki M, et al. A neutrophil response linked to tumor control in immunotherapy. Cell. (2023) 186:1448–64 e20. doi: 10.1016/j.cell.2023.02.032
155. Hirschhorn D, Budhu S, Kraehenbuehl L, Gigoux M, Schroder D, Chow A, et al. T cell immunotherapies engage neutrophils to eliminate tumor antigen escape variants. Cell. (2023) 186:1432–47 e17. doi: 10.1016/j.cell.2023.03.007
156. Xue R, Zhang Q, Cao Q, Kong R, Xiang X, Liu H, et al. Liver tumour immune microenvironment subtypes and neutrophil heterogeneity. Nature. (2022) 612:141–7. doi: 10.1038/s41586-022-05400-x
157. Wang L, Liu Y, Dai Y, Tang X, Yin T, Wang C, et al. Single-cell RNA-seq analysis reveals BHLHE40-driven pro-tumour neutrophils with hyperactivated glycolysis in pancreatic tumour microenvironment. Gut. (2023) 72:958–71. doi: 10.1136/gutjnl-2021-326070
158. Chen S, Saeed A, Liu Q, Jiang Q, Xu H, Xiao GG, et al. Macrophages in immunoregulation and therapeutics. Signal Transduct Target Ther. (2023) 8:207. doi: 10.1038/s41392-023-01452-1
159. Mantovani A, Allavena P, Marchesi F, Garlanda C. Macrophages as tools and targets in cancer therapy. Nat Rev Drug Discovery. (2022) 21:799–820. doi: 10.1038/s41573-022-00520-5
160. Li X, Yao W, Yuan Y, Chen P, Li B, Li J, et al. Targeting of tumour-infiltrating macrophages via CCL2/CCR2 signalling as a therapeutic strategy against hepatocellular carcinoma. Gut. (2017) 66:157–67. doi: 10.1136/gutjnl-2015-310514
161. Gordon SR, Maute RL, Dulken BW, Hutter G, George BM, McCracken MN, et al. PD-1 expression by tumour-associated macrophages inhibits phagocytosis and tumour immunity. Nature. (2017) 545:495–9. doi: 10.1038/nature22396
162. Lu LG, Zhou ZL, Wang XY, Liu BY, Lu JY, Liu S, et al. PD-L1 blockade liberates intrinsic antitumourigenic properties of glycolytic macrophages in hepatocellular carcinoma. Gut. (2022) 71:2551–60. doi: 10.1136/gutjnl-2021-326350
163. Sprinzl MF, Puschnik A, Schlitter AM, SChad A, Ackermann K, Esposito I, et al. Sorafenib inhibits macrophage-induced growth of hepatoma cells by interference with insulin-like growth factor-1 secretion. J Hepatol. (2015) 62:863–70. doi: 10.1016/j.jhep.2014.11.011
164. Yu J, Green MD, Li S, Sun Y, Journey SN, Choi JE, et al. Liver metastasis restrains immunotherapy efficacy via macrophage-mediated T cell elimination. Nat Med. (2021) 27:152–64. doi: 10.1038/s41591-020-1131-x
165. Zhu Y, Yang J, Xu D, Gao XM, Zhang Z, Hsu JL, et al. Disruption of tumour-associated macrophage trafficking by the osteopontin-induced colony-stimulating factor-1 signalling sensitises hepatocellular carcinoma to anti-PD-L1 blockade. Gut. (2019) 68:1653–66. doi: 10.1136/gutjnl-2019-318419
166. Giraud J, Chalopin D, Ramel E, Boyer T, Zouine A, Derieppe MA, et al. THBS1(+) myeloid cells expand in SLD hepatocellular carcinoma and contribute to immunosuppression and unfavorable prognosis through TREM1. Cell Rep. (2024) 43:113773. doi: 10.1016/j.celrep.2024.113773
167. Ho DW, Tsui YM, Chan LK, Sze KM, Zhang X, Cheu JW, et al. Single-cell RNA sequencing shows the immunosuppressive landscape and tumor heterogeneity of HBV-associated hepatocellular carcinoma. Nat Commun. (2021) 12:3684. doi: 10.1038/s41467-021-24010-1
168. Hao X, Zheng Z, Liu H, Zhang Y, Kang J, Kong X, et al. Inhibition of APOC1 promotes the transformation of M2 into M1 macrophages via the ferroptosis pathway and enhances anti-PD1 immunotherapy in hepatocellular carcinoma based on single-cell RNA sequencing. Redox Biol. (2022) 56:102463. doi: 10.1016/j.redox.2022.102463
169. Del Prete A, Salvi V, Soriani A, Laffranchi M, Sozio F, Bosisio D, et al. Dendritic cell subsets in cancer immunity and tumor antigen sensing. Cell Mol Immunol. (2023) 20:432–47. doi: 10.1038/s41423-023-00990-6
170. Suthen S, Lim CJ, Nguyen PHD, Dutertre CA, Lai HLH, Wasser M, et al. Hypoxia-driven immunosuppression by Treg and type-2 conventional dendritic cells in HCC. Hepatology. (2022) 76:1329–44. doi: 10.1002/hep.32419
171. Akkaya B, Oya Y, Akkaya M, Al Souz J, Holstein AH, Kamenyeva O, et al. Regulatory T cells mediate specific suppression by depleting peptide-MHC class II from dendritic cells. Nat Immunol. (2019) 20:218–31. doi: 10.1038/s41590-018-0280-2
172. Pang L, Ng KT, Liu J, Yeung WO, Zhu J, Chiu TS, et al. Plasmacytoid dendritic cells recruited by HIF-1alpha/eADO/ADORA1 signaling induce immunosuppression in hepatocellular carcinoma. Cancer Lett. (2021) 522:80–92. doi: 10.1016/j.canlet.2021.09.022
173. Pedroza-Gonzalez A, Zhou G, Vargas-Mendez E, Boor PP, Mancham S, Verhoef C, et al. Tumor-infiltrating plasmacytoid dendritic cells promote immunosuppression by Tr1 cells in human liver tumors. Oncoimmunology. (2015) 4:e1008355. doi: 10.1080/2162402X.2015.1008355
174. Chen C, Ma YH, Zhang YT, Zhang F, Zhou N, Wang X, et al. Effect of dendritic cell-based immunotherapy on hepatocellular carcinoma: A systematic review and meta-analysis. Cytotherapy. (2018) 20:975–89. doi: 10.1016/j.jcyt.2018.06.002
175. Xiao Z, Li T, Zheng X, Lin L, Wang X, Li B, et al. Nanodrug enhances post-ablation immunotherapy of hepatocellular carcinoma via promoting dendritic cell maturation and antigen presentation. Bioact Mater. (2023) 21:57–68. doi: 10.1016/j.bioactmat.2022.07.027
176. Zhang D, Lin Z, Wu M, Cai Z, Zheng Y, He L, et al. Cytosolic delivery of thiolated neoantigen nano-vaccine combined with immune checkpoint blockade to boost anti-cancer T cell immunity. Adv Sci (Weinh). (2021) 8:2003504. doi: 10.1002/advs.202003504
177. Lu Z, Zuo B, Jing R, Gao X, Rao Q, Liu Z, et al. Dendritic cell-derived exosomes elicit tumor regression in autochthonous hepatocellular carcinoma mouse models. J Hepatol. (2017) 67:739–48. doi: 10.1016/j.jhep.2017.05.019
178. Maier B, Leader AM, Chen ST, Tung N, Chang C, LeBerichel J, et al. A conserved dendritic-cell regulatory program limits antitumour immunity. Nature. (2020) 580:257–62. doi: 10.1038/s41586-020-2134-y
179. Magen A, Hamon P, Fiaschi N, Soong BY, Park MD, Mattiuz R, et al. Intratumoral dendritic cell-CD4(+) T helper cell niches enable CD8(+) T cell differentiation following PD-1 blockade in hepatocellular carcinoma. Nat Med. (2023) 29:1389–99. doi: 10.1038/s41591-023-02345-0
180. Chuah S, Lee J, Song Y, Kim HD, Wasser M, Kaya NA, et al. Uncoupling immune trajectories of response and adverse events from anti-PD-1 immunotherapy in hepatocellular carcinoma. J Hepatol. (2022) 77:683–94. doi: 10.1016/j.jhep.2022.03.039
181. Barsch M, Salie H, Schlaak AE, Zhang Z, Hess M, Mayer LS, et al. T-cell exhaustion and residency dynamics inform clinical outcomes in hepatocellular carcinoma. J Hepatol. (2022) 77:397–409. doi: 10.1016/j.jhep.2022.02.032
182. Rudloff MW, Zumbo P, Favret NR, Roetman JJ, Detres Roman CR, Erwin MM, et al. Hallmarks of CD8(+) T cell dysfunction are established within hours of tumor antigen encounter before cell division. Nat Immunol. (2023) 24:1527–39. doi: 10.1038/s41590-023-01578-y
183. Lim CJ, Lee YH, Pan L, Lai L, Chua C, Wasser M, et al. Multidimensional analyses reveal distinct immune microenvironment in hepatitis B virus-related hepatocellular carcinoma. Gut. (2019) 68:916–27. doi: 10.1136/gutjnl-2018-316510
184. Cheng Y, Gunasegaran B, Singh HD, Dutertre CA, Loh CY, Lim JQ, et al. Non-terminally exhausted tumor-resident memory HBV-specific T cell responses correlate with relapse-free survival in hepatocellular carcinoma. Immunity. (2021) 54:1825–40 e7. doi: 10.1016/j.immuni.2021.06.013
185. Sun L, Su Y, Jiao A, Wang X, Zhang B. T cells in health and disease. Signal Transduct Target Ther. (2023) 8:235. doi: 10.1038/s41392-023-01471-y
186. Borst J, Ahrends T, Babala N, Melief CJM, Kastenmuller W. CD4(+) T cell help in cancer immunology and immunotherapy. Nat Rev Immunol. (2018) 18:635–47. doi: 10.1038/s41577-018-0044-0
187. Ruterbusch M, Pruner KB, Shehata L, Pepper M. In vivo CD4(+) T cell differentiation and function: revisiting the th1/th2 paradigm. Annu Rev Immunol. (2020) 38:705–25. doi: 10.1146/annurev-immunol-103019-085803
188. Zhang JP, Yan J, Xu J, Pang XH, Chen MS, Li L, et al. Increased intratumoral IL-17-producing cells correlate with poor survival in hepatocellular carcinoma patients. J Hepatol. (2009) 50:980–9. doi: 10.1016/j.jhep.2008.12.033
189. Song M, Wang L, Jiang S, Liang J, Li W, Rao W, et al. Pathogenic Th17 cell-mediated liver fibrosis contributes to resistance to PD-L1 antibody immunotherapy in hepatocellular carcinoma. Int Immunopharmacol. (2024) 129:111601. doi: 10.1016/j.intimp.2024.111601
190. Fu J, Xu D, Liu Z, Shi M, Zhao P, Fu B, et al. Increased regulatory T cells correlate with CD8 T-cell impairment and poor survival in hepatocellular carcinoma patients. Gastroenterology. (2007) 132:2328–39. doi: 10.1053/j.gastro.2007.03.102
191. Gao Q, Qiu SJ, Fan J, Zhou J, Wang XY, Xiao YS, et al. Intratumoral balance of regulatory and cytotoxic T cells is associated with prognosis of hepatocellular carcinoma after resection. J Clin Oncol. (2007) 25:2586–93. doi: 10.1200/JCO.2006.09.4565
192. Zhu AX, Abbas AR, de Galarreta MR, Guan Y, Lu S, Koeppen H, et al. Molecular correlates of clinical response and resistance to atezolizumab in combination with bevacizumab in advanced hepatocellular carcinoma. Nat Med. (2022) 28:1599–611. doi: 10.1038/s41591-022-01868-2
193. Khan O, Giles JR, McDonald S, Manne S, Ngiow SF, Patel KP, et al. TOX transcriptionally and epigenetically programs CD8(+) T cell exhaustion. Nature. (2019) 571:211–8. doi: 10.1038/s41586-019-1325-x
194. McLane LM, Abdel-Hakeem MS, Wherry EJ. CD8 T cell exhaustion during chronic viral infection and cancer. Annu Rev Immunol. (2019) 37:457–95. doi: 10.1146/annurev-immunol-041015-055318
195. Jiang D, Huang A, Zhu BX, Gong J, Ruan YH, Liu XC, et al. Targeting CD93 on monocytes revitalizes antitumor immunity by enhancing the function and infiltration of CD8(+) T cells. J Immunother Cancer. (2024) 12:e010148. doi: 10.1136/jitc-2024-010148
196. Laumont CM, Banville AC, Gilardi M, Hollern DP, Nelson BH. Tumour-infiltrating B cells: immunological mechanisms, clinical impact and therapeutic opportunities. Nat Rev Cancer. (2022) 22:414–30. doi: 10.1038/s41568-022-00466-1
197. Kurebayashi Y, Ojima H, Tsujikawa H, Kubota N, Maehara J, Abe Y, et al. Landscape of immune microenvironment in hepatocellular carcinoma and its additional impact on histological and molecular classification. Hepatology. (2018) 68:1025–41. doi: 10.1002/hep.29904
198. Calderaro J, Petitprez F, Becht E, Laurent A, Hirsch TZ, Rousseau B, et al. Intra-tumoral tertiary lymphoid structures are associated with a low risk of early recurrence of hepatocellular carcinoma. J Hepatol. (2019) 70:58–65. doi: 10.1016/j.jhep.2018.09.003
199. Fridman WH, Meylan M, Pupier G, Calvez A, Hernandez I, Sautes-Fridman C. Tertiary lymphoid structures and B cells: An intratumoral immunity cycle. Immunity. (2023) 56:2254–69. doi: 10.1016/j.immuni.2023.08.009
200. Barrow F, Khan S, Wang H, Revelo XS. The emerging role of B cells in the pathogenesis of NAFLD. Hepatology. (2021) 74:2277–86. doi: 10.1002/hep.31889
201. Shao Y, Lo CM, Ling CC, Liu XB, Ng KT, Chu AC, et al. Regulatory B cells accelerate hepatocellular carcinoma progression via CD40/CD154 signaling pathway. Cancer Lett. (2014) 355:264–72. doi: 10.1016/j.canlet.2014.09.026
202. Li Y, Huang H, Wang Q, Zheng X, Zhou Y, Kong X, et al. Identification of prognostic risk model based on plasma cell markers in hepatocellular carcinoma through single-cell sequencing analysis. Front Genet. (2024) 15:1363197. doi: 10.3389/fgene.2024.1363197
203. Sharma A, Seow JJW, Dutertre CA, Pai R, Bleriot C, Mishra A, et al. Onco-fetal reprogramming of endothelial cells drives immunosuppressive macrophages in hepatocellular carcinoma. Cell. (2020) 183:377–94 e21. doi: 10.1016/j.cell.2020.08.040
204. Ruf B, Heinrich B, Greten TF. Immunobiology and immunotherapy of HCC: spotlight on innate and innate-like immune cells. Cell Mol Immunol. (2021) 18:112–27. doi: 10.1038/s41423-020-00572-w
205. Sun C, Xu J, Huang Q, Huang M, Wen H, Zhang C, et al. High NKG2A expression contributes to NK cell exhaustion and predicts a poor prognosis of patients with liver cancer. Oncoimmunology. (2017) 6:e1264562. doi: 10.1080/2162402X.2016.1264562
206. Sun H, Huang Q, Huang M, Wen H, Lin R, Zheng M, et al. Human CD96 correlates to natural killer cell exhaustion and predicts the prognosis of human hepatocellular carcinoma. Hepatology. (2019) 70:168–83. doi: 10.1002/hep.30347
207. Guo X, Tan S, Wang T, Sun R, Li S, Tian P, et al. NAD + salvage governs mitochondrial metabolism, invigorating natural killer cell antitumor immunity. Hepatology. (2023) 78:468–85. doi: 10.1002/hep.32658
208. Mantovani S, Oliviero B, Varchetta S, Mele D, Mondelli MU. Natural killer cell responses in hepatocellular carcinoma: implications for novel immunotherapeutic approaches. Cancers (Basel). (2020) 12:926. doi: 10.3390/cancers12040926
209. Vallera DA, Felices M, McElmurry R, McCullar V, Zhou X, Schmohl JU, et al. IL15 trispecific killer engagers (TriKE) make natural killer cells specific to CD33+ Targets while also inducing persistence, in vivo expansion, and enhanced function. Clin Cancer Res. (2016) 22:3440–50. doi: 10.1158/1078-0432.CCR-15-2710
210. Davis ZB, Vallera DA, Miller JS, Felices M. Natural killer cells unleashed: Checkpoint receptor blockade and BiKE/TriKE utilization in NK-mediated anti-tumor immunotherapy. Semin Immunol. (2017) 31:64–75. doi: 10.1016/j.smim.2017.07.011
211. Chan WK, Kang S, Youssef Y, Glankler EN, Barrett ER, Carter AM, et al. A CS1-NKG2D bispecific antibody collectively activates cytolytic immune cells against multiple myeloma. Cancer Immunol Res. (2018) 6:776–87. doi: 10.1158/2326-6066.CIR-17-0649
212. Xiao L, Cen D, Gan H, Sun Y, Huang N, Xiong H, et al. Adoptive transfer of NKG2D CAR mRNA-engineered natural killer cells in colorectal cancer patients. Mol Ther. (2019) 27:1114–25. doi: 10.1016/j.ymthe.2019.03.011
213. Lee JH, Lee JH, Lim YS, Yeon JE, Song TJ, Yu SJ, et al. Adjuvant immunotherapy with autologous cytokine-induced killer cells for hepatocellular carcinoma. Gastroenterology. (2015) 148:1383–91 e6. doi: 10.1053/j.gastro.2015.02.055
214. Curio S, Belz GT. The unique role of innate lymphoid cells in cancer and the hepatic microenvironment. Cell Mol Immunol. (2022) 19:1012–29. doi: 10.1038/s41423-022-00901-1
215. He Y, Luo J, Zhang G, Jin Y, Wang N, Lu J, et al. Single-cell profiling of human CD127(+) innate lymphoid cells reveals diverse immune phenotypes in hepatocellular carcinoma. Hepatology. (2022) 76:1013–29. doi: 10.1002/hep.32444
216. Heinrich B, Gertz EM, Schaffer AA, Craig A, Ruf B, Subramanyam V, et al. The tumour microenvironment shapes innate lymphoid cells in patients with hepatocellular carcinoma. Gut. (2022) 71:1161–75. doi: 10.1136/gutjnl-2021-325288
217. Chen C, Wang Z, Ding Y, Qin Y. Tumor microenvironment-mediated immune evasion in hepatocellular carcinoma. Front Immunol. (2023) 14:1133308. doi: 10.3389/fimmu.2023.1133308
Keywords: liver cancer, immune microenvironment, artificial intelligence, machine learning, ScRNA-seq
Citation: Bukhari I, Li M, Li G, Xu J, Zheng P and Chu X (2024) Pinpointing the integration of artificial intelligence in liver cancer immune microenvironment. Front. Immunol. 15:1520398. doi: 10.3389/fimmu.2024.1520398
Received: 31 October 2024; Accepted: 02 December 2024;
Published: 20 December 2024.
Edited by:
Bing Yang, Tianjin Medical University, ChinaReviewed by:
Chong Yin, Affiliated Hospital of North Sichuan Medical College, ChinaLiwei Zheng, University of Colorado Anschutz Medical Campus, United States
Copyright © 2024 Bukhari, Li, Li, Xu, Zheng and Chu. This is an open-access article distributed under the terms of the Creative Commons Attribution License (CC BY). The use, distribution or reproduction in other forums is permitted, provided the original author(s) and the copyright owner(s) are credited and that the original publication in this journal is cited, in accordance with accepted academic practice. No use, distribution or reproduction is permitted which does not comply with these terms.
*Correspondence: Xiufeng Chu, Y2h1eGl1ZmVuZzgzMTAzMUBnbWFpbC5jb20=
†These authors have contributed equally to this work