- Business Department, Biocytogen Boston Corporation, Waltham, MA, United States
Precision medicine is revolutionizing the world in combating different disease modalities, including cancer. The concept of personalized treatments is not new, but modeling it into a reality has faced various limitations. The last decade has seen significant improvements in incorporating several novel tools, scientific innovations and governmental support in precision oncology. However, the socio-economic factors and risk-benefit analyses are important considerations. This mini review includes a summary of some commendable milestones, which are not just a series of successes, but also a cautious outlook to the challenges and practical implications of the advancing techno-medical era.
1 Introduction
Oncology therapies are commonly designed to target the highly dysregulated molecular pathways, including Ras/MAPK, Myc, Wnt/β-catenin, TGFβ, PI3K/mTOR, Notch signaling, Hippo pathway, cell cycle, oxidative stress response and/or p53 signaling (1–3). However, therapeutic resistance poses a constant struggle, whether it is ‘intrinsic’ due to genetic/molecular dysregulations or ‘acquired’ due to cancer cells adapting to the cellular changes (4). Tumor heterogeneity, complex tumor microenvironment and genetic predisposition have complicated the treatment options further. Personalized treatment approaches are therefore successfully proving to be the present and future of medicine. Precision Oncology is constantly evolving to acknowledge, accept and utilize every human being’s uniqueness, characterized by a distinct set of genetic make-up (5, 6). As the “one size fits all” theory is challenged at various levels in therapeutic arena, precision medicine has emerged to rescue the unique individual cases (7–9), where common FDA approved chemotherapeutics and/or immunotherapy drugs fail to eliminate the cancer cells (10–13).
As with great power comes bigger economic impact, personalized healthcare requires large sums of investments and some of the underrepresented or minority groups may have limited access to such novel technologies (14). This coincides with Eroom’s law, which describes the ever-slowing rate of drug discovery and applicability with increasing costs associated with it (15). This further widens the gap between research and its practical applications (15). Balancing the resources with medical goals, patient requirements, time involved, and risk assessment is critical.
Although there are multiple tools used to support the personalized approach, attempting to reverse the Eroom’s law, one of the approaches gaining traction is incorporation of artificial intelligence/machine learning (AI/ML) into biotechnological advancements (16). Since 2016, FDA has seen an exponential increase in usage of AI/ML to new oncology clinical trials, in different phases from patient recruitment and precise clinical designs (17) using de-identified electronic health records (18) to data collection and analysis (19–22). These technologies provide a major boost to generating customized treatment plans for specific groups/sub-groups/individuals based on the target mutations. AI/ML algorithms can identify complex patterns and correlations by analyzing large datasets, which may not be possible at human/physician level (22).
Targeting the molecular and cellular characteristics of tumors has been the focus of precision medicine for decades (23). Genetic profiling methods combined with immunophenotyping, transcriptomics and epigenetic analyses assist in de-coding the complex deregulated pathways of tumor microenvironment at a high throughput level, while conventional methods such as FISH (Fluorescence in situ hybridization) and IHC (Immunohistochemistry) are commonly used to detect predictive biomarkers (24). Some common immunotherapeutic drugs targeting PD-1/PD-L1 (nivolumab), CTLA (ipilimumab), TIGIT (tiragolumab), LAG3 (Relatlimab) are well-studied and used by clinicians (25–27). However, in cases concerning rare cancers (such as angiosarcoma (28), metaplastic breast cancer (29)), high risk, relapsed or refractory pediatric cancers (such as Neuroblastoma (30), pediatric brain tumor (31), medulloblastoma, Wilms’ tumor (32, 33)), and resistant cancer sub-types (characterized by overexpression of HER2, Ras/MAPK pathways (34)), customized/personalized cell therapy, gene therapy, immunotherapy, and/or a combination of treatments in a timely manner can successfully aim to prolong symptom free survival in cancer patients (35–41).
2 Current landscape of precision oncology therapy
Modern clinical medicine relies on the 4Ps, serving as pillars to support therapeutic decision making, namely, Predictive, Preventative, Personalized and Participative approach, focusing on robust treatment options in a patient-centric framework (42). Treating the patients with the right medicine at the right time is always the clinical goal, however, the concept of “personalized treatment” has evolved within the last few years. The success and FDA approval of HER2-specific breast cancer targeting drug: trastuzumab in 1998 (43, 44) and BCR-ABL tyrosine kinase inhibitor Chronic Myeloid leukemia drug: Imatinib in 2001 (45) were the first major stepping stones in this field, followed by a wide range of gene-targeting treatment options (46). As cancer is described as both genetic and molecular group of diseases, it became important to encompass other intricate alterations involved, such as epigenetic factors (47), biomarkers (48) and anatomical/histological modifications (49) to understand the disease progression and design individualized “precision medicine” treatments (Figure 1).
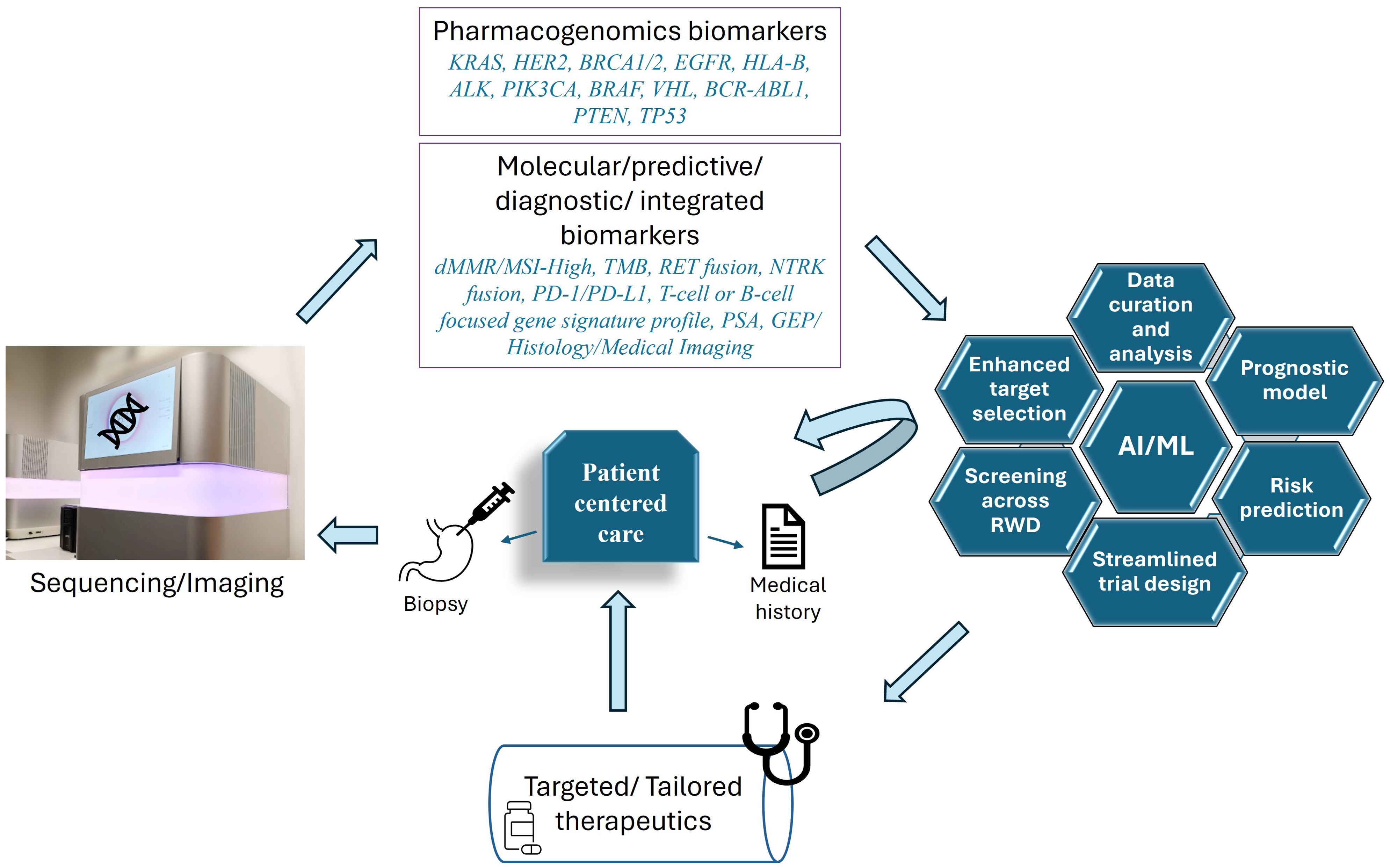
Figure 1. Patient centered personalized care for cancer treatment. To understand the unique molecular make-up of each patient, biopsy samples are analyzed via different sequencing techniques, (including genetic, epigenetic, RNA and ncRNA sequencing) and through medical imaging/histological analysis. These techniques potentially reveal the different pharmacogenomic markers commonly altered in different cancer types, including but not limited to, KRAS (Kristen Rat Sarcoma Viral oncogene homolog), HER2 (human epidermal growth factor receptor 2), BRCA1/2 (breast cancer gene), EGFR (epidermal growth factor receptor), HLA-B (human leukocyte antigen B), ALK (anaplastic lymphoma kinase), PIK3CA (Phosphatidylinositol-4,5-Bisphosphate 3-Kinase Catalytic Subunit Alpha), B-RAF (v-raf murine sarcoma viral oncogene homolog B1), VHL (Von Hippel-Lindau), BCR-ABL1 (breakpoint cluster region and Abelson murine leukemia viral oncogene homolog 1), PTEN (phosphatase and tensin homolog deleted on chromosome 10), TP53 (tumor protein p53) (7, 8, 168). Additionally, different molecular patterns, biomarkers or integrated panels are also analyzed, serving as predictive or diagnostic signatures. These include, but are not limited to, dMMR (deficient DNA mismatch repair)/MSI-high (microsatellite instability-high), TMB (Tumor mutation burden), RET (REarranged during Transfection) genetic fusion, NTRK (neurotrophic tyrosine receptor kinase gene) fusion, PD-1/PD-L1 (Programmed Cell Death Protein 1 and Programmed Cell Death Ligand 1), T-cell or B-cell focused gene signature profile, PSA (prostate-specific antigen), GEP (T cell–inflamed gene expression profile), tumor imaging and histology (34). AI/ML is used for a myriad of functions such as sorting the markers, screening across real world data (RWD), generating prognostic models, risk prediction, selecting specific targets, and testing drug combinations (169). Patient-derived data (based on tested samples and medical history) is curated and streamlined not only for specific clinical trial design, but also to record, statistically analyze, and compare the results (169).
Gene and molecular-targeted therapy (designed to target only cancer cells) and Immunotherapy (used to boost body’s immune system against cancer), are the major approaches used individually or in combination with chemotherapy and/or radiotherapy to treat cancer patients. Within last 20 years, a plethora of drugs, including checkpoint inhibitors, monoclonal/bispecific antibodies, antibody-drug conjugates, chimeric antigen receptor T (CAR-T) cells have been developed to combat the complexities of this disease. The approval of blinatumomab, the first bispecific antibody in 2014 (50) and tisagenlecleucel, the first CAR-T cell therapy in 2017 (51) marked milestones in oncology research. Based on OncoKB (RRID : SCR_014782) (updated June 19, 2024), FDA has approved 186 new targeted therapy drugs since June 1998, out of which 96 are precision oncology drugs.
CAR-T therapy is a highly promising treatment for hematological malignancies. As in most cases it works by using patients’ own T-cells (autologous), this therapy is highly precise and effective (52). Peripheral blood derived T cells are genetically modified to integrate CAR expression cassette into the genome, and CAR proteins are subsequently expressed on surface of T-cells. These modified cells are expanded and infused back into the patients. CAR recognizes specific cancer antigens, forms an immune synapse and lyses the tumor cell by activating granzyme-perforin axis, Fas/Fas ligand pathway and release of cytokines (52). So far, six CAR-T therapies have been FDA approved for use in clinics, targeting two antigens- CD19 and BCMA (53). However, owing to the long term adverse effects of CAR-T, such as cytokine release syndrome and neurological toxicity (54), further research and advancements are moving this field forward, such as integration of CAR with other immune cells - NK/NKT cells, dendritic cells, macrophages, regulatory T cells and B cells (55, 56), which may have the potential to be safer for long-term use and hold high therapeutic potential for clinical use.
Moreover, metabolic dysregulation is a well-known phenomenon in tumors, characterized by accelerated glycolysis, upregulation of lipid and amino acid metabolism, alterations in mitochondrial biogenesis and macromolecule biosynthesis- all of which are considered hallmarks of cancer (57). Various chemotherapeutics targeting the altered molecules in metabolic machinery are well established for clinical use. Some examples include enasidenib for mutated isocitrate dehydrogenase 2 (IDH2) and Ivosidenib for mutated isocitrate dehydrogenase 1 (IDH1) in acute myeloid leukaemia (AML), 5-fluorouracil inhibiting Thymidylate synthase in gastric and breast cancer, and Methotrexate inhibiting dihydrofolate reductase (DHFR) in breast and lung cancer (58, 59). However, activation of DNA repair pathway, induced apoptosis resistance, target alterations, and reprogramming of immune cells by limiting nutrient availability within tumor microenvironment can lead to resistance towards these therapies (60, 61). Understanding the overall picture of the tumor complexity reinforces the concept of combination therapy precisely designed to target the cancer cells from various angles (58, 62).
Traditionally, clinical trials are drug-centered, blinded and randomized to minimize bias. However, due to large variability in patients’ tumor microenvironment, molecular profiles and unique genomic characteristics, the outcomes are far from ideal (63). Therefore, innovative patient- centered trials are now customized to recognize genomic alterations and employ novel biomarker-guided methodologies to address the distinctive needs of patients (64) (Figure 1). A unifying clinical trial framework known as master protocols includes testing multiple drugs in parallel, for patients with same or different types of cancer (65). The major trial designs under master protocols are summarized in Table 1 and are detailed as follows:
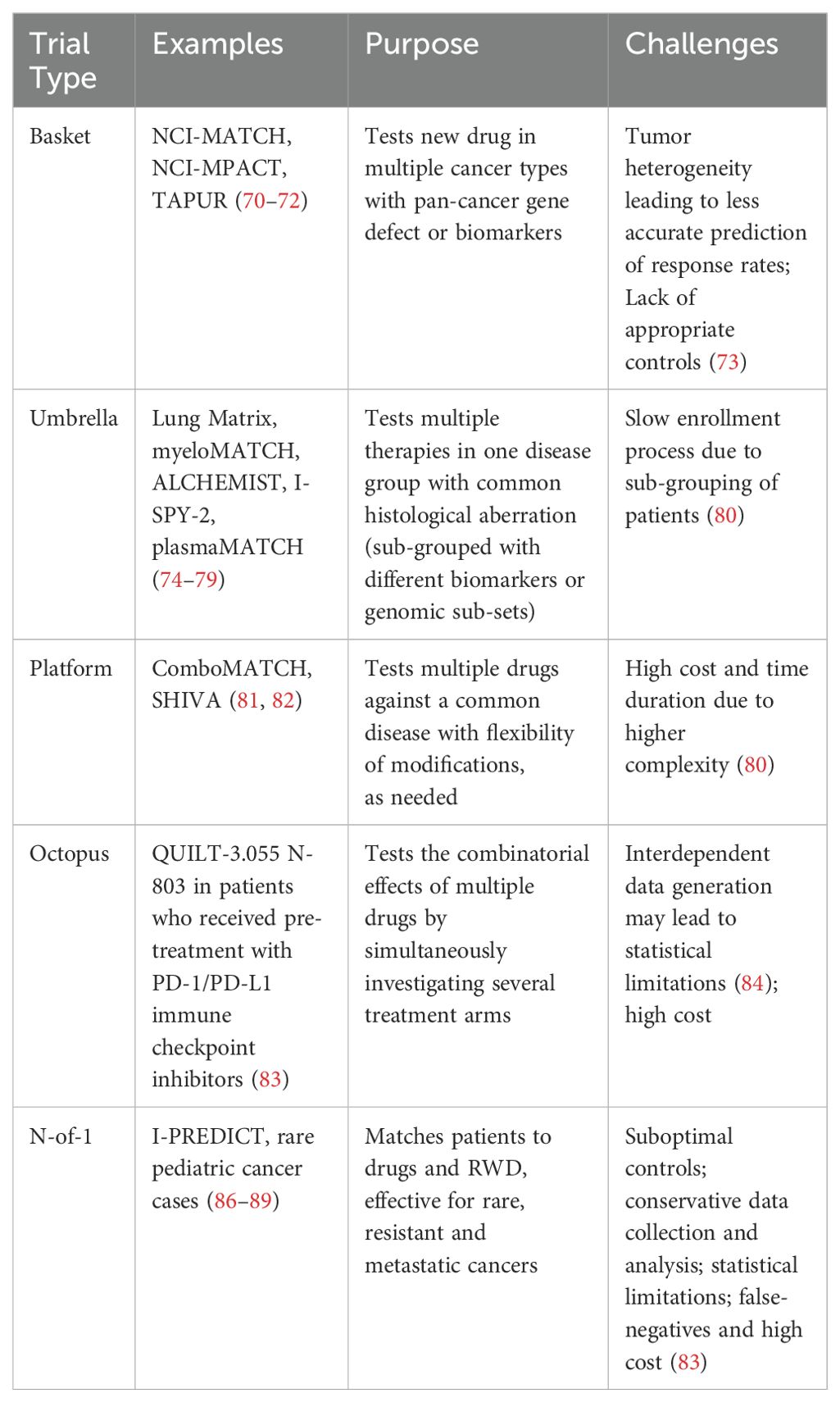
Table 1. This table summarizes the precision oncology clinical trial types, unified as master protocols.
2.1 Basket trials
These include testing of a new drug in patients with common genetic mutation (pan-cancer gene defect) or biomarkers, in more than one cancer types (66). Common examples of drugs targeted to specific genes include Pembrolizumab for tumor mutational burden high (TMB-H) and mismatch repair deficiency/microsatellite instability high (dMMR/MSI-High) (67), and Larotrectinib (68) and entrectinib (69) in tumors with NTRK fusion. Well known basket trials such as NCI‐MATCH (Molecular Analysis for Therapy Choice) (70) and National Cancer Institute’s Molecular Profiling‐Based Assignment of Cancer Therapy (NCI-MPACT) (71), TAPUR (Targeted Agent and Profiling Utilization Registry) (72) were phase 2 trials based on molecular profiling of different cancer sub-types. In some cases, such trials may not accurately predict the response rates due to heterogeneity of the tumors and appropriate control groups may not be available (73).
2.2 Umbrella trials
Test of multiple therapies in one disease group with common histological aberration, stratified in sub-groups based on different biomarker or genomic subsets. Some examples include The Lung Matrix trial (74), Myeloid Malignancies Molecular Analysis for Therapy Choice (myeloMATCH) (75), Adjuvant Lung Cancer Enrichment Marker Identification and Sequencing Trial (ALCHEMIST) (76, 77), Investigation of Serial Studies to Predict Your Therapeutic Response With Imaging And moLecular Analysis 2 (I-SPY-2) (78), and The UK plasma based Molecular profiling of Advanced breast cancer to inform Therapeutic Choices (plasmaMATCH) (79). One challenge with these trials is the requirement of sub-grouping of patients that could slow down the enrollment process in case of rare cancers (80).
2.3 Platform trials
They are also known as multi-arm, multi-stage design trials which include testing multiple drugs against a common disease. Based on the interim analysis, these trials allow changes to the ongoing trial vis à vis addition of a control arm, drug, patient population or even early termination, as needed (80). This flexibility enables platform trials to be confirmatory. Some examples include ComboMATCH (NCI Combination Therapy Platform Trial with Molecular Analysis for Therapy Choice) (81), and SHIVA (Study of Randomized, Molecularly Targeted Therapy Based on Tumor Molecular Profiling versus Conventional Therapy for Advanced Cancer) (82). Since platform trials are large scale and logistically complex, the cost and time duration involved could be high (80).
2.4 Octopus trials
These are completed Phase I/II trials, which evaluate the combinatorial effects of multiple drugs with a common intervention (83). An example is phase IIb multi-cohort study QUILT-3.055, which tests combinations of N-803 (a fusion protein inducing proliferation and activity of natural killer and cytotoxic T-cells) in patients who received pre-treatment with PD-1/PD-L1 immune checkpoint inhibitors (83). Since these trials are multi-arm, data generation could be interdependent, leading to potential statistical limitations (84).
2.5 N-of-1 trial
Randomized and blinded trial conducted in a single patient. These are, in a true sense, personalized trials based on specific biologic characteristics (85). These can be effective in treating rare cancers and to provide objective comparison of different treatments and perform time series analyses (83). Various N-of-1 trials are comprehensively summarized by Gouda et al. (86). Some examples include I-PREDICT study (87), rare pediatric cancer, such as- the case of a 2-year old child with metastatic glomus tumor and activated NOTCH1 (88), and the ALK-fusion positive high grade glioma in a 3-year old (89). However, there are serious considerations to performing these trials, ranging from lack of appropriate control and highly conservative treatment selection to data collection and analysis, statistical limitations, false-negatives and the high cost involved in putting together the infrastructure for each trial (83).
Since the patient-centric biomarker-based studies rely on appropriate detection of the relevant disease indicators, several methods are used to analyze and aid in designing the treatment plans.
3 Onco-precision toolkit
Technological advancements in cancer biology have enabled researchers and clinicians to explore options beyond the common drug targets for patients. Even though the DNA sequencing techniques have been in use since 1970s (90), the most widely accepted next generation sequencing (NGS) was adapted in clinical diagnosis and prognosis within the last decade (91). With the development of clinical applicability of whole genome sequencing (92, 93), whole exome sequencing (WES) (94, 95), RNA-seq (paired with WES (96)) or in single-cell/bulk variations (97)), spatial transcriptomics (98), hybrid capture NGS for targeted oncology panels (99) and comprehensive omics analyses (100, 101), the integration of large-scale genomic data (102) is now possible to drive the personalized treatment approaches. Besides the genetic mutations at DNA and RNA level, several ncRNAs such as miRNA (103, 104), circular RNA (105–107) as well as epigenetic markers (108–111) are being analyzed to comprehensively map individuals at genetic and molecular level. These techniques draw the cellular landscape of tumors and help in discovering biomarkers associated with clinically relevant genomic alterations, as summarized in Figure 1.
Radiomics or high dimensional medical imaging via PET, CT, Ultrasound and MRI (112) for monitoring the tumor characteristics is combined with machine learning to extract the specific features/characteristics of individual tumors, to guide their specific treatment course (113, 114). Theranostics (therapy + diagnostics) utilizes radionuclide linked to targeted biomarkers, which allows diagnosis through targeted imaging (radiomics) and targeted therapy at the same time (115). Some examples include Lutathera (lutetium 177Lu dotatate), the first FDA and EMA approved theranostic drug, which releases radiation to kill cancer cells by binding to cell surface receptors somatostatin on gastroenteropancreatic neuroendocrine tumors (116), and Pluvicto for castration-resistance prostate cancer using Lutetium-177 that targets PSMA on cell surface in prostate cancer (117).
Various ML methods, such as deep neural networks are also used to predict clinical outcomes using the supervised and unsupervised learning models (118, 119) to enhance the efficiency of cancer diagnosis and increase the probability for predictive prognosis. With the use of de-identified electronic health records (EHRs) (120), paired with specific genotypic training data (121) and bioinformatic regression models (122), the auto-encoders can extract intrinsic features of the tumors (118). This high throughput real-world data (RWD) paves the way to deeper understanding of complex biomarkers associated with heterogenous tumor sub-populations, microsatellite instability and tumor mutational burden (TMB) (123–125). Figure 2 summarizes the tools available for personalized cancer treatment for specific population groups, to achieve the clinical goals of treatment and prolonged survival.
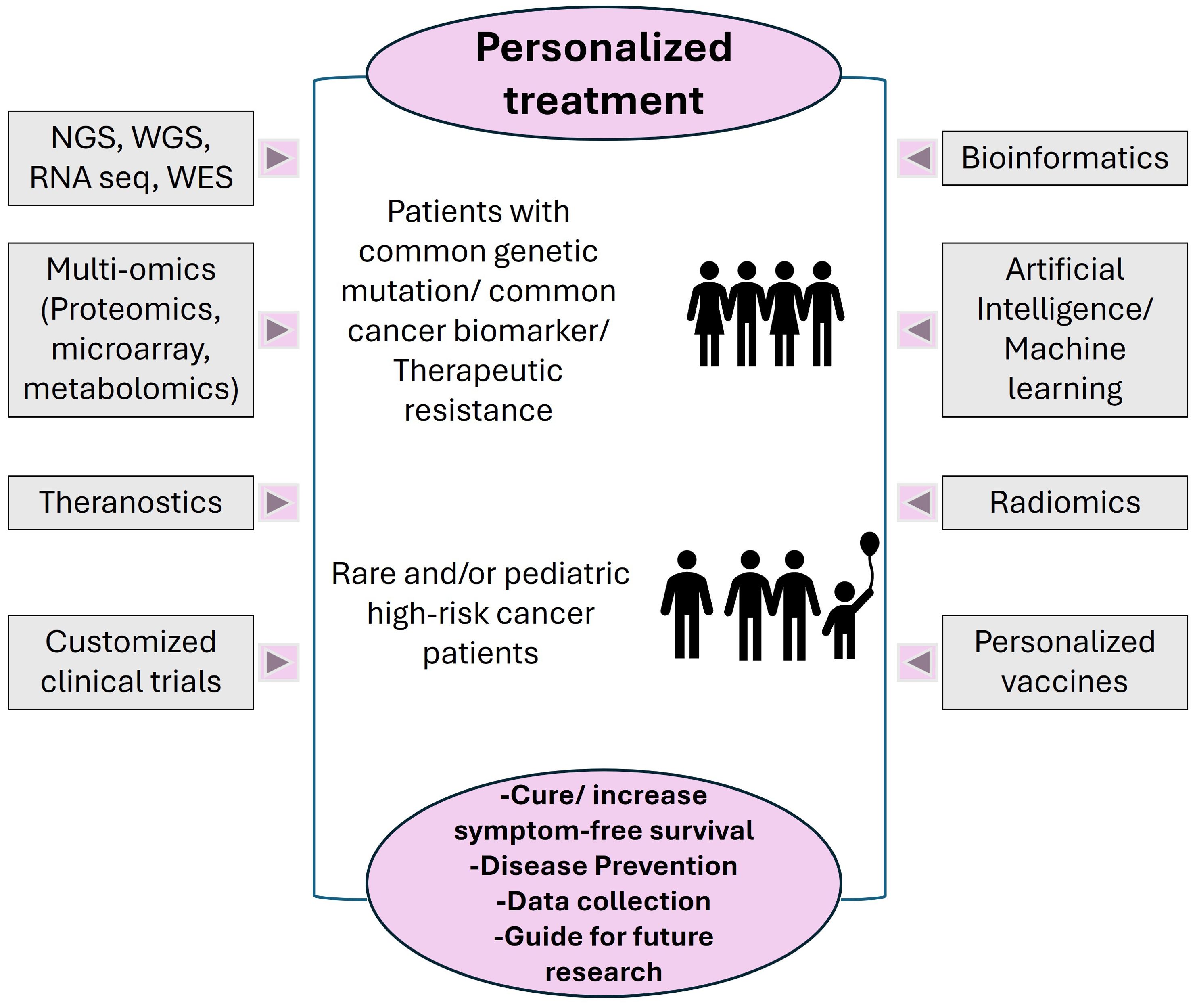
Figure 2. Different Precision Oncology tools utilized for diagnosis, prognosis, data collection and analysis. The target population for personalized treatment includes, but is not limited to, patients that share genetic dysregulation/common biomarkers altered/patients with therapeutic resistance, patients with rare cancer types, pediatric patients with high-risk, relapsed or refractory cancers. The current goals are defined by achievable level of cure or longevity of symptom free survival; some tools such as NGS, preventative personalized vaccines and specific AI/ML technologies can be used for prevention or early precautionary measures. The data collected can also be stored in repositories to guide treatments for other patients, can be used as training data for next generation of advanced technologies and to design novel future medical interventions.
Various governmental organizations such as NCI, NCBI and FDA provide open access public repositories to study the pharmacogenomic pattern of larger population groups with drug response, increased clinical efficacy probability and reduced adverse drug reactions. Some examples include TCGA (reports molecular characterization of 20,000 primary tumors) (RRID : SCR_003193), ClinVar (public archive of human diseases and corresponding drug responses) (RRID : SCR_006169), COSMIC (catalogue of somatic mutations in cancer) (RRID : SCR_002260), PharmKGB (Pharmacogenomics Knowledgebase for genotype-phenotype relationship, genetic variants and drug associated guidelines) (RRID : SCR_025580), Drugbank (for comprehensive drug-target data) (RRID : SCR_002700), FDA’s pharmacogenetic associations and ClinGen (human genetic variants database) (RRID : SCR_014968) (9, 126). Precision FDA is also a free computing platform to analyze large biological datasets and learn from experts in the field.
Utilization of these vast array of tools available carries its fair share of challenges ranging from the cost and time involved in generating the large datasets to managing, storing, aligning and assessing this data, with high quality, accuracy and reproducibility. Aligning multi-reads, incorrect sequence mapping, absence of reference sequences, computational challenges spanning splice or fusion junctions, misalignment and false-positive identification are a few common problems noted with NGS and RNA-seq methods (127, 128). At experimental level, the quality of RNA and DNA extracted from formalin fixed embedded (FFPE) tissues derived from tissue banks may not be the best in some cases for high throughput analyses (129).
Moreover, clonal diversity and tumor heterogeneity is a major challenge in a constantly evolving tumor microenvironment, which can interfere with accurate detection of driver mutations and novel factors leading to resistance towards therapy. Common examples of acquired resistance include splice variants affecting ATP-competitive tyrosine kinase inhibitor binding sites, activating or sub-clonal mutations in PI3K, RAS/MAPK pathways, mutations in “undruggable” genes such as Myc, KRAS and Tp53, FLT3 mutant leukemia (130), and somatic mutations in cancer stem cells (4). Realistically, biopsy at recurrence or relapse is not always possible in case of severe metastasis associated with procedure invasiveness and underlying co-morbidity (131, 132). However, using resources like NCI-MATCH and pairing them with sequential screening tests of samples derived from liquid biopsies, and circulating tumor (ct)-DNA based targeted sequencing (133) based on specific genetic panels can be a way to detect actionable genomic alterations and predict the resistance to adapt customized approaches.
4 Cancer vaccines: a long journey with promising outcome
Cancer vaccines can be categorized into (a) Preventative, such as hepatitis B vaccine and human papilloma virus (HPV) vaccine, administered to reduce the risk of liver cancer and cervical cancer, respectively (134), or (b) Therapeutic, such as Sipuleucel-T against metastatic prostate cancer (134), Nadofaragene firadonevec (Adstiladrin) for early-stage bladder cancer (135), and T-VEC (Imlygic) to treat advanced melanoma (136).
Although the first cancer vaccine trial dates back to 1890s, when William B. Coley used heat-killed streptococcal injections in patients with inoperable sarcomas (137), a major leap forward was in 1959 when Llyod Old showed that BCG infection in mice increased their resistance towards transplanted murine tumor cell lines S-180, carcinoma 755 and Ehlrich ascites (138). The BCG vaccine containing live attenuated Mycobacterium bovis was later approved by FDA for early-stage bladder cancer (139).
As preventative cancer vaccines have limited applicability and efficacy against the plethora of cancer-causing agents, therapeutic vaccines are emerging as an effective means to activate the immune response by enhanced tumor antigen presentation and generating non-exhaustive cytotoxic T cells to improve anti-tumor immunity (140). These vaccines elicit the immune response by recognizing the specific epitopes expressed by tumor cells (140). Though there has been limited success with such vaccines so far, various clinical trials (clinicaltrials.gov) are now focusing on targeting tumor specific antigens (TSAs), which are exclusive to tumors and possess high immunogenicity (141). TSAs can be viral antigens or non-viral neoantigens generated by spontaneous somatic mutations in tumor microenvironment (142, 143). As many neoantigens are unique to either a small sub-population or specific to an individual patient, personalized cancer vaccines are gaining attention for precision targeting.
The most important factors to consider while designing a tailor-made cancer vaccine are: (a) Accurate identification of highly potent and immunogenic neoantigens capable of inducing a robust T cell immunity; (b) Calculative estimation of the probability of TSA-epitopes binding to MHC; (c) Neoantigen prioritization to predict the interaction of TCRs with MHC-neoepitope complex; (d) Selecting appropriate delivery platform for neoantigen based vaccine, which may include autologous dendritic cells (DCs), peptides, DNA, RNA, mRNA or viral vectors (144, 145). Autologous whole tumor cells mixed with immunomodulatory adjuvants, genetically modified autologous tumor cells, autologous cell derived exosomes, DC-tumor cell fusion vaccine, autologous DC-based or DNA/RNA/mRNA-based vaccines are a few examples of the ones undergoing clinical trials for personalized treatment (143, 146, 147). Recombinant viral vectors, such as Great Ape derived adenoviruses (GAd) and modified vaccinia virus Ankara (MVA) also serve as a great tool to trigger effective cytotoxic T cell response, using their intrinsic adjuvant properties (144). Anti-viral vector immunity can serve as a roadblock though, which can lead to ineffective immunity boost at re-administration. This challenge is being eradicated via a ‘heterologous-boost approach’ in various clinical studies, where involving different platforms can provide stronger immune response, examples include GAd - primed with MVA boost (148) or with self-amplifying RNA (149).
We are still in initial stages of personalized cancer vaccine development owing to the complexity and masking skills honed by tumor cells, which makes it difficult to recognize the distinguishing epitopes. A weakened immune system with immunosuppressive proteins expressed on tumor cells (such as PD-L1), loss of TSA expression or spontaneous alterations in antigen processing pathways, could be a few potential challenges (144, 150). Personalized vaccine manufacturing also involves a large cost, unique supply chain and the extensive process involved could cause a lag in timely treatment (144). As scientists are progressing this field forward, there is a need to further refine the cancer vaccine formulation and preparation workflows and make it more accessible to the wider group of patients in need.
5 Discussion
Diligent preclinical steps towards choosing the correct research platform (such as humanized mouse models (151, 152), organoids (153) or organ-on-chip (154)), appropriate drugs (155), and carefully curated experimentation strategies, serve as the foundation of any clinical trial. Undeniably, the molecular framework of tumors needs to be thoroughly studied at a deeper level to align with the required treatment regimens. Understanding resistance mechanisms and adopting alternative approaches is important from the early research steps (4, 156).
Combining these aspects with comprehensive AI-assisted technologies, such as NGS and multi-omics connects the pathway from preclinical (100, 157–160) to ‘personalized’ clinical stages (161). Since generative and multimodal AI models play a major role in patient diagnosis, trial design, planning, patient recruitment, drug delivery, digital monitoring, and data assessment, it is imperative to adopt a precautionary regulatory framework (162). European AI act and FDA have released regulatory policies for the use of AI in medical field (162, 163), however the rules need to be clearer and up to date as the field progresses. Keeping in mind the biases and limitations of large datasets generated from AI-based systems, the risk-benefit scale needs to be fine-tuned. Using real world evidence (RWE) also poses privacy and data confidentiality risks (164), which should be appropriately addressed. Furthermore, using high throughput screening methods for certain subpopulations would need comprehensive training models, absence of which may introduce bias or sub-par results (165). Thorough investigation of medical interventions is needed to be cautious of any false claims from personalized drug developers. Transparent evidence-based information sharing and finding accelerated solutions to unexpected contradictions is required to manage the fragmented regulation in clinical settings (162). Going hand in hand with the ethical considerations, the need for precision medicine outweighs any opposing schools of thought, recognizing that each life is important.
Significant progress has been seen in this field, with the launch of ‘Precision Medicine Initiative’ by Barack Obama in 2015 (166) and the Cancer Moonshot program (167), aiming to bring the public and private sectors together to provide a broader screening, diagnostic, therapeutic and supportive biomedical platform. Though many organizations are focused on developing cutting-edge technologies tailor-made to patients’ needs, we are still many steps away from accessible and affordable personalized healthcare for everyone in need. However, with precision oncology propelling cancer research, there is a gleam of hope for a healthier not-too-distant future.
Author contributions
RC: Conceptualization, Data curation, Formal analysis, Resources, Visualization, Writing – original draft, Writing – review & editing.
Funding
The author(s) declare that no financial support was received for the research, authorship, and/or publication of this article.
Acknowledgments
This is to acknowledge Biocytogen Boston Corporation for their support in publishing this manuscript. Regretfully, this mini review may not have cited all the articles relevant to this topic, due to space limitation.
Conflict of interest
Author RC is employed by the company Biocytogen Boston Corporation.
Publisher’s note
All claims expressed in this article are solely those of the authors and do not necessarily represent those of their affiliated organizations, or those of the publisher, the editors and the reviewers. Any product that may be evaluated in this article, or claim that may be made by its manufacturer, is not guaranteed or endorsed by the publisher.
References
1. Bahar ME, Kim HJ, Kim DR. Targeting the RAS/RAF/MAPK pathway for cancer therapy: from mechanism to clinical studies. Signal Transduct Target Ther. (2023) 8:455. doi: 10.1038/s41392-023-01705-z
2. Peng Y, Wang Y, Zhou C, Mei W, Zeng C. PI3K/akt/mTOR pathway and its role in cancer therapeutics: are we making headway? Front Oncol. (2022) 12:819128. doi: 10.3389/fonc.2022.819128
3. Sanchez-Vega F, Mina M, Armenia J, Chatila WK, Luna A, La KC, et al. Oncogenic signaling pathways in the cancer genome atlas. Cell. (2018) 173:321–37.e10. doi: 10.1016/j.cell.2018.03.035
4. Vasan N, Baselga J, Hyman DM. A view on drug resistance in cancer. Nature. (2019) 575:299–309. doi: 10.1038/s41586-019-1730-1
5. Senft D, Leiserson MDM, Ruppin E, Ronai ZA. Precision oncology: the road ahead. Trends Mol Med. (2017) 23:874–98. doi: 10.1016/j.molmed.2017.08.003
6. Amaral P, Carbonell-Sala S, de la Vega FM, Faial T, Frankish A, Gingeras T, et al. The status of the human gene catalogue. Nature. (2023) 622:41–7. doi: 10.1038/s41586-023-06490-x
7. Cecchin E, Stocco G. Pharmacogenomics and personalized medicine. Genes (Basel). (2020) 11:679. doi: 10.3390/genes11060679
8. PA T, SS M, Jose A, Chandran L, Zachariah SM. Pharmacogenomics: the right drug to the right person. J Clin Med Res. (2009) 1:191–4. doi: 10.4021/jocmr2009.08.1255
9. Zhang G, Zhang Y, Ling Y, Jia J. Web resources for pharmacogenomics. Genomics Proteomics Bioinf. (2015) 13:51–4. doi: 10.1016/j.gpb.2015.01.002
10. Alfarouk KO, Stock CM, Taylor S, Walsh M, Muddathir AK, Verduzco D, et al. Resistance to cancer chemotherapy: failure in drug response from ADME to P-gp. Cancer Cell Int. (2015) 15:71. doi: 10.1186/s12935-015-0221-1
11. Bukowski K, Kciuk M, Kontek R. Mechanisms of multidrug resistance in cancer chemotherapy. Int J Mol Sci. (2020) 21:3233. doi: 10.3390/ijms21093233
12. Nakhoda SK, Olszanski AJ. Addressing recent failures in immuno-oncology trials to guide novel immunotherapeutic treatment strategies. Pharmaceut Med. (2020) 34:83–91. doi: 10.1007/s40290-020-00326-z
13. Blach J, Wojas-Krawczyk K, Nicos M, Krawczyk P. Failure of immunotherapy-the molecular and immunological origin of immunotherapy resistance in lung cancer. Int J Mol Sci. (2021) 22:9030. doi: 10.3390/ijms22169030
14. Shreve JT, Khanani SA, Haddad TC. Artificial intelligence in oncology: current capabilities, future opportunities, and ethical considerations. Am Soc Clin Oncol Educ Book. (2022) 42:1–10. doi: 10.1200/EDBK_350652
15. Scannell JW, Blanckley A, Boldon H, Warrington B. Diagnosing the decline in pharmaceutical R&D efficiency. Nat Rev Drug Discov. (2012) 11:191–200. doi: 10.1038/nrd3681
16. Ringel MS, Scannell JW, Baedeker M, Schulze U. Breaking eroom’s law. Nat Rev Drug Discovery. (2020) 19:833–4. doi: 10.1038/d41573-020-00059-3
17. Niazi SK. The coming of age of AI/ML in drug discovery, development, clinical testing, and manufacturing: the FDA perspectives. Drug Des Devel Ther. (2023) 17:2691–725. doi: 10.2147/DDDT.S424991
18. Ahmed T, Aziz MMA, Mohammed N. De-identification of electronic health record using neural network. Sci Rep. (2020) 10:18600. doi: 10.1038/s41598-020-75544-1
19. Aliper A, Kudrin R, Polykovskiy D, Kamya P, Tutubalina E, Chen S, et al. Prediction of clinical trials outcomes based on target choice and clinical trial design with multi-modal artificial intelligence. Clin Pharmacol Ther. (2023) 114:972–80. doi: 10.1002/cpt.v114.5
20. Harrer S, Shah P, Antony B, Hu J. Artificial intelligence for clinical trial design. Trends Pharmacol Sci. (2019) 40:577–91. doi: 10.1016/j.tips.2019.05.005
21. Zhang B, Zhang L, Chen Q, Jin Z, Liu S, Zhang S. Harnessing artificial intelligence to improve clinical trial design. Commun Med (Lond). (2023) 3:191. doi: 10.1038/s43856-023-00425-3
22. Chopra H, Annu, Shin DK, Munjal K, Priyanka, Dhama K, et al. Revolutionizing clinical trials: the role of AI in accelerating medical breakthroughs. Int J Surg. (2023) 109:4211–20. doi: 10.1097/JS9.0000000000000705
23. Bode AM, Dong Z. Molecular and cellular targets. Mol Carcinog. (2006) 45:422–30. doi: 10.1002/mc.20222
24. Malone ER, Oliva M, Sabatini PJB, Stockley TL, Siu LL. Molecular profiling for precision cancer therapies. Genome Med. (2020) 12:8. doi: 10.1186/s13073-019-0703-1
25. Waldman AD, Fritz JM, Lenardo MJ. A guide to cancer immunotherapy: from T cell basic science to clinical practice. Nat Rev Immunol. (2020) 20:651–68. doi: 10.1038/s41577-020-0306-5
26. Rousseau A, Parisi C, Barlesi F. Anti-TIGIT therapies for solid tumors: a systematic review. ESMO Open. (2023) 8:101184. doi: 10.1016/j.esmoop.2023.101184
27. Su J, Fu Y, Cui Z, Abidin Z, Yuan J, Zhang X, et al. Relatlimab: a novel drug targeting immune checkpoint LAG-3 in melanoma therapy. Front Pharmacol. (2023) 14:1349081. doi: 10.3389/fphar.2023.1349081
28. Tan GF, Chan JY. Towards precision oncology in angiosarcomas using next generation “omic” technologies. Oncotarget. (2021) 12:1953–5. doi: 10.18632/oncotarget.v12i19
29. Bottosso M, Mosele F, Michiels S, Cournede PH, Dogan S, Labaki C, et al. Moving toward precision medicine to predict drug sensitivity in patients with metastatic breast cancer. ESMO Open. (2024) 9:102247. doi: 10.1016/j.esmoop.2024.102247
30. Han JZR, Hastings JF, Phimmachanh M, Fey D, Kolch W, Croucher DR. Personalized medicine for neuroblastoma: moving from static genotypes to dynamic simulations of drug response. J Pers Med. (2021) 11:395. doi: 10.3390/jpm11050395
31. Mochizuki AY, Frost IM, Mastrodimos MB, Plant AS, Wang AC, Moore TB, et al. Precision medicine in pediatric neurooncology: A review. ACS Chem Neurosci. (2018) 9:11–28. doi: 10.1021/acschemneuro.7b00388
32. Suthapot P, Chiangjong W, Chaiyawat P, Choochuen P, Pruksakorn D, Sangkhathat S, et al. Genomics-driven precision medicine in pediatric solid tumors. Cancers (Basel). (2023) 15:1418. doi: 10.3390/cancers15051418
33. Blattner-Johnson M, Jones DTW, Pfaff E. Precision medicine in pediatric solid cancers. Semin Cancer Biol. (2022) 84:214–27. doi: 10.1016/j.semcancer.2021.06.008
34. Duan XP, Qin BD, Jiao XD, Liu K, Wang Z, Zang YS. New clinical trial design in precision medicine: discovery, development and direction. Signal Transduct Target Ther. (2024) 9:57. doi: 10.1038/s41392-024-01760-0
35. Kubo T, Sunami K, Koyama T, Kitami M, Fujiwara Y, Kondo S, et al. The impact of rare cancer and early-line treatments on the benefit of comprehensive genome profiling-based precision oncology. ESMO Open. (2024) 9:102981. doi: 10.1016/j.esmoop.2024.102981
36. Adashek JJ, Kurzrock R. Home-run trials for rare cancers: giving the right drug(s) to the right patients at the right time and in the right place. NPJ Precis Oncol. (2023) 7:129. doi: 10.1038/s41698-023-00487-5
37. McCabe MG, Geoerger B, Chesler L, Hargrave D, Parsons DW, van Tilburg CM, et al. Precision medicine for childhood cancer: current limitations and future perspectives. JCO Precis Oncol. (2024) 8:e2300117. doi: 10.1200/PO.23.00117
38. Lau LMS, Khuong-Quang DA, Mayoh C, Wong M, Barahona P, Ajuyah P, et al. Precision-guided treatment in high-risk pediatric cancers. Nat Med. (2024) 30:1913–22. doi: 10.1038/s41591-024-03044-0
39. Mayoh C, Mao J, Xie J, Tax G, Chow SO, Cadiz R, et al. High-throughput drug screening of primary tumor cells identifies therapeutic strategies for treating children with high-risk cancer. Cancer Res. (2023) 83:2716–32. doi: 10.1158/0008-5472.CAN-22-3702
40. Musyuni P, Bai J, Sheikh A, Vasanthan KS, Jain GK, Abourehab MAS, et al. Precision medicine: Ray of hope in overcoming cancer multidrug resistance. Drug Resist Updat. (2022) 65:100889. doi: 10.1016/j.drup.2022.100889
41. Siemer S, Bauer TA, Scholz P, Breder C, Fenaroli F, Harms G, et al. Targeting cancer chemotherapy resistance by precision medicine-driven nanoparticle-formulated cisplatin. ACS Nano. (2021) 15:18541–56. doi: 10.1021/acsnano.1c08632
42. Marques L, Costa B, Pereira M, Silva A, Santos J, Saldanha L, et al. Advancing precision medicine: A review of innovative in silico approaches for drug development, clinical pharmacology and personalized healthcare. Pharmaceutics. (2024) 16:332. doi: 10.3390/pharmaceutics16030332
43. Slamon DJ, Leyland-Jones B, Shak S, Fuchs H, Paton V, Bajamonde A, et al. Use of chemotherapy plus a monoclonal antibody against HER2 for metastatic breast cancer that overexpresses HER2. N Engl J Med. (2001) 344:783–92. doi: 10.1056/NEJM200103153441101
44. Dawood S, Broglio K, Buzdar AU, Hortobagyi GN, Giordano SH. Prognosis of women with metastatic breast cancer by HER2 status and trastuzumab treatment: an institutional-based review. J Clin Oncol. (2010) 28:92–8. doi: 10.1200/JCO.2008.19.9844
45. Cohen MH, Johnson JR, Pazdur RUS. Food and Drug Administration Drug Approval Summary: conversion of imatinib mesylate (STI571; Gleevec) tablets from accelerated approval to full approval. Clin Cancer Res. (2005) 11:12–9. doi: 10.1158/1078-0432.12.11.1
46. Rusina PV, Falaguera MJ, Romero JMR, McDonagh EM, Dunham I, Ochoa D. Genetic support for FDA-approved drugs over the past decade. Nat Rev Drug Discovery. (2023) 22:864. doi: 10.1038/d41573-023-00158-x
47. Feehley T, O’Donnell CW, Mendlein J, Karande M, McCauley T. Drugging the epigenome in the age of precision medicine. Clin Epigenetics. (2023) 15:6. doi: 10.1186/s13148-022-01419-z
48. Yilmaz BK, Arga KY. Driving precision oncology to clinical practice: the road ahead from biomarker validation to clinical decision systems. OMICS. (2022) 26:358–9. doi: 10.1089/omi.2022.0049
49. Souza da Silva R, Pinto R, Cirnes L, Schmitt F. Tissue management in precision medicine: What the pathologist needs to know in the molecular era. Front Mol Biosci. (2022) 9:983102. doi: 10.3389/fmolb.2022.983102
50. Przepiorka D, Ko CW, Deisseroth A, Yancey CL, Candau-Chacon R, Chiu HJ, et al. FDA approval: blinatumomab. Clin Cancer Res. (2015) 21:4035–9. doi: 10.1158/1078-0432.CCR-15-0612
51. O’Leary MC, Lu X, Huang Y, Lin X, Mahmood I, Przepiorka D, et al. FDA approval summary: tisagenlecleucel for treatment of patients with relapsed or refractory B-cell precursor acute lymphoblastic leukemia. Clin Cancer Res. (2019) 25:1142–6. doi: 10.1158/1078-0432.CCR-18-2035
52. Feins S, Kong W, Williams EF, Milone MC, Fraietta JA. An introduction to chimeric antigen receptor (CAR) T-cell immunotherapy for human cancer. Am J Hematol. (2019) 94:S3–9. doi: 10.1002/ajh.v94.S1
53. Chen Y-J, Abila B, Mostafa Kamel Y. CAR-T: what is next? Cancers. (2023) 15:663. doi: 10.3390/cancers15030663
54. Brudno JN, Kochenderfer JN. Recent advances in CAR T-cell toxicity: Mechanisms, manifestations and management. Blood Rev. (2019) 34:45–55. doi: 10.1016/j.blre.2018.11.002
55. Ebrahimiyan H, Tamimi A, Shokoohian B, Minaei N, Memarnejadian A, Hossein-Khannazer N, et al. Novel insights in CAR-NK cells beyond CAR-T cell technology; promising advantages. Int Immunopharmacol. (2022) 106:108587. doi: 10.1016/j.intimp.2022.108587
56. Patel S, Burga RA, Powell AB, Chorvinsky EA, Hoq N, McCormack SE, et al. Beyond CAR T cells: other cell-based immunotherapeutic strategies against cancer. Front Oncol. (2019) 9:196. doi: 10.3389/fonc.2019.00196
57. Robey RB, Weisz J, Kuemmerle NB, Salzberg AC, Berg A, Brown DG, et al. Metabolic reprogramming and dysregulated metabolism: cause, consequence and/or enabler of environmental carcinogenesis? Carcinogenesis. (2015) 36 Suppl 1:S203–31. doi: 10.1093/carcin/bgv037
58. Stine ZE, Schug ZT, Salvino JM, Dang CV. Targeting cancer metabolism in the era of precision oncology. Nat Rev Drug Discovery. (2022) 21:141–62. doi: 10.1038/s41573-021-00339-6
59. Luengo A, Gui DY, Vander Heiden MG. Targeting metabolism for cancer therapy. Cell Chem Biol. (2017) 24:1161–80. doi: 10.1016/j.chembiol.2017.08.028
60. Khalaf K, Hana D, Chou JT, Singh C, Mackiewicz A, Kaczmarek M. Aspects of the tumor microenvironment involved in immune resistance and drug resistance. Front Immunol. (2021) 12:656364. doi: 10.3389/fimmu.2021.656364
61. Xia L, Oyang L, Lin J, Tan S, Han Y, Wu N, et al. The cancer metabolic reprogramming and immune response. Mol Cancer. (2021) 20:28. doi: 10.1186/s12943-021-01316-8
62. Zheng P, Lin Z, Ding Y, Duan S. Targeting the dynamics of cancer metabolism in the era of precision oncology. Metabolism. (2023) 145:155615. doi: 10.1016/j.metabol.2023.155615
63. Sotelo-Rodriguez DC, Ruiz-Patino A, Ricaurte L, Arrieta O, Zatarain-Barron ZL, Cardona AF. Challenges and shifting paradigms in clinical trials in oncology: the case for immunological and targeted therapies. Ecancermedicalscience. (2019) 13:936. doi: 10.3332/ecancer.2019.936
64. Tong A, Scholes-Robertson N, Hawley C, Viecelli AK, Carter SA, Levin A, et al. Patient-centred clinical trial design. Nat Rev Nephrol. (2022) 18:514–23. doi: 10.1038/s41581-022-00585-w
65. Lu CC, Li XN, Broglio K, Bycott P, Jiang Q, Li X, et al. Practical considerations and recommendations for master protocol framework: basket, umbrella and platform trials. Ther Innov Regul Sci. (2021) 55:1145–54. doi: 10.1007/s43441-021-00315-7
66. Park JJH, Hsu G, Siden EG, Thorlund K, Mills EJ. An overview of precision oncology basket and umbrella trials for clinicians. CA Cancer J Clin. (2020) 70:125–37. doi: 10.3322/caac.21600
67. Marabelle A, Le DT, Ascierto PA, Di Giacomo AM, De Jesus-Acosta A, Delord JP, et al. Efficacy of pembrolizumab in patients with noncolorectal high microsatellite instability/mismatch repair-deficient cancer: results from the phase II KEYNOTE-158 study. J Clin Oncol. (2020) 38:1–10. doi: 10.1200/JCO.19.02105
68. Drilon A, Laetsch TW, Kummar S, DuBois SG, Lassen UN, Demetri GD, et al. Efficacy of larotrectinib in TRK fusion-positive cancers in adults and children. N Engl J Med. (2018) 378:731–9. doi: 10.1056/NEJMoa1714448
69. Doebele RC, Drilon A, Paz-Ares L, Siena S, Shaw AT, Farago AF, et al. Entrectinib in patients with advanced or metastatic NTRK fusion-positive solid tumours: integrated analysis of three phase 1-2 trials. Lancet Oncol. (2020) 21:271–82. doi: 10.1016/S1470-2045(19)30691-6
70. Murciano-Goroff YR, Drilon A, Stadler ZK. The NCI-MATCH: A national, collaborative precision oncology trial for diverse tumor histologies. Cancer Cell. (2021) 39:22–4. doi: 10.1016/j.ccell.2020.12.021
71. Chen AP, Kummar S, Moore N, Rubinstein LV, Zhao Y, Williams PM, et al. Molecular profiling-based assignment of cancer therapy (NCI-MPACT): A randomized multicenter phase II trial. JCO Precis Oncol. (2021) 5:133–44. doi: 10.1200/PO.20.00372
72. Mangat PK, Halabi S, Bruinooge SS, Garrett-Mayer E, Alva A, Janeway KA, et al. Rationale and design of the targeted agent and profiling utilization registry (TAPUR) study. JCO Precis Oncol. (2018) 2018:1–14. doi: 10.1200/PO.18.00122
73. Haslam A, Olivier T, Tuia J, Prasad V. Umbrella review of basket trials testing a drug in tumors with actionable genetic biomarkers. BMC Cancer. (2023) 23:46. doi: 10.1186/s12885-022-10421-w
74. Middleton G, Fletcher P, Popat S, Savage J, Summers Y, Greystoke A, et al. The National Lung Matrix Trial of personalized therapy in lung cancer. Nature. (2020) 583:807–12. doi: 10.1038/s41586-020-2481-8
75. Song IW, Vo HH, Chen YS, Baysal MA, Kahle M, Johnson A, et al. Precision oncology: evolving clinical trials across tumor types. Cancers (Basel). (2023) 15:1967. doi: 10.3390/cancers15071967
76. Alden RS, Mandrekar SJ, Oxnard GR. Designing a definitive trial for adjuvant targeted therapy in genotype defined lung cancer: the ALCHEMIST trials. Chin Clin Oncol. (2015) 4:37. doi: 10.3978/j.issn.2304-3865.2015.09.03
77. Govindan R, Mandrekar SJ, Gerber DE, Oxnard GR, Dahlberg SE, Chaft J, et al. ALCHEMIST trials: A golden opportunity to transform outcomes in early-stage non-small cell lung cancer. Clin Cancer Res. (2015) 21:5439–44. doi: 10.1158/1078-0432.CCR-15-0354
78. Consortium IST, Yee D, DeMichele AM, Yau C, Isaacs C, Symmans WF, et al. Association of event-free and distant recurrence-free survival with individual-level pathologic complete response in neoadjuvant treatment of stages 2 and 3 breast cancer: three-year follow-up analysis for the I-SPY2 adaptively randomized clinical trial. JAMA Oncol. (2020) 6:1355–62. doi: 10.1001/jamaoncol.2020.2535
79. Turner NC, Kingston B, Kilburn LS, Kernaghan S, Wardley AM, Macpherson IR, et al. Circulating tumour DNA analysis to direct therapy in advanced breast cancer (plasmaMATCH): a multicentre, multicohort, phase 2a, platform trial. Lancet Oncol. (2020) 21:1296–308. doi: 10.1016/S1470-2045(20)30444-7
80. Renfro LA, Sargent DJ. Statistical controversies in clinical research: basket trials, umbrella trials, and other master protocols: a review and examples. Ann Oncol. (2017) 28:34–43. doi: 10.1093/annonc/mdw413
81. Meric-Bernstam F, Ford JM, O’Dwyer PJ, Shapiro GI, McShane LM, Freidlin B, et al. National cancer institute combination therapy platform trial with molecular analysis for therapy choice (ComboMATCH). Clin Cancer Res. (2023) 29:1412–22. doi: 10.1158/1078-0432.CCR-22-3334
82. Le Tourneau C, Delord J-P, Gonçalves A, Gavoille C, Dubot C, Isambert N, et al. Molecularly targeted therapy based on tumour molecular profiling versus conventional therapy for advanced cancer (SHIVA): a multicentre, open-label, proof-of-concept, randomised, controlled phase 2 trial. Lancet Oncol. (2015) 16:1324–34. doi: 10.1016/S1470-2045(15)00188-6
83. Fountzilas E, Tsimberidou AM, Vo HH, Kurzrock R. Clinical trial design in the era of precision medicine. Genome Med. (2022) 14:101. doi: 10.1186/s13073-022-01102-1
84. Banerjee S, Giannone G, Clamp AR, Ennis DP, Glasspool RM, Herbertson R, et al. Efficacy and safety of weekly paclitaxel plus vistusertib vs paclitaxel alone in patients with platinum-resistant ovarian high-grade serous carcinoma: the OCTOPUS multicenter, phase 2, randomized clinical trial. JAMA Oncol. (2023) 9:675–82. doi: 10.1001/jamaoncol.2022.7966
85. Selker HP, Dulko D, Greenblatt DJ, Palm M, Trinquart L. The use of N-of-1 trials to generate real-world evidence for optimal treatment of individuals and populations. J Clin Transl Sci. (2023) 7:e203. doi: 10.1017/cts.2023.604
86. Gouda MA, Buschhorn L, Schneeweiss A, Wahida A, Subbiah V. N-of-1 trials in cancer drug development. Cancer Discovery. (2023) 13:1301–9. doi: 10.1158/2159-8290.CD-22-1377
87. Sicklick JK, Kato S, Okamura R, Patel H, Nikanjam M, Fanta PT, et al. Molecular profiling of advanced Malignancies guides first-line N-of-1 treatments in the I-PREDICT treatment-naive study. Genome Med. (2021) 13:155. doi: 10.1186/s13073-021-00969-w
88. Zhang E, Miller A, Clinton C, DeSmith K, Voss SD, Aster JC, et al. Gamma secretase inhibition for a child with metastatic glomus tumor and activated NOTCH1. JCO Precis Oncol. (2022) 6:e2200099. doi: 10.1200/PO.22.00099
89. Bagchi A, Orr BA, Campagne O, Dhanda S, Nair S, Tran Q, et al. Lorlatinib in a child with ALK-fusion-positive high-grade glioma. N Engl J Med. (2021) 385:761–3. doi: 10.1056/NEJMc2101264
90. Heather JM, Chain B. The sequence of sequencers: The history of sequencing DNA. Genomics. (2016) 107:1–8. doi: 10.1016/j.ygeno.2015.11.003
91. Guan YF, Li GR, Wang RJ, Yi YT, Yang L, Jiang D, et al. Application of next-generation sequencing in clinical oncology to advance personalized treatment of cancer. Chin J Cancer. (2012) 31:463–70. doi: 10.5732/cjc.012.10216
92. Khan Z, Jung M, Crow M, Mohindra R, Maiya V, Kaminker JS, et al. Whole genome sequencing across clinical trials identifies rare coding variants in GPR68 associated with chemotherapy-induced peripheral neuropathy. Genome Med. (2023) 15:45. doi: 10.1186/s13073-023-01193-4
93. Xiao W, Ren L, Chen Z, Fang LT, Zhao Y, Lack J, et al. Toward best practice in cancer mutation detection with whole-genome and whole-exome sequencing. Nat Biotechnol. (2021) 39:1141–50. doi: 10.1038/s41587-021-00994-5
94. Menzel M, Ossowski S, Kral S, Metzger P, Horak P, Marienfeld R, et al. Multicentric pilot study to standardize clinical whole exome sequencing (WES) for cancer patients. NPJ Precis Oncol. (2023) 7:106. doi: 10.1038/s41698-023-00457-x
95. Rennert H, Eng K, Zhang T, Tan A, Xiang J, Romanel A, et al. Development and validation of a whole-exome sequencing test for simultaneous detection of point mutations, indels and copy-number alterations for precision cancer care. NPJ Genom Med. (2016) 1:16019–. doi: 10.1038/npjgenmed.2016.19
96. Sun G, Chen J, Liang J, Yin X, Zhang M, Yao J, et al. Integrated exome and RNA sequencing of TFE3-translocation renal cell carcinoma. Nat Commun. (2021) 12:5262. doi: 10.1038/s41467-021-25618-z
97. Zhang Z, Wang ZX, Chen YX, Wu HX, Yin L, Zhao Q, et al. Integrated analysis of single-cell and bulk RNA sequencing data reveals a pan-cancer stemness signature predicting immunotherapy response. Genome Med. (2022) 14:45. doi: 10.1186/s13073-022-01050-w
98. Shi W, Zhang J, Huang S, Fan Q, Cao J, Zeng J, et al. Next-generation sequencing-based spatial transcriptomics: A perspective from barcoding chemistry. JACS Au. (2024) 4:1723–43. doi: 10.1021/jacsau.4c00118
99. Beaubier N, Tell R, Lau D, Parsons JR, Bush S, Perera J, et al. Clinical validation of the tempus xT next-generation targeted oncology sequencing assay. Oncotarget. (2019) 10:2384–96. doi: 10.18632/oncotarget.v10i24
100. Heo YJ, Hwa C, Lee GH, Park JM, An JY. Integrative multi-omics approaches in cancer research: from biological networks to clinical subtypes. Mol Cells. (2021) 44:433–43. doi: 10.14348/molcells.2021.0042
101. Aldea M, Friboulet L, Apcher S, Jaulin F, Mosele F, Sourisseau T, et al. Precision medicine in the era of multi-omics: can the data tsunami guide rational treatment decision? ESMO Open. (2023) 8:101642. doi: 10.1016/j.esmoop.2023.101642
102. Sosinsky A, Ambrose J, Cross W, Turnbull C, Henderson S, Jones L, et al. Insights for precision oncology from the integration of genomic and clinical data of 13,880 tumors from the 100,000 Genomes Cancer Programme. Nat Med. (2024) 30:279–89. doi: 10.1038/s41591-023-02682-0
103. Dreussi E, Biason P, Toffoli G, Cecchin E. miRNA pharmacogenomics: the new frontier for personalized medicine in cancer? Pharmacogenomics. (2012) 13:1635–50. doi: 10.2217/pgs.12.147
104. Mishra PJ, Bertino JR. MicroRNA polymorphisms: the future of pharmacogenomics, molecular epidemiology and individualized medicine. Pharmacogenomics. (2009) 10:399–416. doi: 10.2217/14622416.10.3.399
105. Garcia-Rodriguez JL, Korsgaard U, Ahmadov U, Jarlstad Olesen MT, Dietrich KG, Hansen EB, et al. Spatial profiling of circular RNAs in cancer reveals high expression in muscle and stromal cells. Cancer Res. (2023) 83:3340–53. doi: 10.1158/0008-5472.CAN-23-0748
106. Dong Y, Gao Q, Chen Y, Zhang Z, Du Y, Liu Y, et al. Identification of CircRNA signature associated with tumor immune infiltration to predict therapeutic efficacy of immunotherapy. Nat Commun. (2023) 14:2540. doi: 10.1038/s41467-023-38232-y
107. Pisignano G, Michael DC, Visal TH, Pirlog R, Ladomery M, Calin GA. Going circular: history, present, and future of circRNAs in cancer. Oncogene. (2023) 42:2783–800. doi: 10.1038/s41388-023-02780-w
108. Beltran-Garcia J, Osca-Verdegal R, Mena-Molla S, Garcia-Gimenez JL. Epigenetic IVD tests for personalized precision medicine in cancer. Front Genet. (2019) 10:621. doi: 10.3389/fgene.2019.00621
109. Yu X, Zhao H, Wang R, Chen Y, Ouyang X, Li W, et al. Cancer epigenetics: from laboratory studies and clinical trials to precision medicine. Cell Death Discovery. (2024) 10:28. doi: 10.1038/s41420-024-01803-z
110. Raj S, Kumar D. Early epigenetic markers for precision medicine. Prog Mol Biol Transl Sci. (2023) 198:153–64. doi: 10.1016/bs.pmbts.2023.02.003
111. Gong Z, Bi C, Liu W, Luo B. Comprehensive analysis based on the TCGA database identified SCIN as a key DNA methylation-driver gene in epstein-barr virus-associated gastric cancer. Biochem Genet. (2024). doi: 10.1007/s10528-024-10702-y
112. Aerts HJ, Velazquez ER, Leijenaar RT, Parmar C, Grossmann P, Carvalho S, et al. Decoding tumour phenotype by noninvasive imaging using a quantitative radiomics approach. Nat Commun. (2014) 5:4006. doi: 10.1038/ncomms5006
113. Lambin P, Leijenaar RTH, Deist TM, Peerlings J, de Jong EEC, van Timmeren J, et al. Radiomics: the bridge between medical imaging and personalized medicine. Nat Rev Clin Oncol. (2017) 14:749–62. doi: 10.1038/nrclinonc.2017.141
114. Jiang X, Hu Z, Wang S, Zhang Y. Deep learning for medical image-based cancer diagnosis. Cancers (Basel). (2023) 15:3608. doi: 10.3390/cancers15143608
115. Navalkissoor S, Gnanasegaran G, Baum R. Theranostics and precision medicine special feature. Br J Radiol. (2018) 91:20189004. doi: 10.1259/bjr.20189004
116. Urso L, Nieri A, Uccelli L, Castello A, Artioli P, Cittanti C, et al. Lutathera((R)) orphans: state of the art and future application of radioligand therapy with (177)Lu-DOTATATE. Pharmaceutics. (2023) 15:1110. doi: 10.3390/pharmaceutics15041110
117. Keam SJ. Lutetium lu 177 vipivotide tetraxetan: first approval. Mol Diagn Ther. (2022) 26:467–75. doi: 10.1007/s40291-022-00594-2
118. Zhang X, Venkatachalapathy S, Paysan D, Schaerer P, Tripodo C, Uhler C, et al. Unsupervised representation learning of chromatin images identifies changes in cell state and tissue organization in DCIS. Nat Commun. (2024) 15:6112. doi: 10.1038/s41467-024-50285-1
119. Melarkode N, Srinivasan K, Qaisar SM, Plawiak P. AI-powered diagnosis of skin cancer: A contemporary review, open challenges and future research directions. Cancers (Basel). (2023) 15:1183. doi: 10.3390/cancers15041183
120. Chen JL, Stumpe MC, Cohen E. Evolving from discrete molecular data integrations to actionable molecular insights within the electronic health record. JCO Clin Cancer Inform. (2024) 8:e2400011. doi: 10.1200/CCI.24.00011
121. Ichiuji M, Asakura L, Cain C, Aye N, Kolevska T, Chen D, et al. Improving routine use of clinical pathway decision support through integration of an EHR with a clinical library resource designed to provide evidence-based guidance within oncology workflows. BMC Health Serv Res. (2024) 24:560. doi: 10.1186/s12913-024-11018-8
122. Tang A, Woldemariam S, Roger J, Sirota M. Translational bioinformatics to enable precision medicine for all: elevating equity across molecular, clinical, and digital realms. Yearb Med Inform. (2022) 31:106–15. doi: 10.1055/s-0042-1742513
123. Christopoulos P, Schlenk R, Kazdal D, Blasi M, Lennerz J, Shah R, et al. Real-world data for precision cancer medicine-A European perspective. Genes Chromosomes Cancer. (2023) 62:557–63. doi: 10.1002/gcc.23135
124. Lewis JRR, Kerridge I, Lipworth W. Use of real-world data for the research, development, and evaluation of oncology precision medicines. JCO Precis Oncol. (2017) 1:1–11. doi: 10.1200/PO.17.00157
125. Verkerk K, Voest EE. Generating and using real-world data: A worthwhile uphill battle. Cell. (2024) 187:1636–50. doi: 10.1016/j.cell.2024.02.012
126. Pritchard D, Patel JN, Stephens LE, McLeod HL. Comparison of FDA table of pharmacogenetic associations and clinical pharmacogenetics implementation consortium guidelines. Am J Health Syst Pharm. (2022) 79:993–1005. doi: 10.1093/ajhp/zxac064
127. Han Y, Gao S, Muegge K, Zhang W, Zhou B. Advanced applications of RNA sequencing and challenges. Bioinform Biol Insights. (2015) 9:29–46. doi: 10.4137/BBI.S28991
128. Kulkarni P, Frommolt P. Challenges in the setup of large-scale next-generation sequencing analysis workflows. Comput Struct Biotechnol J. (2017) 15:471–7. doi: 10.1016/j.csbj.2017.10.001
129. Cazzato G, Caporusso C, Arezzo F, Cimmino A, Colagrande A, Loizzi V, et al. Formalin-fixed and paraffin-embedded samples for next generation sequencing: problems and solutions. Genes (Basel). (2021) 12:1472. doi: 10.3390/genes12101472
130. McMahon CM, Ferng T, Canaani J, Wang ES, Morrissette JJD, Eastburn DJ, et al. Clonal selection with RAS pathway activation mediates secondary clinical resistance to selective FLT3 inhibition in acute myeloid leukemia. Cancer Discovery. (2019) 9:1050–63. doi: 10.1158/2159-8290.CD-18-1453
131. Foukakis T, Astrom G, Lindstrom L, Hatschek T, Bergh J. When to order a biopsy to characterise a metastatic relapse in breast cancer. Ann Oncol. (2012) 23 Suppl 10:x349–53. doi: 10.1093/annonc/mds297
132. Falagario UG, Abbadi A, Remmers S, Bjornebo L, Bogdanovic D, Martini A, et al. Biochemical recurrence and risk of mortality following radiotherapy or radical prostatectomy. JAMA Netw Open. (2023) 6:e2332900. doi: 10.1001/jamanetworkopen.2023.32900
133. Cescon DW, Bratman SV, Chan SM, Siu LL. Circulating tumor DNA and liquid biopsy in oncology. Nat Cancer. (2020) 1:276–90. doi: 10.1038/s43018-020-0043-5
134. Finn OJ. The dawn of vaccines for cancer prevention. Nat Rev Immunol. (2018) 18:183–94. doi: 10.1038/nri.2017.140
135. Lee A. Nadofaragene firadenovec: first approval. Drugs. (2023) 83:353–7. doi: 10.1007/s40265-023-01846-z
136. Pol J, Kroemer G, Galluzzi L. First oncolytic virus approved for melanoma immunotherapy. Oncoimmunology. (2016) 5:e1115641. doi: 10.1080/2162402X.2015.1115641
137. McCarthy EF. The toxins of William B. Coley and the treatment of bone and soft-tissue sarcomas. Iowa Orthop J. (2006) 26:154–8.
138. Old LJ, Clarke DA, Benacerraf B. Effect of Bacillus Calmette-Guerin infection on transplanted tumours in the mouse. Nature. (1959) 184:291–2. doi: 10.1038/184291a0
139. Cardillo F, Bonfim M, da Silva Vasconcelos Sousa P, Mengel J, Ribeiro Castello-Branco LR, Pinho RT. Bacillus calmette-guerin immunotherapy for cancer. Vaccines (Basel). (2021) 9:439. doi: 10.3390/vaccines9050439
140. Saxena M, van der Burg SH, Melief CJM, Bhardwaj N. Therapeutic cancer vaccines. Nat Rev Cancer. (2021) 21:360–78. doi: 10.1038/s41568-021-00346-0
141. Janes ME, Gottlieb AP, Park KS, Zhao Z, Mitragotri S. Cancer vaccines in the clinic. Bioeng Transl Med. (2024) 9:e10588. doi: 10.1002/btm2.10588
142. Lin MJ, Svensson-Arvelund J, Lubitz GS, Marabelle A, Melero I, Brown BD, et al. Cancer vaccines: the next immunotherapy frontier. Nat Cancer. (2022) 3:911–26. doi: 10.1038/s43018-022-00418-6
143. Li X, You J, Hong L, Liu W, Guo P, Hao X. Neoantigen cancer vaccines: a new star on the horizon. Cancer Biol Med. (2023) 21:274–311. doi: 10.20892/j.issn.2095-3941.2023.0395
144. Secli L, Leoni G, Ruzza V, Siani L, Cotugno G, Scarselli E, et al. Personalized cancer vaccines go viral: viral vectors in the era of personalized immunotherapy of cancer. Int J Mol Sci. (2023) 24:16591. doi: 10.3390/ijms242316591
145. Biswas N, Chakrabarti S, Padul V, Jones LD, Ashili S. Designing neoantigen cancer vaccines, trials, and outcomes. Front Immunol. (2023) 14:1105420. doi: 10.3389/fimmu.2023.1105420
146. D'Alise AM, Brasu N, De Intinis C, Leoni G, Russo V, Langone F, et al. Adenoviral-based vaccine promotes neoantigen-specific CD8(+) T cell stemness and tumor rejection. Sci Transl Med. (2022) 14:eabo7604. 10.1126/scitranslmed.abo7604
147. Fritah H, Rovelli R, Chiang CL, Kandalaft LE. The current clinical landscape of personalized cancer vaccines. Cancer Treat Rev. (2022) 106:102383. doi: 10.1016/j.ctrv.2022.102383
148. Bechter O, D'Alise AM, Leoni G, Cotugno G, Siani L, Vitale R, et al. Abstract LB196: NOUS-PEV, a personalized cancer immunotherapy targeting neoantigens, induces long lasting, tumor infiltrating memory T cells. Cancer Res. (2023) 83:LB196–LB. doi: 10.1158/1538-7445.AM2023-LB196
149. Palmer CD, Rappaport AR, Davis MJ, Hart MG, Scallan CD, Hong SJ, et al. Individualized, heterologous chimpanzee adenovirus and self-amplifying mRNA neoantigen vaccine for advanced metastatic solid tumors: phase 1 trial interim results. Nat Med. (2022) 28:1619–29. doi: 10.1038/s41591-022-01937-6
150. Shemesh CS, Hsu JC, Hosseini I, Shen BQ, Rotte A, Twomey P, et al. Personalized cancer vaccines: clinical landscape, challenges, and opportunities. Mol Ther. (2021) 29:555–70. doi: 10.1016/j.ymthe.2020.09.038
151. Yan P, Su Y, Shang C, Zhou X, Yang Y, An W, et al. The establishment of humanized IL-4/IL-4RA mouse model by gene editing and efficacy evaluation. Immunobiology. (2020) 225:151998. doi: 10.1016/j.imbio.2020.151998
152. Xie X, Niu Z, Wang L, Zhou X, Yu X, Jing H, et al. Humanized CD36 (hCD36) mouse model supports the preclinical evaluation of therapeutic candidates targeting CD36. Exp Anim. (2023) 72:535–45. doi: 10.1538/expanim.23-0021
153. Fang Z, Li P, Du F, Shang L, Li L. The role of organoids in cancer research. Exp Hematol Oncol. (2023) 12:69. doi: 10.1186/s40164-023-00433-y
154. Regmi S, Poudel C, Adhikari R, Luo KQ. Applications of microfluidics and organ-on-a-chip in cancer research. Biosensors (Basel). (2022) 12:18–25. doi: 10.3390/bios12070459
155. Chen EY, Raghunathan V, Prasad V. An overview of cancer drugs approved by the US food and drug administration based on the surrogate end point of response rate. JAMA Intern Med. (2019) 179:915–21. doi: 10.1001/jamainternmed.2019.0583
156. Chhabra R, Nanjundan M. Lysophosphatidic acid reverses Temsirolimus-induced changes in lipid droplets and mitochondrial networks in renal cancer cells. PloS One. (2020) 15:e0233887. doi: 10.1371/journal.pone.0233887
157. Chhabra R, Guergues J, Wohlfahrt J, Rockfield S, Espinoza Gonzalez P, Rego S, et al. Deregulated expression of the 14q32 miRNA cluster in clear cell renal cancer cells. Front Oncol. (2023) 13:1048419. doi: 10.3389/fonc.2023.1048419
158. Chhabra R, Rockfield S, Guergues J, Nadeau OW, Hill R, Stevens SM Jr., et al. Global miRNA/proteomic analyses identify miRNAs at 14q32 and 3p21, which contribute to features of chronic iron-exposed fallopian tube epithelial cells. Sci Rep. (2021) 11:6270. doi: 10.1038/s41598-021-85342-y
159. Du M, Gu D, Xin J, Peters U, Song M, Cai G, et al. Integrated multi-omics approach to distinct molecular characterization and classification of early-onset colorectal cancer. Cell Rep Med. (2023) 4:100974. doi: 10.1016/j.xcrm.2023.100974
160. Liu Z, Zhao Y, Kong P, Liu Y, Huang J, Xu E, et al. Integrated multi-omics profiling yields a clinically relevant molecular classification for esophageal squamous cell carcinoma. Cancer Cell. (2023) 41:181–95 e9. doi: 10.1016/j.ccell.2022.12.004
161. He X, Liu X, Zuo F, Shi H, Jing J. Artificial intelligence-based multi-omics analysis fuels cancer precision medicine. Semin Cancer Biol. (2023) 88:187–200. doi: 10.1016/j.semcancer.2022.12.009
162. Derraz B, Breda G, Kaempf C, Baenke F, Cotte F, Reiche K, et al. New regulatory thinking is needed for AI-based personalised drug and cell therapies in precision oncology. NPJ Precis Oncol. (2024) 8:23. doi: 10.1038/s41698-024-00517-w
163. Gilbert S. The EU passes the AI Act and its implications for digital medicine are unclear. NPJ Digit Med. (2024) 7:135. doi: 10.1038/s41746-024-01116-6
164. Fang Y, He W, Wang H, Wu M. Key considerations in the design of real-world studies. Contemp Clin Trials. (2020) 96:106091. doi: 10.1016/j.cct.2020.106091
165. Ebrahimian S, Kalra MK, Agarwal S, Bizzo BC, Elkholy M, Wald C, et al. FDA-regulated AI algorithms: trends, strengths, and gaps of validation studies. Acad Radiol. (2022) 29:559–66. doi: 10.1016/j.acra.2021.09.002
166. Terry SF. Obama's precision medicine initiative. Genet Test Mol Biomarkers. (2015) 19:113–4. doi: 10.1089/gtmb.2015.1563
167. Barlas S. The white house launches a cancer moonshot: despite funding questions, the progress appears promising. P T. (2016) 41:290–5.
168. Rodriguez-Antona C, Taron M. Pharmacogenomic biomarkers for personalized cancer treatment. J Intern Med. (2015) 277:201–17. doi: 10.1111/joim.2015.277.issue-2
Keywords: precision oncology, molecular biomarkers, artificial intelligence, clinical trials, personalized treatment
Citation: Chhabra R (2024) Molecular and modular intricacies of precision oncology. Front. Immunol. 15:1476494. doi: 10.3389/fimmu.2024.1476494
Received: 05 August 2024; Accepted: 30 September 2024;
Published: 23 October 2024.
Edited by:
Nicholas Adam Young, Private Health Management Inc., United StatesReviewed by:
Sakshi M., Emory University, United StatesCopyright © 2024 Chhabra. This is an open-access article distributed under the terms of the Creative Commons Attribution License (CC BY). The use, distribution or reproduction in other forums is permitted, provided the original author(s) and the copyright owner(s) are credited and that the original publication in this journal is cited, in accordance with accepted academic practice. No use, distribution or reproduction is permitted which does not comply with these terms.
*Correspondence: Ravneet Chhabra, cmNoaGFicmFAYmlvY3l0b2dlbi5jb20=