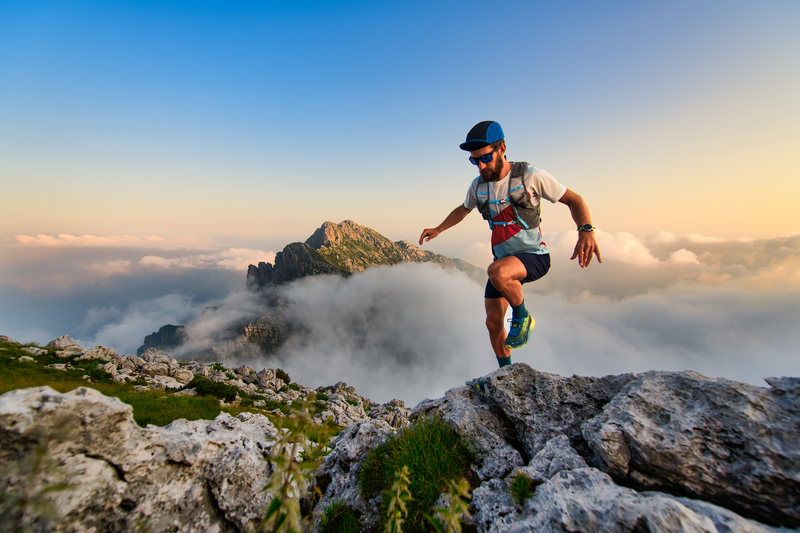
95% of researchers rate our articles as excellent or good
Learn more about the work of our research integrity team to safeguard the quality of each article we publish.
Find out more
ORIGINAL RESEARCH article
Front. Immunol. , 20 September 2024
Sec. Cancer Immunity and Immunotherapy
Volume 15 - 2024 | https://doi.org/10.3389/fimmu.2024.1453232
This article is part of the Research Topic Community Series in Novel Biomarkers in Tumor Immunity and Immunotherapy: Volume II View all 13 articles
Objective: Develop a predictive model utilizing weakly supervised deep learning techniques to accurately forecast major pathological response (MPR) in patients with resectable non-small cell lung cancer (NSCLC) undergoing neoadjuvant chemoimmunotherapy (NICT), by leveraging whole slide images (WSIs).
Methods: This retrospective study examined pre-treatment WSIs from 186 patients with non-small cell lung cancer (NSCLC), using a weakly supervised learning framework. We employed advanced deep learning architectures, including DenseNet121, ResNet50, and Inception V3, to analyze WSIs on both micro (patch) and macro (slide) levels. The training process incorporated innovative data augmentation and normalization techniques to bolster the robustness of the models. We evaluated the performance of these models against traditional clinical predictors and integrated them with a novel pathomics signature, which was developed using multi-instance learning algorithms that facilitate feature aggregation from patch-level probability distributions.
Results: Univariate and multivariable analyses confirmed histology as a statistically significant prognostic factor for MPR (P-value< 0.05). In patch model evaluations, DenseNet121 led in the validation set with an area under the curve (AUC) of 0.656, surpassing ResNet50 (AUC = 0.626) and Inception V3 (AUC = 0.654), and showed strong generalization in external testing (AUC = 0.611). Further evaluation through visual inspection of patch-level data integration into WSIs revealed XGBoost’s superior class differentiation and generalization, achieving the highest AUCs of 0.998 in training and robust scores of 0.818 in validation and 0.805 in testing. Integrating pathomics features with clinical data into a nomogram yielded AUC of 0.819 in validation and 0.820 in testing, enhancing discriminative accuracy. Gradient-weighted Class Activation Mapping (Grad-CAM) and feature aggregation methods notably boosted the model’s interpretability and feature modeling.
Conclusion: The application of weakly supervised deep learning to WSIs offers a powerful tool for predicting MPR in NSCLC patients treated with NICT.
The employment of neoadjuvant chemoimmunotherapy (NICT) has risen as an effective method for managing resectable non-small cell lung cancer (NSCLC). A number of research has explored its viability and efficacy, showcasing that this strategy can enhance pathological response rates and complete tumor removal. Furthermore, it assists in managing microscopically invisible metastases, thus favorably influencing patient outcomes (1–6).
In many trials focusing on neoadjuvant immunotherapy for NSCLC, major pathological response (MPR) is considered a key predictor for overall survival (OS) and disease-free survival (DFS). However, the rates of MPR observed in current clinical research on NICT display a wide variance, ranging from 18% to 83% (1, 3–5, 7–14). This disparity underscores that not all patients derive benefit from NICT; indeed, ineffective treatment may lead to delays in surgical intervention and an increased likelihood of immune-related side effects. Consequently, crafting a dependable predictive model for MPR response to NICT in patients with resectable NSCLC is crucial, offering the potential to tailor treatments more effectively and enhance patient outcomes.
Tissue specimens stained with Hematoxylin and Eosin (H&E) contain a wealth of useful information for routine histopathological analysis. Artificial intelligence (AI) is increasingly used to analyze H&E stained histopathological images for differential diagnosis and prognosis prediction in NSCLC studies, enhancing the evaluation of conventional histological slides (15–22). This approach holds immense potential for disease research, as AI algorithms can assist clinicians and pathologists in their decision-making by analyzing whole slide images (WSIs).
Weakly supervised learning has garnered widespread attention due to its significant advantage in reducing the workload of manual annotation and has been gradually applied in the field of pathological image analysis (23–25). The classic patch-based weakly supervised method provides a specific workflow for processing histological images. Due to the expansive dimensions of WSIs, segmentation into smaller tiles is necessary for processing, with an averaging method subsequently aggregating the tile-level predictions for each slide (26). This approach has introduced a new level of flexibility and application prospects in the realm of weakly supervised learning for pathological image analysis.
In this study, we developed a weakly supervised deep learning model utilizing pre-treatment WSIs to predict MPR in patients undergoing NICT for NSCLC. The model’s predictions can serve as a reference for physicians to enhance treatment planning.
The flowchart illustrating the cohort selection process for this study is presented in Figure 1. This study initially enrolled 302 patients who received NICT followed by surgical intervention from November 24, 2020, to March 10, 2024. However, 116 patients were subsequently excluded based on predefined criteria. All pre-treatment H&E-stained slides were digitized into WSIs using a WISLEAP scanner and then converted to NDPI format via NDPView2 software. Ultimately, 186 patients contributing 212 WSIs diagnosed with NSCLC were retrospectively selected from three institutions. The allocation of patients across these institutions was as follows: 150 from Shandong Cancer Hospital (Database 1), 23 from Shanxi Cancer Hospital (Database 2), and 13 from the First People’s Hospital of Jining City (Database 3). Within the training cohort, samples were apportioned into a training subset and an internal validation subset at a 7:3 ratio. Due to sample size constraints, data from Databases 2 and 3 were amalgamated to constitute the test datasets.
Full details regarding the treatment protocols can be found in Supplementary Data Sheet 2. This study was conducted in accordance with the 8th edition of the American Joint Committee on Cancer (AJCC) Tumor, Node, Metastasis (TNM) staging system. MPR was defined as the presence of less than 10% viable tumor cells in the pathological examination of the surgical specimen (27). The conduct of this study was in strict compliance with the principles of the Declaration of Helsinki and received ethical clearance from the institutional review board (number: SDTHEC2024002010). This study, which was retrospectively registered with the ResearchRegistry (registration ID: researchregistry10216). Additionally, the study received further ethical approvals from the Institutional Review Board of the First People’s Hospital of Jining City (approval number: JNRM-2024-KY-037) and the Medical Ethics Committee of Shaanxi Cancer Hospital [approval number: Ethics Review No. 39 (2024)]. Owing to its retrospective design and the absence of any risk to participants, the need for informed consent was duly waived. Figure 2 depicts the comprehensive workflow of our study.
In processing the WSIs, which typically span dimensions of approximately 100,000 x 50,000 pixels, we utilized a 20x magnification to capture these images, resulting in a pixel resolution of about 0.5 μm/pixel. The WSIs were subsequently divided into smaller segments of 512x512 pixels each. By employing a series of image processing techniques, including grayscale conversion, Otsu’s thresholding, and morphological operations for background removal, we efficiently eliminated all white backgrounds from these patches. This process resulted in over 17,000 distinct, non-overlapping tiles.
During the model’s training phase, we incorporated online data augmentation strategies to increase the dataset’s variability. This included random horizontal and vertical flips of the image patches. To maintain a standardized input size, we meticulously performed center cropping to adjust the dimensions to 224 x 224 pixels, and specifically to 299 x 299 pixels for the Inception V3 architecture. Additionally, Z-score normalization was applied to the RGB channels to normalize the distribution of pixel values.
In our study, we employed deep learning algorithms to facilitate predictive analysis at both the micro (patch) and macro (WSI) levels. The segmentation of WSIs into smaller, discrete patches was undertaken, ensuring that each patch from a single specimen uniformly bore the same MPR designation. To predict outcomes at the patch level, we meticulously evaluated three prominent neural network architectures: DenseNet121, ResNet50, and Inception V3. The objective was to ascertain the precision with which each patch could be classified into a category mirroring its overarching WSI classification.
To improve the generalizability of our pathology model, we optimized the learning rate employing a cosine decay algorithm, ensuring a refined and effective adjustment over the training period. This approach is characterized as follows:
In this formulation, sets the minimum learning rate, establishes the maximum learning rate, and denotes the number of epochs in the iterative training process. This learning rate schedule employs a gradual diminution strategy, enabling precise model refinement throughout the training phase.
For further refinement of the training approach and to increase predictive accuracy, we utilized stochastic gradient descent as the optimization technique. Additionally, softmax cross-entropy served as the loss function, aiding in calculating the probability distribution over the intended target classes.
Upon completing the training of our deep learning model, we directed our efforts towards predicting labels and corresponding probabilities for individual patches. Subsequently, these probabilities were aggregated through a classifier to formulate predictions at the WSI level. In our study, we employed the densenet121 model to predict labels and obtain corresponding probabilities for each patch, denoted as and , respectively. The prediction probabilities were precisely rounded to two decimal places.
In our study, we developed two machine learning strategies for integrating patch-level probabilities. Firstly, employing histogram feature aggregation for the Probability Label Heatmap (PLH), we categorized each unique numerical value as a “bin,” monitoring the occurrence of data types within these bins. We specifically tallied the frequencies of and in each bin and applied min-max normalization across all features. This process culminated in the generation of and , enhancing data interpretability. Secondly, we implemented the Bag of Words (BoW) feature aggregation method, initiating with a comprehensive dictionary comprising unique dataset elements. Each patch was vectorized according to the presence of these elements, with further refinement via term frequency-inverse document frequency (TF-IDF) transformation, emphasizing the significance of unique, informative features. This approach yielded a BoW feature representation for each patch, effectively encapsulating feature presence and relevance. The final BoW features, denoted as and offered a comprehensive, weighted overview, priming them for advanced analytical applications.
In the final phase of our feature fusion approach, based on multi-instance learning, we integrated previously derived features: , , , and . To accomplish this integration, we employed a feature concatenation method symbolized by , effectively merging these distinct feature sets into a single, comprehensive feature vector. The specific formula for this concatenation is as follows:
In our study, we developed a nuanced pathomics signature by integrating patch-level predictions, probability histograms, and TF-IDF features to create individualized patient profiles. To refine feature selection, we employed the Pearson correlation coefficient, retaining only one feature from each pair with a correlation exceeding 0.9. The model integrates a diverse array of machine learning methodologies, encompassing Logistic Regression (LR), Support Vector Machine (SVM), Random Forest, LightGBM, ExtraTrees, and XGBoost. Together, these techniques form what is termed the pathomics signature.
Model accuracy was evaluated through receiver operating characteristic (ROC) curves. Statistical analyses, comprising independent sample t-tests for continuous variables and χ² tests for discrete variables, were performed to evaluate differences in patients’ clinical characteristics. Univariate and multivariate logistic regression analyses were utilized to examine clinical characteristics, retaining those with P-values< 0.05 in the combined model for further use. For practical clinical application, we integrated significant clinical characteristics with the pathomics signature into a combined model, which is visualized through a nomogram for ease of interpretation.
All selected patients were regularly followed up through outpatient visits and telephone check-ins. During the follow-up period, they underwent routine physical examinations and chest-enhanced computed tomography (CT) scans, with additional tests such as positron emission tomography-computed tomography (PET-CT), ultrasound, bronchoscopy, magnetic resonance imaging (MRI), or whole-body bone scans as necessary. For patients with more than one month since the last recorded entry in the case system, we conducted telephone follow-ups to assess their condition and survival status. The last follow-up for all patients was conducted on August 18, 2024, with a median follow-up time of 21 months (range: 3-44 months). In our study, DFS was defined as the interval from the date of curative lung cancer resection to the first occurrence of recurrence, metastasis, death from any cause, or the last follow-up. OS was defined as the time from the initiation of treatment to death from any cause or the last follow-up. Kaplan-Meier analysis was used to estimate DFS and OS, and comparisons between groups were performed using the log-rank test.
The deep learning models in this study were trained on robust hardware, including an Intel i9-14900k CPU, 64GB of RAM, and an NVIDIA RTX 4090 GPU. For our analysis, we employed a blend of software tools alongside custom scripts to achieve precise and efficient processing. Medical image segmentation and processing were facilitated using ITK-SNAP v3.8.0. Our computational work, spanning from modeling to data analysis, was primarily executed in Python v3.7.12, leveraging essential libraries such as PyTorch v1.8.0 for deep learning algorithms, scikit-learn v1.0.2 for machine learning.
Table 1 summarizes the baseline characteristics of our study cohort. Notably, the MPR rate was 63.4%(118/186). The cohort predominantly consisted of male patients, representing 89.2%(166/186), with the majority undergoing 2 to 3 cycles of neoadjuvant therapy, which accounted for 89.8%(167/186). Squamous cell carcinoma emerged as the leading histological type, comprising 72.6%(135/186) of cases, and most patients were classified under clinical TNM stage III (136/186, 73.1%). Through detailed univariate and multivariable analysis of clinical features, histology was identified as an independent prognostic factor for MPR, showing statistical significance with a P-value below 0.05, as illustrated in Table 2.
Table 2. Univariable and multivariable analysis for predicting major pathological response in non-small cell lung cancer after neoadjuvant chemoimmunotherapy.
The performance of the clinical model, assessed using the area under the curve (AUC) metric, revealed distinct levels of discrimination capability across various machine learning algorithms and datasets. LR exhibited modest effectiveness in the training set (AUC = 0.735), but its performance significantly declined in the validation set (AUC = 0.484), highlighting a notable reduction in its discriminative power. The SVM algorithm demonstrated superior discrimination in the training set (AUC = 0.871), though it achieved only moderate results in the validation set (AUC = 0.585). Both Random Forest and ExtraTrees algorithms displayed commendable results in the training set (AUC = 0.799 and 0.810, respectively), yet their efficacy was moderate to modest in the validation and test sets. XGBoost demonstrated consistent performance with good results in both the training (AUC = 0.722) and test sets (AUC = 0.714), but it exhibited suboptimal performance in the validation set (AUC = 0.492). LightGBM presented moderate performance across all datasets, with AUC scores of 0.674, 0.563, and 0.667 for the training, validation, and test sets, respectively (Supplementary Figure 1).
Our pathology models’ ability to discern regional features was rigorously evaluated using ROC curves at the patch level. DenseNet121, among the models assessed, distinguished itself in the validation set, achieving an AUC of 0.656 (95% CI: 0.651-0.661), thereby surpassing both ResNet50 (AUC = 0.626, 95% CI: 0.621-0.631) and Inception V3 (AUC = 0.654, 95% CI: 0.649-0.660). Additionally, DenseNet121 demonstrated commendable generalization with an AUC of 0.611 (95% CI: 0.603-0.619) in the external test set. The comparative analysis of these models is illustrated in Figures 3A–C.
Figure 3. Prediction model evaluation. (A) Patch-level area under the curve (AUC) for the DenseNet121 prediction model across cohorts; (B) Patch-level AUC for the Resnet50 prediction model; (C) Patch-level AUC for the Inception v3 prediction model; (D) whole slide image (WSI)-level AUC for the prediction model in the training cohort; (E) WSI-level AUC for the prediction model in the validation cohort; (F) WSI-level AUC for the prediction model in the testing cohort.
To further evaluate our model’s effectiveness, we visually inspected the amalgamation of patch-level data into WSIs. Among the machine learning techniques assessed, XGBoost outperformed others, delivering the highest AUC scores in the training and testing phases. With an AUC of 0.998 in the training phase, XGBoost demonstrated exemplary discriminative prowess. In the validation phase, it achieved a robust AUC of 0.818 and maintained a strong AUC of 0.805 in the testing phase, indicating reliable performance. XGBoost’s consistent AUC superiority reveals its remarkable capacity for class differentiation and generalization to unseen data (Figures 3D–F).
Gradient-weighted class activation mapping (Grad-CAM) generates visual maps by tracing gradients in the network’s final convolutional layer, preserving key spatial details relevant to the classification task, details that are often lost in fully connected layers. This technique seamlessly fits into existing neural architectures without necessitating any model modifications or retraining. Figure 4 demonstrates this, by providing a clear depiction of the last convolutional layer’s contribution in the model’s predictive response, enhancing interpretability of the model’s decision-making. Predictive label and probability heatmaps were obtained to assist in the evaluation. As depicted in Figure 5, the prediction heatmap vividly showcases our pathological model’s high accuracy when assessing regional tiles. The results indicate that feature modeling has been notably improved following aggregation via the BoW and PLH processes. This signifies the efficacy of our feature aggregation methodology.
Figure 4. Use of Grad-CAM to illustrate activation in the final convolutional layer of the prediction model.
Figure 5. Probability and prediction heatmap of the prediction model. This image displays the whole slide image (WSI)-level hematoxylin and eosin slide (left), a heatmap of the prediction probabilities for each patch (middle), and the result prediction map for the WSI (right). Major pathological response (MPR) is primarily predicted with a probability label of 1, whereas non-major pathological response (Non-MPR) is predominantly predicted with a probability label of 0.
The nomogram, an integrative tool combining clinical and pathomics information, is effectively illustrated in Figure 6. The assessment of AUC scores for clinical, pathomics, and nomogram signatures indicates that the nomogram consistently secures marginally higher AUC values than the pathomics signature on its own, observed in both validation and test datasets. Notably, the nomogram records an AUC of 0.819 in the validation group and 0.820 in the test group, reflecting an effective amalgamation of features for refined discriminative accuracy. The pathomics signature alone exhibits formidable discriminative strength across all datasets, with an almost perfect AUC of 0.998 in training. The clinical signature, however, reveals a descending trajectory in discriminative capacity, with an AUC drop from 0.799 in training to 0.613 in validation and further to 0.584 in testing, thereby emphasizing the incremental benefit of pathomics feature integration in enhancing model efficacy (Supplementary Table 1, Figure 7A). Utilizing the DeLong test for statistical comparison, the nomogram, which synthesizes clinical and pathomics attributes, demonstrated augmented predictive superiority. The performance elevation of the nomogram over the clinical-only model was statistically significant, registering a P-value less than 0.05, hence confirming the added value of integrating pathomics insights into clinical predictions (Figure 7B).
Figure 6. Clinical nomogram to predict major pathological response in non-small cell lung cancer patients post-neoadjuvant chemoimmunotherapy.
Figure 7. Assessment of model efficacy in forecasting major pathological responses to neoadjuvant chemoimmunotherapy in training, validation, and test cohorts. (A) receiver operating characteristic curves depicting prediction accuracy of signatures; (B) DeLong test comparisons among various signatures.
Figures 8A, B present the DFS and OS curves for patients treated with NICT, comparing the MPR group to the Non-MPR group. The analysis reveals that the MPR group exhibited significantly improved DFS and OS outcomes, with statistically significant differences between the two cohorts.
Figure 8. Kaplan-Meier survival analysis of disease-free survival (DFS) (A) and overall survival (OS) (B) between major pathological response (MPR) and non-major pathological response (Non-MPR) groups.
In this study, our objective was to develop an accurate predictive model for MPR in NSCLC patients undergoing NICT. By integrating machine learning analyses of clinical data, we established a clinical signature grounded in machine learning principles. Moreover, we assessed the predictive value of pathomics data on MPR outcomes. Leveraging a weakly supervised deep learning framework trained on WSIs with multi-instance aggregation, we achieved precise predictions of MPR at the patient level, culminating in the establishment of a pathomics signature. The pinnacle of our study involved merging the derived clinical features with the pathomics signature into a unified nomogram, crafted for extensive interpretability and detailed examination, offering a methodology for MPR prediction in NSCLC patients receiving NICT. This integrated approach represents a significant fusion of clinical insights with advanced machine learning techniques and, to our knowledge, it pioneers the use of WSI for the first-time prediction of MPR in NSCLC patients treated with NICT, setting a new benchmark in the field.
MPR is gaining recognition as a pivotal prognostic marker in resectable NSCLC, particularly when considering the context of NICT. The capability of MPR to accurately mirror the tumor’s response to therapeutic interventions is essential for predicting patient outcomes effectively. Research demonstrates MPR’s link to improved long-term OS among NSCLC patients who receive neoadjuvant chemotherapy, underscoring its significance as both a surrogate endpoint for survival and a critical measure for evaluating neoadjuvant therapy in clinical trials (28). Additionally, comprehensive studies exploring the prognostic relevance of MPR in NSCLC patients undergoing NICT have found a strong correlation with enhanced DFS and OS, supporting the use of MPR as a surrogate marker for survival outcomes in the evaluation of NICT’s effectiveness (2, 4, 6, 29, 30). Our research, in conjunction with these findings, accentuates the crucial role of MPR in assessing the success of neoadjuvant treatment approaches in NSCLC, thereby validating our decision to use MPR as a predictor of NICT’s efficacy in this clinical setting.
In the field of deep learning model development, access to large datasets and high-quality annotations is crucial for training high-performance models. However, the high resolution of WSI presents significant challenges for detailed annotation. Consequently, researchers have developed a new training method using limited annotations, known as weak supervision (31, 32). In the realm of WSI classification under weak supervision, a significant portion of research has predominantly concentrated on employing multiple instance learning (MIL) techniques (33–36). The MIL approach identifies the relative importance of each image patch for model prediction by analyzing histopathological images, allowing the model to autonomously learn to recognize morphological features of diseases without the need for manual annotations. In this research, we applied a weakly supervised learning framework using MIL on pre-treatment WSIs to forecast MPR in NSCLC patients post-NICT, achieving an AUC of 0.998 in training, and demonstrating robust performance with AUCs of 0.818 in validation and 0.805 in testing phases. Additionally, we enhanced our model’s interpretability in decision-making by utilizing GradCAM localization mapping, which facilitated the evaluation through predictive labels and probability heatmaps. GradCAM uniquely enables target localization in models trained using only image labels by incorporating guided backpropagation, precisely determining pixel-level importance in predictive areas, thus offering significant benefits for applications like cancer subtype classification (37–39).
This study has several limitations, including a small sample size and reliance on a retrospective cohort, which may affect the generalizability of our findings. To validate our results and strengthen the conclusions drawn, future research with a larger sample size and a prospective design is essential. Furthermore, the developed model focuses on pathological images and clinical features without incorporating conventional imaging data, such as CT scans, or molecular information like genetic and protein expressions. Acknowledging the dynamic nature of AI models, future iterations will aim to incorporate multidimensional patient data to enhance the performance of model predictions.
Moving forward, our research will focus on several key areas. First, we plan to conduct prospective studies to validate our findings and evaluate the model’s applicability across diverse populations and clinical settings. Additionally, we aim to develop advanced visualization and interpretation tools to improve model transparency and facilitate its use by clinicians in decision-making processes. Finally, we will explore strategies for integrating the predictive model into existing clinical workflows, with an emphasis on feasibility, usability, and acceptance in real-world clinical environments.
The utilization of weakly supervised deep learning for analyzing WSIs provides a potent predictive tool for MPR in NSCLC patients undergoing NICT. By enhancing treatment precision, this model promises not only to improve patient outcomes but also to refine therapeutic strategies. Future work will aim to incorporate extensive multimodal data, further improving the predictive accuracy and robustness of our models.
The raw data supporting the conclusions of this article will be made available by the authors, without undue reservation.
The studies involving humans were approved by Shandong First Medical University Affiliated Cancer Hospital Ethics Committee. The studies were conducted in accordance with the local legislation and institutional requirements. The ethics committee/institutional review board waived the requirement of written informed consent for participation from the participants or the participants’ legal guardians/next of kin because Patient consent was waived due to the retrospective nature of this research.
DH: Conceptualization, Writing – original draft, Writing – review & editing. HL: Conceptualization, Writing – original draft, Writing – review & editing. XZ: Data curation, Formal analysis, Funding acquisition, Writing – review & editing. SF: Data curation, Formal analysis, Writing – review & editing. RW: Investigation, Resources, Software, Visualization, Writing – review & editing. QZ: Investigation, Resources, Software, Visualization, Writing – review & editing. CL: Data curation, Writing – review & editing. ZW: Data curation, Writing – original draft. WH: Project administration, Supervision, Writing – review & editing. SH: Project administration, Supervision, Writing – review & editing.
The author(s) declare financial support was received for the research, authorship, and/or publication of this article. This research was supported by the Shandong Provincial Natural Science Foundation -General Project (No. ZR202103040386) in 2021.
The authors declare that this research was conducted without any commercial or financial relationships that could be interpreted as potential conflicts of interest.
All claims expressed in this article are solely those of the authors and do not necessarily represent those of their affiliated organizations, or those of the publisher, the editors and the reviewers. Any product that may be evaluated in this article, or claim that may be made by its manufacturer, is not guaranteed or endorsed by the publisher.
The Supplementary Material for this article can be found online at: https://www.frontiersin.org/articles/10.3389/fimmu.2024.1453232/full#supplementary-material
1. Cascone T, Leung CH, Weissferdt A, Pataer A, Carter BW, Godoy MCB, et al. Neoadjuvant chemotherapy plus nivolumab with or without ipilimumab in operable non-small cell lung cancer: the phase 2 platform neostar trial. Nat Med. (2023) 29:593–604. doi: 10.1038/s41591-022-02189-0
2. Chen Y, Qin J, Wu Y, Lin Q, Wang J, Zhang W, et al. Does major pathological response after neoadjuvant immunotherapy in resectable nonsmall-cell lung cancers predict prognosis? A systematic review and meta-analysis. Int J Surg. (2023) 109:2794–807. doi: 10.1097/JS9.0000000000000496
3. Forde PM, Spicer J, Lu S, Provencio M, Mitsudomi T, Awad MM, et al. Neoadjuvant nivolumab plus chemotherapy in resectable lung cancer. N Engl J Med. (2022) 386:1973–85. doi: 10.1056/NEJMoa2202170
4. Provencio M, Nadal E, Insa A, Garcia-Campelo MR, Casal-Rubio J, Domine M, et al. Neoadjuvant chemotherapy and nivolumab in resectable non-small-cell lung cancer (Nadim): an open-label, multicenter, single-arm, phase 2 trial. Lancet Oncol. (2020) 21:1413–22. doi: 10.1016/S1470-2045(20)30453-8
5. Shu CA, Gainor JF, Awad MM, Chiuzan C, Grigg CM, Pabani A, et al. Neoadjuvant atezolizumab and chemotherapy in patients with resectable non-small-cell lung cancer: an open-label, multicenter, single-arm, phase 2 trial. Lancet Oncol. (2020) 21:786–95. doi: 10.1016/S1470-2045(20)30140-6
6. Zhao J, Hao S, Li Y, Liu X, Liu Z, Zheng C, et al. Comparative efficacy and safety of neoadjuvant immunotherapy with chemotherapy versus chemotherapy alone in non-small cell lung cancer: A propensity score and inverse probability treatment weighting analysis. Immunotargets Ther. (2023) 12:113–33. doi: 10.2147/ITT.S437911
7. Heymach JV, Mitsudomi T, Harpole D, Aperghis M, Jones S, Mann H, et al. Design and rationale for a phase iii, double-blind, placebo-controlled study of neoadjuvant durvalumab+ Chemotherapy followed by adjuvant durvalumab for the treatment of patients with resectable stages ii and iii non-small-cell lung cancer: the aegean trial. Clin Lung Cancer. (2022) 23:e247–e51. doi: 10.1016/j.cllc.2021.09.010
8. Lu S, Wu L, Zhang W, Zhang P, Wang W, Fang W, et al. Perioperative toripalimab + Platinum-doublet chemotherapy vs chemotherapy in resectable stage II/III non-small cell lung cancer (Nsclc): interim event-free survival (Efs) analysis of the phase iii neotorch study. J Clin Oncol. (2023) 41:425126. doi: 10.1200/JCO.2023.41.36_suppl.425126
9. Qiu F, Fan J, Shao M, Yao J, Zhao L, Zhu L, et al. Two cycles versus three cycles of neoadjuvant sintilimab plus platinum-doublet chemotherapy in patients with resectable non-small-cell lung cancer (Neoscore): A randomized, single center, two-arm phase ii trial. J Clin Oncol. (2022) 40:8500. doi: 10.1200/JCO.2022.40.16_suppl.8500
10. Rothschild SI, Zippelius A, Eboulet EI, Savic Prince S, Betticher D, Bettini A, et al. Sakk 16/14: durvalumab in addition to neoadjuvant chemotherapy in patients with stage IIIa(N2) non-small-cell lung cancer-a multicenter single-arm phase II trial. J Clin Oncol. (2021) 39:2872–80. doi: 10.1200/JCO.21.00276
11. Wakelee H, Liberman M, Kato T, Tsuboi M, Lee S-H, Gao S, et al. Perioperative pembrolizumab for early-stage non–small-cell lung cancer. N Engl J Med. (2023) 389:491–503. doi: 10.1056/NEJMoa2302983
12. Yan W, Zhong WZ, Liu YH, Chen Q, Xing W, Zhang Q, et al. Adebrelimab (Shr-1316) in combination with chemotherapy as perioperative treatment in patients with resectable stage II to III nsclcs: an open-label, multicenter, phase 1b trial. J Thorac Oncol. (2022) 18:194–203. doi: 10.1016/j.jtho.2022.09.222
13. Zhao Z, Chen S, Qi H, Yang CP, Lin YB, Jin JT, et al. Phase ii trial of toripalimab plus chemotherapy as neoadjuvant treatment in resectable stage iii non-small cell lung cancer (Neotpd01 study). J Clin Oncol. (2021) 39:8541. doi: 10.1080/2162402X.2021.1996000
14. Zhao ZR, Yang CP, Chen S, Yu H, Lin YB, Lin YB, et al. Phase 2 trial of neoadjuvant toripalimab with chemotherapy for resectable stage III non-small-cell lung cancer. Oncoimmunology. (2021) 10:1996000. doi: 10.1080/2162402X.2021.1996000
15. Huang Z, Chen L, Lv L, Fu CC, Jin Y, Zheng Q, et al. A new ai-assisted scoring system for pd-L1 expression in nsclc. Comput Methods Programs BioMed. (2022) 221:106829. doi: 10.1016/j.cmpb.2022.106829
16. Pan Y, Sheng W, Shi L, Jing D, Jiang W, Chen JC, et al. Whole slide imaging-based deep learning to predict the treatment response of patients with non-small cell lung cancer. Quant Imaging Med Surg. (2023) 13:3547–55. doi: 10.21037/qims-22-1098
17. Park J, Cho HG, Park J, Lee G, Kim HS, Paeng K, et al. Artificial intelligence-powered hematoxylin and eosin analyzer reveals distinct immunologic and mutational profiles among immune phenotypes in non-small-cell lung cancer. Am J Pathol. (2022) 192:701–11. doi: 10.1016/j.ajpath.2022.01.006
18. Park S, Ock C-Y, Kim H, Pereira S, Park S, Ma M, et al. Artificial intelligence–powered spatial analysis of tumor-infiltrating lymphocytes as complementary biomarker for immune checkpoint inhibition in non–small-cell lung cancer. J Clin Oncol. (2022) 40:1916–28. doi: 10.1200/jco.21.02010
19. Prelaj A, Miskovic V, Zanitti M, Trovo F, Genova C, Viscardi G, et al. Artificial intelligence for predictive biomarker discovery in immuno-oncology: A systematic review. Ann Oncol. (2024) 35:29–65. doi: 10.1016/j.annonc.2023.10.125
20. Wang S, Yu H, Gan Y, Wu Z, Li E, Li X, et al. Mining whole-lung information by artificial intelligence for predicting egfr genotype and targeted therapy response in lung cancer: A multicohort study. Lancet Digit Health. (2022) 4:e309–e19. doi: 10.1016/S2589-7500(22)00024-3
21. Wu J, Liu C, Liu X, Sun W, Li L, Gao N, et al. Artificial intelligence-assisted system for precision diagnosis of pd-L1 expression in non-small cell lung cancer. Mod Pathol. (2022) 35:403–11. doi: 10.1038/s41379-021-00904-9
22. Zhao L, Xu X, Hou R, Zhao W, Zhong H, Teng H, et al. Lung cancer subtype classification using histopathological images based on weakly supervised multi-instance learning. Phys Med Biol. (2021) 66(23):10.1088/1361-6560/ac3b32. doi: 10.1088/1361-6560/ac3b32
23. Fu Y, Jung AW, Torne RV, Gonzalez S, Vohringer H, Shmatko A, et al. Pan-cancer computational histopathology reveals mutations, tumor composition and prognosis. Nat Cancer. (2020) 1:800–10. doi: 10.1038/s43018-020-0085-8
24. Kather JN, Heij LR, Grabsch HI, Loeffler C, Echle A, Muti HS, et al. Pan-cancer image-based detection of clinically actionable genetic alterations. Nat Cancer. (2020) 1:789–99. doi: 10.1038/s43018-020-0087-6
25. Muti HS, Heij LR, Keller G, Kohlruss M, Langer R, Dislich B, et al. Development and validation of deep learning classifiers to detect epstein-barr virus and microsatellite instability status in gastric cancer: A retrospective multicenter cohort study. Lancet Digit Health. (2021) 3:e654–e64. doi: 10.1016/S2589-7500(21)00133-3
26. Coudray N, Ocampo PS, Sakellaropoulos T, Narula N, Snuderl M, Fenyo D, et al. Classification and mutation prediction from non-small cell lung cancer histopathology images using deep learning. Nat Med. (2018) 24:1559–67. doi: 10.1038/s41591-018-0177-5
27. Dacic S, Travis W, Redman M, Saqi A, Cooper WA, Borczuk A, et al. International association for the study of lung cancer study of reproducibility in assessment of pathologic response in resected lung cancers after neoadjuvant therapy. J Thorac Oncol. (2023) 18:1290–302. doi: 10.1016/j.jtho.2023.07.017
28. Weissferdt A, Pataer A, Vaporciyan AA, Correa AM, Sepesi B, Moran CA, et al. Agreement on major pathological response in nsclc patients receiving neoadjuvant chemotherapy. Clin Lung Cancer. (2020) 21:341–8. doi: 10.1016/j.cllc.2019.11.003
29. Liu Y, Xiong L, Chen Y, Cai R, Xu X, Wang T, et al. Complete pathological remission and tertiary lymphoid structures are associated with the efficacy of resectable nsclc receiving neoadjuvant chemoimmunotherapy: A double-center retrospective study. Hum Vaccin Immunother. (2023) 19:2285902. doi: 10.1080/21645515.2023.2285902
30. Zhang B, Xiao H, Pu X, Zhou C, Yang D, Li X, et al. A real-world comparison between neoadjuvant chemoimmunotherapy and chemotherapy alone for resectable non-small cell lung cancer. Cancer Med. (2023) 12:274–86. doi: 10.1002/cam4.4889
31. Campanella G, Hanna MG, Geneslaw L, Miraflor A, Werneck Krauss Silva V, Busam KJ, et al. Clinical-grade computational pathology using weakly supervised deep learning on whole slide images. Nat Med. (2019) 25:1301–9. doi: 10.1038/s41591-019-0508-1
32. Qu H, Wu P, Huang Q, Yi J, Yan Z, Li K, et al. Weakly supervised deep nuclei segmentation using partial points annotation in histopathology images. IEEE Trans Med Imaging. (2020) 39:3655–66. doi: 10.1109/TMI.2020.3002244
33. Lu MY, Williamson DFK, Chen TY, Chen RJ, Barbieri M, Mahmood F. Data-efficient and weakly supervised computational pathology on whole-slide images. Nat BioMed Eng. (2021) 5:555–70. doi: 10.1038/s41551-020-00682-w
34. Gadermayr M, Tschuchnig M. Multiple instance learning for digital pathology: A review of the state-of-the-art, limitations & Future potential. Comput Med Imaging Graph. (2024) 112:102337. doi: 10.1016/j.compmedimag.2024.102337
35. Zhou Y, Che S, Lu F, Liu S, Yan Z, Wei J, et al. Iterative multiple instance learning for weakly annotated whole slide image classification. Phys Med Biol. (2023) 68(23):10.1088/1361-6560/ac3b32. doi: 10.1088/1361-6560/acde3f
36. Dov D, Kovalsky SZ, Assaad S, Cohen J, Range DE, Pendse AA, et al. Weakly supervised instance learning for thyroid Malignancy prediction from whole slide cytopathology images. Med Image Anal. (2021) 67:101814. doi: 10.1016/j.media.2020.101814
37. Selvaraju RR, Das A, Vedantam R, Cogswell M, Parikh D, Batra D. Grad-cam: why did you say that? arXiv preprint arXiv:161107450. (2016).
38. Zhang H, Ogasawara K. Grad-cam-based explainable artificial intelligence related to medical text processing. Bioengineering (Basel). (2023) 10:1070. doi: 10.3390/bioengineering10091070
Keywords: non-small cell lung cancer, major pathological response, neoadjuvant chemoimmunotherapy, whole slide image, weakly supervised learning
Citation: Han D, Li H, Zheng X, Fu S, Wei R, Zhao Q, Liu C, Wang Z, Huang W and Hao S (2024) Whole slide image-based weakly supervised deep learning for predicting major pathological response in non-small cell lung cancer following neoadjuvant chemoimmunotherapy: a multicenter, retrospective, cohort study. Front. Immunol. 15:1453232. doi: 10.3389/fimmu.2024.1453232
Received: 22 June 2024; Accepted: 27 August 2024;
Published: 20 September 2024.
Edited by:
Takaji Matsutani, Maruho, JapanReviewed by:
Yong Ren, Sun Yat-sen University, ChinaCopyright © 2024 Han, Li, Zheng, Fu, Wei, Zhao, Liu, Wang, Huang and Hao. This is an open-access article distributed under the terms of the Creative Commons Attribution License (CC BY). The use, distribution or reproduction in other forums is permitted, provided the original author(s) and the copyright owner(s) are credited and that the original publication in this journal is cited, in accordance with accepted academic practice. No use, distribution or reproduction is permitted which does not comply with these terms.
*Correspondence: Wei Huang, YWx2aW5iaXJkQDEyNi5jb20=; Shaoyu Hao, aHNoYW95dTE5ODVAMTI2LmNvbQ==
†These authors have contributed equally to this work and share first authorship
Disclaimer: All claims expressed in this article are solely those of the authors and do not necessarily represent those of their affiliated organizations, or those of the publisher, the editors and the reviewers. Any product that may be evaluated in this article or claim that may be made by its manufacturer is not guaranteed or endorsed by the publisher.
Research integrity at Frontiers
Learn more about the work of our research integrity team to safeguard the quality of each article we publish.