- 1Korea National Institute of Health, Korea Disease Control and Prevention Agency, CheongJu, Republic of Korea
- 2Department of Biochemistry, College of Life Science and Biotechnology, Yonsei University, Seoul, Republic of Korea
- 3Chemicals Research Division, National Institute of Environmental Research, Incheon, Republic of Korea
As Bacille Calmette-Guérin (BCG) vaccine’s effectiveness is limited to only children, the development of new tuberculosis (TB) vaccines is being studied using several platforms, and a novel TB vaccine that overcomes this limitation is required. In this study, we designed an effective multi-epitope vaccine against Mycobacterium tuberculosis using immunoinformatic analysis. First, we selected 11 highly antigenic proteins based on previous research: Ag85A, Ag85B, Ag85C, ESAT-6, MPT64, Rv2660c, TB10.4, HspX, GlfT2, Fas, and IniB. Among these antigens, 10 linear B-cell epitopes, 9 helper T-cell epitopes, and 16 cytotoxic T-cell epitopes were predicted to design the multi-epitope vaccine. To improve the immunogenicity of the candidate vaccine, three different adjuvants, griselimycin, human beta-defensin 3 (HBD3), and 50s ribosomal protein (50sRP), were attached with linker sequences to the vaccine model. The immunogenic, antigenic, allergenic, and physicochemical properties of the resulting designed multi-epitope vaccines were predicted in silico. Moreover, 3D structural modeling, refinement, and validation were used to select a model for further evaluation. Molecular docking analysis revealed a consistent and significant binding affinity of the candidate vaccine for toll-like receptors (TLRs), TLR-2, -3, and -4. Immune simulation performed using C-ImmSim demonstrated that three rounds of immunization with multi-epitope vaccines induced a high production of cytokines and immunoglobulins related with both cellular and humoral immune response. Moreover, we constructed vaccine candidate composed of 50sRP and evaluated its immunogenicity in a mouse model. Consequently, this in silico-engineered multi-epitope structure can elicit adaptive immune responses and represents a promising novel candidate for TB vaccine development.
1 Introduction
Tuberculosis (TB), a highly contagious disease, is one of the most prominent causes of death worldwide and was the leading cause of death from a single infectious agent before the coronavirus disease (COVID-19) pandemic. According to the World Health Organization 2023 report, TB caused by Mycobacterium tuberculosis was projected to infect approximately one-quarter of the global population and kill approximately 1.5 million individuals in 2022 (1).
Once M. tuberculosis bacteria are inhaled, bacilli are primarily encountered in alveolar macrophages (AMs) located in the airway and migrate to the lung interstitium through host IL-1β signaling and the M. tuberculosis type VII secretion system, ESX-I (2). After entering the lung interstitium, the bacilli infect additional macrophages including monocyte-derived- or lung resident cells. Circulating dendritic cells (DCs) migrate to the draining lymph nodes, where they present antigen peptides bound to major histocompatibility complex (MHC) molecules on their surface to prime antigen-specific T cells. The MHC-antigen peptide complex interacts with the T cell receptor (TCR) on the surface of T cells (3). Upon binding of the TCR to the MHC-antigen peptide complex, antigen recognition signals are transmitted into the T cell, initiating its activation. Once activated, T cells differentiate into effector cells that secrete a variety of cytokines. In the case of M. tuberculosis, Th1 cells, which differentiated from CD4+ T cells, are the primary immune responders. They secrete cytokines such as IFN-γ, TNF-α, and IL-2, which recruit monocytes and neutrophils, enhance macrophage cytotoxicity, and induce the production of inflammatory mediators and reactive oxygen and nitrogen species, leading to the elimination of M. tuberculosis.
An effective TB vaccine relies on the generation of long-lived memory T cells, necessitating the activation of B- and T-cells. A multi-epitope vaccine, binding to MHCs, stimulates CTLs and HTLs by engaging TLRs to activate key immune components (4–6). B-cell epitopes are also vital in triggering memory immune responses through antibody production. Identifying specific epitope regions is crucial for predicting immune responses (7). Compared to a single subunit vaccine, the multi-epitope vaccine has an advantage in inducing immune response, especially considering the limited efficacy of single-subunit TB vaccines in humans due to the disease’s complex progression (6).
Vaccinating individuals against TB faces ongoing challenges because Bacillus Calmette-Guérin (BCG), the only approved TB vaccine, has limited efficacy in adults and adolescents (8, 9). To overcome the limitations of BCG, novel TB vaccines have been developed either as a booster to the current BCG vaccine or as prime vaccines to replace it. The protection provided by BCG is lacking in adults, which may be the result of waning immunity during childhood, leading to a deficiency in immunological memory (10). According to the report released by the WHO, 17 TB vaccine candidates are in the clinical trial stage (1). The most promising vaccine, M72/AS01E which is comprised of Mtb32A and Mtb39A combined with the AS01E adjuvant, showed good protection in healthy adults (11, 12) and HIV-infected adults (13). A Phase II b trial of M72/AS01E showed 54.0% protective efficacy without substantial safety concerns (14). Furthermore, its effectiveness was 49.7% after three years of follow-up in final analyses of the efficacy, safety, and immunogenicity (15). However, the findings need to be confirmed over a longer period and in a larger population with different age groups and ethnicities. Therefore, studies for the development of more effective TB vaccines are a continuous endeavor.
In the realm of development of a TB vaccine, subunit vaccines utilizing specific antigens, such as M72/AS01E, H56:IC31, and ID93 + GLA-SE, which are currently under investigation in various clinical trials, offer the advantage of targeted and efficient development. However, their potential protective range might be limited due to the specificity of the selected antigens. In contrast, the multi-epitope TB vaccine strategy, anchored on a diverse set of antigens, holds promise for enhanced protection and broader efficacy. Bacterial pan-genomics analysis is recently being utilized to identify core genomes and potential vaccine targets in the development of multi-epitope-based vaccine candidates (16, 17). A proteomic analysis approach was also attempted, which utilized 242 virulent factors, 18 membrane proteins, 10 repair proteins, and 8 secretory proteins as potential candidates (18). For a comprehensive understanding of the approach to select pivotal antigens for the TB vaccine, comparing this method with other research endeavors that have evaluated M. tuberculosis vaccine candidates using various techniques is expected to be beneficial.
Additionally, ongoing research is harnessing various bioinformatics strategies for the judicious selection of epitope sequences. Investigations have been conducted to select effective MHC epitope regions by analyzing alleles and haplotypes that cover more than 95% of the world population (19), predict epitope sites in the RD1 region to overcome the limitations of the BCG vaccine (20), and use structural vaccinology tools to identify epitope sites that exhibit stability and antigenic tendencies (21). Such diverse approaches have advanced the design of antigen and epitope sequences. However, M. tuberculosis possesses an extensive array of over 4,000 antigens, and there remain unexplored areas with regard to its mechanisms of immune evasion and infection within the host.
To overcome the limitations of the BCG vaccine, next-generation TB vaccine or BCG-booster vaccines have been studied and developed using various platforms. Among them, peptide-based vaccines have advantages compared with traditional subunit vaccines. Although subunit vaccines are poor inducers of T-cell responses, peptide-based vaccines induce a more robust immune response with dominant epitope regions and reduce side effects caused by eliminating unwanted material from full-length protein (4, 22). Generally, peptide-based vaccines are designed by identification with bioinformatic tools and validated by using ELISpot in healthy and donor PBMCs (23). However, in the case of TB, there are numerous unrevealed TB antigens and their functions are largely unknown. Consequently, we have selected the Ag85 complex, ESAT-6, MPT64, Rv2660c, TB10.4, and HspX, as antigens from among the virulence factors of M. tuberculosis and those acting as immunogens within the host, with their vaccine efficacy validated through in vivo studies using animal models or clinical trials (24–34). Also, we include the antigens GlfT2, Fas, and IniB, based on a previous antigen identification study (35). Bettencourt et al. identified and studied antigens presented by MHC class I and II molecules in infected macrophages (35). The vaccine candidates, which were constructed with adenoviral vectors, carried respective antigens and showed immunogenicity and protective effectiveness as a BCG-booster vaccine. Furthermore, we predicted and validated their possibilities of use with its adjuvants, griselimycin, HBD3, and 50sRP, by using various bioinformatic tools. We selected the most soluble and the lowest Gibbs free energy model, which was best suited for TLR4 binding, and confirmed its immunogenicity in BCG-primed mice through in vitro expression and in vivo analysis.
2 Materials and methods
2.1 Antigen selection for vaccine preparation
Antigens were selected based on those included in the ongoing clinical trials and the results of another previous study (Table 1) (24, 25, 35–40). Ag85A, Ag85B, ESAT-6, Rv2660c, TB10.4, and HspX were constructed as subunit or virus vector vaccines and their effectiveness has been verified in animal models. Some antigens have progressed to the clinical trial phase in healthy adults and patients with TB (24, 36–39). Ag85C and MPT64 were reported as immunodominant antigens and are being investigated as vaccine candidates in several studies (25, 40). Additionally, the GlfT2, Fas, and IniB antigens were selected based on the results of another previous study (35). These antigens were immunoprecipitated with the MHC-I and MHC-II from human macrophage cells and their effectiveness has been evaluated in an animal model. Amino acid sequences of all antigens are acquired from Mycobrowser (https://mycobrowser.epfl.ch).
2.2 Predicting linear B-cell epitopes
Among the various tools for B-cell epitope prediction, including BcePred (41) and BepiPred (42), ABCPred distinguishes itself by employing Artificial Neural Networks (ANN) (43). While BcePred relies on physicochemical properties and BepiPred utilizes a combination of the hidden Markov model and propensity scale method (41, 42), ABCPred harnesses the capabilities of ANN to detect patterns without predefined rules and this approach allows ABCPred to learn more complex interactions from the data achieving an accuracy of 65.93% (43). The ABCpred server (http://www.imtech.res.in/raghava/abcpred) was used to identify linear B-cell epitopes. The prediction process involved using the default threshold of 0.51, and a score > 0.87 was required for the 16-mer epitopes. Among the 11 antigens, Ag85A was removed from the analysis since Ag85A and Ag85B had identical amino acid sequences. A higher score indicates a probability of being a B-cell epitope. Therefore, we selected the final epitope sequence based on the highest score.
2.3 Predicting helper T lymphocyte epitopes
HTL epitopes serve play a pivotal role in coordinating the immune defense against intracellular pathogens such as M. tuberculosis. They act as a crucial link between innate immune recognition and the activation of cytotoxic responses, ensuring a targeted and effective immune reaction. To predict HTL epitope sequence, the Immune Epitope Database (IEDB) MHC-II server was utilized to identify human leukocyte antigen (HLA) class II epitopes, also known as HTL epitopes (44, 45). The species/locus was chosen as Human/HLA-DR, and a 7-allele HLA reference set (HLA-DRB1*03:01, HLA-DRB1*07:01, HLA-DRB1*15:01, HLA-DRB3*01:01, HLA-DRB3*02:02, HLA-DRB4*01:01, HLA-DRB5*01:01) was selected for the HTL epitope prediction. The server predicted the binding affinity of 15-mer peptides and generated percentile scores for each peptide based on its predicted binding affinity relative to a large set of random peptides. The peptides were then categorized based on their percentile scores, with those having higher scores considered to have stronger predicted binding affinity to the HLA class II allele (HLA-DR). Finally, the HTL epitopes were selected based on both their percentile scores and predicted ability to induce the production of IFN-γ, a cytokine involved in the immune response.
2.4 Predicting IFN-γ immune response-inducing epitopes
To determine the capacity of the predicted HTL epitopes to induce an IFN-γ immune response, they were analyzed using the IFNepitope server (http://crdd.osdd.net/raghava/ifnepitope/) (46). The server calculates an IFN-γ score for each peptide based on a hybrid approach that combines a motif approach and support vector machine (SVM) approach (46, 47). Through this hybrid approach, positive-scoring epitopes were selected as IFN-γ-inducible epitopes based on their IFN-γ scores. We selected the epitope sequence with the highest IFN-γ score to maximize its potential for inducing IFN-γ production, thereby anticipating a stronger immune response.
2.5 Predicting cytotoxic T lymphocyte epitopes
In immune response against M. tuberculosis infection, cytotoxic T lymphocyte attributes cytolytic action against pathogen by means of cell-to-cell contact determines the apoptosis of the pathogen infected macrophages (48, 49). Predictions of the 9-mer CTL epitopes were conducted using the NetCTL 1.2 server (http://www.cbs.dtu.dk/services/NetCTL) and based on A1 supertypes, which are commonly found in humans (50). The analysis included MHC-I binding affinity, C-terminal cleavage affinity, and efficiency of antigen processing transport (51) and each parameter was set 0.75, 0.15, and 0.05, respectively.
2.6 Designing multi-epitope vaccine sequence candidates
Based on the analyses described, high-scoring B-cell, CTL, and HTL epitopes were chosen to design a vaccine candidate with strong antigenicity and immunogenicity, and low toxicity. The linear B-cell and HTL epitopes were attached with GPGPG sequences, while the CTL epitopes were attached with AAY sequences. Griselimycin (52, 53), human β-defensin 3 (HBD3) (54, 55), and 50s ribosomal protein (50sRP) (56) were selected as adjuvants to enhance vaccine immunogenicity and were added to both the N- and C-terminals via EAAAK sequences (7, 52). This adjuvant sequence was sourced from the UniProt database (http://www.uniprot.org/).
2.7 Predicting multi-epitope vaccine sequence as inducers of pro- or anti-inflammatory cytokines
The potential immunogenicity of the final sequence for the induction of cytokines, IL-4, IL-6, and IL-10, was systematically assessed. This assessment employed specialized web servers, including IL4Preb, IL6Preb, and IL10Preb (57–60). For IL-4 induction, a hybrid analytical approach integrating SVM with Motif was adopted. Peptides were designated as IL-4 inducers based on a predetermined threshold exceeding 0.2. For predictions for IL-6 and IL-10 secretion, an array of machine-learning methodologies was explored. Notably, the Random Forest-based model showed superior predictive accuracy for IL-6 secretion. Peptides were discerned as inducers for IL-6 and IL-10 based on threshold values exceeding 0.11 and -0.03, respectively (61, 62).
2.8 Predicting antigenicity and allergenicity
The antigenicity of each candidate multi-epitope vaccine attached to its respective adjuvant was predicted using two online tools: VaxiJen 2.0 (http://www.ddg-pharmfac.net/vaxijen/VaxiJen/VaxiJen.html) (63) and the ANTIGENpro server (http://scratch.proteomics.ics.uci.edu) (64). VaxiJen 2.0 uses autocross covariance (ACC) transformation of protein sequences to determine antigenicity (63), while ANTIGENpro is a sequence-based, pathogen-independent tool for predicting antigenicity (64). ToxinPred server (http://crdd.osdd.net/raghava/toxinpred/) were employed to predict toxicity of multi-epitope sequence. This server facilitates the design of toxic peptides and the identification of toxin regions within proteins (65). AllerTOP v2.0 (http://www.pharmfac.net/allertop) and AllergenFP (http://ddg-pharmfac.net/AllergenFP/) were employed to predict the allergenicity of each of the vaccine candidates. AllerTOP v2.0 is a server that uses the physicochemical properties of proteins to predict allergens and is the first alignment-free tool of its kind. AllergenFP is an alignment-free method that predicts allergenicity based on properties such as hydrophobicity, size, relative abundance, helix formation, and β-strand forming tendencies of the amino acids (66).
2.9 Predicting physicochemical properties and solubility
The Expasy ProtParam server (https://web.expasy.org/protparam) was used to predict various physicochemical properties of the final multi-epitope vaccines. These properties include the grand average of hydropathicity (GRAVY), aliphatic index, in vitro and in vivo half-lives, instability index, theoretical isoelectric point (pI), and molecular weight. In addition, the Protein-Sol server (http://protein-sol.manchester.ac.uk) was utilized to predict the solubilities of the multi-epitope vaccine candidates (67). The predicted solubility (QuerySol) was represented as a scaled solubility value, where any value above 0.45 was considered to have higher solubility than the average value (PopAvrSol) from the experimental dataset. Conversely, proteins with lesser-scaled solubility values were considered to have lower solubility (67).
2.10 Predicting secondary and tertiary structure
To predict the secondary structures of the multi-epitope vaccines designed with their respective genetic adjuvants, we used the PSIPRED (Position-Specific Iterated PREDiction) protein structure prediction server (http://bioinf.cs.ucl.ac.uk/psipred). The PSIPRED is a highly accurate method for predicting secondary structure, with an average Q3 score of 76.5%, suggesting that it is a reliable tool for determining the secondary structures of the multi-epitope vaccine candidates (68). Furthermore, the RaptorX Property web server (http://raptorx.uchicago.edu/StructurePropertyPred/predict/) was used to predict the secondary structure properties of the multi-epitope vaccine in the absence of a template. This server is designed to predict various structural properties of a protein using a deep-learning model. Hence, it is a useful tool for predicting secondary structure properties.
Then, the I-TASSER (Iterative Threading ASSEmbly Refinement) server (https://zhanglab.ccmb.med.Umich.Edu/I-TASSER/) was utilized to construct the tertiary models of the multi-epitope vaccines. This server follows a sequence-to-structure-to-function approach, which involves predicting and identifying similar structural patterns from the Protein Data Bank (PDB) to create a 3D model. To assess the accuracy of the generated models, the server provides a template modeling (TM) score. A TM score greater than 0.5 indicates a reliable topological model, whereas a TM score less than 0.17 suggests a random similarity. These TM score cut-off values are not dependent on the length of the protein being modeled (69). Therefore, these TM score cut-offs could be used to evaluate the accuracy of the tertiary models of the multi-epitope vaccines generated by the I-TASSER server. The confidence scores (C-scores) calculated by I-TASSER typically range from -5 to 2, where a higher C-score indicates higher accuracy (69).
2.11 Refinement of the tertiary structure
To further improve the accuracy of the generated tertiary model of the multi-epitope vaccines, the GalaxyRefine server (http://galaxy.seoklab.org/cgi-bin/submit.cgi?type=REFINE) was used to refine the model. The GalaxyRefine method combines side-chain repacking followed by molecular dynamics simulations to relax the overall structure (70). GalaxyRefine is effective in improving the local structure quality, as demonstrated by the results of the CASP10 assessment. The quality of the refined model was evaluated based on several metrics, including GDT-HA, root-mean-square deviation (RMSD), MolProbity, clash, and Ramachandran plot scores. These metrics are commonly used to assess the accuracy and quality of protein models and provide a comprehensive evaluation of the refined model’s structural features.
2.12 Validation of the tertiary structure
Several web servers were used to assess and validate the reliability of the refined tertiary structures. First, the ProSA web server (https://prosa.services.came.sbg.ac.at/prosa.php) was used to determine the overall quality score for the input structure. A calculated score outside the range characteristic of native proteins suggests the presence of errors in the structure. Next, the ERRAT server (http://services.mbi.ucla.edu/ERRAT/) was utilized to compare the non-bonded atom-atom interactions with those of reliable high-resolution crystallographic structures, helping identify any potential errors or inaccuracies in the model. Finally, the RAMPAGE web server (http://mordred.bioc.cam.ac.uk/~rapper/rampage.php) was used to generate a Ramachandran plot (71). The RAMPAGE output includes the percentages of residues in the allowed and disallowed regions, providing information about the overall quality of the modeled structure. These validation steps provide confidence in the accuracy and reliability of the final refined tertiary model of the multi-epitope vaccine (72).
2.13 Predicting discontinuous B-cell epitopes
To identify the discontinuous B-cell epitopes in the refined final 3-D structure models, we used the ElliPro server (http://tools.iedb.org/ellipro). ElliPro employs a geometry-based approach to analyze protein structure and predict B-cell epitopes based on their spatial arrangement. The server provides an AUC (Area Under the Curve) value of 0.732, which is considered the best calculation out of all proteins (73). By using ElliPro, we were able to predict the locations of the discontinuous B-cell epitopes in the refined final model of the multi-epitope vaccine.
2.14 Molecular docking analysis of the final vaccine with toll-like receptors
The designed multi-epitope vaccines were subjected to molecular docking analysis with TLR2, TLR3, and TLR4 (PDB ID:1ZIW, 3A7B, 4G8A) using the HADDOCK 2.4 web server (http://bianca.science.uu.nl/haddock2.4), predicting the appropriate immune response and interactions between the final vaccine structures and these specific immune receptors (74). The HADDOCK 2.4 web server employs an integrative approach that combines biochemical and biophysical information with computational modeling to predict protein-protein interactions. The server calculates the lowest energy score for the final docking complex, which is indicative of the most stable complex (75). By using HADDOCK 2.4, the binding affinity of the multi-epitope vaccine to TLR2, TLR3, and TLR4 was predicted, a process that can help in designing effective vaccines.
2.15 Normal mode analysis
To better understand the stability and dynamicity of the interactions of the final vaccine structures with the TLR4 receptors, a normal mode analysis was performed. The iMODS web server (http://imods.Chanconlab.org/) was used for this purpose, as it allows for the analysis of protein flexibility and conformational changes over time (76). The simulation results were analyzed in terms of deformability, eigenvalue, b-factor, variance, correlation matrix, and the elastic network model.
2.16 Codon optimization and in silico cloning
The Java Codon Adaptation Tool (JCat) server (http://www.prodoric.de/JCat) was employed to improve protein expression efficiency via codon optimization (77). JCat is an adjustable tool that tailors the codon usage of an input sequence to better suit specific organisms, enhancing expression levels; in this study, it was used to adjust codon usage for E. coli. Following optimization, the codon-optimized sequence was cloned into the E. Coli pET30a (+) vector using the Snapgene 7.0.2 tool (https://snapgen.com/).
2.17 Immune simulation
Finally, the C-ImmSim server (http://150.146.2.1/C-IMMSIM/index.php) was used for in silico immune simulations to assess the immunogenicity and immune response generated by the multi-epitope vaccines. C-ImmSim is an agent-based model that utilizes position-specific scoring matrices for peptide prediction derived from machine-learning techniques (78). The default simulation parameters were used, with time steps set at 1, 84, and 168. Each time step represents 8 h, with the first injection given at time = 0, followed by two more injections at twelve-week intervals (79). The simulations aimed to characterize the immune interactions and evaluate the efficacy of the multi-epitope vaccines.
2.18 Cloning the designed gene and expression and purification of the recombinant protein
To construct the in silico designed recombinant protein, the nucleotide sequence of 50sRP, verified by in silico cloning, along with the addition of a poly histidine-tag (6x his tag) at the C-terminus was synthesized and cloned into a commercial pET-30a (+) expression vector (80). The recombinant expression vector was transformed into E. Coli BL21 (ED3) cells. A single colony was inoculated into the LB medium containing kanamycin. Expression of the recombinant protein was induced by adding isopropyl-β-thiogalactopyranoside (IPTG; 0.5mM) and assayed by using SDS-PAGE and western blotting using an anti-His antibody (Genscript, Piscataway, NJ, USA) at dilution of 1:1000. The expressed recombinant protein was purified with an Ni-NTA column under denaturing conditions following the manufacturer’s instructions (81). The purified protein was dialyzed using a urea solution and phosphate-buffered saline (pH 7.2) overnight at 4°C.
2.19 Animals, ethics statement and immunization
Four to five-week-old female C57BL/6 mice were purchased from Samtako (Seoul, Korea). Mice were kept under standard environmental conditions with commercial food and tap water (both ad libitum). Mice were acclimated at facility during 7 days. After 1 week, five to six-week-old mice (n = 5) were vaccinated with PBS or BCG Pasteur 1173P2 (2 × 105 CFUs/mouse) subcutaneously (week 0). After 6 weeks, BCG-primed mice were boosted with vaccine candidate; 50sRP-TB (5 μg/mouse) twice at an interval of 3 weeks (1st boost: 6 weeks, 2nd boost: 9 weeks). One week after the final immunization (at 10 weeks), the mice (n = 5) were euthanized via CO2 inhalation to analyze immunogenicity.
2.20 Preparation of M. bovis BCG
Mycobacterium bovis (M. bovis) BCG Pasteur 1173P2 were provided by the Korea Disease Control and Prevention Agency (KDCA) and cultured in Middlebrook’s 7H9 broth (Difco Laboratories, Detroit, MI) supplemented with 10% Oleic Albumin Dextrose Catalase (OADC) enrichment (Becton Dickinson, Sparks, MD) and 0.2% glycerol at 37°C on a shaker at 200 rpm under aerobic conditions for 14–20 d (82). To obtain single-cell suspensions, mycobacterial cell culture media were centrifugated at 10,000 × g for 20 min and washed thrice with PBS. The pellet was resuspended in PBS supplemented with 0.05% tyloxapol (Sigma Aldrich, Saint Louse, MO) to prevent clumping and passed through 40-, 20-, 10-, and finally, 8-μm filters (Millipore Corp., Burlington, MA, USA). The final stock was stored in small aliquots at −80°C until further use. Colony-forming units (CFUs) per milliliter of stock were measured using a counting assay on 7H10 agar plates (Difco Laboratories).
2.21 Preparation of single lymphocyte cells
Five mice from each group were sacrificed 1 week after the last immunization. Lung and spleen were harvested aseptically from euthanized mice and single cells extracted. To isolate lung lymphocyte, tissue was incubated with 40U/ml DNase I (Roche, Swiss) and 2mg/ml collagenase D (Roche) in a plain RPMI-1640 medium (GenDEPOT, TX, USA) at 37°C for 1 h. Enzyme-treated lung tissue and spleen were homogenized using a gentleMACS μTissue Dissociator (Miltenyl Biotec, Germany), washed in RPMI-1640 medium (GenDEPOT, TX, USA), and supplemented with 10% FBS. Spleen was rinsed in ammonium-chloride-potassium buffer to remove erythrocytes. Lung lymphocytes were separated using Lymphoprep (STEMCELL Technologies, Canada) with density centrifugation and resuspended in RPMI containing 10% fetal bovine serum (FBS, Gibco, MA, USA) and 1% penicillin/streptavidin (P/S, Gibco).
2.22 Antigen specific enzyme-linked immune spot
The ELISpot assay was performed using an IFN-γ secretion ELISpot kit. Briefly, a single-cell suspension (5 × 105 cells) was stimulated with pooled multi-epitope peptides (100ng/mL each) for 36 h at 37°C in anti-IFN-γ antibody-coated filter plates. Afterward, biotinylated anti-IFN-γ antibody, streptavidin-horseradish peroxidase (HRP) conjugate, and 3-amino-9-ethylcarbazole were added as substrates to develop secreted cell spots, which were quantified using an Immunospot S6 analyzer (Cellular Immunospot Limited). The results are presented as mean values of triplicate wells for each group. All substrates and the ELISpot kits were purchased from Becton Dickinson.
2.23 Enzyme-linked immune sorbent assay
For the measurement of immunoglobulin titers, 96-well flat bottom Immuno Plates (Thermo Fisher Scientific, MA, USA) were coated with pooled epitope peptides (100ng/mL each) diluted with ELISA coating buffer (BioLegned, CA, USA) for 18 h at 4°C. The plates were washed thrice with PBS containing 0.5% Tween 20 and blocked with 5% skimmed milk (Difco Laboratories). After washing, the mouse serum samples were diluted at 1:200 with PBS containing 3% BSA and incubated for 2 h at 37°C. Subsequently, a 1:2,000 dilution of goat anti-mouse IgG-HRP (Thermo Fisher Scientific) was added and incubated for 1 h at 37°C. The substrate, tetramethylbenzidine (TMB; Thermo Fisher Scientific), was added to each well, and the plates were incubated at 37°C for 15–30 min. Thereafter, a stop solution for TMB was added, and the plates were read using a spectrophotometer (Spectramax i3x, Molecular Devices, CA, USA) at 450 nm.
2.24 Statistical analyses
To determine statistical significance, one-way Analysis of Variance (ANOVA) using Dunnett’s multiple comparison test (comparing to PBS immunized mice only) was used for evaluation of significant differences between more than two vaccine groups. The differences with a p-value <0.05 were considered significant. Data expressed in graphs are presented as mean ± standard deviation. All analyses were performed using GraphPad Prism v10 (GraphPad Software, CA, USA).
3 Results
3.1 Linear B-cell epitope prediction
The functional sequences of the eleven proteins were subjected to linear B-cell epitope prediction using the ABCpred server (Table 2). The 16 mer peptides of the Ag85B, Ag85C, ESAT-6, MPT64, Rv2660c, TB10.4, HspX, GlfT2, Fas, and IniB were selected for the final vaccine based on their binding scores (> 0.51) and highest predicted score (83).
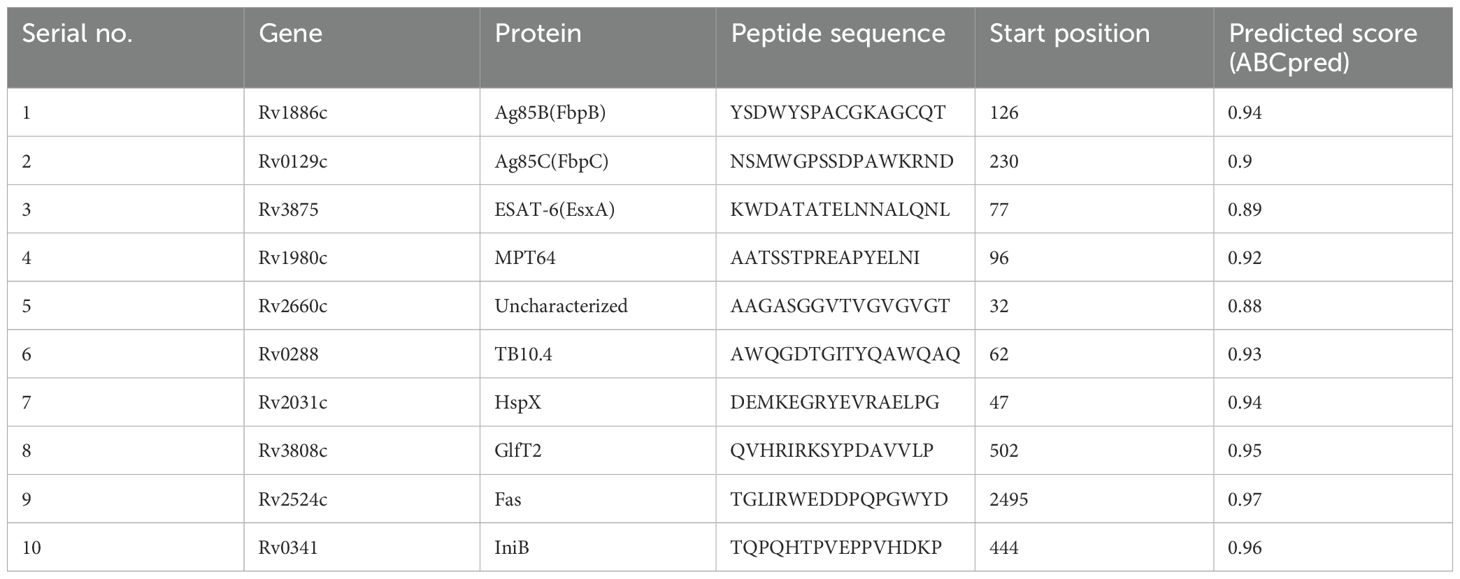
Table 2. Predictions of linear B-cell epitopes, the highest predicted score epitope was selected for the final multi-epitope tuberculosis vaccine construct.
3.2 T-cell epitope prediction
Inducing CTL- and HTL-mediated immune responses is a critical step in vaccine design. Within eleven of the antigens, several epitope regions with high binding affinity for the human HLA-DR alleles (a 7-allele HLA reference set) were identified using the IEDB MHC-II server, allowing the selection of promising epitopes. As a result, nine peptide sequences distinguished by their robust IFN-γ induction potential and low percentile scores were incorporated into the final vaccine candidates to induce an HTL immune response (Table 3). Specifically, antigens Ag85C and MPT64 were excluded from the final antigen list because Ag85C had a similar amino acid sequence to that of Ag85B and MPT64 elicited an extremely low IFN-γ immune response (IFN-γ score: 0.2038). As lower percentile scores represent higher MHC-II binding affinity (84), we choose the final epitope region of each antigen showing the lowest percentile score. An SVM-based approach was used to examine the positive versus negative IFN-γ responses to identify epitope regions that induce an IFN-γ response.
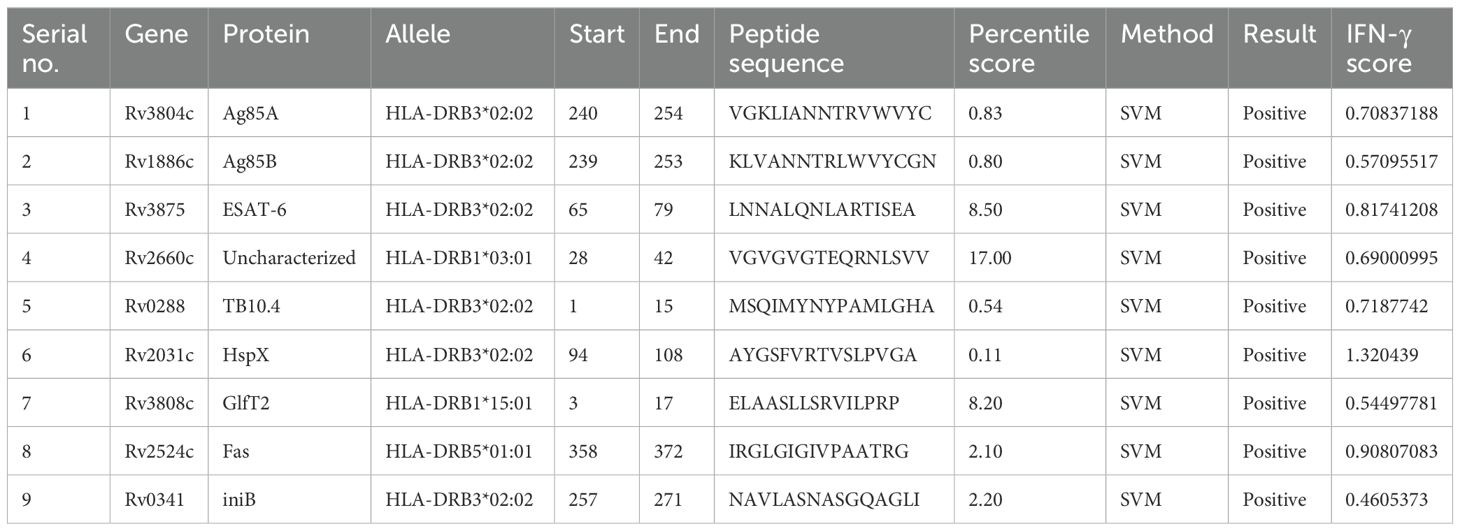
Table 3. List of the helper T lymphocyte epitopes selected from the Immune Epitope Database (IEDB) MHC-Ⅱ server based on their high binding affinity for major histocompatibility complex (MHC)-Ⅱ human leukocyte antigen (HLA)-DR alleles.
The final 16 epitope regions of the 9 antigens were identified using the NetCTL 1.2 web server based on their MHC-I binding affinity, C-terminal cleavage affinity, and transport efficiency to select those that induce a CTL response. Notably, ESAT-6 and Ag85A were excluded from the final antigen list because ESAT-6 had an extremely low transport efficiency (-0.656) and Ag85A had a similar amino acid sequence to Ag85B. Therefore, we selected multiple sequences of Ag85B with higher affinity and efficiency scores instead (Table 4). The final epitope sequences were characterized based on the pro- or anti-inflammatory features. Using several immune-informative tools, IL-4, IL-6, and IL-10 inducibility of respective epitope sequence was predicted (Table 5). 6 B cell epitope, 5 HTL epitope, and 12 CTL epitope were predicted as IL-4 inducer, and 8 B cell epitope, 1 HTL epitope, 16 CTL epitope were predicted as IL-6 inducer. Taken together, a considerable proportion of the CTL epitope sequence was predicted as IL-4 and IL-6 inducers which were pro-inflammatory cytokines engaged in the regulation of M. tuberculosis (85–88). Only one, namely the HTL epitope of Rv3808c (GlfT2), was confirmed as an inducer of IL-10, known as the anti-inflammatory cytokine in the pathogenesis of Mycobacteria (Table 5).
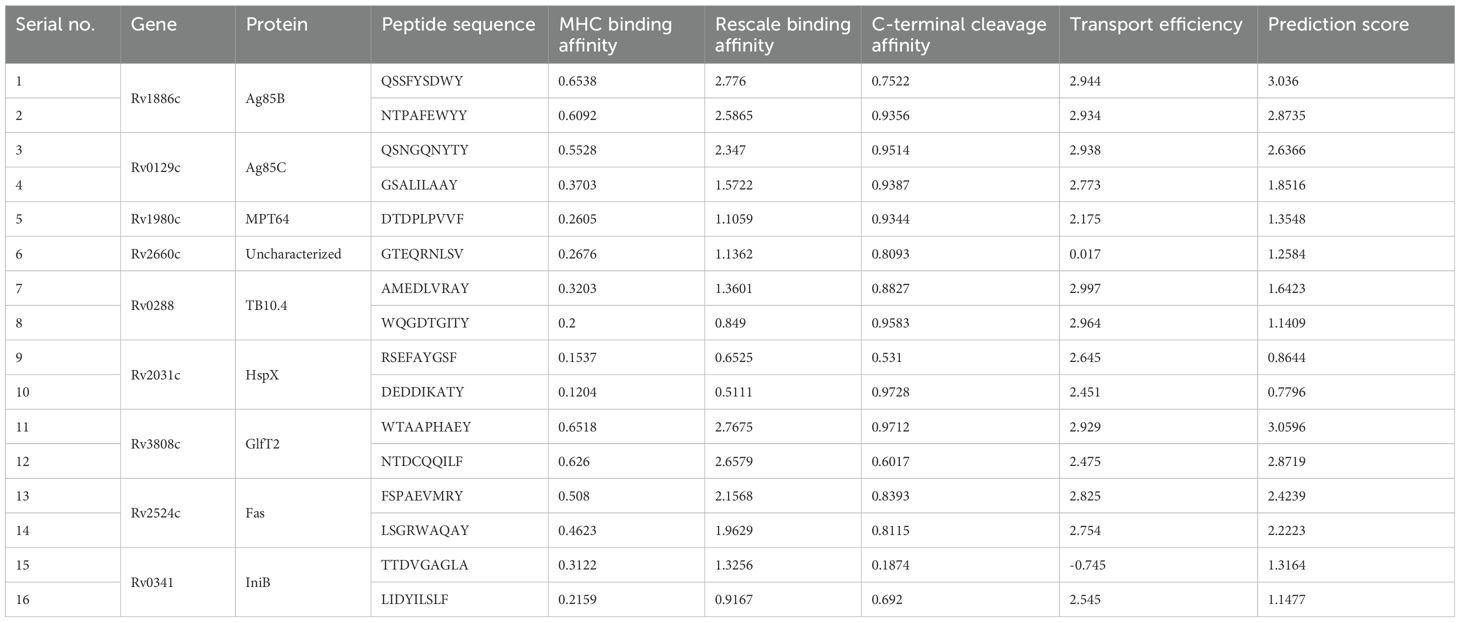
Table 4. List of the cytotoxic T lymphocyte (CTL) epitopes selected from NetCTL 1.2 server based on their high binding affinity with MHC-Ⅰ A1-supertype alleles and antigenicity as well as low/no allergenicity and toxicity.
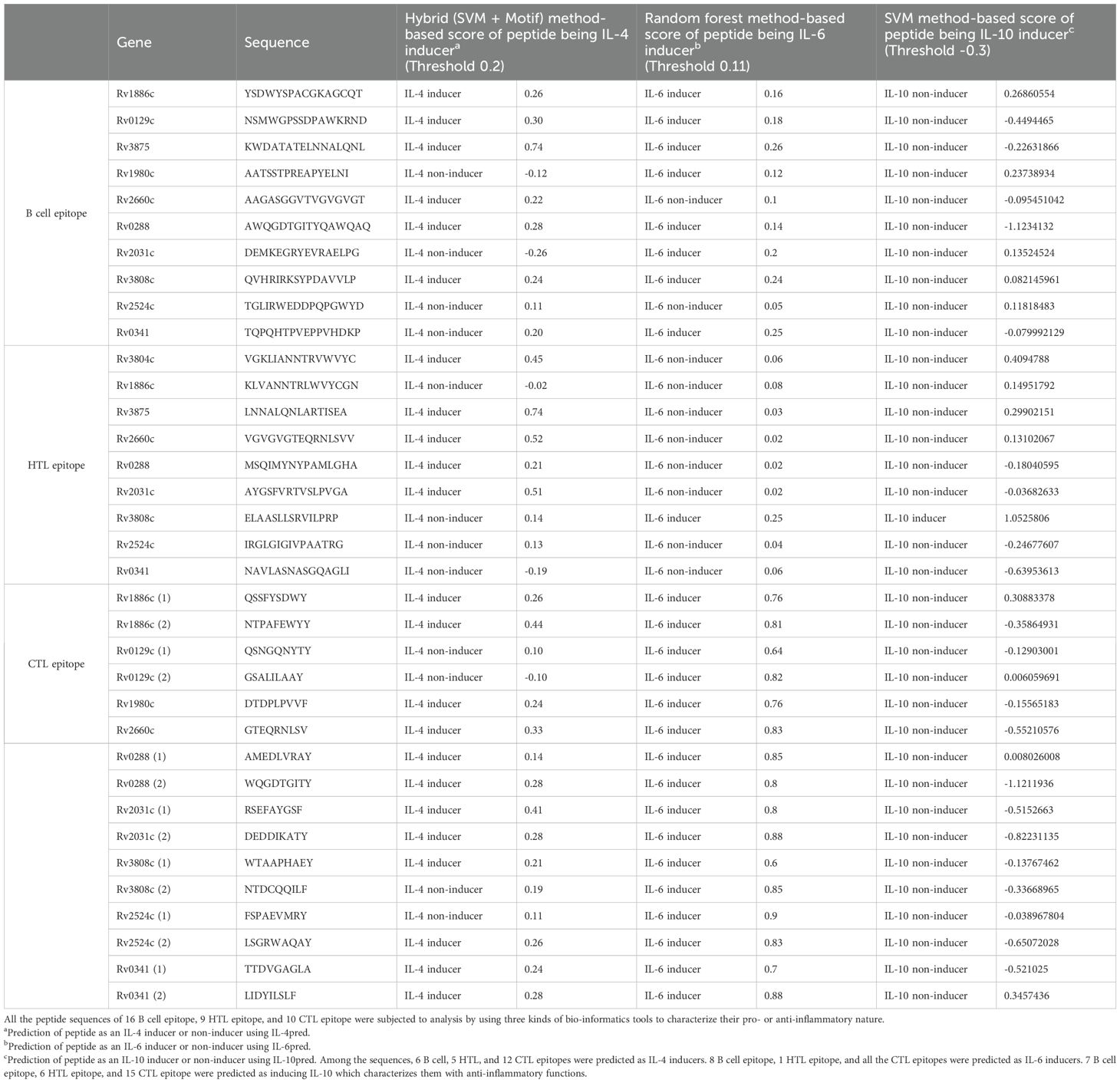
Table 5. In-silico characterization of multi-epitope sequence of TB vaccine model as pro- or anti-inflammatory.
3.3 Construction of the multi-epitope vaccines
Selected based on their higher antigenic and immunogenic properties as well as MHC class binding affinity, epitopes used for the multi-epitope vaccine candidates included 10 linear B-cell epitopes, 9 HTL epitopes, and 16 CTL epitopes. In addition, to augment the effectiveness of the multi-epitope vaccines, one of three adjuvants, including griselimycin (52, 53), HBD3 (54, 55), and 50sRP (56), were linked at the N- and C-terminals of the vaccine to produce three different vaccine constructs. Moreover, EAAAK, GPGPG, and AAY linkers were added between the adjuvant, B-, HTL, and CTL epitopes, respectively, to increase the accuracy of the expression of each peptide (Figure 1).
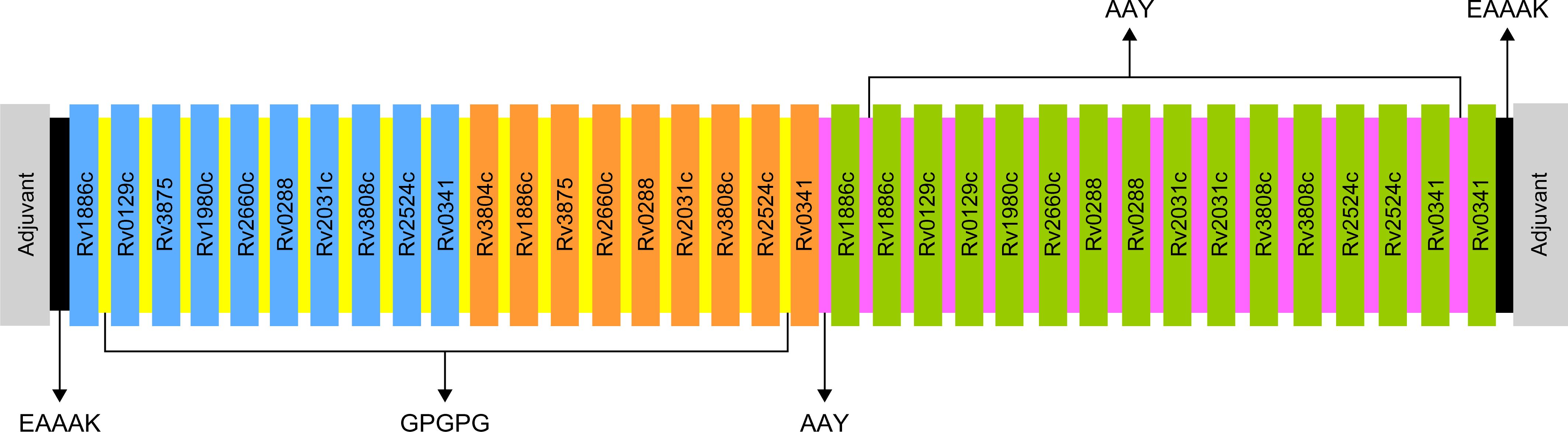
Figure 1. Schematic representation of the final multi-epitope vaccine peptide. Blue, orange, and green depict the designed vaccine construct with B-cell, HTL, and CTL epitopes. EAAAK linkers (black) were used to link the adjuvant with the N- and C-terminal sequences. B-cell and HTL epitopes were linked with GPGPG linkers (yellow), and CTL epitopes were linked with AAY linkers (purple).
3.4 Antigenic, allergenic, toxicity, and physicochemical evaluation of the designed vaccines
The antigenicity of each vaccine candidate represents their ability to be recognized as antigens and their ability to advance immune responses. The antigenicity of each multi-epitope vaccine was predicted using VaxiJen 2.0, and those with a score >0.4 were considered to be antigenic. The griselimycin-, HBD3-, and 50sRP-TB vaccines showed scores of 0.7339, 0.7380, and 0.6375, respectively. Additionally, antigenicity was confirmed using ANTIGENpro, in which griselimycin-TB scored 0.791234, HBD3-TB scored 0.815016, and 50sRP-TB scored 0.873047. Based on the results from analysis using AllerTOP v2.0 and AllergenFP and ToxinPred, the multi-epitope vaccines were estimated to be non-allergenic and non-toxic (Supplementary Table S1).
The physicochemical properties of the multi-epitope vaccines were evaluated using Expasy ProtParam. Molecular weight of griselimycin-, HBD3, and 50sRP-adjuvanted vaccine candidates which are named as griselimycin-TB, HBD3-TB, and 50sRP-TB are 61kDa, 69kDa, and 85kDa in order. Supplementary Table S1 shows that the theoretical pI values of the griselimycin- and 50sRP-TB models were 5.00 and 4.74, respectively, suggesting slight acidity, whereas that of the HBD3-TB model appeared to be slightly basic. The aliphatic index of the three vaccine models ranged from 67 to 76, indicating the thermostability of the vaccine constructs. The griselimycin-TB model has an estimated half-life of 100 h in mammalian reticulocytes, >20 h in yeasts, and >10 h in Escherichia coli. The estimated half-lives of the HBD3- and 50sRP-TB models are 30 h in mammalian reticulocytes, >20 h in yeasts, and >10 h in E. coli. The GRAVY scores were -0.202, -0.318, and -0.12 for the griselimycin-, HBD3-, and 50sRP-TB models, respectively, with negative GRAVY scores indicating that the structures are maintained under natural hydrophilic conditions and that they interact with water molecules. In addition, the instability indices of the griselimycin-, HBD3-, and 50sRP-TB models were 32.99, 34.25, and 28.18, respectively, suggesting they are stable, as an instability index >40 is considered unstable (Supplementary Table S1). Moreover, the solubility scores for griselimycin-, HBD3-, and 50sRP-TB were 0.302, 0.315, and 0.514, respectively (Supplementary Figures S1Ai–Ci). The whole sequences of griselimycin-, HBD3-, and 50sRP-TB models were estimated to have 33.3%, 30.4%, and 37.5% α-helix and 11.4%, 15.9%, and 13.0% β-strands (Supplementary Figures S1Aii–Cii).
Furthermore, a number of peptide-based vaccines and therapeutics for infectious diseases have received approval, and the strategic utilization of already sanctioned vaccines through matching or similarity queries within the THPdb database could lead to more auspicious outcomes (89). In this context, our research was directed specifically toward the THPdb database with an emphasis on data pertinent to contagious maladies such as tuberculosis. Unfortunately, we were unable to locate a specific peptide; only the PPD (Tuberculin Purified Protein Derivative) (ThPP ID as TH1194) could be retrieved. Additionally, we confirmed the potential of our multi-epitope model as a TB therapeutic strategy by validating the results using antiTBpdb (https://webs.iiitd.edu.in/raghava/antitbpdb/index.html) (90). Upon setting the E value in antiTBpdb at < 0.1, HTL and CTL epitopes of Rv0288 (TB10.4) and HTL epitope of Rv2031c (HspX) exhibited high similarities to the anti-tubercular peptides (Supplementary Table S2, The E values were in the following order: 4E-05, 0.037, 0.063). Therefore, there is potential for our model to be considered a therapeutic option, possibly after sequence modification.
3.5 Structural modeling, refinement, and validation of the multi-epitope vaccines
The tertiary structure of each model was predicted using the I-TASSER server based on TM value, C-score, and RMSD. Typically, C-score values range from -5 to 2, with higher scores indicating a greater level of confidence (69). A TM value >0.5 indicates an accurate topology model, while a TM value <0.17 indicates random similarity. RMSD indicates the conformational stability of the protein complex (91). The five predicted models from the griselimycin-TB construct had C-score values ranging from -3.63 to -1.57. The griselimycin-TB model had an estimated TM value of 0.52 ± 0.15 and RMSD of 11.4 ± 4.5Å. The five predicted models from the HBD3-TB construct had C-score values ranging from -3.53 to -1.33, an estimated TM value of 0.55 ± 1.5, and an RMSD of 11.1 ± 4.6Å. Finally, the five predicted models from the 50sRP-TB construct had C-score values ranging from -4.3 to -1.47, an estimated TM value of 0.53 ± 0.15, and an RMSD of 12.0 ± 4.4Å. The highest C-score models from each vaccine were selected for further refinement and validation (Figure 2A).
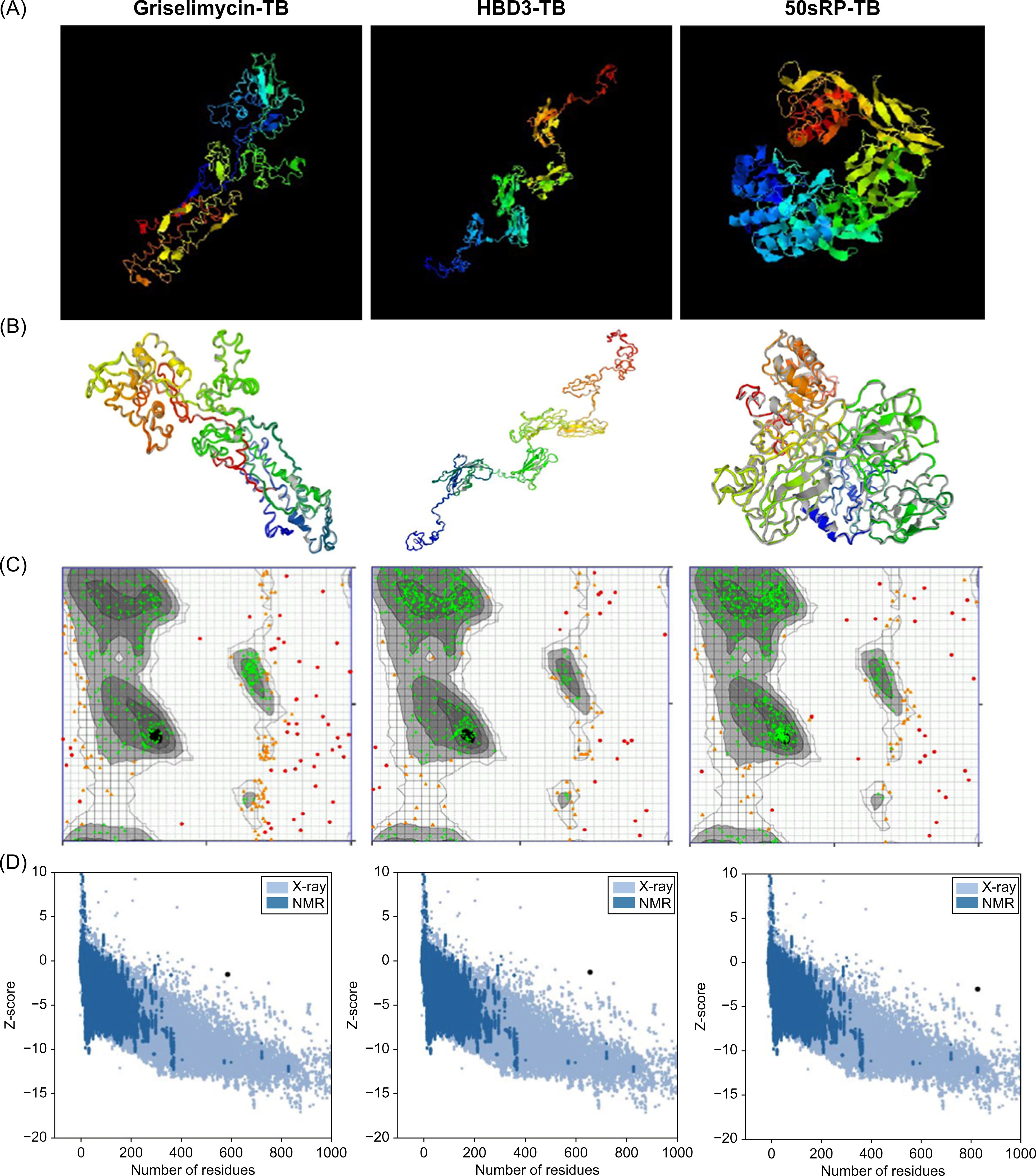
Figure 2. Protein 3D modeling, refinement, and validation. (A) Final 3D model of each multi-epitope vaccine depicted using I-TASSER. (B) Refinement of each construct was conducted using the GalaxyRefine web server. The tertiary structure was validated using the (C) Ramachandran plot and (D) ProSA web server.
The GalaxyRefine web server was used to identify the initial “crude” vaccine models. Model 1 from each construct, the one that showed the highest C-score value and TM-score >0.5, was the most reliable refined structure based on structural quality (Figure 2B). The refined model was validated using Ramachandran plot analysis, ERRAT, and the ProSA web server. In the Ramachandran plot analysis, 50sRP-TB had both the highest and lowest disallowed scores out of all three vaccine models (Figure 2C; Table 6). The quality and potential errors of the refined models were verified using the ProSA-web and ERRAT servers. A good model has a Z-score of -6.07 predicted using the ProSA-web and an accepted range >50 in ERRAT (92). Among these models, 50sRP-TB showed the most promising results through Ramachandran plot analysis, as well as the closest Z-score (griselimycin-TB: -1.4, HBD3-TB: -1.2, and 50sRP-TB: -2.9). Moreover, the overall quality factor predicted using the ERRAT server also indicated a good model (griselimycin-TB: 41.2, HBD3-TB: 75.2, and 50sRP-TB: 68.7) (Figure 2D; Table 6).

Table 6. Summary of 3D tertiary structure validation of the multi-epitope TB vaccine with various adjuvants.
3.6 Prediction of B-cell epitopes
Structural epitope prediction was performed using the Ellipro tool from the IEDB server. For discontinuous peptide predictions, an AUC score of 0.69 or higher was selected. A total of 59 residues in griselimycin-TB, 48 in HBD3-TB, and 103 in 50sRP-TB were identified within discontinuous B-cell epitopes, with respective AUC scores of 0.787, 0.913, and 0.717 (Figure 3 and Table 7).
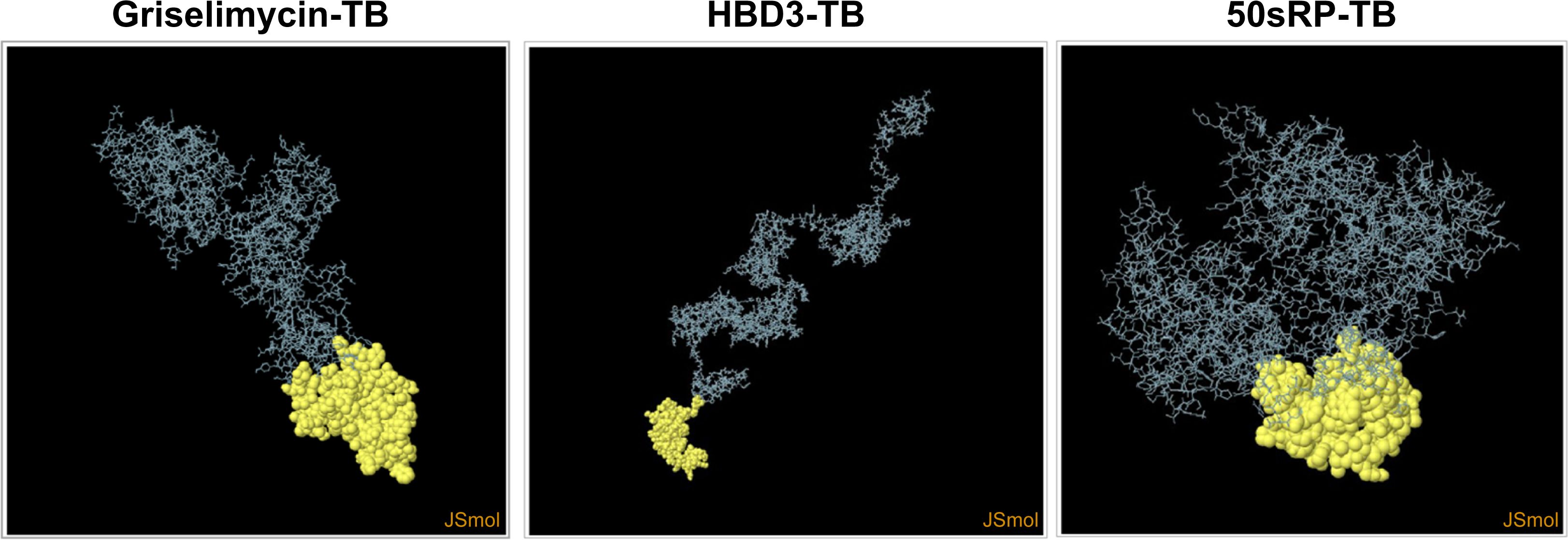
Figure 3. Representation of conformational or discontinuous B-cell epitopes of each designed multi-epitope vaccine. The conformational or discontinuous B-cell epitopes were represented with yellow circles, and the rest of the protein was represented with grey bars.
3.7 Molecular docking analysis and dynamics simulations
To verify the immune response induced by interactions of the vaccines with TLRs, molecular docking analysis and dynamic simulations were performed using the HADDOCK server. TLRs are proteins expressed in target immune cells that recognize pathogen-associated molecular patterns to induce an innate immune response (93). Notably, TLR4 plays a crucial role in M. tuberculosis infection (94) and TLR4 agonist have been developed as vaccine adjuvant candidate for TB to increase the efficacy of the existing vaccine (95); therefore, the vaccine models were subjected to molecular docking analysis with TLR4 in the normal mode. Several parameters of the multi-epitope vaccine models and the TLR4-MD2 (PDB: 3FXI)-docked complex were evaluated using normal mode analysis (NMA) (Figure 4). The b-factor shows the relationship between the NMA and PDB of the docked model and represents the protein flexibility and mobility (96–98). The variance and covariance matrix provides the correlation between amino acid duplets and infers the most rigid models (99); a higher correlation means the better complex. Red indicates correlated residues; white indicates uncorrelated residues, and blue indicates anti-correlated residues (100). The elastic network shows the connecting matrix with rigid regions and classifies the atom pairs connected by a spring (101). Among the vaccine models, the 50sRP-TB model was identified as it includes several hinge regions, suggestive of the most deformative candidate. All models showed that the calculated b-factor was minimized compared to the native b-factor of PDB, implying minimization of the deformability of the structure. The eigenvalue of our vaccine models, griselimycin-, and 50sRP-TB was, respectively, 1.411600E-05, and 4.278009E-05, while that of TLR4 was 6.823114E-06 (Figures 4A, C). These findings show that docked complexes are stable with low deformation characteristics and have higher structural rigidity. For the griselimycin-, HBD3-, and 50sRP-TB vaccine models, the binding free energies were -44.64kcal/mol, -23.42kcal/mol, and -63.09kcal/mol, respectively.
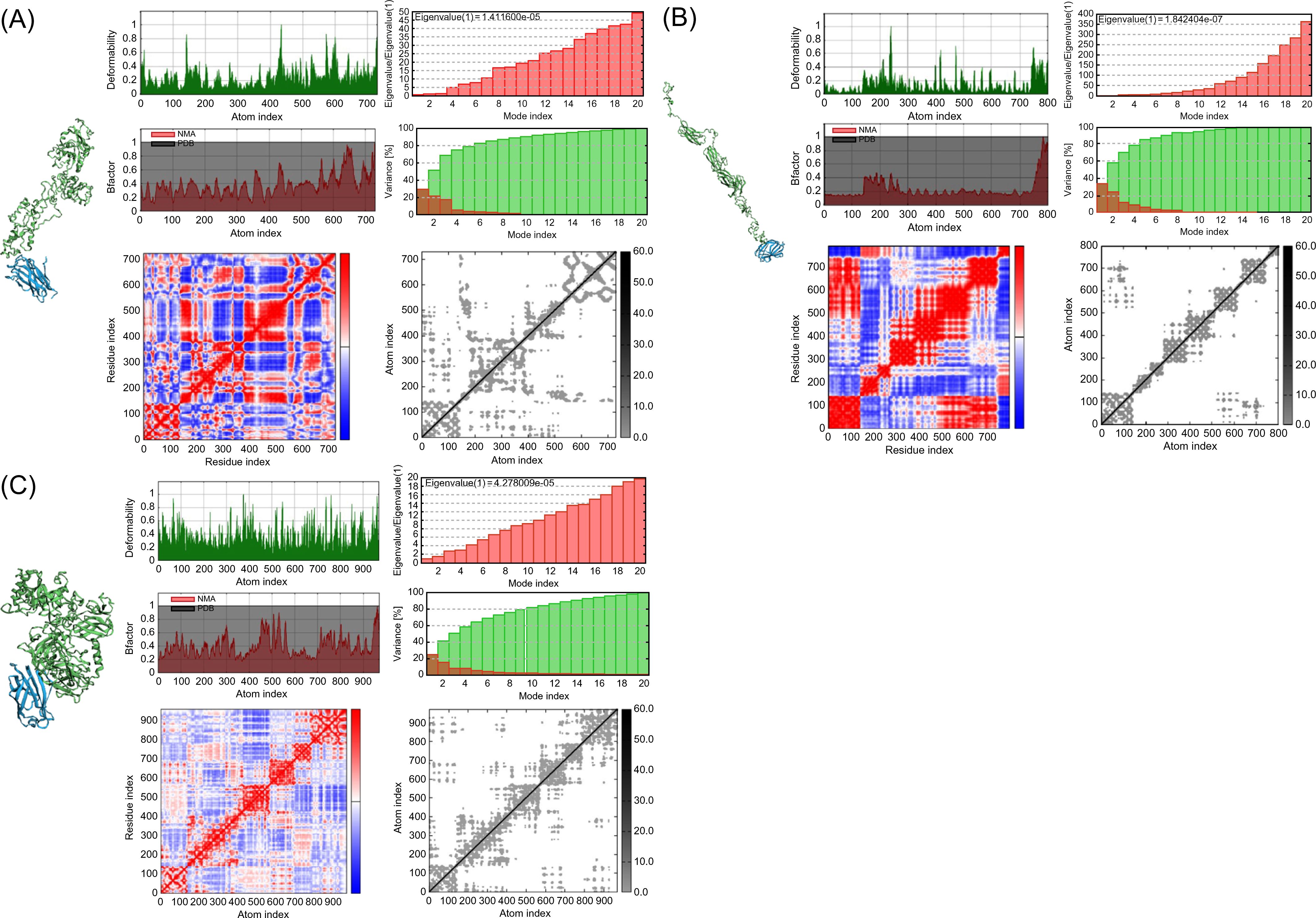
Figure 4. Normal mode analysis of vaccine constructs and their molecular docking with immune receptor TLR4. Normal mode analysis (NMA) mobility, deformability, eigenvalues, B factor, variance, co-variance map, and elastic network were evaluated in the vaccine constructs with the following adjuvants: (A) griselimycin, (B) HBD3, and (C) 50sRP.
Additionally, TLR2 and TLR3 play key roles in the innate immune response against M. tuberculosis infection (102–104). Based on docking analyses (Supplementary Figure S2), griselimycin-TB exhibited the highest binding affinity with TLR3, followed by TLR4 and TLR2. Similarly, HBD3-TB demonstrated the highest affinity for TLR4, TLR3, and TLR2, in that order. For 50sRP-TB, the highest binding affinity was observed with TLR3, followed by TLR2 and TLR4.
3.8 Codon optimization
The Java codon adaptation tool JCat was used with each of the respective multi-epitope vaccine models to optimize the codons for maximal expression in E. coli (strain K12). In codon-optimized sequences of the designed vaccines (griselimycin-, HBD3-, 50sRP-TB), the codon adaptation index values were 1.0, and the GC content values were 58.76%, 57.27%, and 55.25%, respectively. Additionally, the adapted codon sequences were optimized with sticky end restriction sites of NdeⅠ and HindIII at the N-terminus and C-terminus to facilitate restriction and cloning and inserted into the recombinant plasmid vector, pET-30a (+), using the Snapgene tool to design and effective cloning strategy (Figure 5).
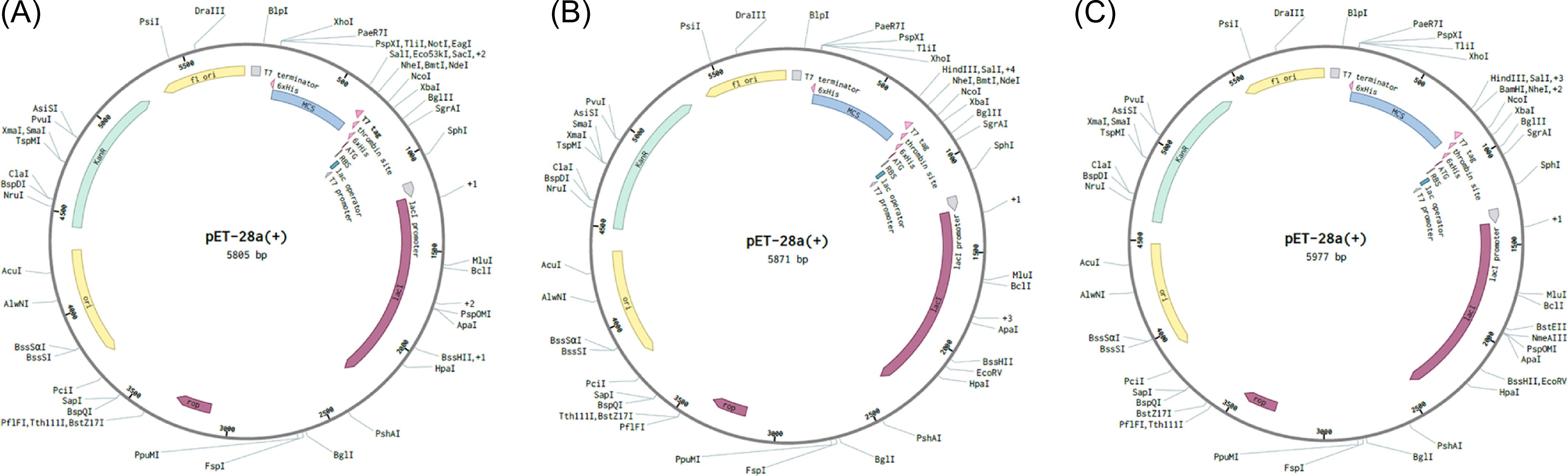
Figure 5. In silico restriction cloning of multiple-epitope vaccines into the expression vector pET30a(+). The codon sequence of each multi-epitope vaccine, (A) griselimycin-TB (7,020bp), (B) HBD3-TB (7,230bp), and (C) 50sRP-TB (7,740bp), was inserted in the multiple cloning site (MCS) of the pET30a(+) expression vector using the Snapgene sequence alignment tool.
3.9 Immune simulations
In silico immune simulations were conducted using the C-ImmSim immune server to predict the immunological profile of the multi-epitope vaccines (Figures 6, 7). The griselimycin-, HBD3-, and 50sRP-TB vaccine models elicited a significant increase in B cell population, including memory B cells, following repeated immunizations, indicating their capacity for isotype switching and memory cell formation (Figure 6). In Figure 6, antibody levels (IgM+IgG, IgG1+IgG2, IgM, and IgG) were found to increase during secondary and tertiary immunizations, accompanied by a decrease in antigen count. Furthermore, both CTL and HTL populations increased following secondary and tertiary immunization (Figure 7). Notably, a significant increase in IFN-γ production and a moderate increase in IL-2 were observed after the third vaccination. These data underscore the potential of our vaccine candidate to induce an effective immune response.
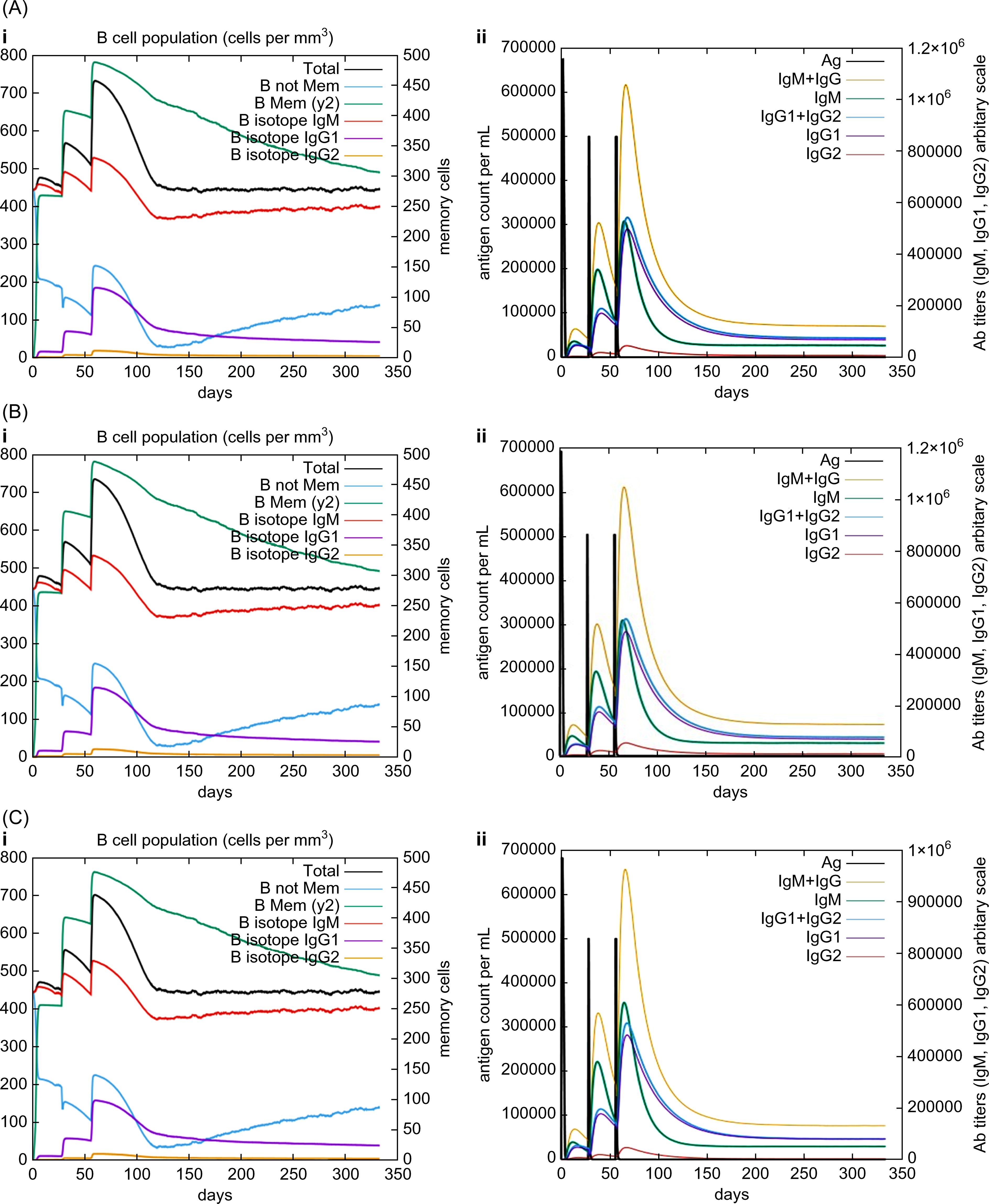
Figure 6. In silico C-ImmSim simulation of generated immune responses. Immunoglobulin production induced by multi-epitope vaccines with each respective adjuvant: (A) griselimycin, (B) HBD3, and (C) 50sRP. (i) The evolution of the B-cell populations and (ii) the production of various immunoglobulins in response to vaccination administered three times at twelve-week intervals.
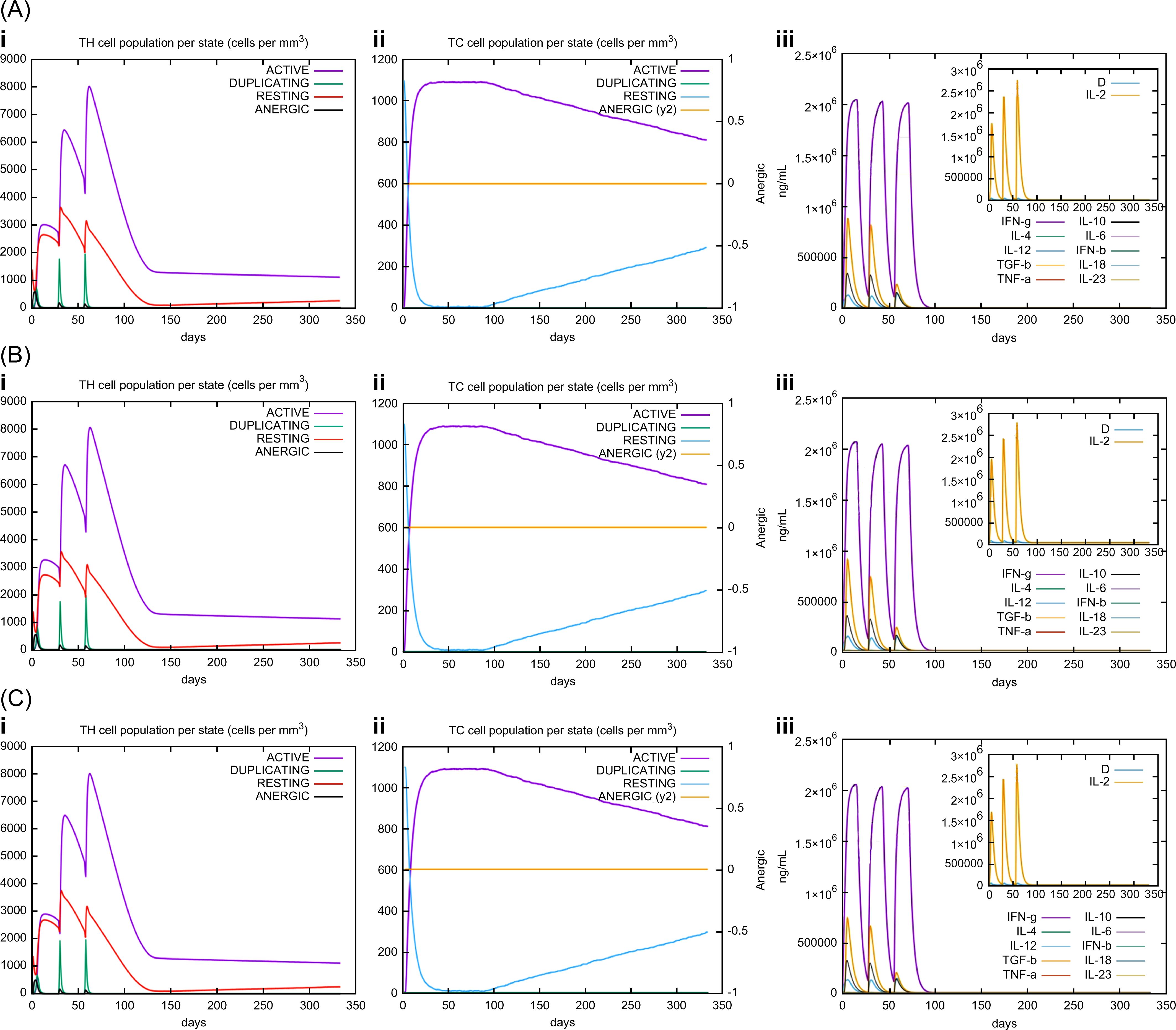
Figure 7. C-ImmSim simulation of the cytokine levels and antibody production. Predicted changes in (i) HTL population, (ii) CTL population, and (iii) cytokine levels in response to multi-epitope vaccines with respective adjuvants: (A) griselimycin, (B) HBD3, and (C) 50sRP.
3.10 In vitro expression
To verify the expression of the vaccine model, we selected on candidate based on solubility and TLR4 docking model analysis. Predicting protein solubility is crucial for the selection of highly effective candidate proteins, as it can help avoid protein aggregation, which adversely affects biological activity and can lead to failures in the recombinant protein pipeline (105). Consequently, 50sRP-TB, which demonstrated the highest solubility score, was selected for expression in E. coli. Moreover, the 50sRP-TB model exhibited the lowest Gibbs free energy in the TLR4 docking model, suggesting its potential to induce an active TLR4-mediated immune response. We validated in vitro expression and purification using SDS-PAGE and western blot. The protein was best expressed in 16-h induction at 15°C in supernatant of cell lysate and molecular weight inclusive of His-tag molecular weight was approximately 80kDa (Supplementary Figure S3).
To verify the expression of the vaccine model, 50sRP-TB which showed the highest solubility score, was selected and expressed in E. coli. We validated in vitro expression and purification using SDS-PAGE and western blot. The protein was best expressed in 16-h induction at 15°C and molecular weight inclusive of His-tag molecular weight was approximately 80 kDa (Supplementary Figure S3).
3.11 Immunogenicity of vaccine candidate designed by in silico analysis
To examine the effectiveness of artificially designed vaccine product, we investigated immunogenicity of 50sRP-TB in BCG-primed mouse. Mice were immunized subcutaneously with BCG and boosted with two doses of 50sRP-TB (Figure 8A). Immunologic analysis was performed with 50sRP-TB specific IFN-γ secretion and IgG, IgG1, and IgG2b measurement. Using ELISpot assay, multi-epitope peptides specific IFN-γ secreted cells were detected in lung lymphocytes and splenocytes. The number of IFN-γ spots in the BCG/50sRP-TB immunized group was significantly higher than those in the PBS or BCG only immunized groups, both in lung lymphocytes and splenocytes (Figures 8B, C). In addition, 50sRP-TB immunized mice generated higher titers of pooled multi-epitope peptides-specific IgG(H+L) and IgG1 than the other groups (Figures 8D, E). Remarkably, 50sRP-TB immunization elicited IgG2b production, which is associated with Th1 response (Figure 8F).
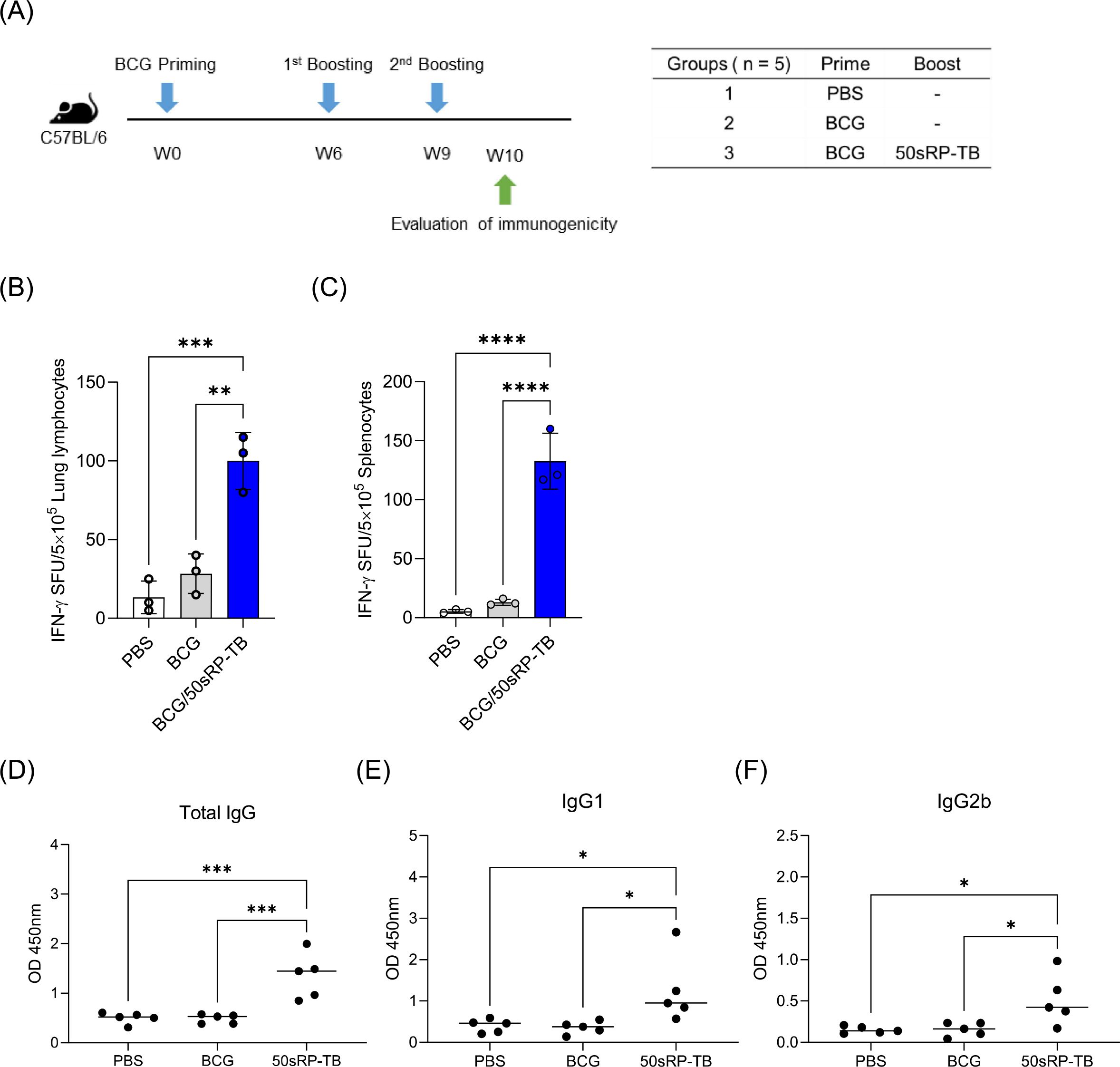
Figure 8. Immunogenicity of 50sRP-TB as a BCG-booster vaccine. (A) Schematic of the immunization schedule and subsequent evaluation. Mice (n = 5) were immunized by BCG 6 weeks before subunit vaccination. Subcutaneous immunization of 50sRP-TB was conducted and immunological analysis was assessed one week after the last immunization. Mice were immunized and euthanized as described in the methods section. Single cells stimulated with 50sRP-TB was detected interferon-γ (IFN-γ) secretion using an ELISpot assay in (B) lung lymphocyte and (C) splenocyte. (D) Antigen-specific total IgG, (E) IgG1, and (F) titer in serum was measured using ELISA. Data show the mean ± standard deviation from triplicate wells in each group; *p < 0.05, **p < 0.01, ***p < 0.001, and ****p < 0.0001 obtained using unpaired t-test.
4 Discussion
TB remains a life-threatening disease, despite the presence of the approved BCG vaccine, as BCG shows limited protection against TB in adolescents and adults. Therefore, new vaccine candidates against TB are being studied and evaluated in clinical trials. Recently, the severity of COVID-19-TB co-infection was reported, along with its serious economic impact and hazard to global public health (106). Specifically, TB patients co-infected with SARS-CoV-2, showed severe symptoms caused by a “cytokine storm”, particularly within the lungs, heart, and liver (106–108). To prevent simultaneous infection with TB and COVID-19 studies on the development of vaccines against co-infection in several groups are ongoing (109).
The inherent complexities of tuberculosis present significant challenges to vaccine development. In the present study, we aimed to overcome such obstacles by designing a novel adjuvanted, epitope-based vaccine, grounded on antigens that have demonstrated efficacy in in vivo and clinical studies, with the goal of enhancing immunogenicity. Therefore, in this study, we have selected antigens with their vaccine efficacy validated through in vivo studies using animal models or clinical trials the virulence factor of M. tuberculosis with (24–34, 110). Also, we include the antigens GlfT2, Fas, and IniB, based on a previous antigen identification study (35). We predicted B- and T-cell epitopes using these selected antigens and designed peptide-based vaccines. Peptide-based vaccines have the advantage of improved immunogenicity due to their characteristic, aggregated immunodominant epitopes, and reduced side effects (22). The epitope sequences under consideration were distinctly demarcated by their potential to modulate inflammatory responses, either amplifying or mitigating the cascade. By leveraging a comprehensive set of immunoinformatics methodologies, the modulation capacity of each epitope sequence was determined for cytokines, IL-4, IL-6, and IL-10. From this analysis, it was inferred that six B-cell epitopes, five HTL epitopes, and twelve CTL epitopes could potentially serve as inducers of IL-4 secretion. In juxtaposition, eight B-cell epitopes, one singular HTL epitope, and sixteen CTL epitopes were projected to function as inducers of IL-6 secretion. Taken together, a notable fraction of the CTL epitope sequences appeared to be predisposed as inducers for both IL-4 and IL-6, cytokines intrinsically associated with the regulatory mechanisms underlying M. tuberculosis infection. Notably, only the HTL epitope derived from Rv3808c (GlfT2) was validated as an inducer for IL-10, a cytokine representative of the anti-inflammatory prowess in mycobacterial disease dynamics.
In this study, vaccine candidates were linked epitope sequences with AAY and GPGPG linkers to express respective antigens with reduced junctional immunogenicity (111). In mammalian cells, the AAY (Ala-Ala-Tyr) linker acts as the cleavage site and multi-epitope vaccines assembled with the AAY linker showed enhanced epitope presentation and structure stability (112). The Glycine-rich linker, GPGPG, was designed as a universal spacer and is known to induce the HTL immune response (113, 114). An EAAAK linker incorporated between epitopes and adjuvants improves the bioactivity of the fused proteins and increases the expression level and stability of the vaccine construct (115).
Several bioinformatics tools were employed to assess physicochemical properties, antigenicity, allergenicity, and solubility for epitope-based vaccine design. Expasy ProtParam computes various physicochemical characteristics derived from a protein sequence without additional information. This tool calculates both pI and MW and forecasts amino acid composition, atomic composition, extinction coefficient, estimated half-life, and instability index, among other parameters (116). According to the GRAVY score, which assesses maintenance ability in hydrophilic or hydrophobic environments, all three models displayed negative GRAVY values, suggesting a higher structural stability in a hydrophilic environment. This aspect can be correlated with solubility, critical in determining in vitro protein expression. To enhance immunogenicity, we designed and forecasted vaccine properties involving adjuvants such as griselimycin, HBD3, and 50sRP. As an adjuvant sequence was added into the N- and C-terminus of the epitope sequence, its protein solubility was predicted to be higher than that of the original sequence (although data are not included, the solubility of the original sequence was 0.292).
Furthermore, all models were considered both stable and thermostable. The prediction of secondary and tertiary structures of the target proteins is essential in vaccine development to induce an immune response (117). The 3D structure of our multi-epitope models showed desirable stability after all refining processes and appropriate characteristics based on the results of the Ramachandran plots. Furthermore, based on the Ramachandran plots, which showed that the residues were present in the favorable regions (118), the 50sRP-TB model was the most acceptable.
TLRs is expressed in monocyte, immature DC and macrophage cells (119) and binds to several antigens derived from M. tuberculosis of pathogen itself, resulting development of TLRs targeted vaccine candidates and adjuvants (120). In this study, TLR binding affinity and dynamic simulation of each model were used to predict their immune response induction capacity. The conformational changes in the TLR molecule upon binding the vaccine suggested that the complex can process downstream signal cascades (99). To evaluate conformational changes of vaccine candidate and TLR complex, protein flexibility was examined by the NMA study through various analyzed results. A greater part of the complex individual chains showed higher rigid regions in all models. Binding affinities of the TLR4-MD2 complex-, TLR2-, and TLR3-vaccine-docked complexes were predicted. The binding affinity of the vaccines to the TLR4-MD2 complex, particularly the 50sRP-TB model, suggests significant induction of innate and adaptive immunity. Additionally, vaccine binding affinity with TLR2 and TLR3, which play a crucial role in M. tuberculosis protection following infection, demonstrated that all models elicit a good immune response.
In the context of TB vaccine research, it is imperative to underscore the dual necessity of prevention and therapeutic intervention. Notably, there exists a clinical phenomenon where patients who have ostensibly recovered from TB witness a reactivation or relapse of latent TB. Given this clinical challenge, the emphasis on developing therapeutic vaccines has garnered significant attention. Stemming from this perspective, certain vaccine candidates, derived from foundational research, have showcased both prophylactic and therapeutic potential, subsequently progressing to the clinical evaluation phase, and prominent among these are ID93/GLA-SE, H56:IC31, and VPM1002 (121).
The findings of the present study suggest that vaccine designed with multiple epitopes of TB are potential candidates, as one of the candidates, 50sRP-TB, exhibited soluble characteristic in E. coli and immunogenic properties in a mouse. Although there are no distinct immune correlates of protection, CD4+ T cell response, especially IFN-γ, is one of the important indicators in TB vaccine (122). Based on immunogenicity evaluation, 50sRP-TB elicited IFN-γ secreted T cell response and IgG2b production which is engaged in Th1 response in BCG-primed mice. This result implies that 50sRP-TB is a promising T cell response-inducible vaccine. Moreover, we anticipate protective effectiveness of 50sRP-TB in a M. tuberculosis challenged mouse in the further study.
Although our candidates showed good protein characteristic and immunogenicity, our study had a limitation. Since the vaccine candidate was designed using human MHC epitopes, its effectiveness needs to be evaluated with a humanized mouse model. G.W. et al. reported the reliable immunogenicity and protective efficacy of MP3RP, designed in silico in the lungs and spleen of a humanized mouse model to overcome the limitation posed by differences in human and mouse MHC allele (123). However, they also have several drawbacks when evaluating vaccine efficacy. Due to the distinction in antigen presentation between murine MHC (H-2) and human MHC (HLA), an MHC-humanized mouse model has been recently adopted in research to evaluate MHC-restricted epitope-based vaccines (110, 123, 124). Based on these studies, going forward, for the development of a preventive and therapeutic vaccine for TB, the development of a preventive and therapeutic vaccine for TB, this proposed vaccine will be validated for its efficacy in in vivo studies in a suitable mouse model.
Data availability statement
The original contributions presented in the study are included in the article/Supplementary Material. Further inquiries can be directed to the corresponding author.
Ethics statement
The animal study was approved by Laboratory Animal Welfare and Ethics Committee, Korea Disease Control and Prevention Agency (KDCA), in compliance with Institutional Animal Care and Use Committee guidelines for the care and use of animals (permit number: KDCA-22-035). The study was conducted in accordance with the local legislation and institutional requirements.
Author contributions
J-SY: Data curation, Writing – original draft, Formal analysis, Investigation, Project administration, Writing – review & editing. AK: Formal analysis, Investigation, Writing – review & editing. SK: Investigation, Resources, Writing – review & editing. ES: Investigation, Writing – review & editing. S-JH: Supervision, Writing – review & editing. DK: Supervision, Writing – review & editing. H-SJ: Conceptualization, Project administration, Supervision, Validation, Writing – review & editing.
Funding
The author(s) declare that financial support was received for the research, authorship, and/or publication of this article. This work was supported by grants from the Korea National Institute of Health (No. 6600-6634-313 and No. 6600-6634-329), including those for intramural research (No. KNIH 2020-NI-003-00 and KNIH 2022-NI-070-00).
Conflict of interest
The authors declare that the research was conducted in the absence of any commercial or financial relationships that could be construed as a potential conflict of interest.
Publisher’s note
All claims expressed in this article are solely those of the authors and do not necessarily represent those of their affiliated organizations, or those of the publisher, the editors and the reviewers. Any product that may be evaluated in this article, or claim that may be made by its manufacturer, is not guaranteed or endorsed by the publisher.
Supplementary material
The Supplementary Material for this article can be found online at: https://www.frontiersin.org/articles/10.3389/fimmu.2024.1474346/full#supplementary-material
References
2. Jamwal SV, Mehrotra P, Singh A, Siddiqui Z, Basu A, Rao KVS. Mycobacterial escape from macrophage phagosomes to the cytoplasm represents an alternate adaptation mechanism. Sci Rep. (2016) 6:23089. doi: 10.1038/srep23089
3. Dustin ML. T-cell activation through immunological synapses and kinapses. Immunol Rev. (2008) 221:77–89. doi: 10.1111/j.1600-065X.2008.00589.x
4. Andersen P, Scriba TJ. Moving tuberculosis vaccines from theory to practice. Nat Rev Immunol. (2019) 19:550–62. doi: 10.1038/s41577-019-0174-z
5. De Groot AS, McMurry J, Marcon L, Franco J, Rivera D, Kutzler M, et al. Developing an epitope-driven tuberculosis (Tb) vaccine. Vaccine. (2005) 23:2121–31. doi: 10.1016/j.vaccine.2005.01.059
6. Skeiky YA, Sadoff JC. Advances in tuberculosis vaccine strategies. Nat Rev Microbiol. (2006) 4:469–76. doi: 10.1038/nrmicro1419
7. Shey RA, Ghogomu SM, Esoh KK, Nebangwa ND, Shintouo CM, Nongley NF, et al. In-silico design of a multi-epitope vaccine candidate against onchocerciasis and related filarial diseases. Sci Rep. (2019) 9:4409. doi: 10.1038/s41598-019-40833-x
8. Abubakar I, Pimpin L, Ariti C, Beynon R, Mangtani P, Sterne JA, et al. Systematic review and meta-analysis of the current evidence on the duration of protection by bacillus calmette-guérin vaccination against tuberculosis. Health Technol Assess. (2013) 17:1–372, v-vi. doi: 10.3310/hta17370
9. Hatherill M, Cobelens F. Infant bcg vaccination is beneficial, but not sufficient. Lancet Glob Health. (2022) 10:e1220–e1. doi: 10.1016/s2214-109x(22)00325-4
10. Whittaker E, Nicol MP, Zar HJ, Tena-Coki NG, Kampmann B. Age-related waning of immune responses to bcg in healthy children supports the need for a booster dose of bcg in tb endemic countries. Sci Rep. (2018) 8:15309. doi: 10.1038/s41598-018-33499-4
11. Day CL, Tameris M, Mansoor N, van Rooyen M, de Kock M, Geldenhuys H, et al. Induction and regulation of T-cell immunity by the novel tuberculosis vaccine M72/as01 in South African adults. Am J Respir Crit Care Med. (2013) 188:492–502. doi: 10.1164/rccm.201208-1385OC
12. Montoya J, Solon JA, Cunanan SR, Acosta L, Bollaerts A, Moris P, et al. A randomized, controlled dose-finding phase ii study of the M72/as01 candidate tuberculosis vaccine in healthy ppd-positive adults. J Clin Immunol. (2013) 33:1360–75. doi: 10.1007/s10875-013-9949-3
13. Thacher EG, Cavassini M, Audran R, Thierry AC, Bollaerts A, Cohen J, et al. Safety and immunogenicity of the M72/as01 candidate tuberculosis vaccine in hiv-infected adults on combination antiretroviral therapy: A phase I/ii, randomized trial. Aids. (2014) 28:1769–81. doi: 10.1097/qad.0000000000000343
14. Van Der Meeren O, Hatherill M, Nduba V, Wilkinson RJ, Muyoyeta M, Van Brakel E, et al. Phase 2b controlled trial of M72/as01e vaccine to prevent tuberculosis. New Engl J Med. (2018) 379:1621–34. doi: 10.1056/NEJMoa1803484
15. Tait DR, Hatherill M, van der Meeren O, Ginsberg AM, Van Brakel E, Salaun B, et al. Final analysis of a trial of M72/as01e vaccine to prevent tuberculosis. New Engl J Med. (2019) 381:2429–39. doi: 10.1056/NEJMoa1909953
16. Ismail S, Alsowayeh N, Abbasi HW, Albutti A, Tahir Ul Qamar M, Ahmad S, et al. Pan-genome-assisted computational design of a multi-epitopes-based vaccine candidate against helicobacter cinaedi. Int J Environ Res Public Health. (2022) 19(18). doi: 10.3390/ijerph191811579
17. Albutti A. An integrated computational framework to design a multi-epitopes vaccine against mycobacterium tuberculosis. Sci Rep. (2021) 11:21929. doi: 10.1038/s41598-021-01283-6
18. Nagpal G, Usmani SS, Raghava GPS. A web resource for designing subunit vaccine against major pathogenic species of bacteria. Front Immunol. (2018) 9:2280. doi: 10.3389/fimmu.2018.02280
19. Ruaro-Moreno M, Monterrubio-López GP, Reyes-Gastellou A, Castelán-Vega JA, Jiménez-Alberto A, Aparicio-Ozores G, et al. Design of a multi-epitope vaccine against tuberculosis from mycobacterium tuberculosis pe_Pgrs49 and pe_Pgrs56 proteins by reverse vaccinology. Microorganisms. (2023) 11(9). doi: 10.3390/microorganisms11071647
20. Mustafa AS. In silico analysis and experimental validation of mycobacterium tuberculosis -specific proteins and peptides of mycobacterium tuberculosis for immunological diagnosis and vaccine development. Med Princ Pract. (2013) 22 Suppl 1:43–51. doi: 10.1159/000354206
21. Martinez-Olivares CE, Hernández-Pando R, Mixcoha E. In silico esxg esxh rational epitope selection: candidate epitopes for vaccine design against pulmonary tuberculosis. PLoS One. (2023) 18:e0284264. doi: 10.1371/journal.pone.0284264
22. Gong W, Pan C, Cheng P, Wang J, Zhao G, Wu X. Peptide-based vaccines for tuberculosis. Front Immunol. (2022) 13:830497. doi: 10.3389/fimmu.2022.830497
23. McMurry J, Sbai H, Gennaro ML, Carter EJ, Martin W, De Groot AS. Analyzing mycobacterium tuberculosis proteomes for candidate vaccine epitopes. Tuberculosis (Edinb). (2005) 85:95–105. doi: 10.1016/j.tube.2004.09.005
24. Jenum S, Tonby K, Rueegg CS, Rühwald M, Kristiansen MP, Bang P, et al. A phase I/ii randomized trial of H56:Ic31 vaccination and adjunctive cyclooxygenase-2-inhibitor treatment in tuberculosis patients. Nat Commun. (2021) 12:6774. doi: 10.1038/s41467-021-27029-6
25. Warrier T, Tropis M, Werngren J, Diehl A, Gengenbacher M, Schlegel B, et al. Antigen 85c inhibition restricts mycobacterium tuberculosis growth through disruption of cord factor biosynthesis. Antimicrob Agents Chemother. (2012) 56:1735–43. doi: 10.1128/aac.05742-11
26. Backus KM, Dolan MA, Barry CS, Joe M, McPhie P, Boshoff HI, et al. The three mycobacterium tuberculosis antigen 85 isoforms have unique substrates and activities determined by non-active site regions. J Biol Chem. (2014) 289:25041–53. doi: 10.1074/jbc.M114.581579
27. Wilkie M, Satti I, Minhinnick A, Harris S, Riste M, Ramon RL, et al. A phase I trial evaluating the safety and immunogenicity of a candidate tuberculosis vaccination regimen, Chadox1 85a prime - mva85a boost in healthy uk adults. Vaccine. (2020) 38:779–89. doi: 10.1016/j.vaccine.2019.10.102
28. Jeyanathan M, Fritz DK, Afkhami S, Aguirre E, Howie KJ, Zganiacz A, et al. Aerosol delivery, but not intramuscular injection, of adenovirus-vectored tuberculosis vaccine induces respiratory-mucosal immunity in humans. JCI Insight. (2022) 7(3). doi: 10.1172/jci.insight.155655
29. Refai A, Gritli S, Barbouche MR, Essafi M. Mycobacterium tuberculosis virulent factor esat-6 drives macrophage differentiation toward the pro-inflammatory M1 phenotype and subsequently switches it to the anti-inflammatory M2 phenotype. Front Cell Infect Microbiol. (2018) 8:327. doi: 10.3389/fcimb.2018.00327
30. Mustafa T, Wiker HG, Mørkve O, Sviland L. Reduced apoptosis and increased inflammatory cytokines in granulomas caused by tuberculous compared to non-tuberculous mycobacteria: role of mpt64 antigen in apoptosis and immune response. Clin Exp Immunol. (2007) 150:105–13. doi: 10.1111/j.1365-2249.2007.03476.x
31. Stamm CE, Pasko BL, Chaisavaneeyakorn S, Franco LH, Nair VR, Weigele BA, et al. Screening mycobacterium tuberculosis secreted proteins identifies mpt64 as a eukaryotic membrane-binding bacterial effector. mSphere. (2019) 4(3). doi: 10.1128/mSphere.00354-19
32. Yihao D, Hongyun H, Maodan T. Latency-associated protein rv2660c of mycobacterium tuberculosis augments expression of proinflammatory cytokines in human macrophages by interacting with tlr2. Infect Dis (Lond). (2015) 47:168–77. doi: 10.3109/00365548.2014.982167
33. Hervas-Stubbs S, Majlessi L, Simsova M, Morova J, Rojas MJ, Nouzé C, et al. High frequency of cd4+ T cells specific for the tb10.4 protein correlates with protection against mycobacterium tuberculosis infection. Infect Immun. (2006) 74:3396–407. doi: 10.1128/iai.02086-05
34. Hu Y, Movahedzadeh F, Stoker NG, Coates AR. Deletion of the mycobacterium tuberculosis alpha-crystallin-like hspx gene causes increased bacterial growth in vivo. Infect Immun. (2006) 74:861–8. doi: 10.1128/iai.74.2.861-868.2006
35. Bettencourt P, Müller J, Nicastri A, Cantillon D, Madhavan M, Charles PD, et al. Identification of antigens presented by mhc for vaccines against tuberculosis. NPJ Vaccines. (2020) 5:2. doi: 10.1038/s41541-019-0148-y
36. Woodworth JS, Cohen SB, Moguche AO, Plumlee CR, Agger EM, Urdahl KB, et al. Subunit vaccine H56/caf01 induces a population of circulating cd4 T cells that traffic into the mycobacterium tuberculosis-infected lung. Mucosal Immunol. (2017) 10:555–64. doi: 10.1038/mi.2016.70
37. Aagaard C, Hoang T, Dietrich J, Cardona PJ, Izzo A, Dolganov G, et al. A Multistage Tuberculosis Vaccine That Confers Efficient Protection before and after Exposure. Nat Med. (2011) 17:189–94. doi: 10.1038/nm.2285
38. Vasilyev K, Shurygina AP, Zabolotnykh N, Sergeeva M, Romanovskaya-Romanko E, Pulkina A, et al. Enhancement of the local cd8(+) T-cellular immune response to mycobacterium tuberculosis in bcg-primed mice after intranasal administration of influenza vector vaccine carrying tb10.4 and hspx antigens. Vaccines (Basel). (2021) 9(11). doi: 10.3390/vaccines9111273
39. Sergeeva M, Romanovskaya-Romanko E, Zabolotnyh N, Pulkina A, Vasilyev K, Shurigina AP, et al. Mucosal influenza vector vaccine carrying tb10.4 and hspx antigens provides protection against mycobacterium tuberculosis in mice and Guinea pigs. Vaccines. (2021) 9:394. doi: 10.3390/vaccines9040394
40. Sali M, Di Sante G, Cascioferro A, Zumbo A, Nicolò C, Donà V, et al. Surface expression of mpt64 as a fusion with the pe domain of pe_Pgrs33 enhances mycobacterium bovis bcg protective activity against mycobacterium tuberculosis in mice. Infect Immun. (2010) 78:5202–13. doi: 10.1128/iai.00267-10
41. Saha S, Raghava GPS. Bcepred: prediction of continuous B-cell epitopes in antigenic sequences using physico-chemical properties. Artif Immune Syst. (2004), 197–204. doi: 10.1007/978-3-540-30220-9_16
42. Jespersen MC, Peters B, Nielsen M, Marcatili P. Bepipred-2.0: improving sequence-based B-cell epitope prediction using conformational epitopes. Nucleic Acids Res. (2017) 45:W24–W9. doi: 10.1093/nar/gkx346
43. Saha S, Raghava GP. Prediction of continuous B-cell epitopes in an antigen using recurrent neural network. Proteins. (2006) 65:40–8. doi: 10.1002/prot.21078
44. Nielsen M, Lund O, Buus S, Lundegaard C. Mhc class ii epitope predictive algorithms. Immunology. (2010) 130:319–28. doi: 10.1111/j.1365-2567.2010.03268.x
45. Zhang Q, Wang P, Kim Y, Haste-Andersen P, Beaver J, Bourne PE, et al. Immune epitope database analysis resource (Iedb-ar). Nucleic Acids Res. (2008) 36:W513–8. doi: 10.1093/nar/gkn254
46. Dhanda SK, Vir P, Raghava GP. Designing of interferon-gamma inducing mhc class-ii binders. Biol Direct. (2013) 8:30. doi: 10.1186/1745-6150-8-30
47. Lata S, Bhasin M, Raghava GP. Application of machine learning techniques in predicting mhc binders. Methods Mol Biol. (2007) 409:201–15. doi: 10.1007/978-1-60327-118-9_14
48. Lin PL, Flynn JL. Cd8 T cells and mycobacterium tuberculosis infection. Semin Immunopathol. (2015) 37:239–49. doi: 10.1007/s00281-015-0490-8
49. de Martino M, Lodi L, Galli L, Chiappini E. Immune response to mycobacterium tuberculosis: A narrative review. Front Pediatr. (2019) 7:350. doi: 10.3389/fped.2019.00350
50. Larsen MV, Lundegaard C, Lamberth K, Buus S, Lund O, Nielsen M. Large-scale validation of methods for cytotoxic T-lymphocyte epitope prediction. BMC Bioinf. (2007) 8:424. doi: 10.1186/1471-2105-8-424
51. Larsen MV, Lundegaard C, Lamberth K, Buus S, Brunak S, Lund O, et al. An integrative approach to ctl epitope prediction: A combined algorithm integrating mhc class I binding, tap transport efficiency, and proteasomal cleavage predictions. Eur J Immunol. (2005) 35:2295–303. doi: 10.1002/eji.200425811
52. Bibi S, Ullah I, Zhu B, Adnan M, Liaqat R, Kong W-B, et al. In silico analysis of epitope-based vaccine candidate against tuberculosis using reverse vaccinology. Sci Rep. (2021) 11:1249. doi: 10.1038/s41598-020-80899-6
53. Kling A, Lukat P, Almeida DV, Bauer A, Fontaine E, Sordello S, et al. Targeting dnan for tuberculosis therapy using novel griselimycins. Science. (2015) 348:1106–12. doi: 10.1126/science.aaa4690
54. Funderburg N, Lederman MM, Feng Z, Drage MG, Jadlowsky J, Harding CV, et al. Human -defensin-3 activates professional antigen-presenting cells via toll-like receptors 1 and 2. Proc Natl Acad Sci U.S.A. (2007) 104:18631–5. doi: 10.1073/pnas.0702130104
55. Tani K, Murphy WJ, Chertov O, Salcedo R, Koh CY, Utsunomiya I, et al. Defensins act as potent adjuvants that promote cellular and humoral immune responses in mice to a lymphoma idiotype and carrier antigens. Int Immunol. (2000) 12:691–700. doi: 10.1093/intimm/12.5.691
56. Lee SJ, Shin SJ, Lee MH, Lee M-G, Kang TH, Park WS, et al. A potential protein adjuvant derived from mycobacterium tuberculosis rv0652 enhances dendritic cells-based tumor immunotherapy. PLoS One. (2014) 9:e104351. doi: 10.1371/journal.pone.0104351
57. Dhanda SK, Gupta S, Vir P, Raghava GPS. Prediction of il4 inducing peptides. Clin Dev Immunol. (2013) 2013:263952. doi: 10.1155/2013/263952
58. Dhall A, Patiyal S, Sharma N, Usmani SS, Raghava GPS. A web-based method for the identification of il6-based immunotoxicity in vaccine candidates. Methods Mol Biol. (2023) 2673:317–27. doi: 10.1007/978-1-0716-3239-0_22
59. Dhall A, Patiyal S, Sharma N, Usmani SS, Raghava GPS. Computer-aided prediction and design of il-6 inducing peptides: il-6 plays a crucial role in Covid-19. Briefings Bioinf. (2020) 22:936–45. doi: 10.1093/bib/bbaa259
60. Nagpal G, Usmani SS, Dhanda SK, Kaur H, Singh S, Sharma M, et al. Computer-aided designing of immunosuppressive peptides based on il-10 inducing potential. Sci Rep. (2017) 7:42851. doi: 10.1038/srep42851
61. Lemes MR, Rodrigues TCV, Jaiswal AK, Tiwari S, Sales-Campos H, Andrade-Silva LE, et al. In silico designing of a recombinant multi-epitope antigen for leprosy diagnosis. J Genet Eng Biotechnol. (2022) 20:128. doi: 10.1186/s43141-022-00411-7
62. Medha, Bhatt P, Priyanka, Sharma M, Sharma S. Prediction and identification of T cell epitopes of covid-19 with balanced cytokine response for the development of peptide based vaccines. In Silico Pharmacol. (2021) 9:40. doi: 10.1007/s40203-021-00098-7
63. Doytchinova IA, Flower DR. Vaxijen: A server for prediction of protective antigens, tumour antigens and subunit vaccines. BMC Bioinf. (2007) 8:4. doi: 10.1186/1471-2105-8-4
64. Magnan CN, Zeller M, Kayala MA, Vigil A, Randall A, Felgner PL, et al. High-throughput prediction of protein antigenicity using protein microarray data. Bioinformatics. (2010) 26:2936–43. doi: 10.1093/bioinformatics/btq551
65. Gupta S, Kapoor P, Chaudhary K, Gautam A, Kumar R, Raghava GP. In silico approach for predicting toxicity of peptides and proteins. PLoS One. (2013) 8:e73957. doi: 10.1371/journal.pone.0073957
66. Dimitrov I, Naneva L, Doytchinova I, Bangov I. Allergenfp: allergenicity prediction by descriptor fingerprints. Bioinformatics. (2014) 30:846–51. doi: 10.1093/bioinformatics/btt619
67. Hebditch M, Carballo-Amador MA, Charonis S, Curtis R, Warwicker J. Protein-sol: A web tool for predicting protein solubility from sequence. Bioinf (Oxford England). (2017) 33:3098–100. doi: 10.1093/bioinformatics/btx345
68. McGuffin LJ, Bryson K, Jones DT. The psipred protein structure prediction server. Bioinformatics. (2000) 16:404–5. doi: 10.1093/bioinformatics/16.4.404
69. Yang J, Yan R, Roy A, Xu D, Poisson J, Zhang Y. The I-tasser suite: protein structure and function prediction. Nat Methods. (2015) 12:7–8. doi: 10.1038/nmeth.3213
70. Heo L, Park H, Seok C. Galaxyrefine: protein structure refinement driven by side-chain repacking. Nucleic Acids Res. (2013) 41:W384–8. doi: 10.1093/nar/gkt458
71. Rampage. Cambridge: Department of Biochemistry, University of Cambridge. Available at: http://mordred.bioc.cam.ac.uk/~rapper/rampgae.php.
72. Lovell SC, Davis IW, Arendall WB III, de Bakker PIW, Word JM, Prisant MG, et al. Structure validation by Cα Geometry: ϕ,Ψ and Cβ Deviation. Proteins: Structure Function Bioinf. (2003) 50:437–50. doi: 10.1002/prot.10286
73. Ponomarenko J, Bui H-H, Li W, Fusseder N, Bourne PE, Sette A, et al. Ellipro: A new structure-based tool for the prediction of antibody epitopes. BMC Bioinf. (2008) 9:514. doi: 10.1186/1471-2105-9-514
74. Kathwate GH. In silico design and characterization of multi-epitopes vaccine for sars-cov2 from its spike protein. Int J Pept Res Ther. (2022) 28:37. doi: 10.1007/s10989-021-10348-z
75. van Zundert GCP, Rodrigues JPGLM, Trellet M, Schmitz C, Kastritis PL, Karaca E, et al. The haddock2.2 web server: user-friendly integrative modeling of biomolecular complexes. J Mol Biol. (2016) 428:720–5. doi: 10.1016/j.jmb.2015.09.014
76. López-Blanco JR, Aliaga JI, Quintana-Ortí ES, Chacón P. Imods: internal coordinates normal mode analysis server. Nucleic Acids Res. (2014) 42:W271–6. doi: 10.1093/nar/gku339
77. Grote A, Hiller K, Scheer M, Münch R, Nörtemann B, Hempel DC, et al. Jcat: A novel tool to adapt codon usage of a target gene to its potential expression host. Nucleic Acids Res. (2005) 33:W526–31. doi: 10.1093/nar/gki376
78. Abraham Peele K, Srihansa T, Krupanidhi S, Ayyagari VS, Venkateswarulu TC. Design of Multi-Epitope Vaccine Candidate against Sars-Cov-2: A in-Silico Study. J Biomol Struct Dyn. (2021) 39:3793–801. doi: 10.1080/07391102.2020.1770127
79. Rapin N, Lund O, Bernaschi M, Castiglione F. Computational immunology meets bioinformatics: the use of prediction tools for molecular binding in the simulation of the immune system. PLoS One. (2010) 5:e9862. doi: 10.1371/journal.pone.0009862
80. Evangelista FMD, van Vliet AHM, Lawton SP, Betson M. In silico design of a polypeptide as a vaccine candidate against ascariasis. Sci Rep. (2023) 13:3504. doi: 10.1038/s41598-023-30445-x
81. Golshani M, Vaeznia N, Sahmani M, Bouzari S. In silico analysis of brucella abortus omp2b and in vitro expression of somp2b. Clin Exp Vaccine Res. (2016) 5:75–82. doi: 10.7774/cevr.2016.5.1.75
82. Kang TG, Kwon KW, Kim K, Lee I, Kim MJ, Ha SJ, et al. Viral coinfection promotes tuberculosis immunopathogenesis by type I ifn signaling-dependent impediment of th1 cell pulmonary influx. Nat Commun. (2022) 13:3155. doi: 10.1038/s41467-022-30914-3
83. Khan Z, Ualiyeva D, Amissah OB, Sapkota S, Hameed HMA, Zhang T. Insight into novel anti-tuberculosis vaccines by using immunoinformatics approaches. Front Microbiol. (2022) 13:866873. doi: 10.3389/fmicb.2022.866873
84. Cheng P, Wang L, Gong W. In silico analysis of peptide-based biomarkers for the diagnosis and prevention of latent tuberculosis infection. Front Microbiol. (2022) 13:947852. doi: 10.3389/fmicb.2022.947852
85. Pooran A, Davids M, Nel A, Shoko A, Blackburn J, Dheda K. Il-4 subverts mycobacterial containment in mycobacterium tuberculosis-infected human macrophages. Eur Respir J. (2019) 54:1802242. doi: 10.1183/13993003.02242-2018
86. Rook GAW, Hernandez-Pando R, Dheda K, Teng Seah G. Il-4 in tuberculosis: implications for vaccine design. Trends Immunol. (2004) 25:483–8. doi: 10.1016/j.it.2004.06.005
87. Boni FG, Hamdi I, Koundi LM, Shrestha K, Xie J. Cytokine storm in tuberculosis and il-6 involvement. Infection Genet Evol. (2022) 97:105166. doi: 10.1016/j.meegid.2021.105166
88. Martinez AN, Mehra S, Kaushal D. Role of interleukin 6 in innate immunity to mycobacterium tuberculosis infection. J Infect Dis. (2013) 207:1253–61. doi: 10.1093/infdis/jit037
89. Usmani SS, Bedi G, Samuel JS, Singh S, Kalra S, Kumar P, et al. Thpdb: database of fda-approved peptide and protein therapeutics. PLoS One. (2017) 12:e0181748. doi: 10.1371/journal.pone.0181748
90. Usmani SS, Kumar R, Kumar V, Singh S, Raghava GPS. Antitbpdb: A knowledgebase of anti-tubercular peptides. Database (Oxford). (2018) 2018. doi: 10.1093/database/bay025
91. Mirzadeh A, Kobakhidze G, Vuillemot R, Jonic S, Rouiller I. In silico prediction, characterization, docking studies and molecular dynamics simulation of human P97 in complex with P37 cofactor. BMC Mol Cell Biol. (2022) 23:39. doi: 10.1186/s12860-022-00437-2
92. Messaoudi A, Belguith H, Ben Hamida J. Homology modeling and virtual screening approaches to identify potent inhibitors of veb-1 Β-lactamase. Theor Biol Med Model. (2013) 10:22–. doi: 10.1186/1742-4682-10-22
93. Kawasaki T, Kawai T. Toll-like receptor signaling pathways. Front Immunol. (2014) 5:461. doi: 10.3389/fimmu.2014.00461
94. Abel B, Thieblemont N, Quesniaux VJ, Brown N, Mpagi J, Miyake K, et al. Toll-like receptor 4 expression is required to control chronic mycobacterium tuberculosis infection in mice. J Immunol. (2002) 169:3155–62. doi: 10.4049/jimmunol.169.6.3155
95. Penn-Nicholson A, Tameris M, Smit E, Day TA, Musvosvi M, Jayashankar L, et al. Safety and immunogenicity of the novel tuberculosis vaccine id93 + Gla-se in bcg-vaccinated healthy adults in South Africa: A randomised, double-blind, placebo-controlled phase 1 trial. Lancet Respir Med. (2018) 6:287–98. doi: 10.1016/s2213-2600(18)30077-8
96. Ali A, Mir GJ, Ayaz A, Maqbool I, Ahmad SB, Mushtaq S, et al. In silico analysis and molecular docking studies of natural compounds of withania somnifera against bovine nlrp9. J Mol Modeling. (2023) 29:171. doi: 10.1007/s00894-023-05570-z
97. Shahrear S, Islam A. Modeling of mt. P495, an mrna-based vaccine against the phosphate-binding protein psts1 of mycobacterium tuberculosis. Mol Divers. (2023) 27:1613–32. doi: 10.1007/s11030-022-10515-4
98. Padma S, Patra R, Sen Gupta PS, Panda SK, Rana MK, Mukherjee S. Cell surface fibroblast activation protein-2 (Fap2) of fusobacterium nucleatum as a vaccine candidate for therapeutic intervention of human colorectal cancer: an immunoinformatics approach. Vaccines (Basel). (2023) 11(2). doi: 10.3390/vaccines11030525
99. Das NC, Gupta PSS, Panda SK, Rana MK, Mukherjee S. Reverse vaccinology assisted design of a novel multi-epitope vaccine to target wuchereria bancrofti cystatin: an immunoinformatics approach. Int Immunopharmacol. (2023) 115:109639. doi: 10.1016/j.intimp.2022.109639
100. Isa MA, Mustapha A, Qazi S, Raza K, Allamin IA, Ibrahim MM, et al. In silico molecular docking and molecular dynamic simulation of potential inhibitors of 3c-like main proteinase (3clpro) from severe acute respiratory syndrome coronavirus-2 (Sars-Cov-2) using selected African medicinal plants. Adv Traditional Med. (2022) 22:107–23. doi: 10.1007/s13596-020-00523-w
101. Grudinin S, Laine E, Hoffmann A. Predicting protein functional motions: an old recipe with a new twist. Biophys J. (2020) 118:2513–25. doi: 10.1016/j.bpj.2020.03.020
102. Hu W, Spaink HP. The role of tlr2 in infectious diseases caused by mycobacteria: from cell biology to therapeutic target. Biol (Basel). (2022) 11(2). doi: 10.3390/biology11020246
103. Bai W, Liu H, Ji Q, Zhou Y, Liang L, Zheng R, et al. Tlr3 regulates mycobacterial rna-induced il-10 production through the pi3k/akt signaling pathway. Cell Signalling. (2014) 26:942–50. doi: 10.1016/j.cellsig.2014.01.015
104. Hwanga E-H, Kim T-H, Park J-Y, Hong JJ, Kim D-H, Ha S-J, et al. Tlr2 contributes to trigger immune response of pleural mesothelial cells against mycobacterium bovis bcg and M. Tuberculosis Infection. Cytokine. (2017) 95:80–7. doi: 10.1016/j.cyto.2017.02.021
105. Wang C, Zou Q. Prediction of protein solubility based on sequence physicochemical patterns and distributed representation information with deepsolue. BMC Biol. (2023) 21:12. doi: 10.1186/s12915-023-01510-8
106. Shah T, Shah Z, Yasmeen N, Baloch Z, Xia X. Pathogenesis of Sars-Cov-2 and mycobacterium tuberculosis coinfection. Front Immunol. (2022) 13:909011. doi: 10.3389/fimmu.2022.909011
107. Liu B, Li M, Zhou Z, Guan X, Xiang Y. Can we use interleukin-6 (Il-6) blockade for coronavirus disease 2019 (Covid-19)-induced cytokine release syndrome (Crs)? J Autoimmun. (2020) 111:102452. doi: 10.1016/j.jaut.2020.102452
108. Xie P, Ma W, Tang H, Liu D. Severe Covid-19: A review of recent progress with a look toward the future. Front Public Health. (2020) 8:189. doi: 10.3389/fpubh.2020.00189
109. Peng C, Tang F, Wang J, Cheng P, Wang L, Gong W. Immunoinformatic-based multi-epitope vaccine design for co-infection of mycobacterium tuberculosis and Sars-Cov-2. J Pers Med. (2023) 13(1). doi: 10.3390/jpm13010116
110. Kratochvil S, Shen C-H, Lin Y-C, Xu K, Nair U, Da Silva Pereira L, et al. Vaccination in a humanized mouse model elicits highly protective pfcsp-targeting anti-malarial antibodies. Immunity. (2021) 54:2859–76.e7. doi: 10.1016/j.immuni.2021.10.017
111. Khatoon N, Pandey RK, Prajapati VK. Exploring leishmania secretory proteins to design B and T cell multi-epitope subunit vaccine using immunoinformatics approach. Sci Rep. (2017) 7:8285. doi: 10.1038/s41598-017-08842-w
112. Ayyagari VS, CV T, AP K, Srirama K. Design of a multi-epitope-based vaccine targeting M-protein of Sars-Cov2: an immunoinformatics approach. J Biomol Struct Dyn. (2022) 40:2963–77. doi: 10.1080/07391102.2020.1850357
113. Li X, Guo L, Kong M, Su X, Yang D, Zou M, et al. Design and evaluation of a multi-epitope peptide of human metapneumovirus. Intervirology. (2015) 58:403–12. doi: 10.1159/000445059
114. Arai R, Ueda H, Kitayama A, Kamiya N, Nagamune T. Design of the linkers which effectively separate domains of a bifunctional fusion protein. Protein Eng. (2001) 14:529–32. doi: 10.1093/protein/14.8.529
115. Mahmud S, Rafi MO, Paul GK, Promi MM, Shimu MSS, Biswas S, et al. Designing a multi-epitope vaccine candidate to combat mers-cov by employing an immunoinformatics approach. Sci Rep. (2021) 11:15431. doi: 10.1038/s41598-021-92176-1
116. Chauhan R, Bhattacharya J, Solanki R, Ahmad FJ, Alankar B, Kaur H. Gud-ve visualization tool for physicochemical properties of proteins. MethodsX. (2023) 10:102226. doi: 10.1016/j.mex.2023.102226
117. Meza B, Ascencio F, Sierra-Beltrán AP, Torres J, Angulo C. A novel design of a multi-antigenic, multistage and multi-epitope vaccine against helicobacter pylori: an in silico approach. Infect Genet Evol. (2017) 49:309–17. doi: 10.1016/j.meegid.2017.02.007
118. Gorai S, Das NC, Gupta PSS, Panda SK, Rana MK, Mukherjee S. Designing efficient multi-epitope peptide-based vaccine by targeting the antioxidant thioredoxin of bancroftian filarial parasite. Infect Genet Evol. (2022) 98:105237. doi: 10.1016/j.meegid.2022.105237
119. Vaure C, Liu Y. A comparative review of toll-like receptor 4 expression and functionality in different animal species. Front Immunol. (2014) 5:316. doi: 10.3389/fimmu.2014.00316
120. Pulendran B S, Arunachalam P, O’Hagan DT. Emerging concepts in the science of vaccine adjuvants. Nat Rev Drug Discovery. (2021) 20:454–75. doi: 10.1038/s41573-021-00163-y
121. Holleman M. The Pipeline of Tuberculosis Vaccines (2024). Available online at: https://www.tbvi.eu/what-we-do/pipeline-of-vaccines/ (Accessed January 8, 2024).
122. Nemes E, Fiore-Gartland A, Boggiano C, Coccia M, D'Souza P, Gilbert P, et al. The quest for vaccine-induced immune correlates of protection against tuberculosis. Vaccine Insights. (2022) 1:165–81. doi: 10.18609/vac/2022.027
123. Gong W, Liang Y, Mi J, Jia Z, Xue Y, Wang J, et al. Peptides-based vaccine mp3rt induced protective immunity against mycobacterium tuberculosis infection in a humanized mouse model. Front Immunol. (2021) 12:666290. doi: 10.3389/fimmu.2021.666290
124. Wada Y, Nithichanon A, Nobusawa E, Moise L, Martin WD, Yamamoto N, et al. A humanized mouse model identifies key amino acids for low immunogenicity of H7n9 vaccines. Sci Rep. (2017) 7:1283. doi: 10.1038/s41598-017-01372-5
Keywords: tuberculosis (TB), peptide-based vaccine, immunoinformatics analysis, multi-epitope, adjuvanted vaccine
Citation: Yun J-S, Kim AR, Kim SM, Shin E, Ha S-J, Kim D and Jeong H-S (2024) In silico analysis for the development of multi-epitope vaccines against Mycobacterium tuberculosis. Front. Immunol. 15:1474346. doi: 10.3389/fimmu.2024.1474346
Received: 01 August 2024; Accepted: 24 October 2024;
Published: 18 November 2024.
Edited by:
T. Mark Doherty, GlaxoSmithKline, BelgiumReviewed by:
Maria Agallou, Pasteur Hellenic Institute, GreeceMark Endsley, University of Texas Medical Branch at Galveston, United States
Copyright © 2024 Yun, Kim, Kim, Shin, Ha, Kim and Jeong. This is an open-access article distributed under the terms of the Creative Commons Attribution License (CC BY). The use, distribution or reproduction in other forums is permitted, provided the original author(s) and the copyright owner(s) are credited and that the original publication in this journal is cited, in accordance with accepted academic practice. No use, distribution or reproduction is permitted which does not comply with these terms.
*Correspondence: Hye-Sook Jeong, jeongnih@korea.kr