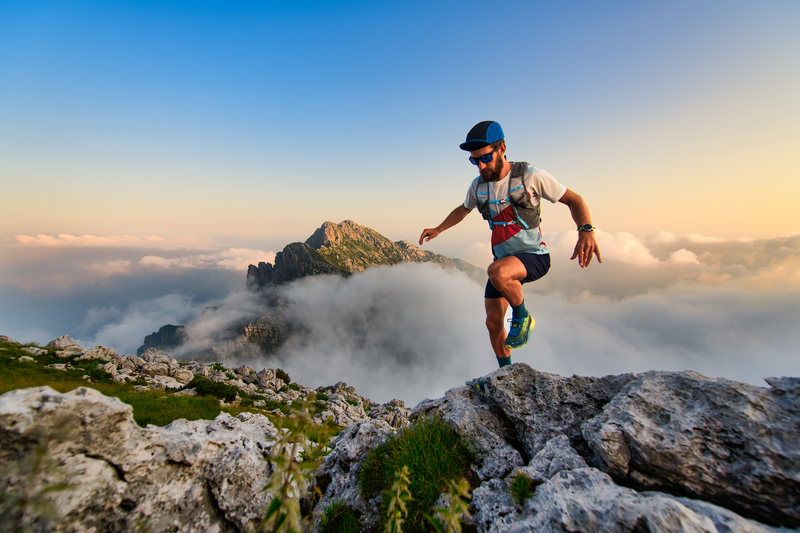
95% of researchers rate our articles as excellent or good
Learn more about the work of our research integrity team to safeguard the quality of each article we publish.
Find out more
ORIGINAL RESEARCH article
Front. Immunol. , 21 January 2025
Sec. Primary Immunodeficiencies
Volume 15 - 2024 | https://doi.org/10.3389/fimmu.2024.1508780
This article is part of the Research Topic Enhancing Early Detection of Primary Immunodeficiencies (PIDs) View all 7 articles
Introduction: Patients with activated PI3Kδ syndrome (APDS) may elude diagnoses for nearly a decade. Methods to hasten the identification of these patients, and other patients with inborn errors of immunity (IEIs), are needed. We sought to demonstrate that querying electronic health record (EHR) systems by aggregating disparate signs into a risk score can identify these patients.
Methods: We developed a structured query language (SQL) script using literature-validated APDS-associated clinical concepts mapped to ICD-10-CM codes. We ran the query across EHRs from 7 large, US-based medical centers encompassing approximately 17 million patients. The query calculated an “APDS Score,” which stratified risk for APDS for all individuals in these systems. Scores for all known patients with APDS (n=46) were compared.
Results: The query identified all but one known patient with APDS (98%; 45/46) as well as patients with other complex disease. Median score for all patients with APDS was 9 (IQR = 5.75; range 1-25). Sensitivity analysis suggested an optimal cutoff score of 7 (sensitivity = 0.70).
Conclusion: Disease-specific queries are a relatively simple method to foster patient identification across the rare-disease spectrum. Such methods are even more important for disorders such as APDS where an approved, pathway-specific treatment is available in the US.
Activated PI3Kδ syndrome (APDS) is an inborn error of immunity (IEI) with a heterogenous clinical presentation with signs of both immune deficiency and immune dysregulation (1–3). Hallmarks of immune deficiency include recurrent sinopulmonary infections and susceptibility to herpesvirus viremia, while dysregulation may manifest as atopy, lymphadenopathy, organomegaly, and autoimmunity (2, 4, 5). The proximate cause of increased risk of lymphoma in APDS is debated, but both deficiency and dysregulation could be implicated (6).
The complex array of symptoms frequently drives a patient to seek care from several types of clinicians. Uncoordinated care, misdiagnoses, status as a rare disease, range of severity of symptoms, unavailability of genetic testing, and other factors may contribute to the 7-year median diagnostic delay (7, 8). APDS is a progressive illness; therefore, like many IEIs, this diagnostic odyssey may increase healthcare utilization, lead to permanent organ damage such as bronchiectasis, and result in other poor outcomes (4, 7, 9–11).
Organizations such as the National Organization for Rare Disorders and Jeffrey Modell Foundation have led robust educational initiatives focused on rare diseases and IEIs specifically (12–14). Despite these and other efforts, diagnostic rates of IEIs remain stable apart from extreme presentations in infants (11, 15). The growing fields of bioinformatics and artificial intelligence investigate and provide methods to search electronic health records (EHRs) to weave together the patient encounters distributed throughout a hospital system (16–18). We sought to demonstrate that querying EHR systems to aggregate the disparate signs into a risk score could help identify patients with APDS.
At study origination, APDS did not have its own ICD-10-CM code, though it has since been assigned a code (81.82). This will presumably raise awareness of the disease; however, to our knowledge, there has been no impact on patient identification to date. We surmise that undiagnosed patients with APDS could be more efficiently identified by EHR query methods. This may be especially useful in the adult population, who may more easily escape diagnoses of an IEI (11, 15). Firstly, genetic disease has the bias of assumed fulminant presentation while the patient is young. Secondly, smoldering presentations in IEIs are not well characterized. They may remain unremarkable, present differently from the pediatric population, or become urgent only in adulthood (19, 20). For example, lymphoma was reported as the first clinical sign of APDS in 20.5% of patients in one cohort (n=39) who had a primary diagnosis charted (7).
Importantly, this disease-specific EHR query method could be mapped to the other nearly 500 IEIs. Interested researchers could also apply structured (16) approaches to initially cull a cohort of high risk or leverage unstructured data approaches such as natural language processing (NLP) additively to this effort (17, 21).
This study was approved by the Institutional Review Board (IRB; H-50212) of Texas Children’s Hospital (TCH), Houston, Texas, with reciprocal approvals by the IRBs at Children’s Hospital Los Angeles, Los Angeles, California, University of South Florida, Tampa, Florida, Mount Sinai School of Medicine, New York, New York, and Spectrum (now Corewell) Health, Grand Rapids, Michigan; as well as a standing IRB for informatics research at University of California Los Angeles, Los Angeles, California.
We developed a structured query language (SQL) script using literature-validated APDS-associated clinical features mapped to ICD-10-CM codes (https://www.icd10data.com/) to identify patients. The initial features used were based upon relevant APDS clinical signs described in the literature up until 2021 (Supplementary File 1).
The raw query was then refined by adding relative-risk weights to selected APDS clinical features as learned from clinical data and previously published (22) and noted in Table 1. Briefly, weights for each feature were mined from a cohort of verified IEI patients (n=1762) by comparing frequencies of clinical conditions to that of a medically complex and diverse set of controls (n=1698). Relative risk values for features relevant to APDS were calculated by normalizing counts per capita as described previously. The learned feature weights and strategy for this approach are noted in Supplementary File 2. APDS score was calculated by summing the weighted features detected for every member of a cohort. For example, if bronchiectasis (weight = 4) and pneumonia (weight = 2) were detected from an individual’s records, their APDS score would be 6. Initial query validation took place within the TCH enterprise data warehouse (EDW) (Supplementary File 4). Having found all known patients at this center, and a patient there who had known PTEN (phosphatase and tensin homolog) syndrome, we sought to validate it at other institutions with known patients with APDS. Ultimately, this disease-specific query extracts predefined codes on each member of a population, builds a cohort of patients with any one of the relevant codes, applies the specified weight to each code-based clinical concept (ie, only counted once if present numerous times) then aggregates and ranks population members by APDS score in descending fashion.
Table 1. Feature weights (22).
We further validated this query by running it through 6 additional large US-based hospitals with known patients with APDS. These patients have been diagnosed by genetic testing performed by the expert immunologists caring for and treating these patients. The query was run directly in the EHR systems at Mount Sinai, New York, NY; Helen DeVos, Spectrum Health, Grand Rapids, MI; Phoenix Children’s Hospital, Phoenix, AZ, Children’s Hospital of Los Angeles (CHLA), LA, CA, and University of South Florida (USF), Tampa, FL. At the University of California, Los Angeles (UCLA), Los Angeles, California, the query was run in a deidentified data warehouse (DDW). The query was initially written for Epic Systems Corporation software and table structure (ie, Clarity) (Supplementary File 2). The refined and weighted query was converted to a language compatible with Cerner by Precision Extract so it could be run at CHLA (Supplementary File 3).
Scores for all known patients with APDS were accumulated using all available data from each center. Median values and interquartile ranges were calculated with Python (3.12.4) and SciPy (1.11.1). A sensitivity analysis was conducted using the Python package NumPy (2.0.1) and plotted in Matplotlib (3.9.1). Optimal sensitivity was determined by plotting sensitivity vs score (Figure 1).
Figure 1. Sensitivity analysis for scores from all known patients with APDS. Balancing sensitivity with number of records captured, the arrow denotes an optimal cutoff score (7) and corresponding sensitivity of 0.70 calibrated from the centers evaluated here. Optimal threshold suggests a sensitivity of 100% and a score of 1, above which a very large number of subjects would be found effectively nullifying the need for our query.
The query identified 98% (45/46) of patients with APDS across all centers (Table 2). One patient was not identified (ie, no scorable features in record); however, that individual is healthy and APDS was diagnosed pre-symptomatically via genetic testing prompted by family history (PIK3R1: c.343C>G p.Leu115Val). The median risk score for known patients with APDS across all institutions was 9, with a range from 1 to 25. The age range of known patients with APDS was 3 years to 69 years of age, with a median of 15 years of age. There did not appear to be a pattern of risk score vs age; however, this may be attributed to limitations of the EHR data. Sensitivity analysis suggested an optimal score cutoff of 7 (sensitivity = 70%). Across nearly 17 million individuals assessed, 41,041 (0.2%) had a score of ≥7 or 7741 patients per site (Figure 1). This represents an APDS high-risk population, which is reduced in number from the total population by more than 400-fold.
The results of the query at each institution are summarized in Table 2.
Some salient results not reflected in Table 2 are as follows: UCLA, USF, and Mount Sinai each had patients with artificially low scores but for slightly differing reasons. At UCLA, the patients with scores of 2 and 3 had APDS features of bronchiectasis and splenomegaly listed in the notes. Further, one patient had marginal hyperplasia, but our query focused on nodular hyperplasia. If the query had also captured unstructured data and expanded the ICD-10-CM codes for hyperplasia, these patients would have had APDS risk scores of 7 and 12, respectively.
Similarly, when a patient is referred into the system at Mount Sinai, the patient’s history is primarily captured in the notes, and ICD-10-CM codes are rarely associated with these historical clinical features. Had there been codes in the structured data for the 3 patients’ complex histories, the scores would have been much higher. Further, these patients had cytopenias, which are a hallmark of APDS, but were not included in this script, and should be in future iterations (9).
At USF, the patient with a score of 1 was referred to the immunology department with a severe case of human papillomavirus (HPV). Our script focused on herpesviruses, a cardinal feature of APDS. However, HPV has also been reported. (4, 7, 23, 24). Up to 50% of patients with APDS have chronic viral infections with a wide range of presentations (25). This patient also had hypogammaglobulinemia and autism. These are common features of APDS and while not in the original query, we suggest adding them to future queries (2, 26).
Finally, variants of uncertain significance (VUSs) in either of the genes that cause APDS are of great interest. The query identified a patient at CHLA who has a VUS in an APDS gene, but two patients at USF who have VUSs in APDS genes were not identified.
We validated our query in seven large, US-based medical centers with 46 known patients with APDS. The query found all but one known patient with APDS (45/46), and that patient is asymptomatic. This relatively simple SQL script was a successful first effort and proof of concept for an EHR-based intervention to find patients with APDS, albeit with a suspected high false positive rate, that we draw attention to with refinement suggestions below. We suspect that this approach could find patients with other disorders of immune dysregulation that share clinical features and will probably be most useful in conditions with unique features or a complex and variable phenotype. Given that APDS and many IEIs are progressive, early diagnosis is critical to patient outcomes and to quality of life; thus, our aim was to create a process for reducing the diagnostic odyssey for patients with APDS. (4, 7, 9–11)
APDS was discovered in 2013, and though the impact of the genetic variants on the immune system was well described by both Angulo and Lucas, the understanding of clinical features in large cohorts continues to expand. (27–29) In 2017 and 2018, the ESID (European Society for Immunodeficiency) registry papers illustrated the main urgent immune features, but it was not until 2021 that atopy and asthma were well-established features of the disease. (2, 4, 9, 30) Thus, other important phenotypic features of APDS have only recently come to light. For example, neurological and behavioral concerns (26) and the specific timing of infections (31) are also recent attributes of the broader APDS phenotype. These findings, and those of other studies (32), emphasize the heterogeneous clinical presentation of APDS and suggest that we still have much to learn.
In newly discovered rare diseases without established natural histories, there may be considerable variation in which signs present, how those are captured in any EHR system, and how much of a patient’s history will be localized at the specialist’s hospital vs scattered among the records systems of the patient’s primary care physician, pulmonologist, gastroenterologist, hematologist, psychologist, and other clinicians. We have presented a script that captured the main features represented in the literature at that time, but we suggest updating this script before use.
Though the script did find nearly all of the patients, it did so while capturing a significant number of presumed false-positive cases. The number of records returned for even the median APDS risk score at each hospital would not be immediately clinically actionable at most of the institutions. Though beyond the purview of our validation effort, many suggestions for refining the script exist. These are a few: running a targeted sensitivity analysis, then refining the script and rerunning it; adding additional ICD-10-CM codes, such as those for autism, hypogammaglobinemia, atopy, common variable immune deficiency or other immune disorders, which the patient may have been previously diagnosed with. Finally, the script could also be revised by adding a “number of encounters” or “events per year” feature, which would capture common signs (eg, atopy, otitis, specific infections) only if they appeared chronically across time or at an above-normal frequency per year, as recommended in the Jeffrey Modell Foundation 10 warning signs of immunodeficiency (33).
PTEN is a negative regulator of the PI3Kδ/ATP pathway; its deficiency can cause a hyperactivity of this pathway, leading to immunological manifestations similar to those observed in APDS (34). We were aware of a patient at TCH with PTEN deficiency and autism, and we intentionally confirmed that this patient record was captured by the script. Given the high record return, the script capture was unsurprising; however, we believe the script would find this record even after refinement. Similarly, it stands to reason that other patients who have IEIs of immune dysregulation (Category IV of the International Union of Immunological Societies classification system) could be identified with a refined version of this script (3).
We have provided the open-source code (Supplementary File 2) to be refined accordingly and used for similar efforts at other institutions. There are many practical considerations to navigate. Efforts to codify the use of AI in large institutions are beginning to appear in the literature, and these roadmaps will be valuable (35, 36). Running a query like this can require coordination with several hospital stakeholders. In our experience, the IRB process varied institution to institution. The FDA guidance for AI tools is evolving (37). Presently, if the tools are considered to be diagnostic, the requirements related to IRB approval might be more significant than what we encountered for script validation. Further, one will need to identify the appropriate people in the information services (IS) department who can help ensure the query is compatible with each EHR, and who can run the query. We have included Epic- and Cerner-compatible scripts in the supplement, but there may be additional changes made based upon unique parameters in a hospital’s system. Finally, though deidentified data warehouses (copies of the hospital’s EHR system with the medical record numbers removed) are compelling systems for research and can expedite IRB approval, they create a nontrivial barrier to clinical implementation in queries like these. Without medical record numbers, it is not easy to refer the appropriate patients.
Our results displayed variability in part due to record management. This includes how each institution codes, refers, and records medical histories; some hospitals did not attach ICD-10-CM codes to any records transferred from outside systems, which meant the patients’ extensive histories were only captured in notes. The amount of care managed locally vs by the hospital specialist will also impact the results.
The most important part of any patient identification effort is how to facilitate accurate diagnosis and access to appropriate care for newly discovered patients. We conducted a survey to this end. Expert considerations ranged from clinician availability to perform chart review, genetic testing and counseling to patient-centered identification and plan for unexpected financial burden. (38) Health systems will need to operationalize a workflow that is sensitive to existing and optimal clinical decision support processes for that organization. Critically, any healthcare analytics of this nature will require ongoing assessment and monitoring to ensure that its performance does not drift.
This was a proof-of-concept effort, and there are numerous limitations to this study. The evolving natural history of APDS is a significant limitation of the script. We included ICD-10-CM codes based on the literature at the time. We did not include cognition codes, which should be included. Also absent were autoimmune, atopy, cytopenias, additional viral infection codes, and some laboratory values. We suggest adding these to the script for further use.
Limitations of the data sets include patients who have few encounters with that system may be missed or have deceptively low scores. ICD-10-CM codes are useful structured data, but there is clinician-based variability in codes used. Some structured data may simply be absent or found in the unstructured patient notes. This last limitation can be corrected by using natural language processing models on top of the SQL query (17, 21).
Finally, this pilot study showcases the utility of a disease-specific query, which may not sufficiently detect other important IEIs or fully capture the phenotypic heterogeneity of APDS. Our experience, as described, offers a potential strategy to compress the diagnostic odyssey for patients with APDS but not patients with IEIs as a whole.
EHR analytical approaches represent a strategy for reducing diagnostic delays among patients with APDS and other IEIs, but further methods to refine this search, and means to expeditiously evaluate new referrals, need to be devised. Our goal is to hasten diagnoses in these devastating diseases, and though AI is an excellent tool to do so, without the human intelligence to change large institutional practices, patients will languish undiagnosed.
The data analyzed in this study is subject to the following licenses/restrictions: The data from the actual electronic health records cannot be supplied as it is not de-identified; however, the metadata is found in the article and the original source codes to generate data are found in the supplement.
The studies involving humans were approved by Institutional Review Board (IRB; H-50212) of Texas Children’s Hospital (TCH), Houston, Texas, with reciprocal approvals by the IRBs at Children’s Hospital Los Angeles, Los Angeles, California, University of South Florida, Tampa, Florida, Mount Sinai School of Medicine, New York, New York, and Spectrum (now Corewell) Health, Grand Rapids, Michigan; as well as a standing IRB for informatics research at University of California Los Angeles, Los Angeles, California. The studies were conducted in accordance with the local legislation and institutional requirements. Written informed consent for participation was not required from the participants or the participants’ legal guardians/next of kin in accordance with the national legislation and institutional requirements.
AF: Conceptualization, Data curation, Project administration, Supervision, Visualization, Writing – original draft, Writing – review & editing, Formal Analysis, Methodology. AC: Data curation, Investigation, Writing – review & editing. SN: Data curation, Investigation, Writing – review & editing. CC-R: Data curation, Investigation, Writing – review & editing. KS: Investigation, Writing – review & editing. JP: Data curation, Investigation, Writing – review & editing. JD: Data curation, Investigation, Writing – review & editing. AT: Data curation, Investigation, Writing – review & editing. JM: Data curation, Investigation, Writing – review & editing. JC: Project administration, Writing – review & editing. JW: Data curation, Investigation, Writing – review & editing. NH: Data curation, Investigation, Writing – review & editing. NI: Investigation, Writing – review & editing, Data curation. MP: Data curation, Investigation, Writing – review & editing. MB: Data curation, Investigation, Writing – review & editing. KL: Data curation, Investigation, Methodology, Software, Writing – original draft. AR: Funding acquisition, Writing – review & editing. NR: Methodology, Project administration, Software, Supervision, Validation, Visualization, Writing – original draft, Writing – review & editing, Conceptualization, Data curation, Formal analysis, Investigation.
The author(s) declare financial support was received for the research, authorship, and/or publication of this article. The authors declare that this study received funding from Pharming Healthcare, Inc. The funder was not involved in the study design, collection, analysis, interpretation of data, the original writing of this article, or the decision to submit it for publication.
We would like to thank Tim VanAken of PRECISIONxtract for the original iteration of the script, for help with converting the script to Cerner, and for guidance about EHRs in general. We also thank Cindy Bauer and Holly Miller at Phoenix Children’s Hospital for their help assessing presentation variability within one family. We also thank Celisse Zabalo and Chaitanya Chaphalkar at University of South Florida for their help in coordinating the teams and executing the script.
AR is an employee of Pharming Healthcare, Inc. NR and CC-R serve as advisory board and steering committee members and as consultants for Pharming Healthcare, Inc. JW serves as speaker for Pharming Healthcare, Inc. NH serves as speaker and consultant for Pharming Healthcare, Inc. MB serves as a steering committee member for Pharming Healthcare, Inc. KS serves as consultant for Pharming Healthcare, Inc.
The remaining authors declare that the research was conducted in the absence of any commercial or financial relationships that could be construed as a potential conflict of interest.
The author(s) declare that no Generative AI was used in the creation of this manuscript.
All claims expressed in this article are solely those of the authors and do not necessarily represent those of their affiliated organizations, or those of the publisher, the editors and the reviewers. Any product that may be evaluated in this article, or claim that may be made by its manufacturer, is not guaranteed or endorsed by the publisher.
The Supplementary Material for this article can be found online at: https://www.frontiersin.org/articles/10.3389/fimmu.2024.1508780/full#supplementary-material
1. Nunes-Santos CJ, Uzel G, Rosenzweig SD. PI3K pathway defects leading to immunodeficiency and immune dysregulation. J Allergy Clin Immunol. (2019) 143:1676–87. doi: 10.1016/j.jaci.2019.03.017
2. Oh J, Garabedian E, Fuleihan R, Cunningham-Rundles C. Clinical manifestations and outcomes of activated phosphoinositide 3-kinase δ syndrome from the USIDNET Cohort. J Allergy Clin Immunol Pract. (2021) 9:4095–102. doi: 10.1016/j.jaip.2021.07.044
3. Tangye SG, Al-Herz W, Bousfiha A, Cunningham-Rundles C, Franco JL, Holland SM, et al. Human inborn errors of immunity: 2022 update on the classification from the International Union of Immunological Societies Expert Committee. J Clin Immunol. (2022) 42:1473–507. doi: 10.1007/s10875-022-01289-3
4. Coulter TI, Chandra A, Bacon CM, Babar J, Curtis J, Screaton N, et al. Clinical spectrum and features of activated phosphoinositide 3-kinase δ syndrome: A large patient cohort study. J Allergy Clin Immunol. (2017) 139:597–606.e594. doi: 10.1016/j.jaci.2016.06.021
5. Lucas CL, Chandra A, Nejentsev S, Condliffe AM, Okkenhaug K. PI3Kδ and primary immunodeficiencies. Nat Rev Immunol. (2016) 16:702–14. doi: 10.1038/nri.2016.93
6. Durandy A, Kracker S. Increased activation of PI3 kinase-δ predisposes to B-cell lymphoma. Blood. (2020) 135:638–43. doi: 10.1182/blood.2019002072
7. Jamee M, Moniri S, Zaki-Dizaji M, Olbrich P, Yazdani R, Jadidi-Niaragh F, et al. Clinical, immunological, and genetic features in patients with activated PI3Kδ syndrome (APDS): A systematic review. Clin Rev Allergy Immunol. (2020) 59:323–33. doi: 10.1007/s12016-019-08738-9
8. Lougaris V, Cancrini C, Rivalta B, Castagnoli R, Giardino G, Volpi S, et al. Activated phosphoinositide 3-kinase delta syndrome (APDS): An update. Pediatr Allergy Immunol. (2022) 33:69–72. doi: 10.1111/pai.13634
9. Maccari ME, Abolhassani H, Aghamohammadi A, Aiuti A, Aleinikova O, Bangs C, et al. Disease evolution and response to rapamycin in activated phosphoinositide 3-kinase δ Syndrome: The European Society for Immunodeficiencies-Activated Phosphoinositide 3-Kinase δ Syndrome Registry. Front Immunol. (2018) 9:543. doi: 10.3389/fimmu.2018.00543
10. Condliffe AM, Chandra A. Respiratory manifestations of the activated phosphoinositide 3-kinase delta syndrome. Front Immunol. (2018) 9:338. doi: 10.3389/fimmu.2018.00338
11. Anderson JT, Cowan J, Condino-Neto A, Levy D, Prusty S. Health-related quality of life in primary immunodeficiencies: Impact of delayed diagnosis and treatment burden. Clin Immunol. (2022) 236:108931. doi: 10.1016/j.clim.2022.108931
12. Quinn J, Modell V, Johnson B, Poll S, Aradhya S, Orange JS, et al. Global expansion of Jeffrey's Insights: Jeffrey Modell Foundation's genetic sequencing program for primary immunodeficiency. Front Immunol. (2022) 13:906540. doi: 10.3389/fimmu.2022.906540
13. Rare Revolution Magazine. An overview of etiological factors, clinical presentation and disease management of activated PI3K delta syndrome (APDS) (2021). Available online at: http://rarerevolutionsmagazinecom.eu-west-1.elasticbeanstalk.com/digitalspotlight/activated-pi3k-delta-syndrome-apds/ (Accessed July 19, 2024).
14. NORD. Continuing medical education (CME) (2024). Available online at: https://rarediseases.org/living-with-a-rare-disease/continuing-medical-education-cme/ (Accessed July 19, 2024).
15. Odnoletkova I, Kindle G, Quinti I, Grimbacher B, Knerr V, Gathmann B, et al. The burden of common variable immunodeficiency disorders: a retrospective analysis of the European Society for Immunodeficiency (ESID) registry data. Orphanet J Rare Dis. (2018) 13:201. doi: 10.1186/s13023-018-0941-0
16. Rider NL, Coffey M, Kurian A, Quinn J, Orange JS, Modell V, et al. A validated artificial intelligence-based pipeline for population-wide primary immunodeficiency screening. J Allergy Clin Immunol. (2023) 151:272–9. doi: 10.1016/j.jaci.2022.10.005
17. Roberts K, Chin AT, Loewy K, Pompeii L, Shin H, Rider NL. Natural language processing of clinical notes enables early inborn error of immunity risk ascertainment. J Allergy Clin Immunol Glob. (2024) 3:100224. doi: 10.1016/j.jacig.2024.100224
18. Johnson R, Stephens AV, Mester R, Knyazev S, Kohn LA, Freund MK, et al. Electronic health record signatures identify undiagnosed patients with common variable immunodeficiency disease. Sci Transl Med. (2024) 16:eade4510. doi: 10.1126/scitranslmed.ade4510
19. Chitty Lopez M, Yilmaz M, Diaz-Cabrera NM, Saco T, Ishmael L, Sotoudeh S, et al. Separating the wheat from the chaff in asthma and bronchiectasis: The saga trajectory of a patient with adult-onset RAG1 deficiency. J Allergy Clin Immunol Pract. (2023) 11:1972–80. doi: 10.1016/j.jaip.2023.04.005
20. Wang JJF, Dhir A, Hildebrand KJ, Turvey SE, Schellenberg R, Chen LYC, et al. Inborn errors of immunity in adulthood. Allergy Asthma Clin Immunol. (2024) 20:6. doi: 10.1186/s13223-023-00862-8
21. Lin S, Nateqi J, Weingartner-Ortner R, Gruarin S, Marling H, Pilgram V, et al. An artificial intelligence-based approach for identifying rare disease patients using retrospective electronic health records applied for Pompe disease. Front Neurol. (2023) 14:1108222. doi: 10.3389/fneur.2023.1108222
22. Rider NL, Cahill G, Motazedi T, Wei L, Kurian A, Noroski LM, et al. PI Prob: A risk prediction and clinical guidance system for evaluating patients with recurrent infections. PLoS One. (2021) 16:e0237285. doi: 10.1371/journal.pone.0237285
23. Carpier JM, Lucas CL. Epstein-Barr virus susceptibility in activated PI3Kδ syndrome (APDS) immunodeficiency. Front Immunol. (2018) 8:2005. doi: 10.3389/fimmu.2017.02005
24. Elkaim E, Neven B, Bruneau J, Mitsui-Sekinaka K, Stanislas A, Heurtier L, et al. Clinical and immunologic phenotype associated with activated phosphoinositide 3-kinase δ syndrome 2: A cohort study. J Allergy Clin Immunol. (2016) 138:210–8. doi: 10.1016/j.jaci.2016.03.022
25. Brodsky NN, Lucas CL. Infections in activated PI3K delta syndrome (APDS). Curr Opin Immunol. (2021) 72:146–57. doi: 10.1016/j.coi.2021.04.010
26. Serra I, Manusama OR, Kaiser FMP, Floriano II, Wahl L, van der Zalm C, et al. Activated PI3Kδ syndrome, an immunodeficiency disorder, leads to sensorimotor deficits recapitulated in a murine model. Brain Behav Immun Health. (2021) 18:100377. doi: 10.1016/j.bbih.2021.100377
27. Angulo I, Vadas O, Garçon F, Banham-Hall E, Plagnol V, Leahy TR, et al. Phosphoinositide 3-kinase δ gene mutation predisposes to respiratory infection and airway damage. Science. (2013) 342:866–71. doi: 10.1126/science.1243292
28. Lucas CL, Kuehn HS, Zhao F, Niemela JE, Deenick EK, Palendira U, et al. Dominant-activating germline mutations in the gene encoding the PI(3)K catalytic subunit p110δ result in T cell senescence and human immunodeficiency. Nat Immunol. (2014) 15:88–97. doi: 10.1038/ni.2771
29. Lucas CL, Zhang Y, Venida A, Wang Y, Hughes J, McElwee J, et al. Heterozygous splice mutation in PIK3R1 causes human immunodeficiency with lymphoproliferation due to dominant activation of PI3K. J Exp Med. (2014) 211:2537–47. doi: 10.1084/jem.20141759
30. Kubala S, Rasooly M, Uzel G, Frischmeyer-Guerrerio P. (65) Increased susceptibility to allergic and autoimmune diseases in patients with activated phosphoinositide 3-kinase (PI3K) delta syndrome (APDS). J Clin Immunol. (2021) 41:1–135. doi: 10.1007/s10875-021-01001-x
31. Bloomfield M, Klocperk A, Zachova R, Milota T, Kanderova V, Sediva A. Natural course of activated phosphoinositide 3-kinase delta syndrome in childhood and adolescence. Front Pediatr. (2021) 9:697706. doi: 10.3389/fped.2021.697706
32. Maccari ME, Wolkewitz M, Schwab C, Lorenzini T, Leiding JW, Aladjdi N, et al. Activated phosphoinositide 3-kinase δ syndrome: Update from the ESID Registry and comparison with other autoimmune-lymphoproliferative inborn errors of immunity. J Allergy Clin Immunol. (2023) 152(4):984–996.e10. doi: 10.1016/j.jaci.2023.06.015
33. Eldeniz FC, Gul Y, Yorulmaz A, Guner SN, Keles S, Reisli I. Evaluation of the 10 warning signs in primary and secondary immunodeficient patients. Front Immunol. (2022) 13:900055. doi: 10.3389/fimmu.222.900055
34. Plamper M, Gohlke B, Woelfle J. PTEN hamartoma tumor syndrome in childhood and adolescence-a comprehensive review and presentation of the German pediatric guideline. Mol Cell Pediatr. (2022) 9:3. doi: 10.1186/s40348-022-00135-1
35. Edfeldt K, Edwards AM, Engkvist O, Günther J, Hartley M, Hulcoop DG, et al. A data science roadmap for open science organizations engaged in early-stage drug discovery. Nat Commun. (2024) 15:5640. doi: 10.1038/s41467-024-49777-x
36. Wang F, Beecy A. Implementing AI models in clinical workflows: a roadmap. BMJ Evid Based Med. (2024). doi: 10.1136/bmjebm-2023-112727
37. Muehlematter UJ, Bluethgen C, Vokinger KN. FDA-cleared artificial intelligence and machine learning-based medical devices and their 510(k) predicate networks. Lancet Digit Health. (2023) 5:e618–26. doi: 10.1016/S2589-7500(23)00126-7
Keywords: APDS, EHR query, AI, inborn errors of immunity, diagnostic delay, IEI diagnosis
Citation: FitzPatrick AM, Chin AT, Nirenberg S, Cunningham-Rundles C, Sacco K, Perlmutter J, Dasso JF, Tsalatsanis A, Maru J, Creech J, Walter JE, Hartog N, Izadi N, Palmucci M, Butte MJ, Loewy K, Relan A and Rider NL (2025) Piloting an automated query and scoring system to facilitate APDS patient identification from health systems. Front. Immunol. 15:1508780. doi: 10.3389/fimmu.2024.1508780
Received: 09 October 2024; Accepted: 20 December 2024;
Published: 21 January 2025.
Edited by:
Maryam Nourizadeh, Tehran University of Medical Sciences, IranReviewed by:
Luis Ignacio Gonzalez-Granado, University Hospital October 12, SpainCopyright © 2025 FitzPatrick, Chin, Nirenberg, Cunningham-Rundles, Sacco, Perlmutter, Dasso, Tsalatsanis, Maru, Creech, Walter, Hartog, Izadi, Palmucci, Butte, Loewy, Relan and Rider. This is an open-access article distributed under the terms of the Creative Commons Attribution License (CC BY). The use, distribution or reproduction in other forums is permitted, provided the original author(s) and the copyright owner(s) are credited and that the original publication in this journal is cited, in accordance with accepted academic practice. No use, distribution or reproduction is permitted which does not comply with these terms.
*Correspondence: Nicholas L. Rider, bmljazcwQHZ0LmVkdQ==
Disclaimer: All claims expressed in this article are solely those of the authors and do not necessarily represent those of their affiliated organizations, or those of the publisher, the editors and the reviewers. Any product that may be evaluated in this article or claim that may be made by its manufacturer is not guaranteed or endorsed by the publisher.
Research integrity at Frontiers
Learn more about the work of our research integrity team to safeguard the quality of each article we publish.