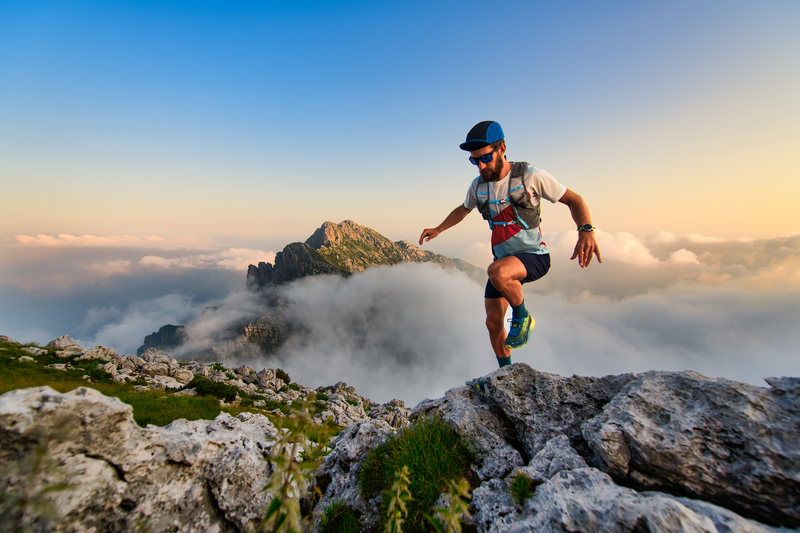
94% of researchers rate our articles as excellent or good
Learn more about the work of our research integrity team to safeguard the quality of each article we publish.
Find out more
REVIEW article
Front. Immunol. , 21 August 2024
Sec. Cancer Immunity and Immunotherapy
Volume 15 - 2024 | https://doi.org/10.3389/fimmu.2024.1351584
This article is part of the Research Topic Community Series in Biomarkers in the Era of Cancer Immunotherapy: Zooming in from Periphery to Tumor Microenvironment, Volume II View all 14 articles
Over the last decade, a new paradigm for cancer therapies has emerged which leverages the immune system to act against the tumor. The novel mechanism of action of these immunotherapies has also introduced new challenges to drug development. Biomarkers play a key role in several areas of early clinical development of immunotherapies including the demonstration of mechanism of action, dose finding and dose optimization, mitigation and prevention of adverse reactions, and patient enrichment and indication prioritization. We discuss statistical principles and methods for establishing the prognostic, predictive aspect of a (set of) biomarker and for linking the change in biomarkers to clinical efficacy in the context of early development studies. The methods discussed are meant to avoid bias and produce robust and reproducible conclusions. This review is targeted to drug developers and data scientists interested in the strategic usage and analysis of biomarkers in the context of immunotherapies.
Targeted therapies and cancer immuno-therapies (CIT), including immune checkpoint inhibitors (CPI) have revolutionized the treatment paradigm for a number of cancers, leading to improvements in progression-free and overall survival, and more durable responses [reviewed in: Shahid et al. (1); Esfahani et al. (2); Robert (3); Murciano-Goroff et al. (4)]. Despite these successes, many patients do not or only transiently benefit from such treatments and additional efforts are required to truly unleash the full potential of precision and personalized medicine. Biomarkers play an integral role in this process and can guide and impact clinical research and development and, ultimately, actual patient care [reviewed in: (5–8)].
With emerging and evolving technologies, our understanding of cancer biology and tumor immunology is growing, which also is helping the discovery and development of novel biomarkers. Biomarkers may already play an important role by providing answers to key questions in the following areas of early clinical development of immunotherapies (please also refer to Table 1 in the supplementary section for examples): 1) Demonstration of mechanism of action (MoA) by analyzing the pharmacodynamic effect. For CIT, characterization of drug effects and efficacy may differ from classical therapies focused on direct tumor cell killing and tumor shrinkage. 2) Dose finding and dose optimization. 3) Mitigation and prevention of adverse reaction to the drug. In particular, new challenges arise from the need to improve tolerability of immunotherapies, and to predict and mitigate adverse immune reactions. 4) Patient enrichment and indication prioritization, typically based on baseline characteristics of the respective tumor such as target expression. Considerations regarding the variety of immune targets leveraged by the new immune therapies, as well as emergence of resistance mechanisms are key to the success of these therapies.
A number of research studies have highlighted challenges and a lack of reproducibility in several areas of biomarker research (10, 11). These challenges are accentuated in the context of immunotherapies due to the complexity of the immune system and the variety of biomarkers studied. A comprehensive review of the statistical principles for biomarkers in CIT is lacking. In this review, we describe these statistical principles focusing to the application of biomarkers in early clinical development studies of CIT. We believe that adherence to these principles improves the quality of biomarker studies and the generalizability and robustness of their findings
The remainder of this manuscript is structured as follows: In Section 2, we review biomarker definitions, examples, and technologies, and typical clinical endpoints. We also connect the previously mentioned four areas of early CIT drug development listed above with the statistical analyses reviewed in the next section. Section 3 describes appropriate statistical methods for biomarkers, starting with the choice of data transformation and probabilistic models (Section 3.1), and relevant biomarker considerations for dose selection and optimization (Section 3.2). Sections 3.3 and 3.4 discuss the assessment of prognostic and predictive characteristics of baseline biomarkers. Section 3.5 covers analysis methods for on-treatment biomarkers including landmarking and joint modeling. Challenges related to high-dimensional biomarker analyses are described in Section 3.6. Finally, Section 3.7 provides examples of the use of PK/PD models in relation to biomarkers. We conclude the manuscript with a brief discussion.
The complexity of the human immune system is reflected in the plethora of biomarkers that have been developed to capture the activation of the immune system against cancer cells, and the interaction between immunotherapies and the immune system. Supplementary Table 1 in the supplemental material provides examples of biomarkers which have been proposed in the scientific literature. This diversity strengthens the need for a rigorous and robust analysis of the effect of biomarkers.
Biomarkers may serve four different purposes as summarized in Table 1 and further described below (9, 12).
First, prognostic baseline biomarkers differentiate patients with regards to the outcome of the disease independently of the treatment. A typical example of a prognostic biomarker is the total CD8 count in the tumor. Patients with a high number of CD8+ cells, which identifies the T-cell, have immune response against the tumor and better prognosis (13).
Second, predictive biomarkers at baseline are markers that differentiate patients that benefit most from a treatment. Hence, predictive biomarkers are specific to a (class of) treatments and, sometimes, specific to a tumor type. PD-L1 expression is a predictive biomarker for CPI for some tumor types and disease stages though it has limitations (14).
In clinical trials, prognostic and predictive biomarkers are utilized for the enrichment of the study population to obtain a more homogeneous population. For example, prognostic biomarkers may be used to select only subjects with a poor prognosis who are in most urgent need of better treatment options into the study. Moreover, the power of a trial with a time-to-event endpoint (such as progression- free survival) to detect a targeted hazard ratio depends on the observed number of events and not on the number of recruited subjects (15, chapter 15). In a population with a worse prognosis, these events are observed more quickly allowing for trials with a lower sample size and/or a shorter trial duration. Predictive biomarkers are more specifically utilized to investigate restrictions on the patient population (in the drug label). In a first phase of development, early studies may enroll all-comers. The emerging understanding of cancer biology and the mechanism of action of the drug, supported by data analysis of these early studies may allow definition of a biomarker-positive subgroup with an enhanced treatment effect. In a second phase of development, subsequent trials may restrict the population to patients who benefit most from the drug, based on the identified biomarker or continue with two primary populations: all-comers and the biomarker-positive populations (see section 3.4 for an example), in both cases the biomarker status is determined by a diagnostic test. Moreover, prognostic and predictive biomarkers are also used in randomized clinical trials as stratification factors (to ensure balance between groups) and for covariate adjustment purposes (to increase power). Finally, adjustment for such biomarkers is important to render non-randomized groups more comparable (e.g. through propensity score methods, see Austin (16) and Brookhart et al. (17), or regression adjustment).
Third, on-study pharmacodynamic biomarkers capture the effect of the drug after its administration. The goal of these biomarkers is to demonstrate that the drug has the anticipated biological effect (i.e. establishes a proof of mechanism (PoM)). These biomarkers are usually linked to the MoA (e.g. the activation of natural killer cells, CD8 T cells during the treatment with Il15 (18)), but can be independent of it. A goal of analyses involving pharmacodynamic biomarkers is to relate the measured biological effect indicated by the biomarker itself to the observed clinical efficacy. In the optimal case, these biomarkers help in understanding the dose-response relationship and may be used as surrogates for clinical efficacy endpoints (formal surrogacy needs to be established with extensive subject level-data and specific methods (see Burzykowski et al. (19)).
Fourth, safety biomarkers are on-study biomarkers intended to measure the likelihood, presence, or extent of toxicity (e.g. IL6 serum levels in the context of cytokine release syndrome (CRS), see Pabst et al. (20) and Section 3.2). Detection of or change in a biomarker can allow dose modification or treatment interruption before toxicity becomes severe. These markers may also serve to characterize the dose-safety relationship.
Along with these biomarkers, the efficacy benefits that patients derive from a drug is measured through classical clinical endpoints. In early phase trials and for classical cytotoxic drugs for solid tumors, the benefit is typically measured in terms of tumor shrinkage induced by the drug. The tumor shrinkage has been classified into a response criteria by Therasse et al. (21). Even though long term benefit, typically measured by the time to death (or overall survival, abbreviated as OS), is not always associated to responses (RECIST criteria defined by Therasse et al. (21)), the goal of drugs other than immunotherapies remains to shrink the tumors. For drugs with an indirect effect on the tumors, like immunotherapies, tumor shrinkage may no longer be representative of the MoA and of long term clinical benefit (22). Therefore, endpoints like progression-free survival (PFS), which can capture the absence or a slow regrowth, and especially OS are more relevant to represent the benefit of immunotherapies. Also tumor growth kinetic models, based on the total tumor size, can provide additional insights in the clinical benefit of immunotherapies (23). The analysis of biomarkers and the link to these endpoints introduce additional complexities (reviewed in Section 3.5).
To illustrate the structure of the manuscript, Table 2 connects the biomarker objectives described above with the statistical models and consideration that we cover below.
This section provides an overview of relevant statistical considerations and models. Many of the described methods and statistical principles have not been developed specifically for CIT biomarkers but we believe that adherence to good statistical practice is particularly important in this context because of the complexity of questions related to the immune system and the variety of new biomarkers analyzed. We would also like to stress that prior to any statistical analysis, a statistical analysis plan should be written which describes the precise scientific questions and all planned analyses. While exploratory analysis also play an important role, they should still be guided by a predefined analysis strategy to avoid data dredging.
An important step prior to any statistical analysis is to have a thorough understanding of how the biomarker data were obtained. Additional data normalization might be required depending on the steps already performed during the data acquisition process. The awareness of these details ultimately guides the various statistical modeling considerations, e.g. the choice of distribution, the need for covariate adjustment, or the treatment of missing values. Let’s illustrate this with an example. In the field of immuno-oncology a technology called flow cytometry plays a key role in measuring immune cell markers. An important step in the data acquisition is called “manual gating” which is typically employed to classify single cells into discrete cell types based on emitted fluorescent signals. As a consequence, the readout for flow cytometry data are proportions, i.e. number of cells relative to some reference cell population. The statistical challenge is then to choose a model with adequate distributional assumptions. For example linear regression relating the dose of a drug with the change in the proportion of activated T cells (adjusted by baseline) assumes that the residual errors are normally, independently and identically distributed with mean zero. Oftentimes biomarker data do not conform with these standard statistical assumptions (e.g. flow cytometry data). Statistics offers three possible solutions to deal with this challenge: the first approach is to transform the original values using a deterministic function such that the standard statistical assumptions are approximately met. The logarithmic and square root functions are popular examples of data transformations. The Box-Cox transformation family (24) is often used to approach data transformation more systematically using statistical estimation techniques. A transformation particularly useful in the example outlined above is the empirical logit transformation which maps proportions monotonically to the whole real line while keeping the interpretation of regression coefficients simple (25). The second approach extends linear regression by allowing the linear model to be related to the response variable via a link function and by allowing the magnitude of the variance of each measurement to be a function of its predicted value (26). In the case of the flow cytometry example, a logit-link function and binomial mean-variance relationship is well suited; overdispersion is dealt with either by including a random effect to account for the between sample variation or by adding a dispersion parameter to the mean-variance relationship (the latter approach is referred to as the quasi-binomial approach). The third approach consists in avoiding any distributional assumption and resort to non-parametric methods. In our view, in the context of exploratory biomarker analysis, the data transformation approach has two key advantages: firstly, statistical inference based on standard linear regression is robust and goes hand-in-hand with familiar visualizations (e.g. boxplot and scatter plot), which in-turn facilitates communication of results to non-statisticians; secondly, transformed data can be readily used as input to a variety of advanced statistical methods and data mining tools.
Two additional complications of biomarker analyses are: First, measurements below the assay’s detection limit may occur. Such measurements should not be excluded from the analysis. If their frequency is low, ad-hoc approaches such as imputing them with, say, half of the detection limit may be sufficient but if they are frequent, more sophisticated methods such as treating them as left-censored may be preferable (27). Second, the assay may be impacted by measurement errors. In this case, models, such as those described in the next sections, need to account for the additional variability potentially bias induced by the measurement error. To elicit the components (e.g. distribution assumptions) needed for a proper handling of measurement error, one needs to have data, either within the dataset considered in the analysis (internal), or outside (external, e.g. a assay development dataset or preclinical experiments) in which the true value can be linked to the assay. Several models can then be implemented (see Carroll et al. (28) for a general reference).
The introduction of new mechanisms of action has led to new safety risks and challenges in the determination of the optimal dose to be administered. In this context, biomarkers are used to provide a more granular observation of the drug’s anti-tumor activity or safety and allow the determination of the dose based on a more precise benefit risk assessment (compared to clinical endpoints). In this section, we highlight some challenges of dose finding for immunotherapies and provide some options to optimize dose finding using biomarkers.
A typical issue with some classes of immunotherapies (T-cell engagers) is the cytokine release syndrome (CRS), a trigger of a systemic inflammatory response characterized by a large amount of cytokines being released. CRS limits the exploration of high and effective doses. A mitigation strategy was found by which one or more low priming doses (also called step-up) attenuate the cytokine release upon repeated doses and allow the subsequent administration of a high and effective target dose (Bartlett et al. (29)). The dose finding exercise then requires the determination of the priming doses and the target dose, as opposed to, for classical drugs, the determination of the tolerable target dose. Dose escalation designs have been proposed in this context by Xu et al. (30); Gerard et al. (31) and more recently by Dejardin et al. (32).
Other dose finding challenges are summarized by Wages et al. (33). Challenges include late onset toxicities, i.e. toxicities occurring beyond the typical observation period (set to 21-28 days after dosing). In the presence of late toxicities, one could prolong the observation period for each patient. However, this causes the dose escalation trial to be significantly prolonged in some cases. Wages et al. (33) reports dose escalation designs to allow partial observation of patients in the dose escalation decisions. Another challenge is the fact that higher doses may not be the best in terms of efficacy. Indeed, some drugs may have an optimal biological activity at intermediate dose ranges (“bell-shaped-response”) with suboptimal activity at higher doses, for which however toxicity may be observed. Dose escalation designs must therefore be constructed such that both safety and efficacy are optimized. Wages et al. (33) reports options to use both safety and efficacy in the determination of the next dose in a dose escalation design. These approaches work conditionally on the fact that the efficacy endpoint is available at the time of the dose escalation decision.
When optimizing on efficacy, a key question is the identification of the most appropriate function that describes dose-response relationship. A structured procedure to study the relationship with dose has been proposed by Bretz et al. (34); Nie et al. (35). In this procedure, a set of coefficients from candidate models are calculated, allowing different dose response shapes. Then, a multiple test procedure is implemented to select the dose response signals, followed by a model selection step. This procedure allows flexible modeling of the dose response signal, preserving the robustness to misspecification that are associated with multiple testing. A simpler approach consists in using a general model that can accommodate many different dose-response shapes. The EMACS model (see Seber and Wild (36) Section 7) is an example of such models.
The prognostic association of one or multiple baseline biomarkers with clinical outcomes is typically assessed using regression models, such as the logistic model for binary outcomes (e.g. the overall response rate) or the Cox proportional hazards model for time-to-event outcomes (e.g. PFS or OS). As described in Section 3.1 it is important that adequate transformations are applied to biomarkers before including them as covariates. If there’s uncertainty regarding the shape of the association between a biomarker and the outcome, spline models can easily be incorporated into regression models (Harrell (37)). In order to reduce the complexity of a multivariable regression model, naive step-wise variable selection methods are frequently used but have a number of issues including that they yield over-optimistic regression coefficients and p-values. More modern selection procedures such as the lasso, boosting, or Bayesian model averaging are recommended (Harrell (37)). To aid clinical decision making, medical practitioners are used to discretizing continuous biomarker into different risk categories by applying cut-points. A number of statistical pitfalls are associated with this practice. First, discretization introduces a substantial loss of information. Second, data-driven cut-point selection algorithms may lead to overfitting and an exaggerated association between the dichotomized biomarker and the clinical outcome. For a review of these pitfalls and guidance on the selection of cutpoints, we refer to Polley and Dignam (38) and (39, Chapter 16).
A dataset of sufficient size and quality is essential in order to develop a robust prognostic model (Riley et al. (40)). Sample sizes of early development studies are typically insufficient for this purpose and data from large cohort studies or confirmatory trials are required. Moreover, for most cancer types, a plethora of published prognostic models exist already. As an example, a systematic review of prognostic models in breast cancer identified 58 published models but only a few of them had been validated widely in different settings and, frequently, their performance was suboptimal in independent populations (Phung et al. (41)). For this reason, it may be scientifically more relevant to externally validate or update existing prognostic models in new contexts or to assess whether the addition of a new biomarker improves an already existing prognostic model (Steyerberg (39)).
We refer to Harrell (37); Steyerberg (39), and Collins et al. (42) for comprehensive resources to developing and reporting prognostic models and only highlight a few points relevant to performance assessment and validation below. First, it is important to characterize the performance and clinical utility of a prognostic model. Performance measures should report the overall performance of a model, its discrimination, i.e. its ability to discriminate subjects with an event from those without an event, as well as its calibration, i.e. the agreement between observed outcomes and predictions. Frequently reported measures for these three performance aspects are the Brier score, the concordance probability or c-index, and calibration plots. For a detailed review of performance measures, we refer to (39, Chapter 15).
A prognostic model is internally valid (or “reproducible”) if its reported performance adequately reflects the actual performance of the model that would be observed in an independent random data sample from the same source population as the dataset used for model development. One reason why a prognostic model may not be reproducible is that performance is overestimated due to overfitting. A common reason for this is that model development and performance assessment are performed on the same dataset. If one builds a complex prognostic model and then evaluates its performance in the same dataset, then one may obtain a very good accuracy on that dataset, but that is not a fair assessment of the future performance of the model. Rather, the appropriate question is whether this prognostic model will provide a sufficient level of accuracy to be of use when applied to a truly independent test set. In internal validation studies where only one dataset is used for model development and validation, there are two main options to mimic performance in an independent dataset. The first option is to reserve a fixed portion of the dataset (e.g. 20%) for validation. That is, model development is based on training data, i.e. the data not included in the validation dataset, and a hold-out validation dataset is strictly shielded from access during model development. Once model development is completed, its performance is evaluated in the independent hold-out dataset. Since this approach discards a part of the dataset for training, it has some drawbacks (see Section 17.2.2.2 in Steyerberg (39)), one of which is the stability of the findings. Therefore, a second option is to implement internal validation using re-sampling approaches such as cross-validation or bootstrapping. These approaches include repeatedly splitting the data into a training and a validation data set, developing the model including parameter estimation in the training data set, and then assessing performance in the validation dataset. In general, cross-validation and bootstrapping are more efficient approaches to assess average model performance than a single random data split (see Steyerberg (43) and the discussion about prediction stability and error in boostrap sample in Riley and Collins (44).
The above steps outline the discovery and development stages of prognostic markers which includes the identification of prognostic biomarkers or the building of a prognostic model, and their performance assessment in internal validation studies. The full development of such biomarkers or models for clinical use is typically split into three phases (Moons et al. (45); Ou et al. (46)): discovery or development studies, external validation studies, and clinical impact studies. Analytical validation of the biomarker is another important phase that is outside of the scope for this article (Ou et al. (46); Kraus (47)). The purpose of external validation studies is to confirm the performance of the biomarker/prognostic model in a completely independent dataset and to assess its generalizability to a wider population. Finally, impact studies assess whether the use of the biomarkers/prognostic model by practicing doctors actually improves clinical outcomes. Ideally, impact studies are randomized controlled trials which compare biomarker-guided treatment with the standard of care.
The exploratory assessment whether a baseline biomarker is predictive, i.e. whether it impacts the magnitude or direction of a treatment effect, typically requires data from randomized controlled clinical trials. Predictive biomarker effects can be modeled by including a treatment × biomarker interaction term in the regression model. Of note, logistic and Cox regression model the relative reductions in the odds or rate of an event. If a biomarker is prognostic, then the absolute risk reduction may vary across biomarker levels even if the relative risk reduction is the same. Standard analyses to assess whether a biomarker is predictive are statistical tests for the treatment × biomarker interaction term as well as forest plots which visualize treatment effects in subgroups defined by the biomarker (Alosh et al. (48)). For a tutorial on data-driven identification of predictive subgroups we refer to Lipkovich et al. (49).
Limitations of exploring several biomarkers and clinical variables for predictive effects are well-recognized: an increased risk of false-positive findings due to multiplicity combined with an increased risk of false-negative findings and highly variable treatment effect estimates in subgroups due to the limited sample size and power in subgroups. Therefore, findings from such analyses should only be considered credible if additional criteria are fulfilled, e.g. that the predictive effect of the biomarker was correctly pre-specified (including the direction of the effect), that the predictive effect is supported by prior evidence, that only a low number of potential predictive markers was explored (ideally 3 or fewer), that chance cannot explain the finding, and that arbitrary cut points were avoided (Schandelmaier et al. (50)). Otherwise, the findings should be considered exploratory and hypothesis-generating only.
For the confirmatory assessment of a predictive biomarker and its inclusion in a drug label (e.g. the restriction of a label to a biomarker-positive subgroup or a claim of enhanced efficacy in this subgroup), a randomized clinical trial with type I error control across the all-comers population and the biomarker-positive subgroups is typically required. As an example, the IMpassion130 trial, a randomized phase 3 trial of the CPI atezolizumab in advanced triple-negative breast cancer, hierarchically tested the clinical endpoints first in the all-comers (intention-to-treat) population and then in the PD-L1–positive subgroup (Schmid et al. (51)). As another example, the IMpassion031 trial of the same molecule in early-stage triple-negative breast cancer was an adaptive enrichment trial with two primary populations (PD-L1 positives and all-comers) allowing for population section at an interim analysis (Nguyen Duc et al. (52); Mittendorf et al. (53)).
In this section, we discuss models which analyze the impact of one or a low number of biomarkers measured on-treatment, i.e. pharmacodynamic biomarkers, on clinical outcome. A key aspect of on-study biomarkers is the timing at which measures are taken. For some biomarkers, repeated measurements are available (e.g. parameters taken in the blood) while tumor parameters (requiring invasive techniques or tumor imaging data, for which the amount of radiation received by the patient is a limiting factor) are usually taken only once after treatment start. The timing aspects need to be explicitly accounted for in the analyses, which, in our experience, is often not done.
Before discussing appropriate analysis methods for on-treatment biomarkers, we list five additional challenges which are important to consider while planning the analyses and writing a statistical analysis plan. First, it is critical to avoid immortal-time bias in the analysis (Mantel and Byar (54)). This occurs if a clinical endpoint that is timed from study enrolment is compared across groups defined by a classifying event occurring during follow-up e.g. by an on-treatment biomarker. A classical example of this bias in oncology is the naive comparison of overall survival by tumor response categories (Anderson et al. (55)). More generally, any analysis which treats an on-treatment biomarker as if it was a baseline biomarker or which reverses time, i.e. tries to explain the past with the future, should be avoided.
Second, when studying the relationship between the risk of a clinical event (response or progression) and the longitudinal evolution of the biomarker, models need to capture the impact of changes in the biomarker on the risk of the event. This implies that the biomarker and the clinical event need to be modeled jointly across time. An example of this type of analysis is the construction of a dynamic prognostic score for the risk of recurrence of prostate cancer depending on longitudinal assessments of a prostate-specific antigen (PSA) biomarker (56).
Third, considerations regarding which aspects of the longitudinal biomarker dynamics affect the clinical endpoint are important. In the previous example, the current value of the PSA marker may directly affect the rate of the clinical event. In other settings, there might be a lag-effect, i.e. the risk of event at time t is influenced by the biomarker at time t − ℓ for a fixed or variable lag time ℓ. Alternatively, the impact of the rate of change or the accumulation of a biomarker is of primary interest. In the latter setting, the biomarker’s area under the (time-)curve (AUC) may be an alternative as the covariate of primary interest.
Fourth, competing events such as adverse events requiring treatment interruptions, may occur which affect either the interpretation or the existence of subsequent measurements of the biomarker and the clinical event. It is typically not plausible that the occurrence of such adverse events is independent of on-treatment biomarkers and clinical endpoint. For example, the biological activity of the drug may induce a change in the biomarker and in the clinical endpoint, but at the same time lead to toxicity. In such cases, the joint evaluation of the biomarker and the clinical endpoint is impacted by the competing risk of an interruption of treatment due to the toxicity which needs to be accounted for in the analysis.
Fifth, the biological variation of a biomarker may vary substantially by subject or by dose. Typically the protocol defined sampling schedule is common to all patients and dose levels. As a consequence, the biomarker measurements may not be taken at the same time relative to the biological process unfolding in each patient. An example of a biomarker where this issue arises are natural killer (NK) cells measured in blood: these immune cells go through a marginalization process at which time they become non observable in the blood (see Conlon et al. (18)).
These considerations may be addressed using the analysis methods presented below.
For biomarkers measured infrequently (e.g. via biopsies), landmark analyses may be used (Van Houwelingen (57)). In such analyses, a landmark time is defined as the time of a specific biomarker measurement which defines a new baseline (time zero) for subsequent analysis. That is, the value of the biomarker at the landmark is treated as a baseline covariate, subsequent biomarker measurements are ignored, the clinical time-to-event endpoint is re-defined based on the new time origin, and all subjects with a clinical event or censoring prior to the landmark are excluded from the analysis. Subsequently, standard regression methods for modeling the clinical endpoints such as Cox regression are used. Landmark analysis avoid immortal-time bias but care needs to be taken when comparing randomized treatment groups because they are no longer comparable after conditioning on the landmark.
In order to model longitudinal biomarkers and clinical endpoints simultaneously, joint models are popular (Rizopoulos (58)). These models have two components, a longitudinal model for the biomarker (typically a linear mixed effects model) and a time-to-event model for the clinical outcome (e.g. a Cox model), and shared parameters which determine how the biomarker evolution affects the rate of clinical event. Such models allow that the actual (measurement-error free) value of the biomarker, or a lagged value, or a rate of change, or the area under the curve is included as a covariate for modeling the clinical event. Alternatively, when relevant thresholds are defined for the biomarker values, the subject’s evolution through different states of biomarker categories and clinical outcomes can be modeled using multi-state models (59). Multi-state models are also useful to address the competing risk problem described above.
In the context of randomized trials, relevant clinical questions may arise regarding the treatment effect in subpopulations of patients, which could experience certain clinical or disease related events post-randomization. As an example, subjects in the CIT group may experience anti-drug antibodies and it is of interest to know whether this affects CIT efficacy. A principal stratification strategy can be applied to these events to define a causal treatment effect (Bornkamp et al. (60)). Specifically, Kong et al. (61) describe an approach where subjects with an ADA in the CIT arm are compared to the control arm which is re-weighted based on relevant baseline characteristics to be rendered more comparable to the ADA-positive CIT subpopulation. These weighting approaches for principal stratification are similar to inverse probability of treatment weighting (IPTW) approaches which are frequently used to address causal questions, e.g. to determine the treatment effect in subgroups defined by baseline biomarkers in the presence of additional confounding variables [see Austin and Stuart (62)].
To address the confounding effect linked to biological variability of the biomarker, an option is to leverage the (semi) mechanistic models described in Section 3.7. These models provide a more complete description of the longitudinal profile, that may be patient- and covariate- dependent (e.g. to dose) compared to measurements at a fixed time for all patients. Using these profile, quantities such as the maximum value over a period of time, or the AUC are more robustly estimated. A two-stage approach combining the mechanistic model and models such as the landmark or the joint models can be used to study in a more robust manner the relationship between the clinical endpoint and the biomarker.
In addition to these specific models, one may need to take potential imbalances in value of the biomarker at baseline into account. These imbalances may lead to false conclusions on the impact of the treatment on the on-study biomarker value. Authors [see Vickers (63), (64, Section 2.4)] suggest to incorporate the baseline value in models of the on-study value. This conclusion is also true when baseline values are partially missing [see Kenward et al. (65)].
Besides testing key biomarkers hypothesis (as usually specified in the study protocol) early clinical development many times involves extensive exploratory data analysis work. The aim of these analysis efforts is to generate new hypotheses related to MoA, PoC (proof of concept) and patient selection/enrichment. Omics-based technologies typically measure thousands of molecular features (e.g. genomics, transcriptomics and proteomics) in parallel. An important characteristics of such high-dimensional data is that the number of features p is typically much larger compared to the number of samples n (e.g. number of patients). Such data sets present a variety of statistical challenges, since classical theory and methodology can break down in surprising and unexpected ways Buhlmann¨ and Van De Geer (66), Wainwright (67), Hastie et al. (68). Hypothesis generation based on high-dimensional biomarker data can be typically divided into three types of analysis tasks:
The first task considers p biomarkers and one response variable and the aim is prediction and variable selection. Such analysis can be the starting point of the development of a novel prognostic or predictive biomarker which then could potentially be used for patient selection/enrichment (see section 3.3 on the prognostic/predictive validation of biomarker). Numerous computational approaches have been developed for this purpose which adequately address overfitting and simultaneously provide information on variable importance. These methods use modern statistical concept such as such as L1/L2-regularization (e.g. Ridge-, Lasso Regression), bootstrap aggregation (Random forest) and boosting. A recent example is the construction of a polygenic risk scores (PRS) for anti-PD-L1 induced hypothyroidism and the subsequent assessment of PRS variant importance using Lasso Regression [Khan et al. (69)].
The second task considers one (or few) potential explanatory variable whereas the p biomarkers take the role of response variables. The aim is to identify those biomarkers which show a relationship with the explanatory variable. An example is the exploration of dose relationship with molecular PD markers (e.g. as measured by flow cytometry or gene expression). This involves simultaneous testing of many hypothesis. It is essential to perform multiple testing correction in order to correct for occurrence of false positives [Dudoit et al. (70)]. In addition, empirical Bayes approaches which borrow information across the p biomarkers have been shown to be superior in small n setting compared to a one-biomarker-at-a-time analysis [Smyth (71)].
The third task explores commonalities across the samples at the level of the p biomarkers. For example exploring the molecular heterogeneity of patients can provide new insights on the disease biology and the mode of action of a new molecular entity. Several approaches have been developed for unsupervised learning in the p >> n setting [Monti et al. (72), Städler et al. (73)]. In addition, dimensionality reduction methods such as principle component analysis (PCA), multidimensional scaling (MDS) and t-distributed stochastic neighbor embedding (t-SNE) are frequently used to visualize the high-dimensional data with fewer dimensions and identify patters. In a recent example such statistical approaches helped to decipher the single-cell level phenotypical and transcriptional consequences of treatment with anti-PD-1 and with PD1-TIM3 and PD1-LAG3 bispecific antibodies [Natoli et al. (74)].
Longitudinal mathematical models are powerful tools that describe temporal changes of a biomarker upon treatment administration and therefore leverage the entirety of the data collected during a study, without the need to rely on a single time-point (landmark analysis) or to discretize data. Here we focus on top-down population pharmacokinetic (PK)/PD models which are predominantly built on observed clinical data and are mostly empirical or semi-mechanistic. These non-linear mixed effect models describe the relationship between the pharmacokinetics of the drug (e.g. the plasma concentration-time course) and the dynamics of a response variable. The model structure is formulated via a set of differential equations defined by estimated model parameters. The model includes statistical distributions (e.g. normal, log-normal) representing between-patient variability in both PK and PD parameters, which can be quantified and distinguished from residual unexplained variability. The PK component describes how the drug is absorbed, distributed, and cleared from the body, including target-mediated drug disposition when applicable. The PD component offer a simplified mathematical representation of the patho-physiological processes relevant to biomarker response. A link function between PK and PD characterizes the exposure-response relationship and quantifies the part of the biomarker response variability that can be explained by variability in PK. In addition, covariates such as patient baseline characteristics can be evaluated during model building to explain (part of) the variability in PK and PD. By further including additional components associated to clinical response or safety outcomes of interest, modeling frameworks can be built to answer specific questions (fit-for-purpose). Model-based simulations can then be used for in-silico exploration of untested dosing scenarios that can be further evaluated in the clinic, project long-term response, or make prospective predictions for a new patient population. Published examples (Ribba et al. (75), Silber Baumann et al. (76), Netterberg et al. (77), Chen et al. (78)) show the potential of PK/PD models to help the selection of doses and schedules (e.g. induction-maintenance doses, priming doses) to maximize efficacy and/or mitigate safety risks of CIT. These models may be updated during clinical drug development based on new arising data and questions (learn and confirm paradigm). Of note, identification of relevant biomarkers based on exploratory data analysis are a prerequisite to the development of such models. In addition, informative schedule of assessments for PK and biomarker, and timely availability of data to modeling teams, are key elements to the ensure a meaningful delivery of modeling outputs for decision making. Other mathematical modeling approaches, including mechanistic bottom-up and middle-out approaches that rely less on clinical data are out of scope but have been recently reviewed elsewhere (79).
Well conducted biomarker-driven clinical trials can increase the success rates in drug development. However, a critical condition for success is that relevant prognostic or predictive baseline biomarkers or pharmacodynamic on-treatment biomarkers for clinical efficacy or safety outcomes can be identified. Despite a plethora of examples and some success stories (see Table 1 in Supplemental Material), the identification of an optimal biomarker proves extremely challenging, most likely due to the complex and dynamic interplay between the tumor and the host immune system. Many publications identify new biomarkers but there are much fewer studies which aim to demonstrate their clinical validity and utility and fewer again enter clinical practice. Moreover, a number of research studies have highlighted deficiencies in some areas of biomarker research. As an example, Malats et al. (10) concluded in a systematic review of 168 publications from 117 studies that despite all of this research, there is still no sufficient evidence to conclude whether changes in p53 act as markers of outcome in patients with bladder cancer. More recently, a systematic review of Kempf et al. (11) found evidence of frequent overinterpretation of findings of prognostic factor assessment in high-impact medical oncology journals.
In the introduction, we introduced four key challenges in the development of CIT: 1) demonstration of MoA, 2) dose selection and optimization, 3) mitigation and prevention of immune related adverse events and 4) patient enrichment and patient selection. A major complexity of CIT development is the indirect targeting of the tumor through the immune system which requires a better understanding of the immune system and how it is impacted by the drug. Biomarker technologies provide tools that can support addressing these key challenges. In this manuscript, we reviewed important methodological aspects in the analysis of biomarkers, (summarized in Supplementary Table 2). We covered basic aspects of biomarker modeling, analyses of low-dimensional baseline and on-treatment biomarkers, high-dimensional analysis, and topics related to dose finding and PK/PD modeling.
This article covers a broad range of statistical methods for biomarker analyses relevant to the early development of cancer immunotherapies. However, we also acknowledge some limitations. First, we did not provide a detailed description of statistical methods and their relative merits, as this would have required extending the article substantially. Instead, we aimed to provide an overview of methods, highlight potential pitfalls, and give relevant references that cover these methods in-depth. Second, analytical validation of biomarkers is not covered in this article at all (Ou et al. (46), Kraus (47)). Third, our article primarily focuses on an early development program setting where biomarkers are analyzed for exploratory and hypothesis-generating purposes. In such settings, study designs (e.g., dose escalation designs covered in Section 3.2) are usually not specifically designed for biomarker analyses. We have only briefly discussed the important topic of confirmatory randomized clinical trials which are required to establish the clinical utility of baseline biomarkers at the end of Sections 3.3 and 3.4. Important design options at this stage include biomarker-stratified designs, enrichment designs, and biomarker-strategy designs [Freidlin et al. (80), Tajik et al. (81)]. A general overview of the strategic use of biomarkers as a drug development tool and regulatory pathways is provided by Kraus (47).
The aim of this manuscript was to highlight key statistical consideration and present valid analysis methods for the identification and evaluation of biomarkers relevant to early clinical development in the CIT field. We hope that it supports data scientists and other drug developers to derive robust and reproducible conclusions.
DD: Conceptualization, Methodology, Writing – original draft, Writing – review & editing. AK: Conceptualization, Methodology, Writing – original draft, Writing – review & editing. ES: Conceptualization, Methodology, Writing – original draft, Writing – review & editing. NS: Conceptualization, Methodology, Writing – original draft, Writing – review & editing. MW: Conceptualization, Methodology, Writing – original draft, Writing – review & editing.
The author(s) declare financial support was received for the research, authorship, and/or publication of this article. The authors declare that this work was funded by F. Hoffmann-La Roche AG. The views, opinions, and content expressed in this publication are those of the authors and do not necessarily reflect the views, positions, or policies of the funder.
All authors were employed by company F.Hoffmann-La Roche AG.
All claims expressed in this article are solely those of the authors and do not necessarily represent those of their affiliated organizations, or those of the publisher, the editors and the reviewers. Any product that may be evaluated in this article, or claim that may be made by its manufacturer, is not guaranteed or endorsed by the publisher.
The Supplementary Material for this article can be found online at: https://www.frontiersin.org/articles/10.3389/fimmu.2024.1351584/full#supplementary-material
1. Shahid K, Khalife M, Dabney R, Phan AT. Immunotherapy and targeted therapy—the new roadmap in cancer treatment. Ann Trans Med. (2019) 7. doi: 10.21037/atm.2019.05.58
2. Esfahani K, Roudaia L, Buhlaiga N, Del Rincon S, Papneja N, Miller W. A review of cancer immunotherapy: from the past, to the present, to the future. Curr Oncol. (2020) 27:87–97. doi: 10.3747/co.27.5223
3. Robert C. A decade of immune-checkpoint inhibitors in cancer therapy. Nat Commun. (2020) 11:3801. doi: 10.1038/s41467-020-17670-y
4. Murciano-Goroff YR, Warner AB, Wolchok JD. The future of cancer immunotherapy: microenvironment-targeting combinations. Cell Res. (2020) 30:507–19. doi: 10.1038/s41422-020-0337-2
5. Hartl D, de Luca V, Kostikova A, Laramie J, Kennedy S, Ferrero E, et al. Translational precision medicine: an industry perspective. J Trans Med. (2021) 19:1–14. doi: 10.1186/s12967-021-02910-6
6. Aronson JK, Ferner RE. Biomarkers—a general review. Curr Protoc Pharmacol. (2017) 76:9–23. doi: 10.1002/cpph.19
7. Sankar K, Ye JC, Li Z, Zheng L, Song W, Hu-Lieskovan S. The role of biomarkers in personalized immunotherapy. biomark Res. (2022) 10:1–13. doi: 10.1186/s40364-022-00378-0
8. Lee JY, Kannan B, Lim BY, Li Z, Lim AH, Loh JW, et al. The multi-dimensional biomarker landscape in cancer immunotherapy. Int J Mol Sci. (2022) 23:7839. doi: 10.3390/ijms23147839
9. Downing G. Biomarkers definitions working group. biomarkers and surrogate endpoints. Clin Pharmacol Ther. (2001) 69:89–95. doi: 10.1067/mcp.2001.113989
10. Malats N, Bustos A, Nascimento CM, Fernandez F, Rivas M, Puente D, et al. P53 as a prognostic marker for bladder cancer: a meta-analysis and review. Lancet Oncol. (2005) 6:678–86. doi: 10.1016/S1470-2045(05)70315-6
11. Kempf E, de Beyer JA, Cook J, Holmes J, Mohammed S, Nguyên T-L, et al. Overinterpretation and misreporting of prognostic factor studies in oncology: a systematic review. Br J Cancer. (2018) 119:1288–96. doi: 10.1038/s41416-018-0305-5
12. Food and Drug Administration. Enrichment strategies for clinical trials to support approval of human drugs and biological products. Rockville, MD: Food and Drug Administration (2012).
13. Galon J, Pagès F, Marincola FM, Angell HK, Thurin M, Lugli A, et al. Cancer classification using the immunoscore: a worldwide task force. J Trans Med. (2012) 10:1–10. doi: 10.1186/1479-5876-10-1
14. Davis AA, Patel VG. The role of pd-l1 expression as a predictive biomarker: an analysis of all us food and drug administration (fda) approvals of immune checkpoint inhibitors. J immunotherapy Cancer. (2019) 7:1–8. doi: 10.1186/s40425-019-0768-9
16. Austin PC. An introduction to propensity score methods for reducing the effects of confounding in observational studies. Multivariate Behav Res. (2011) 46:399–424. doi: 10.1080/00273171.2011
17. Brookhart MA, Schneeweiss S, Rothman KJ, Glynn RJ, Avorn J, Stürmer T. Variable selection for propensity score models. Am J Epidemiol. (2006) 163:1149–56. doi: 10.1093/aje/kwj149
18. Conlon KC, Lugli E, Welles HC, Rosenberg SA, Fojo AT, Morris JC, et al. Redistribution, hyperproliferation, activation of natural killer cells and CD8 T cells, and cytokine production during first-in-human clinical trial of recombinant human interleukin-15 in patients with cancer. J Clin Oncol. (2015) 33:74–82. doi: 10.1200/JCO.2014.57.3329
19. Burzykowski T, Molenberghs G, Buyse M. The evaluation of surrogate endpoints. New York: Springer (2005).
20. Pabst T, Joncourt R, Shumilov E, Heini A, Wiedemann G, Legros M, et al. Analysis of IL-6 serum levels and CAR t cell-specific digital PCR in the context of cytokine release syndrome. Exp Hematol. (2020) 88:7–14.e3. doi: 10.1016/j.exphem.2020.07.003
21. Therasse P, Arbuck SG, Eisenhauer EA, Wanders J, Kaplan RS, Rubinstein L, et al. New guidelines to evaluate the response to treatment in solid tumors. J Natl Cancer Institute. (2000) 92:205–16. doi: 10.1093/jnci/92.3.205
22. Anagnostou V, Yarchoan M, Hansen AR, Wang H, Verde F, Sharon E, et al. Immuno-oncology trial endpoints: capturing clinically meaningful activity. Clin Cancer Res. (2017) 23:4959–4969. doi: 10.1158/1078-0432.CCR-16-3065
23. Stein WD, Gulley JL, Schlom J, Madan RA, Dahut W, Figg WD, et al. Tumor regression and growth rates determined in five intramural nci prostate cancer trials: The growth rate constant as an indicator of therapeutic efficacy. Clin Cancer Res. (2011) 907–17. doi: 10.1158/1078-0432.CCR-10-1762
24. Box GE, Cox DR. An analysis of transformations. J R Stat Society: Ser B (Methodological). (1964) 26:211–43. doi: 10.1111/j.2517-6161.1964.tb00553.x
25. Warton DI, Hui FK. The arcsine is asinine: the analysis of proportions in ecology. Ecology. (2011) 92:3–10. doi: 10.1890/10-0340.1
26. McCullagh P, Nelder JA. Generalized linear models. Routledge: Taylor and francis New York Statistics in Biopharmaceutical Research (2019) 16(3):326–37.
27. Helsel DR. More than obvious: better methods for interpreting nondetect data. Environ Sci Technol. (2005) 39:419A–23A. doi: 10.1021/es053368a
28. Carroll RJ, Ruppert D, Stefanski LA. Measurement error in nonlinear models. In: Measurment-error, vol. 105. Boca Raton: CRC press (1995).
29. Bartlett NL, Sehn LH, Assouline SE, Bosch F, Magid Diefenbach CS, Flinn I, et al. Managing cytokine release syndrome (crs) and neurotoxicity with step-fractionated dosing of mosunetuzumab in relapsed/refractory (r/r) b-cell non-hodgkin lymphoma (nhl). J Clin Oncol. (2019) 37:7518–8. doi: 10.1200/JCO.2019.37.15\suppl.7518
30. Xu C, Zhuo B, Rasmussen HE. Dose intra-subject escalation to an event (diete): A new method for phase 1 dose-finding utilizing systematic intra-subject dose escalation with application to t-cell engagers. Pharm Stat. (2021) 1200–15. doi: 10.1002/pst.2140
31. Gerard E, Zohar S, Lorenzato C, Ursino M, Riviere M-K. Bayesian modeling of a bivariate toxicity outcome for early phase oncology trials evaluating dose regimens. Stat Med. (2021) 40:5096–114. doi: 10.1002/sim.9113
32. Dejardin D, Huang B, Yuan Y, Beyer U, Fridlyand J, Zhu J. Dose optimization for novel oncology agents: Design options and strategies. Stat Biopharmaceutical Res. (2024) 16(3):326–37. doi: 10.1080/19466315.2024.2308856
33. Wages NA, Chiuzan C, Panageas KS. Design considerations for early-phase clinical trials of immune-oncology agents. J immunotherapy Cancer. (2018) 6:1–10. doi: 10.1186/s40425-018-0389-8
34. Bretz F, Pinheiro JC, Branson M. Combining multiple comparisons and modeling techniques in dose-response studies. Biometrics. (2005) 61:738–48. doi: 10.1111/j.1541-0420.2005.00344.x
35. Nie L, Rubin EH, Mehrotra N, Pinheiro J, Fernandes LL, Roy A, et al. Rendering the 3 + 3 design to rest: More efficient approaches to oncology dose-finding trials in the era of targeted therapy. Clin Cancer Res. (2016) 22:2623–9. doi: 10.1158/1078-0432.CCR-15-2644
37. Harrell FE. Regression modeling strategies. 2nd ed. Boca Raton: Springer Series in Statistics (2015).
38. Polley M-YC, Dignam JJ. Statistical considerations in the evaluation of continuous biomarkers. J Nucl Med. (2021) 62:605–11. doi: 10.2967/jnumed.120.251520
39. Steyerberg E. Clinical prediction models: A practical approach to development, validation, and updating. 2nd ed. New York: Springer - Statistics for Biology and Health (2019).
40. Riley RD, Ensor J, Snell KI, Harrell FE, Martin GP, Reitsma JB, et al. Calculating the sample size required for developing a clinical prediction model. Bmj. (2020) 368:m441. doi: 10.1136/bmj.m441
41. Phung MT, Tin Tin S, Elwood JM. Prognostic models for breast cancer: a systematic review. BMC Cancer. (2019) 19:1–18. doi: 10.1186/s12885-019-5442-6
42. Collins GS, Reitsma JB, Altman DG, Moons KG. Transparent reporting of a multivariable prediction model for individual prognosis or diagnosis (tripod): the tripod statement. Ann Internal Med. (2015) 162:55–63. doi: 10.7326/M14-0697
43. Steyerberg EW. Validation in prediction research: the waste by data splitting. J Clin Epidemiol. (2018) 103:131–3. doi: 10.1016/j.jclinepi.2018.07.010
44. Riley RD, Collins GS. Stability of clinical prediction models developed using statistical or machine learning methods. Biometrical J. (2023) 65:2200302. doi: 10.1002/bimj.202200302
45. Moons KG, Royston P, Vergouwe Y, Grobbee DE, Altman DG. Prognosis and prognostic research: what, why, and how? BMJ. (2009) 338:b375. doi: 10.1136/bmj.b375
46. Ou F-S, Michiels S, Shyr Y, Adjei AA, Oberg AL. Biomarker discovery and validation: statistical considerations. J Thorac Oncol. (2021) 16:537–45. doi: 10.1016/j.jtho.2021.01.1616
47. Kraus VB. Biomarkers as drug development tools: discovery, validation, qualification and use. Nat Rev Rheumatol. (2018) 14:354–62. doi: 10.1038/s41584-018-0005-9
48. Alosh M, Huque MF, Bretz F, D’Agostino SRB. Tutorial on statistical considerations on subgroup analysis in confirmatory clinical trials. Stat Med. (2017) 36:1334–60. doi: 10.1002/sim.7167
49. Lipkovich I, Dmitrienko A, B D’Agostino SR. Tutorial in biostatistics: data-driven subgroup identification and analysis in clinical trials. Stat Med. (2017) 36:136–96. doi: 10.1002/sim.7064
50. Schandelmaier S, Briel M, Varadhan R, Schmid CH, Devasenapathy N, Hayward RA, et al. Development of the instrument to assess the credibility of effect modification analyses (iceman) in randomized controlled trials and meta-analyses. Cmaj. (2020) 192:E901–6. doi: 10.1503/cmaj.200077
51. Schmid P, Adams S, Rugo HS, Schneeweiss A, Barrios CH, Iwata H, et al. Atezolizumab and nab-paclitaxel in advanced triple-negative breast cancer. New Engl J Med. (2018) 379:2108–21. doi: 10.1056/NEJMoa1809615
52. Nguyen Duc A, Heinzmann D, Berge C, Wolbers M. A pragmatic adaptive enrichment design for selecting the right target population for cancer immunotherapies. Pharm Stat. (2021) 20:202–11. doi: 10.1002/pst.2066
53. Mittendorf EA, Zhang H, Barrios CH, Saji S, Jung KH, Hegg R, et al. Neoadjuvant atezolizumab in combination with sequential nab-paclitaxel and anthracycline-based chemotherapy versus placebo and chemotherapy in patients with early-stage triple-negative breast cancer (impassion031): a randomised, double-blind, phase 3 trial. Lancet. (2020) 396:1090–100. doi: 10.1016/S0140-6736(20)31953-X
54. Mantel N, Byar DP. Evaluation of response-time data involving transient states: An illustration using heart-transplant data. J Am Stat Assoc. (1974) 69:81–6. doi: 10.1080/01621459.1974.10480131
55. Anderson JR, Cain KC, Gelber RD. Analysis of survival by tumor response. J Clin Oncol. (1983) 1:710–9. doi: 10.1200/JCO.1983.1.11.710
56. Proust-Lima C, Taylor JMG. Development and validation of a dynamic prognostic tool for prostate cancer recurrence using repeated measures of posttreatment PSA: a joint modeling approach. Biostatistics. (2009) 10:535–49. doi: 10.1093/biostatistics/kxp009
57. Van Houwelingen HC. Dynamic prediction by landmarking in event history analysis. Scandinavian J Stat. (2007) 34:70–85. doi: 10.1111/j.1467-9469.2006.00529.x
58. Rizopoulos D. Joint models for longitudinal and time-to-event data: With applications in R. Boca Raton: CRC press (2012).
59. Putter H, Fiocco M, Geskus RB. Tutorial in biostatistics: competing risks and multi-state models. Stat Med. (2007) 26:2389–430. doi: 10.1002/sim.2712
60. Bornkamp B, Rufibach K, Lin J, Liu Y, Mehrotra DV, Roychoudhury S, et al. Principal stratum strategy: potential role in drug development. Pharm Stat. (2021) 20:737–51. doi: 10.1002/pst.2104
61. Kong S, Heinzmann D, Lauer S, Tian L. Weighted approach for estimating effects in principal strata with missing data for a categorical post-baseline variable in randomized controlled trials. Stat Biopharmaceutical Res. (2022) 15(1):187–97. doi: 10.1080/19466315.2021.2009020
62. Austin PC, Stuart EA. Moving towards best practice when using inverse probability of treatment weighting (iptw) using the propensity score to estimate causal treatment effects in observational studies. Stat Med. (2015) 34:3661–79. doi: 10.1002/sim.6607
63. Vickers AJ. Change/Percent Change From Baseline. In Wiley Encyclopedia of Clinical Trials eds. D'Agostino R.B., Sullivan L., Massaro J. (2008). doi: 10.1002/9780471462422.eoct429
64. Steyerberg EW, Vergouwe Y. Towards better clinical prediction models: seven steps for development and an ABCD for validation. Eur Heart J. (2014) 35(29):1925–31.
65. Kenward MG, White IR, Carpenter JR. Letter to the editor: Should baseline be a covariate or dependent variable in analyses of change from baseline in clinical trials? Stat Med. (2009) 28:2509–30. doi: 10.1002/sim.3868
66. Bühlmann P, Van De Geer S. Statistics for high-dimensional data: methods, theory and applications. New York: Springer Science & Business Media (2011).
67. Wainwright MJ. High-dimensional statistics: A non-asymptotic viewpoint Vol. 48. Cambridge: Cambridge University Press (2019).
68. Hastie T, Tibshirani R, Friedman JH, Friedman JH. The elements of statistical learning: data mining, inference, and prediction. 2nd ed. New York: Springer Series in Statistics (2009).
69. Khan Z, Hammer C, Carroll J, Di Nucci F, Acosta SL, Maiya V, et al. Genetic variation associated with thyroid autoimmunity shapes the systemic immune response to pd-1 checkpoint blockade. Nat Commun. (2021) 12:3355. doi: 10.1038/s41467-021-23661-4
70. Dudoit S, van der Laan MJ, van der Laan MJ. Multiple testing procedures with applications to genomics. New York: Springer (2008).
71. Smyth GK. Linear models and empirical bayes methods for assessing differential expression in microarray experiments. Stat Appl Genet Mol Biol. (2004) 3. doi: 10.2202/1544-6115.1027
72. Monti S, Tamayo P, Mesirov J, Golub T. Consensus clustering: a resampling-based method for class discovery and visualization of gene expression microarray data. Mach Learn. (2003) 52:91–118. doi: 10.1023/A:1023949509487
73. Städler N, Dondelinger F, Hill SM, Akbani R, Lu Y, Mills GB, et al. Molecular heterogeneity at the network level: high-dimensional testing, clustering and a tcga case study. Bioinformatics. (2017) 33:2890–6. doi: 10.1093/bioinformatics/btx322
74. Natoli M, Hatje K, Gulati P, Junker F, Herzig P, Jiang Z, et al. Deciphering molecular and cellular ex vivo responses to bispecific antibodies pd1-tim3 and pd1-lag3 in human tumors. J Immunotherapy Cancer. (2022) 10. doi: 10.1136/jitc-2022-005548
75. Ribba B, Boetsch C, Nayak T, Grimm HP, Charo J, Evers S, et al. Prediction of the optimal dosing regimen using a mathematical model of tumor uptake for immunocytokine-based cancer immunotherapy optimal dosing regimen for cancer immunotherapy. Clin Cancer Res. (2018) 24:3325–33. doi: 10.1158/1078-0432.CCR-17-2953
76. Silber Baumann HE, Boetsch C, Charo J, Petry C, Teichgräber V, Cosson V. PKPD analysis of soluble CD25 to characterize the concentration-effect relationship observed following the administration of cergutuzumab amunaleukin, a targeted immunocytokine for cancer immunotherapy. (2018).
77. Netterberg I, Li C-C, Molinero L, Budha N, Sukumaran S, Stroh M, et al. A pk/pd analysis of circulating biomarkers and their relationship to tumor response in atezolizumab-treated non-small cell lung cancer patients. Clin Pharmacol Ther. (2019) 105:486–95. doi: 10.1002/cpt.1198
78. Chen X, Kamperschroer C, Wong G, Xuan D. A modeling framework to characterize cytokine release upon t-cell–engaging bispecific antibody treatment: methodology and opportunities. Clin Trans Sci. (2019) 12:600–8. doi: 10.1111/cts.12662
79. Valentinuzzi D, Jeraj R. Computational modelling of modern cancer immunotherapy. Phys Med Biol. (2020) 65:24TR01. doi: 10.1088/1361-6560/abc3fc
80. Freidlin B, McShane LM, Korn EL. Randomized clinical trials with biomarkers: design issues. J Natl Cancer Institute. (2010) 102:152–60. doi: 10.1093/jnci/djp477
Keywords: biomarkers, statistical methods, validation, immunotherapy, prognostic model, predictive model
Citation: Dejardin D, Kraxner A, Schindler E, Städler N and Wolbers M (2024) An overview of statistical methods for biomarkers relevant to early clinical development of cancer immunotherapies. Front. Immunol. 15:1351584. doi: 10.3389/fimmu.2024.1351584
Received: 06 December 2023; Accepted: 29 July 2024;
Published: 21 August 2024.
Edited by:
Brian J. Czerniecki, Moffitt Cancer Center, United StatesReviewed by:
Reynaldo Martina, Utrecht University, NetherlandsCopyright © 2024 Dejardin, Kraxner, Schindler, Städler and Wolbers. This is an open-access article distributed under the terms of the Creative Commons Attribution License (CC BY). The use, distribution or reproduction in other forums is permitted, provided the original author(s) and the copyright owner(s) are credited and that the original publication in this journal is cited, in accordance with accepted academic practice. No use, distribution or reproduction is permitted which does not comply with these terms.
*Correspondence: David Dejardin, ZGF2aWQuZGVqYXJkaW5Acm9jaGUuY29t
†These authors have contributed equally to this work.
Disclaimer: All claims expressed in this article are solely those of the authors and do not necessarily represent those of their affiliated organizations, or those of the publisher, the editors and the reviewers. Any product that may be evaluated in this article or claim that may be made by its manufacturer is not guaranteed or endorsed by the publisher.
Research integrity at Frontiers
Learn more about the work of our research integrity team to safeguard the quality of each article we publish.