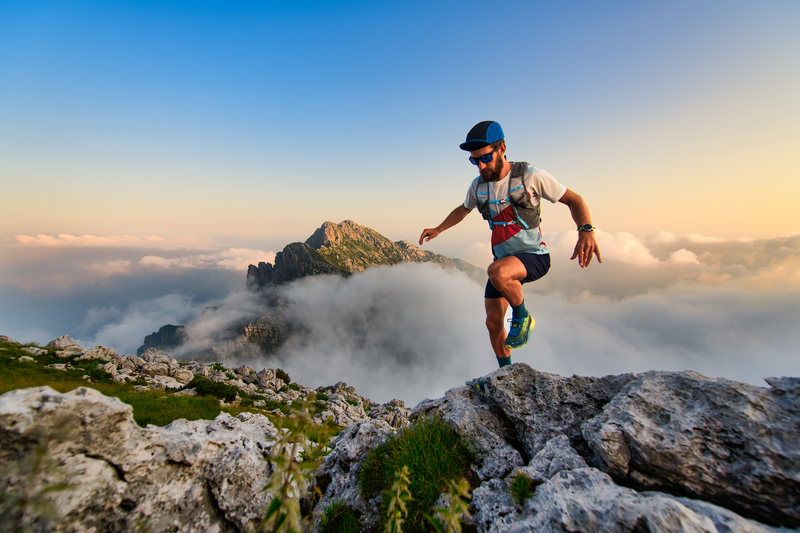
94% of researchers rate our articles as excellent or good
Learn more about the work of our research integrity team to safeguard the quality of each article we publish.
Find out more
ORIGINAL RESEARCH article
Front. Immunol. , 27 January 2025
Sec. Cancer Immunity and Immunotherapy
Volume 15 - 2024 | https://doi.org/10.3389/fimmu.2024.1516524
This article is part of the Research Topic Advancements in Multi-Omics and Bioinformatics for the Management of Solid Malignancies View all 17 articles
Background: Immune checkpoint inhibitors have proven efficacy against hepatitis B-virus positive hepatocellular. However, Immunotherapy-related adverse reactions are still a major challenge faced by tumor immunotherapy, so it is urgent to establish new methods to effectively predict immunotherapy-related adverse reactions.
Objective: Multi-machine learning model were constructed to screen the risk factors for irAEs in ICIs for the treatment of HBV-related hepatocellular and build a prediction model for the occurrence of clinical IRAEs.
Methods: Data from 274 hepatitis B virus positive tumor patients who received PD-1 or/and CTLA4 inhibitor treatment and had immune cell detection results were collected from Henan Cancer Hospital for retrospective analysis. Models were established using Lasso, RSF (RandomForest), and xgBoost, with ten-fold cross-validation and resampling methods used to ensure model reliability. The impact of influencing factors on irAEs (immune-related adverse events) was validated using Decision Curve Analysis (DCA). Both uni/multivariable analysis were accomplished by Chi-square/Fisher’s exact tests. The accuracy of the model is verified in the DCA curve.
Results: A total of 274 HBV-related liver cancer patients were enrolled in the study. Predictive models were constructed using three machine learning algorithms to analyze and statistically evaluate clinical characteristics, including immune cell data. The accuracy of the Lasso regression model was 0.864, XGBoost achieved 0.903, and RandomForest reached 0.961. Resampling internal validation revealed that RandomForest had the highest recall rate (AUC = 0.892). Based on machine learning-selected indicators, antiviral therapy and The HBV DNA copy number showed a significant correlation with both the occurrence and severity of irAEs. Antiviral therapy notably reduced the incidence of IRAEs and may modulate these events through regulation of B cells. The DCA model also demonstrated strong predictive performance. Effective control of viral load through antiviral therapy significantly mitigates the occurrence of irAEs.
Conclusion: ICIs show therapeutic potential in the treatment of HBV-HCC. Following antiviral therapy, the incidence of severe irAEs decreases. Even in cases where viral load control is incomplete, continuous antiviral treatment can still mitigate the occurrence of irAEs.
Hepatocellular carcinoma, distinguished by its high incidence and fatality rates, is the sixth most common of cancer-related deaths worldwide (1). Primary liver cancer is mainly hepatocellular carcinoma, accounting for more than 70% (2). Research shows that high risk factors related to liver cancer are related to multiple viral infections, the main ones being hepatitis B virus and hepatitis C virus (3). For patients with liver cancer in early clinical stages, clinical treatment methods mainly include surgical resection, local therapeutic intervention, liver allotransplantation, etc (4, 5). However, for patients with high clinical stages of liver cancer, current clinical intervention methods still cannot effectively control recurrence or metastasis within 5 years (4). Currently, the preferred clinical treatment for advanced liver cancer is targeted therapy based on anti-tumor angiogenesis-related drugs, but the clinical benefits are still poor (6).
T cells’ intrinsic negative immune regulators, such as CTLA-4, PD-1, and their ligands, can be blocked by immune checkpoint inhibitors (ICIs), which enhance T cell cytotoxicity and augment the antitumor activity of T lymphocytes (7). Studies have shown that ICIs have provided significant benefits in treating various cancers, including lung cancer, melanoma, renal cell carcinoma, and head and neck tumors (8–11). In individuals with early-stage hepatocellular carcinoma, PD-1 inhibitors like nivolumab and pembrolizumab have shown substantial clinical efficacy, markedly improving both overall survival and disease-free survival rates (12–14). The combination of anti-PD-1 antibodies and anti-angiogenic therapy, such as bevacizumab, has shown even greater clinical benefits in patients with advanced liver cancer (15, 16). Moreover, the therapeutic potential of cabozantinib in accompanied with pembrolizumab for the remedy of advanced hepatocellular carcinoma is actively being assessed (17). Despite the remarkable success of ICIs in advanced liver cancer, predictive factors for their clinical efficacy remain limited, with microsatellite instability, gut microbiota and TMB (tumor mutation burden) being among the few identified (18–20).
irAEs are a manifestation of the inherent limitations of immune tolerance, primarily induced by immune checkpoint inhibitors (ICIs) that trigger the production of auto-antibodies and pathogenic antibodies (21–24). These irAEs have the potential to impact any organ system and are categorized into five distinct grades according to their severity (9, 25). Clinically, patients receiving ICI therapy require frequent monitoring to mitigate the risk of irAEs (26, 27). Research indicates that irAEs are intricately linked to the function of immune checkpoint inhibitors (ICIs) in preserving immune homeostasis. Multiple potential mechanisms have been suggested, including T cell activation against self-antigens, the production of auto-antibodies and pro-inflammatory cytokines, as well as increased complement activation targeting self-antigens (7, 28). The mechanism of occurrence of immune-related adverse events determines the specificity of their systemic pathogenesis, including inflammatory arthritis, Sjögren’s disease, vasculitis, joint pain, or tendinopathy (29–31). In severe cases, bone marrow suppression may even occur (32). Because of this, the occurrence of immune-related adverse events seriously affects the clinical treatment of cancer patients and has become an important issue that must be addressed (33).
Recent studies have revealed that severe irAEs can interrupt cancer patients’ immunotherapy, potentially hindering the clinical benefits of these treatments (28). Although there has been some research on the management of irAEs, identifying clinical indicators and methods to predict or mitigate irAEs remains an urgent need. In this study, we analyzed clinical data from liver cancer patients undergoing immunotherapy to assess the risk factors and associated indicators of irAEs.
This retrospective study encompassed patients diagnosed with liver cancer at the Affiliated Cancer Hospital of Zhengzhou University from January 2019 to February 2024, the process of including cases in the study is shown in the Figure 7. Diagnosis was based on clinical pathology and imaging in accordance with the criteria set by the American Association for the Study of Liver Diseases (AASLD), including laboratory-confirmed positive HBV DNA serology, with all patients having undergone at least one PD-1 inhibitor treatment. Clinical data were meticulously gathered through manual examination of patient records and pertinent test outcomes. Brought into criteria were: 1. Patients older than 18. 2. Positive laboratory results for HBV DNA. 3. Eastern Cooperative Oncology Group performance status (ECOG PS) scores ranging 0 - 2, with at least one measurable lesion per the Response Evaluation Criteria in Solid Tumors (RECIST) 1.1 guidelines. The efficacy of immunotherapy was evaluated based on RECIST 1.1 standards, categorizing outcomes as complete response (CR), partial response (PR), stable disease (SD), or progressive disease (PD). 4. The severity of immune-related adverse events (irAEs) was classified by the Common Terminology Criteria for Adverse Events (CTCAE 5.0) established by the U.S. National Cancer Institute. 5. Patients on antiviral therapy were included if they had received such treatment prior to or concurrently with PD-1 inhibitors. Among the included cases, 20 patients unreceived anti-viral treatment during ICIs, 44 exhibited poor compliance and ceased antiviral therapy before hospitalization, 18 discontinued due to financial difficulties, and 7 self-discontinued antiviral therapy prior to immunotherapy. 6. Laboratory evaluations encompassed peripheral blood immune cell assays, treatment protocols, clinical outcomes, and related biochemical results. HBV reactivation was defined per the 2018 AASLD hepatitis B guidelines, meeting at least one of the following criteria: (i) virus DNA increase of ≥ 2 log (100-fold) versus baseline; (ii) DNA increase ≥ 3 log (1,000) IU/mL (for patients non-detectable previously serum virus DNA, recognizing the potential for fluctuations in HBV DNA levels); or (iii) if baseline levels were unavailable, virus DNA increase ≥ 4 log (10,000) IU/mL (34). The albumin/bilirubin (ALBI) grade was calculated using the formula: (0.66 × log10 bilirubin) + (−0.085 × albumin), with bilirubin measured in μmol/L and albumin in g/L. The grading criteria are defined as follows: Grade 1, ALBI ≤ −2.60; Grade 2, −2.60 < ALBI ≤ −1.39; and Grade 3, ALBI > −1.39 (35).
The results of this study, along with the relevant statistical analyses, were completed by R language (version 4.4.0). Numerical variables that adhering normal distribution are expressed as mean ± standard, chi-square or Fisher’s exact test were utilized for analysis of categorical variables. Lasso (glmnet-4.1-8), RSF (randomForest-4.7), and XGBoost (xgBoost-2.1.3) were used to assess the importance of both categorical and numerical variables in predicting outcomes over the observation period. Rank-sum tests were used to evaluate differences in stratified data, and univariate analyses were performed using two-tailed t-tests. p <0.05 means statistically significant.
A total of 274 HBV-positive liver cancer patients who received ICIs treatment were enrolled in the research. clinical characteristics are summarized in Supplementary Table 1. All the enrolled samples, 191 were male (69.7%) and 83 were female (30.3%). Among male patients, 72% experienced grade 1-2 irAEs, while 28% of female patients experienced grade 1-2 irAEs. The cohort included 119 patients (43.4%) aged 60 and older, and 155 patients (56.6%) under 60. A total of 214 patients (78.1%) developed grade 1-2 irAEs, while 60 patients (21.9%) experienced grade 3-4 irAEs.
As shown in Table 1, 42.1% of patients aged 60 and older developed grade 1-2 irAEs, compared to 57.9% of patients under 60. Of the 184 patients who received antiviral treatment during PD-1 inhibitor therapy, 168 (78.5%) experienced grade 1-2 irAEs, and 16 (26.7%) experienced grade 3-4 irAEs. According to the RECIST evaluation, 7 patients achieved complete response (CR), 81 had partial response (PR), 128 had stable disease (SD), and 51 experienced progressive disease (PD). Among patients with PR and CR, 72 (77.4%) received antiviral treatment, compared to 21 (22.6%) who did not, with a significant difference (p = 0.014, Supplementary Table 1).
Table 1. Baseline information on clinical subgroups of patients with different grades of immune adverse events.
Patients with grade 1-2 irAEs had a lower proportion of HBV DNA levels above 500 IU/mL compared to those with grade 3-4 irAEs (p < 0.001). Additionally, patients who received antiviral therapy had a significantly higher proportion of irAEs (p < 0.001), with notably elevated absolute B cell counts (p = 0.005) and significantly lower ALBI scores (p = 0.006). However, no significant differences were observed in other immune cell proportions and absolute counts, tumor size, ECOG scores, alpha-fetoprotein levels, treatment regimens, vascular invasion, or liver function across the different grades of irAEs. Supplementary Table 2 demonstrates that liver cancer patients who received antiviral therapy had a significantly higher proportion of clinical benefit from immunotherapy (p = 0.014), Supplementary Tables 3 and 4 present the statistics of the occurrence of immune-related adverse reactions in different organs and the effects of different antiviral drugs on the efficacy of immunotherapy, respectively.
To identify clinical indicators associated with irAEs, we conducted a lasso regression analysis on the selected clinical parameters, utilizing ten-fold cross-validation. The results from the lasso regression are displayed in Figure 1A, showing the distribution of clinical characteristics after applying the lasso regression model. Cross-validation parameters were optimized using the minimum lambda value (lambda min), and both the optimal lambda min and lambda standard error (lambda se) were used to generate the ten-fold cross-validation curve (Figure 1B). The minimum standard value was identified through cross-validation, and the corresponding ten-fold cross-validation curve was plotted (Figure 1B). As a result, we identified seven clinical parameters with non-zero coefficients (Figure 2E). Univariate and multivariate logistic regression analyses further confirmed that antiviral therapy and HBV DNA levels were independent risk factors for the occurrence of irAEs (Table 2).
Figure 1. LASSO coefficient was used to analyze the risk factors of immune-related adverse events. (A) Lasso regression ten-fold cross validation curve. (B) In the LASSO model, the non-zero coefficient characteristic curve is extracted from the log (A) series. The vertical dashed lines are drawn at the minimum mean square error (λ = 0.0013) and the minimum distance standard error (λ = 0.073).
Figure 2. Machine learning feature screening. (A) Random forest graph model error curve. (B, C) Random forest ranking of clinical features by importance. (D) Xgboost clinical feature importance ranking. (E) Clinical characteristics of non-zero coefficients in lasso regression.
Table 2. Univariate and multivariate logistic regression with non-zero coefficients in lasso regression.
Randomforest and XGBoost regression are commonly used tree-based machine learning methods for predicting variable importance. In this study, to identify effective predictors of IRAEs (irAEs), we employed both random forest (package_version 4.7) and XGBoost (package_version 2.1.3) models to evaluate the importance of relevant variables. Using random forest analysis, we selected the top 10 variables based on importance rankings and illustrated the model’s error rate (Figures 2A–C). After constructing the XGBoost model, we similarly extracted and ranked the top 10 variables based on importance (Figure 2D). Next, we took the intersection of the variables identified by lasso regression, random forest, and XGBoost, and visualized the results. Notably, only two variables were consistently predicted by all three models: HBV DNA and antiviral therapy (Figure 3A). To further validate the reliability of these models, we applied a ten-fold cross-validation method. All three models demonstrated high accuracy (lasso AUC = 0.864, random forest AUC = 0.961, XGBoost AUC = 0.903) (Figure 4A). The precision-recall (PR) curves also showed satisfactory precision and recall rates for all models (lasso PR AUC = 0.607, random forest PR AUC = 0.892, XGBoost PR AUC = 0.768) (Figure 4B).
Figure 3. Machine learning model performance analysis. (A) Boostrap resampling verifies the accuracy AUC curve of the machine learning model. (B) ROC curve of bootstrap resampling to verify the accuracy of machine learning model.
Figure 4. SHAP interpretation of xgboost clinical parameters. (A) Xgboost screening clinical parameter shape value importance ranking. (B, C) The shap value represents the predictive characteristics of each clinical parameter and the contribution of each parameter to the occurrence of immune-related adverse events. f(x) represents the probability prediction value, red indicates low risk, and yellow indicates high risk.
To further provide a clear and intuitive explanation of the selected variables, SHAP (Shapley Additive explanations) values were utilized to elucidate the contribution of the variables in predicting IRAEs (irAEs) within the models. Figure 5A illustrates the SHAP values of the top 10 most important variables in the model. In the plot, blue represents high-risk factors, while yellow indicates low-risk factors. Antiviral therapy was identified as a low-risk factor for irAE occurrence, whereas high levels of HBV DNA were found to be a high-risk factor for irAEs. Other variables, such as the percentage and absolute counts of immune cells, including CD4+ T cells, NK cells, and B cells, were not significantly predictive of irAEs. Figure 5B ranks the SHAP absolute values of the top 10 variables identified by the XGBoost model, with the x-axis indicating the importance of the variables in predicting irAEs. Additionally, we enhanced the interpretability of the XGBoost prediction model using a typical SHAP model (Figure 5C). In this model, antiviral therapy had the lowest score, indicating its role as a protective factor against irAEs, while HBV DNA had the highest score, reinforcing the notion that uncontrolled HBV DNA levels or significant HBV reactivation is a strong driver of irAE development. This finding further supports that antiviral therapy can effectively mitigate the risk of irAEs.
Figure 5. Relationship between screening indicators and clinical events based on machine learning. (A) Intersection of multiple machine learning screening indicators. (B) Comparison of the proportion of immune-related adverse events at all levels between the antiviral treatment and non-antiviral treatment groups. (C) Comparison of the proportion of immune-related adverse events at each level in patients with different HBV DNA copies. (D) DCA curves for predicting immune-related adverse events by antiviral therapy, HBV DNA alone or in combination.
By constructing multiple machine learning models, this study identified antiviral therapy and low HBV DNA copy numbers as effective predictors of IRAEs (irAEs). Subsequently, we compared the incidence of irAEs between two groups: patients receiving antiviral therapy and those who were not, as well as among patients with different levels of HBV DNA copies. The results revealed that irAEs in patients receiving antiviral therapy were primarily concentrated in Grades 1–2, while patients not receiving antiviral therapy predominantly experienced Grade 3–4 irAEs (Figure 3B). This indicates that antiviral therapy effectively reduces the occurrence of severe irAEs. Among patients with low HBV DNA copy numbers, the proportion of Grade 3–4 irAEs was significantly lower, following a similar trend to that observed in patients receiving antiviral therapy (Figure 3C). We hypothesize that antiviral therapy either effectively controls HBV DNA replication or inhibits the reactivation of HBV DNA triggered by immune checkpoint inhibitors, thereby reducing the occurrence of irAEs. DCA (Decision Curve Analysis) further demonstrated that the predictive performance of antiviral therapy for irAE occurrence outperformed that of HBV DNA copy number alone. This suggests that antiviral therapy not only suppresses HBV DNA replication but also modulates immune factors or immune cells involved in irAE development. However, the combined prediction of both factors yielded the best predictive performance (Figure 3D).
The preceding results indicated that antiviral therapy effectively reduces the occurrence of irAEs and, when combined with low HBV DNA copy numbers, serves as a reliable predictor of irAE development. To further explore the relationship between antiviral therapy and immune cells, we analyzed the differences in peripheral blood immune cell levels between patients receiving and not receiving antiviral therapy. The findings revealed a significant increase in the absolute number of B cells in patients undergoing antiviral treatment, whereas no notable changes were observed in the levels of other immune cells (Figures 6A–P).
Figure 6. Analysis of the relationship between antiviral treatment and circulating immune cell levels. The changes in the levels of circulating immune cells in the peripheral blood of patients receiving antiviral treatment and not receiving antiviral treatment included the percentage of T cells (A), the percentage of CD8+T cells (B), the percentage of CD4+T cells (C), the percentage of NK cells (D), the percentage of B cells (E), the percentage of Tregs cells (F), the percentage of PD-1+ cells (G), the percentage of PD-1+CD3+ lymphocytes (H), the percentage of PD-1+CD4+T cells (I), the percentage of PD-1+CD8+T cells (J), the total number of lymphocytes (K), the total number of T cells (L), the absolute value of CD4 (M), the absolute value of CD8 (N), the absolute value of NK (O), and the absolute value of B cells (P).
This study evaluated the safety and efficacy of PD-1 immune checkpoint inhibitors in the treatment of HBV-associated hepatocellular carcinoma. Using multiple machine learning models, we identified biomarkers that can predict IRAEs (irAEs).
Among HBV positive hepatocellular carcinoma patients received treatment with anti-PD-1, 60 patients experienced grade 3-4 IRAEs (irAEs). Of these, 16 patients were undergoing anti-viral. When comparing patients receiving anti-viral to those who were not, irAEs in patients treated with antiviral therapy were predominantly grade 1-2, whereas those without antiviral treatment mainly exhibited grade 3-4 irAEs. Additionally, the analysis revealed that patients with low HBV DNA copy numbers or lower viral activity primarily experienced grade 1-2 irAEs, while those with high HBV DNA copy numbers or reactivated HBV exhibited more frequent grade 3-4 irAEs.
Machine learning is a mathematical discipline that primarily focuses on enabling computers to learn from data (36, 37). In medical research, machine learning models can process data using supervised or unsupervised methods to develop models that identify effective clinical predictors. These models have been applied in areas such as drug response prediction, surgical readmission risk, and patient prognosis (38–41). Common techniques for building clinical machine learning models include LASSO regression, random forest, and XGBoost, which have already been widely used for the selection and prediction of various clinical indicators. For example, machine learning has been used to predict lung cancer recurrence and assess the risk of postoperative thrombosis (42, 43). The combined use of multiple machine-learning models can further enhance the precision of these predictions. Previous studies have utilized various machine learning methods in tandem to predict clinically relevant indicators, demonstrating the reliability and improved performance of these integrated approaches (44, 45).
Here, we first employed lasso-regression to analyze the included clinical indicators with the aim of identifying biomarkers capable of predicting the coming up and severity of irAEs. The results indicated that factors such as age, gender, HBV DNA copy number, antiviral treatment, absolute B cell count, and CD4 T cell percentage were associated with irAE occurrence. Subsequent uni/multivariate logistic regression analyses revealed that HBV DNA copy number, antiviral treatment, and PD1CD3 lymphocytes may serve as independent risk factors for predicting the occurrence of irAEs. According to existing reports, irAEs arise due to ICIs not only blocking immune targets but also activating the immune system, which can trigger autoimmune responses. This activation leads to the release of related effector molecules, which in turn conduce to the development of irAEs (46, 47). HBV-virus infection can recruit a large number of inflammatory factors within the liver, which in turn attract regulatory immune cells (48). These regulatory immune cells are involved in the occurrence of irAEs (46), aligning with our predicted results. Antiviral therapy is currently the mainstay treatment for HBV infection. It has the potential to reverse T cell exhaustion and maintain immune tolerance (49), which may be the underlying reason why antiviral treatment can mitigate the occurrence of irAEs.
CD8+T cells make a crucial role in viral clearance and are also key components of anti-tumor immunity (50, 51). However, in patients with chronic HBV infection, CD8+T cells exhibit signs of exhaustion, with elevated expression of inhibitory checkpoints like PD-1, along with reduced cytotoxic and killing functions. PD-1 inhibitors, by blocking-up the PD-1/PD-L1 singling pathway, can recover CD8+T cell functionality and assist in clearing HBV. However, studies have shown that PD-1 inhibitors may lead to the reactivation of HBV DNA in patients with HBV-related liver cancer (52), suggesting that high HBV DNA levels are a significant risk factor for irAEs. This finding aligns with our prediction that antiviral therapy can effectively reduce the incidence of irAEs.
Additionally, this retrospective study revealed that antiviral therapy can modulate immune cell activity. In HBV positive hepatocellular carcinoma patients receiving anti-viral treatment, there was an evidently increase in the absolute count of circulating B cells, whereas changes in other circulating immune cells were not as pronounced. Previous reports have also identified a reduction in circulating cells as being closely related with the occurrence of severe irAEs (53). However, the underlying mechanisms warrant further investigation. Finally, we conducted Decision Curve Analysis (DCA) to compare the accuracy of predicting irAEs between antiviral treatment and HBV DNA copy.
B cells, as an important component of humoral immunity, participate in the process of clearing viruses in the body. Studies have found that when B cells are cleared by rituximab, HBV replication will be reactivated, leading to aggravated HBV infection (54, 55). In addition, HBVAg-specific B cells can highly express genes for cross-presenting dendritic cell recruitment (XCL1 and CD40LG) and innate immunity (MYD88, IFNA1/13, IFNa2 and IFNB1) to assist humoral immunity in resisting HBV infection (56). In the study, we found that after receiving antiviral treatment, the absolute number of B cells circulating in the patient’s peripheral blood increased, which may be due to the increased release of B cells induced by antiviral treatment, or it may be related to the accelerated promotion of B cells.
The novelty of this study lies in the development of an AI model specifically designed for predicting irAEs in HBV-positive liver cancer patients. This study utilized three machine learning algorithms, incorporating ten-fold cross-validation and bootstrapping for internal validation. Moreover, the comprehensive analysis of clinical indicators based on various machine learning models enhances the precision of the predictions. Nonetheless, this study has inherent limitations due to the restricted sample size. Firstly, it is a retrospective analysis based on clinical treatment data. Secondly, the study’s dataset is limited to patients from a specific geographic region, which may affect the generalizability to multi-regional populations. Finally, although the internal validation of the data confirms the reliability of the predictive model, extensive prospective data are required to further evaluate its applicability.
In summary, our study developed a novel predictive model using three machine learning algorithms to forecast irAEs in HBV-positive liver cancer patients receiving immune checkpoint inhibitors. Among these, the RSF model demonstrated the best predictive performance. This provides theoretical and data support for clinicians to implement early intervention measures to prevent IRAEs.
The original contributions presented in the study are included in the article/Supplementary Material. Further inquiries can be directed to the corresponding author.
This study was approved by the Ethics Committee of the Affiliated Cancer Hospital of Zhengzhou University & Henan Cancer Hospital. The studies were conducted in accordance with the local legislation and institutional requirements. Written informed consent for participation was not required from the participants or the participants’ legal guardians/next of kin in accordance with the national legislation and institutional requirements.
SP: Conceptualization, Data curation, Formal analysis, Investigation, Methodology, Project administration, Resources, Software, Supervision, Validation, Visualization, Writing – original draft, Writing – review & editing. ZW: Conceptualization, Data curation, Formal analysis, Funding acquisition, Investigation, Methodology, Project administration, Resources, Software, Supervision, Validation, Visualization, Writing – original draft, Writing – review & editing.
The author(s) declare financial support was received for the research, authorship, and/or publication of this article. This study was supported by the National Natural Science Foundation of China (81972690), Henan Province Young and Middle-aged Health Science and Technology Innovation Leading Talent Training Project (YXKC2021007),Henan Provincial Health Young and Middle-aged Discipline Leader (HNSWJW-2021024).
The authors declare that the research was conducted in the absence of any commercial or financial relationships that could be construed as a potential conflict of interest.
The author(s) declare that no Generative AI was used in the creation of this manuscript.
All claims expressed in this article are solely those of the authors and do not necessarily represent those of their affiliated organizations, or those of the publisher, the editors and the reviewers. Any product that may be evaluated in this article, or claim that may be made by its manufacturer, is not guaranteed or endorsed by the publisher.
The Supplementary Material for this article can be found online at: https://www.frontiersin.org/articles/10.3389/fimmu.2024.1516524/full#supplementary-material
1. Bray F, Laversanne M, Sung H, Ferlay J, Siegel RL, Soerjomataram I, et al. Global cancer statistics 2022: GLOBOCAN estimates of incidence and mortality worldwide for 36 cancers in 185 countries. CA Cancer J Clin. (2024) 74:229–63. doi: 10.3322/caac.21834
2. Baecker A, Liu X, La Vecchia C, Zhang ZF. Worldwide incidence of hepatocellular carcinoma cases attributable to major risk factors. Eur J Cancer Prev. (2018) 27:205–12. doi: 10.1097/CEJ.0000000000000428
4. Forner A, Reig M, Bruix J. Hepatocellular carcinoma. Lancet. (2018) 391:1301–14. doi: 10.1016/S0140-6736(18)30010-2
5. Zhang L, Liu R, Deng T, Ba Y. Advances in medical treatment of advanced hepatobiliary and pancreatic cancer in 2022. Cancer Innov. (2023) 2:36–51. doi: 10.1002/cai2.60
6. Chen S, Cao Q, Wen W, Wang H. Targeted therapy for hepatocellular carcinoma: Challenges and opportunities. Cancer Lett. (2019) 460:1–9. doi: 10.1016/j.canlet.2019.114428
7. Postow MA, Sidlow R, Hellmann MD. Immune-related adverse events associated with immune checkpoint blockade. N Engl J Med. (2018) 378:158–68. doi: 10.1056/NEJMra1703481
8. Borghaei H, de Marinis F, Dumoulin D, Reynolds C, Theelen W, Percent I, et al. SAPPHIRE: phase III study of sitravatinib plus nivolumab versus docetaxel in advanced nonsquamous non-small-cell lung cancer. Ann Oncol. (2024) 35:66–76. doi: 10.1016/j.annonc.2023.10.004
9. Weber JS, D'Angelo SP, Minor D, Hodi FS, Gutzmer R, Neyns B, et al. Nivolumab versus chemotherapy in patients with advanced melanoma who progressed after anti-CTLA-4 treatment (CheckMate 037): a randomised, controlled, open-label, phase 3 trial. Lancet Oncol. (2015) 16:375–84. doi: 10.1016/S1470-2045(15)70076-8
10. Cella D, Grunwald V, Nathan P, Doan J, Dastani H, Taylor F, et al. Quality of life in patients with advanced renal cell carcinoma given nivolumab versus everolimus in CheckMate 025: a randomised, open-label, phase 3 trial. Lancet Oncol. (2016) 17:994–1003. doi: 10.1016/S1470-2045(16)30125-5
11. Harrington KJ, Ferris RL, Gillison M, Tahara M, Argiris A, Fayette J, et al. Efficacy and safety of nivolumab plus ipilimumab vs nivolumab alone for treatment of recurrent or metastatic squamous cell carcinoma of the head and neck: The phase 2 CheckMate 714 randomized clinical trial. JAMA Oncol. (2023) 9:779–89. doi: 10.1001/jamaoncol.2023.0147
12. El-Khoueiry AB, Sangro B, Yau T, Crocenzi TS, Kudo M, Hsu C, et al. Nivolumab in patients with advanced hepatocellular carcinoma (CheckMate 040): an open-label, non-comparative, phase 1/2 dose escalation and expansion trial. Lancet. (2017) 389:2492–502. doi: 10.1016/S0140-6736(17)31046-2
13. Yau T, Park JW, Finn RS, Cheng AL, Mathurin P, Edeline J, et al. Nivolumab versus sorafenib in advanced hepatocellular carcinoma (CheckMate 459): a randomised, multicentre, open-label, phase 3 trial. Lancet Oncol. (2022) 23:77–90. doi: 10.1016/S1470-2045(21)00604-5
14. Kudo M, Matilla A, Santoro A, Melero I, Gracian AC, Acosta-Rivera M, et al. CheckMate 040 cohort 5: A phase I/II study of nivolumab in patients with advanced hepatocellular carcinoma and child-pugh B cirrhosis. J Hepatol. (2021) 75:600–9. doi: 10.1016/j.jhep.2021.04.047
15. Qin S, Ren Z, Feng YH, Yau T, Wang B, Zhao H, et al. Atezolizumab plus bevacizumab versus sorafenib in the chinese subpopulation with unresectable hepatocellular carcinoma: Phase 3 randomized, open-label IMbrave150 study. Liver Cancer. (2021) 10:296–308. doi: 10.1159/000513486
16. Galle PR, Finn RS, Qin S, Ikeda M, Zhu AX, Kim TY, et al. Patient-reported outcomes with atezolizumab plus bevacizumab versus sorafenib in patients with unresectable hepatocellular carcinoma (IMbrave150): an open-label, randomised, phase 3 trial. Lancet Oncol. (2021) 22:991–1001. doi: 10.1016/S1470-2045(21)00151-0
17. Rizzo A, Dadduzio V, Ricci AD, Massari F, Di Federico A, Gadaleta-Caldarola G, et al. Lenvatinib plus pembrolizumab: the next frontier for the treatment of hepatocellular carcinoma? Expert Opin Investig Drugs. (2022) 31:371–8. doi: 10.1080/13543784.2021.1948532
18. Rizzo A, Brandi G. Biochemical predictors of response to immune checkpoint inhibitors in unresectable hepatocellular carcinoma. Cancer Treat Res Commun. (2021) 27:100328. doi: 10.1016/j.ctarc.2021.100328
19. Zheng J, Shao M, Yang W, Ren J, Chen X, Yang H. Benefits of combination therapy with immune checkpoint inhibitors and predictive role of tumour mutation burden in hepatocellular carcinoma: A systematic review and meta-analysis. Int Immunopharmacol. (2022) 112:109244. doi: 10.1016/j.intimp.2022.109244
20. Zheng Y, Wang T, Tu X, Huang Y, Zhang H, Tan D, et al. Gut microbiome affects the response to anti-PD-1 immunotherapy in patients with hepatocellular carcinoma. J Immunother Cancer. (2019) 7:193. doi: 10.1186/s40425- 019-0650-9
21. Johnson DB, Balko JM, Compton ML, Chalkias S, Gorham J, Xu Y, et al. Fulminant myocarditis with combination immune checkpoint blockade. N Engl J Med. (2016) 375:1749–55. doi: 10.1056/NEJMoa1609214
22. Berner F, Bomze D, Diem S, Ali OH, Fassler M, Ring S, et al. Association of checkpoint inhibitor-induced toxic effects with shared cancer and tissue antigens in non-small cell lung cancer. JAMA Oncol. (2019) 5:1043–7. doi: 10.1001/jamaoncol.2019.0402
23. Calabrese LH, Calabrese C, Cappelli LC. Rheumatic immune-related adverse events from cancer immunotherapy. Nat Rev Rheumatol. (2018) 14:569–79. doi: 10.1038/s41584-018- 0074-9
24. Godwin JL, Jaggi S, Sirisena I, Sharda P, Rao AD, Mehra R, et al. Nivolumab-induced autoimmune diabetes mellitus presenting as diabetic ketoacidosis in a patient with metastatic lung cancer. J Immunother Cancer. (2017) 5:40. doi: 10.1186/s40425-017-0245-2
25. Dueck AC, Mendoza TR, Mitchell SA, Reeve BB, Castro KM, Rogak LJ, et al. Validity and reliability of the US national cancer institute's patient-reported outcomes version of the common terminology criteria for adverse events (PRO-CTCAE). JAMA Oncol. (2015) 1:1051–9. doi: 10.1001/jamaoncol.2015.2639
26. Teraoka S, Fujimoto D, Morimoto T, Kawachi H, Ito M, Sato Y, et al. Early immune-related adverse events and association with outcome in advanced non-small cell lung cancer patients treated with nivolumab: A prospective cohort study. J Thorac Oncol. (2017) 12:1798–805. doi: 10.1016/j.jtho.2017.08.022
27. Weber JS, Hodi FS, Wolchok JD, Topalian SL, Schadendorf D, Larkin J, et al. Safety profile of nivolumab monotherapy: A pooled analysis of patients with advanced melanoma. J Clin Oncol. (2017) 35:785–92. doi: 10.1200/JCO.2015.66.1389
28. Esfahani K, Elkrief A, Calabrese C, Lapointe R, Hudson M, Routy B, et al. Moving towards personalized treatments of immune-related adverse events. Nat Rev Clin Oncol. (2020) 17:504–15. doi: 10.1038/s41571-020-0352-8
29. Roberts J, Ennis D, Hudson M, Ye C, Saltman A, Himmel M, et al. Rheumatic immune-related adverse events associated with cancer immunotherapy: A nationwide multi-center cohort. Autoimmun Rev. (2020) 19:102595. doi: 10.1016/j.autrev.2020.102595
30. Cappelli LC, Gutierrez AK, Bingham CO 3rd, Shah AA. Rheumatic and musculoskeletal immune-related adverse events due to immune checkpoint inhibitors: A systematic review of the literature. Arthritis Care Res (Hoboken). (2017) 69:1751–63. doi: 10.1002/acr.23177
31. Braaten TJ, Brahmer JR, Forde PM, Le D, Lipson EJ, Naidoo J, et al. Immune checkpoint inhibitor-induced inflammatory arthritis persists after immunotherapy cessation. Ann Rheum Dis. (2020) 79:332–8. doi: 10.1136/annrheumdis-2019-216109
32. Rapoport BL, Shannon VR, Cooksley T, Johnson DB, Anderson L, Blidner AG, et al. Pulmonary toxicities associated with the use of immune checkpoint inhibitors: An update from the immuno-oncology subgroup of the neutropenia, infection & myelosuppression study group of the multinational association for supportive care in cancer. Front Pharmacol. (2021) 12:743582. doi: 10.3389/fphar.2021.743582
33. Reid P, Cappelli LC. Treatment of rheumatic adverse events of cancer immunotherapy. Best Pract Res Clin Rheumatol. (2022) 36:101805. doi: 10.1016/j.berh.2022.101805
34. Terrault NA, Lok ASF, McMahon BJ, Chang KM, Hwang JP, Jonas MM, et al. Update on prevention, diagnosis, and treatment of chronic hepatitis b: AASLD 2018 hepatitis B guidance. Hepatology. (2018) 67:1560–99. doi: 10.1002/hep.29800
35. Johnson PJ, Berhane S, Kagebayashi C, Satomura S, Teng M, Reeves HL, et al. Assessment of liver function in patients with hepatocellular carcinoma: a new evidence-based approach-the ALBI grade. J Clin Oncol. (2015) 33:550–8. doi: 10.1200/JCO.2014.57.9151
36. Lip GY, Nieuwlaat R, Pisters R, Lane DA, Crijns HJ. Refining clinical risk stratification for predicting stroke and thromboembolism in atrial fibrillation using a novel risk factor-based approach: the euro heart survey on atrial fibrillation. Chest. (2010) 137:263–72. doi: 10.1378/chest.09-1584
37. O'Mahony C, Jichi F, Pavlou M, Monserrat L, Anastasakis A, Rapezzi C, et al. Hypertrophic cardiomyopathy outcomes i. a novel clinical risk prediction model for sudden cardiac death in hypertrophic cardiomyopathy (HCM risk-SCD). Eur Heart J. (2014) 35:2010–20. doi: 10.1093/eurheartj/eht439
38. Ota R, Yamashita F. Application of machine learning techniques to the analysis and prediction of drug pharmacokinetics. J Control Release. (2022) 352:961–9. doi: 10.1016/j.jconrel.2022.11.014
39. Martinez O, Martinez C, Parra CA, Rugeles S, Suarez DR. Machine learning for surgical time prediction. Comput Methods Programs Biomed. (2021) 208:106220. doi: 10.1016/j.cmpb.2021.106220
40. Lei H, Li X, Ma W, Hong N, Liu C, Zhou W, et al. Comparison of nomogram and machine-learning methods for predicting the survival of non- small cell lung cancer patients. Cancer Innov. (2022) 1:135–45. doi: 10.1002/cai2.24
41. Lv L, Zhang Z, Zhang D, Chen Q, Liu Y, Qiu Y, et al. Machine-learning radiomics to predict bone marrow metastasis of neuroblastoma using magnetic resonance imaging. Cancer Innov. (2023) 2:405–15. doi: 10.1002/cai2.92
42. Wang Q, Qiao W, Zhang H, Liu B, Li J, Zang C, et al. Nomogram established on account of Lasso-Cox regression for predicting recurrence in patients with early-stage hepatocellular carcinoma. Front Immunol. (2022) 13:1019638. doi: 10.3389/fimmu.2022.1019638
43. Tang G, Qi L, Sun Z, Liu J, Lv Z, Chen L, et al. Evaluation and analysis of incidence and risk factors of lower extremity venous thrombosis after urologic surgeries: A prospective two-center cohort study using LASSO-logistic regression. Int J Surg. (2021) 89:105948. doi: 10.1016/j.ijsu.2021.105948
44. Dai P, Chang W, Xin Z, Cheng H, Ouyang W, Luo A. Retrospective study on the influencing factors and prediction of hospitalization expenses for chronic renal failure in China based on random forest and LASSO regression. Front Public Health. (2021) 9:678276. doi: 10.3389/fpubh.2021.678276
45. Chen D, Liu J, Zang L, Xiao T, Zhang X, Li Z, et al. Integrated machine learning and bioinformatic analyses constructed a novel stemness-related classifier to predict prognosis and immunotherapy responses for hepatocellular carcinoma patients. Int J Biol Sci. (2022) 18:360–73. doi: 10.7150/ijbs.66913
46. Okiyama N, Tanaka R. Immune-related adverse events in various organs caused by immune checkpoint inhibitors. Allergol Int. (2022) 71:169–78. doi: 10.1016/j.alit.2022.01.001
47. Khan S, Gerber DE. Autoimmunity, checkpoint inhibitor therapy and immune-related adverse events: A review. Semin Cancer Biol. (2020) 64:93–101. doi: 10.1016/j.semcancer.2019.06.012
48. Zhong S, Zhang T, Tang L, Li Y. Cytokines and chemokines in HBV infection. Front Mol Biosci. (2021) 8:805625. doi: 10.3389/fmolb.2021.805625
49. Michalak TI. Diverse virus and host-dependent mechanisms influence the systemic and intrahepatic immune responses in the woodchuck model of hepatitis b. Front Immunol. (2020) 11:853. doi: 10.3389/fimmu.2020.00853
50. Tang TJ, Kwekkeboom J, Mancham S, Binda RS, de Man RA, Schalm SW, et al. Intrahepatic CD8+ t-lymphocyte response is important for therapy-induced viral clearance in chronic hepatitis B infection. J Hepatol. (2005) 43:45–52. doi: 10.1016/j.jhep.2005.01.038
51. Benechet AP, Iannacone M. Determinants of hepatic effector CD8(+) T cell dynamics. J Hepatol. (2017) 66:228–33. doi: 10.1016/j.jhep.2016.07.011
52. Hakim MS, Rahmadika N, Jariah ROA. Expressions of inhibitory checkpoint molecules in acute and chronic HBV and HCV infections: Implications for therapeutic monitoring and personalized therapy. Rev Med Virol. (2020) 30:e2094. doi: 10.1002/rmv.2094
53. Nishimura K, Konishi T, Ochi T, Watanabe R, Noda T, Fukumoto T, et al. CD21(lo) B cells could be a potential predictor of immune- related adverse events in renal cell carcinoma. J Pers Med. (2022) 12(6):888. doi: 10.3390/jpm12060888
54. Li Y, Yin S, Issa R, Tong X, Wang G, Xia J, et al. B cell-mediated humoral immunity in chronic hepatitis B infection. J Clin Transl Hepatol. (2021) 9:592–7. doi: 10.14218/JCTH.2021.00051
55. Vanwolleghem T, Adomati T, Van Hees S, Janssen HLA. Humoral immunity in hepatitis B virus infection: Rehabilitating the B in HBV. JHEP Rep. (2022) 4:100398. doi: 10.1016/j.jhepr.2021.100398
Keywords: hepatocellular carcinoma, immunotherapy, ICIS, irAEs, machine learning
Citation: Pan S and Wang Z (2025) Antiviral therapy can effectively suppress irAEs in HBV positive hepatocellular carcinoma treated with ICIs: validation based on multi machine learning. Front. Immunol. 15:1516524. doi: 10.3389/fimmu.2024.1516524
Received: 24 October 2024; Accepted: 30 December 2024;
Published: 27 January 2025.
Edited by:
Pengpeng Zhang, Nanjing Medical University, ChinaReviewed by:
Ge Zhang, The First Affiliated Hospital of Zhengzhou University, ChinaCopyright © 2025 Pan and Wang. This is an open-access article distributed under the terms of the Creative Commons Attribution License (CC BY). The use, distribution or reproduction in other forums is permitted, provided the original author(s) and the copyright owner(s) are credited and that the original publication in this journal is cited, in accordance with accepted academic practice. No use, distribution or reproduction is permitted which does not comply with these terms.
*Correspondence: Zibing Wang, emx5eXd6YjIxMThAenp1LmVkdS5jbg==
Disclaimer: All claims expressed in this article are solely those of the authors and do not necessarily represent those of their affiliated organizations, or those of the publisher, the editors and the reviewers. Any product that may be evaluated in this article or claim that may be made by its manufacturer is not guaranteed or endorsed by the publisher.
Research integrity at Frontiers
Learn more about the work of our research integrity team to safeguard the quality of each article we publish.