- 1College of Public Health, The Ohio State University, Columbus, OH, United States
- 2College of Arts and Sciences, The Ohio State University, Columbus, OH, United States
- 3Department of Biomedical Informatics, The Ohio State University, Columbus, OH, United States
Background: Immune checkpoint inhibitors (ICIs) have reshaped the treatment landscape of small cell lung cancer (SCLC), but only a minority of patients benefit from this therapy. Therefore, it is critical to identify potential risk factors that could predict the efficacy of ICI treatment in SCLC patients and identify patient subgroups who may benefit the most from ICI therapy.
Methods: Our study included a total of 183 SCLC patients who had received at least one dose of ICI treatment. We utilized both logistic regression and Cox proportional hazard regression to evaluate whether various patient clinical factors and serum biomarkers could serve as predictors of patient response to treatment and overall survival (OS) during ICI therapy.
Results: Logistic regression showed that patients with a history of surgery (p=0.003, OR 9.06, 95% CI: (2.17, 37.9)) and no metastasis (p=0.008, OR 7.82, 95% CI: (1.73, 35.4)) exhibited a higher odds of response to ICI treatment. Cox regression analyses demonstrated that pretreatment blood albumin (p=0.003, HR 1.72, 95% CI: (1.21, 2.45)) and derived neutrophil to lymphocyte ratio (dNLR) (p=0.003, HR 1.71, 95% CI: (1.20–2.44)) were independent predictors for OS in SCLC patients. By establishing a pre-treatment prognostic scoring system based on baseline albumin and dNLR, we found that patients with high albumin and low dNLR exhibited a significantly better prognosis than those with low albumin and high dNLR in both the full (P<.0001, HR 0.33, 95% CI: 0.20–0.55) and the metastatic cohort (P<.0001, HR 0.28, 95% CI: 0.15–0.51). The better prognostic group also had younger age, higher BMI and lower systemic inflammatory biomarker values than the unfavorable group (P<.0001).
Conclusion: Our data reveals the significant role of metastasis status and treatment history in predicting the initial response of SCLC patients to ICI treatment. However, baseline serum albumin and dNLR provide a more precise prognostic prediction for patient OS. The scoring system based on albumin and dNLR enhances the ability to stratify patient prognosis and holds the potential to guide clinical decision-making for SCLC patients undergoing ICI therapy.
Introduction
Small cell lung cancer (SCLC) is an exceptionally aggressive malignancy characterized by early metastasis and rapid tumor growth, resulting in a discouraging 5-year overall survival rate ranging only from 1% to 5% (1, 2). SCLC represents approximately 13–15% of total lung cancer cases (3) and results in between 25,000–30,000 deaths annually in the United States. SCLC is categorized into two primary stages based on the staging system: limited-disease SCLC (LD-SCLC) and extensive-disease SCLC (ED-SCLC). Majority of patients, around two-thirds, are diagnosed with extensive stage or metastatic disease at the time of diagnosis (4). In contrast to non-small cell lung cancer (NSCLC), which benefits from a wide array of treatment options, including targeted agents and immunotherapies (5–9), the treatment landscape for SCLC has seen little evolution over the past few decades, while platinum-based chemotherapy in combination with etoposide remains the standard of care for SCLC (10).
Immunotherapy, particularly immune checkpoint inhibitors (ICIs), have revolutionized cancer treatment, yielding increased response rates and prolonged survival for a wide range of cancer types. By targeting key immune checkpoint molecules like programmed cell death protein 1 (PD-1), programmed cell death ligand 1 (PD-L1), and cytotoxic T lymphocyte-associated antigen 4 (CTLA-4), ICIs have the capacity to stimulate T cells and activate the immune system, enabling it to target and kill cancer cells (11). In the case of SCLC, the recent introduction of ICIs has reshaped its treatment landscape. Notably, the combination of anti-PD-L1 antibody durvalumab and atezolizumab with platinum-based chemotherapy (either carboplatin or cisplatin plus etoposide) has emerged as a first line treatment for patients with extensive stage SCLC, exhibiting significantly improved overall survival (12–15). However, the efficacy of immunotherapy remains limited, benefiting only a small subset of SCLC patients (16–19). Hence, it is critical to identify factors and biomarkers predicting the efficacy of ICI therapy in SCLC patients and identify SCLC patients who may benefit from ICI treatment.
Recent studies have explored various potential risk factors for predicting the effectiveness of ICI treatment. Tumor mutational burden (TMB) (20) and PD-L1 positivity (21) are among the most studied (22, 23). Additionally, factors such as metastasis status (24, 25) and several serum-based biomarkers, including systemic inflammatory biomarkers like neutrophil to lymphocyte ratio (NLR) (23, 26, 27) and derived NLR (28), albumin levels (29, 30), platelet to lymphocyte ratio (23, 31), lactate dehydrogenase (LDH) (32–34), Lung Immune Prognostic Index that integrates dNLR and LDH (23, 35, 36), have shown promise as prognostic indicators for ICI efficacy (37). However, it’s important to note that increased TMB and high PD-L1 expression do not consistently result in better treatment responses (17, 20, 22, 38). Additionally, other biomarkers have also produced conflicting results, underscoring the necessity for further research to validate their predictive roles (34, 37). Furthermore, given the lack of prior assessment of these factors in SCLC patients who receive ICI-involved immunochemotherapy, it is of particular importance to investigate these biomarkers in predicting ICI outcomes in SCLC. This research holds significant potential to aid in the development of more precise and effective treatment strategies for SCLC patients.
In this study, we present the results of a retrospective SCLC cohort data analysis conducted at a single institution. This analysis investigates the impact of baseline clinical factors and various serum inflammatory biomarkers, on the effectiveness of ICI treatment in patients with SCLC. Our primary objective is to contribute to the identification of specific patient subgroups that are best suited for ICI treatment. We believe that these findings can offer valuable guidance for clinical decision-making and ultimately lead to the improvement of patient care.
Methods
Study cohort and patient clinical information
The clinical data of SCLC patients were collected by Pelotonia Institute for Immuno-Oncology (PIIO) at The Ohio State University (OSU) Wexner Medical Center under approved Institutional Review Board (IRB) protocol IRB 2020C0145. The data gathered retrospectively from the Clarity electronic medical record database, which stores Epic/IHIS data of patients. The study included a total of 183 SCLC patients who received at least one dose of immune checkpoint inhibitor treatment (which included anti-PD1 inhibitors: Pembrolizumab, Nivolumab, Cemiplimab; anti-PD-L1 inhibitors: Atezolizumab, Durvalumab, and Avelumab; and anti-CTLA4 inhibitors: Ipilimumab) between November 2011 and December 2021. The patient inclusion criteria required that patients needed to be over 18 years of age at enrollment, had only one cancer diagnosis, received treatment within the study period, and had available overall survival data.
The patient clinical information and categorization of each variable are provided as a codebook in Supplementary Data 1. The patient baseline characteristics are summarized in Supplementary Table S1. The median age was 64 (range: 32–91) years, and the median Body Mass Index (BMI) was 25.3 (range: 13.7–56.0) kg/m2. There was a higher representation of males (56.3%) than females. Majority of patients were white (90.2%), married (57.4%), and retired (54.6%).
Among all the patients who had clinical stage information, 43.1% (59 out of 137) had advanced tumor stage, while 78.2% (129 out of 165) had advanced N stage, and 76.8% (129 out of 168) had metastasis. All 183 patients received a combination of ICI and chemotherapy. Regarding the ICI type, 168 patients (91.8%) received anti-PD-1/PD-L1 inhibitor therapy, and 15 patients received a combination of anti-CTLA4 inhibitor (ipilimumab) and anit-PD1 inhibitor (nivolumab) treatment. Only 31 (16.9%) underwent surgery, and 70 (38.3%) received radiation treatment among all the patients.
Baseline blood was taken before treatment, and hematological markers included serum lactate dehydrogenase (LDH) (U/L), albumin (Alb) (g/L), eosinophil, neutrophil to lymphocyte ratio (NLR), derived neutrophil to lymphocyte ratio (dNLR= ANC/(WBC−ANC)), platelet to lymphocyte ratio (PLR), lymphocyte to monocyte ratio (LMR), systemic inflammation index (SII=Platelet counts x neutrophil counts/lymphocyte counts), and systemic inflammation response index (SIRI= neutrophil count × monocyte count/lymphocyte count). These hematological biomarkers were calculated as previously described (39, 40), and the data was dichotomized based on the median value (albumin, NLR, dNLR, PLR, LMR, SII, and SIRI) or upper limit of normal range (LDH>190 g/L as used in OSU medical center) (Supplementary Data 1). Levels of individual blood cells including neutrophils, WBC, lymphocytes, monocytes, and platelets were used for univariate analysis to test their association with patient survival but were not in the multivariate model even if the cell type was significantly associated with survival. This is to avoid repeated use of the same value and collinearity of variables as the systemic inflammatory biomarkers (NLR, dNLR, PLR, LMR, SII and SIRI) were calculated from these individual values.
Outcomes
This study focused on patient complete response and overall survival (OS). The data recorded patients’ first recurrence as “no recurrence”, “recurred”, and “never disease free” with date of recurrence defined as the date of radiographic detected disease. For response endpoint, we considered patients with “no recurrence” and “recurred” as those who were responders with complete response and others as non-responders. The OS time was defined as the time between the earliest ICI ordering and death for any reason or the end of the study period.
Statistical analyses
Continuous variables were analyzed with two-sample t-test or ANOVA. The hematological variables were first log transformed to reduce skewness and variance before comparison. Categorical variables were analyzed with Chi-square test or Fisher’s exact test. Univariate and multivariate logistic regression was used to test the association of risk factors with patient response. Log rank test with Kaplan-Meier curves, univariate and multivariate Cox proportional hazard regression was performed to evaluate the association between the listed factors and overall survival. Since missing values are a common issue for observational data, our data also have different levels of missingness for different variables. Therefore, for univariate analyses, we used the available values for each variable. For multivariate analyses, we used both complete data and imputed data. Variables with p < 0.05 in univariate analysis were included in the logistic regression or multivariate Cox proportional-hazards model and then backward selected until no further variables could be eliminated based on model fit (p < 0.05). Tests were considered statistically significant with a two-sided P-value of ≤0.05. SAS 9.4 and R-studio were used for analyses and making figures.
Prognostic scoring system development
For the development of a predictive scoring system for overall survival using pretreatment serum albumin and dNLR level, both variables were dichotomized into low (<median) and high (≥median) based on the individual median value. Patients with a low dNLR and high albumin levels received a score of 2 and were assigned into the “good prognosis group.” Patients with high dNLR and low albumin levels were assigned a score of 0, designating them as the “bad prognosis group.” Patients with either both high or both low levels of pretreatment albumin and dNLR received a score of 1 and were categorized as the “intermediate group”. Then the association between the different prognostic groups and OS was tested using Log-rank test, and further validated using k (k=3)-fold cross validation.
Results
Patient laboratory values
In this study, over 97% of patients had documented pre-treatment laboratory results. Table 1 and Supplementary Table S2 provides a summary of the median and interquartile range (IQR) for the available lab values. However, it’s important to note that LDH had a very high percentage of missing data (74.9%), whereas the remaining laboratory values had missingness ranging from 2.7% to 7.1%. For our multivariate model, all the selected lab biomarkers were imputed for separate analysis to ensure a more accurate examination.
Patient response
We first compared the clinical characteristics between responders and non-responders, as illustrated in Supplementary Table S3. Among all SCLC patients, only 15 out of 183 patients were considered as responders with complete response (8.2%), with 7 experiencing a later recurrence. Univariate logistic regression revealed that patients who underwent surgery (p<0.001), had a lower N stage (p<0.001), and had no metastasis (p<0.001) had significantly higher odds of response (Table 2). None of the blood biomarkers were significantly associated with patient response to ICI treatment. Multivariate analyses demonstrated that undergoing surgery (p=0.003, OR=9.06 (2.17, 37.86)) and absence of metastasis (p=0.008, OR=7.82 (1.73, 35.43)) were the independent variables associated with higher odds of response to ICI treatment (Table 2).
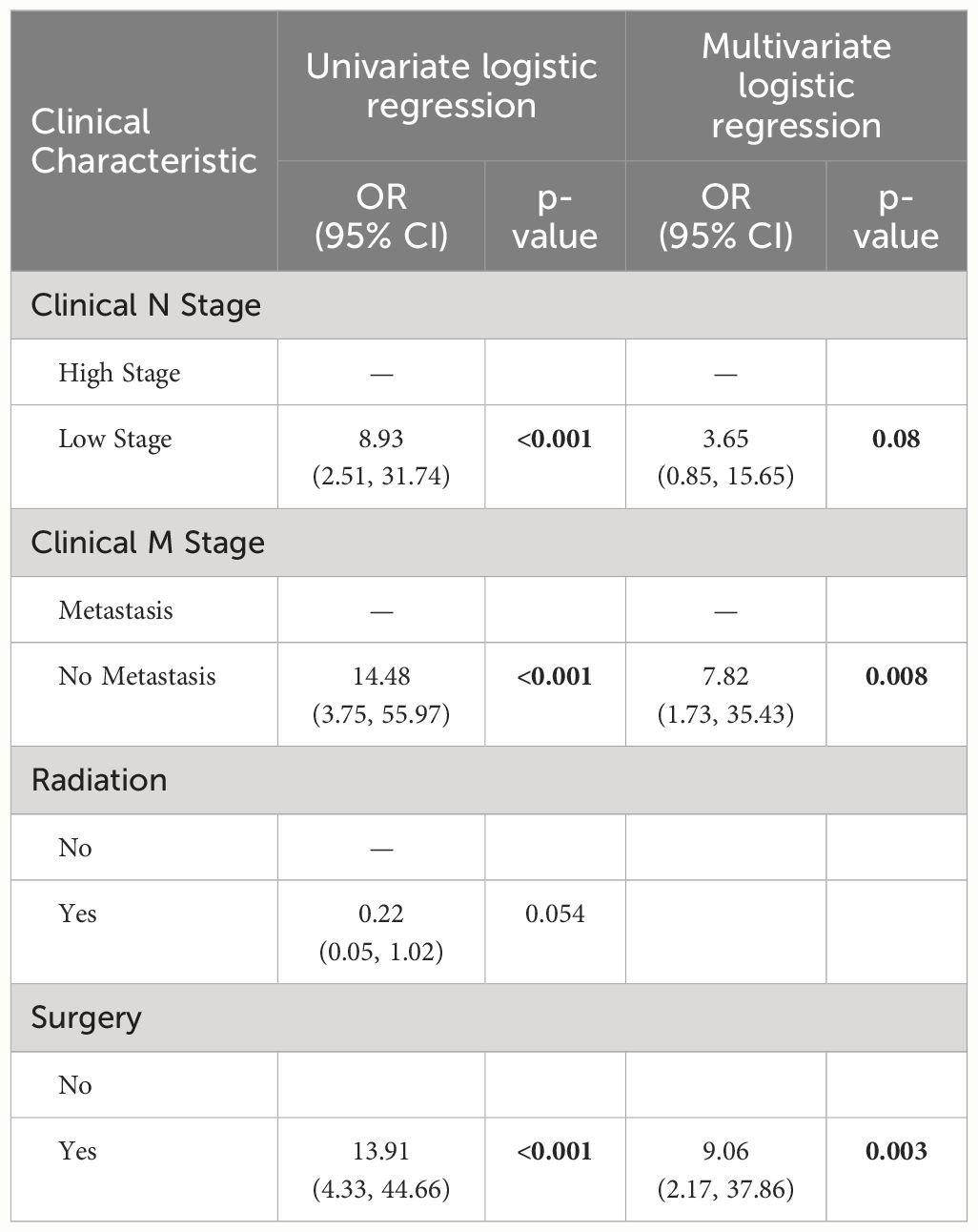
Table 2 Logistic regression analyses results showing the association of clinical factors with response to ICI therapy in SCLC patients (including only the variables with P<0.1 from univariate analysis).
Overall survival analysis of all patients
By the end of the study, 40 patients (21.9%) were still alive. The median survival of all patients was 8 (95%CI: 9.4,12.5) months. In the univariate analyses we found that patients with older age (≥64 years) (p=0.039, HR=1.42, 95%CI:1.02, 1.97), low albumin (≤3.7g/dL) (p<0.001, HR=1.80, 95%CI:1.28, 2.54), high NLR (>4.8) (p=0.006, HR=1.63, 95%CI:1.15, 2.31), and high dNLR (>2.5) (p=0.001, HR=1.76, 95%CI:1.24, 2.50) had significantly increased risk for worse OS (Table 3; Supplementary Table S4). Subsequently, based on the complete data (N=168, event=128), low blood albumin levels (p=0.003, HR 1.72, 95% CI: 1.21–2.45) and high dNLR (p=0.003, HR 1.71, 95% CI: 1.20–2.44) were identified as the independent risk factors predicting worse OS for SCLC in multivariate analysis. Figures 1A, B display the association of albumin and dNLR with OS, respectively, based on the Log-rank tests. Imputed data analyses with all 183 patients also showed similar results (Supplementary Data 2), where albumin and dNLR were the only independent predictors for OS.
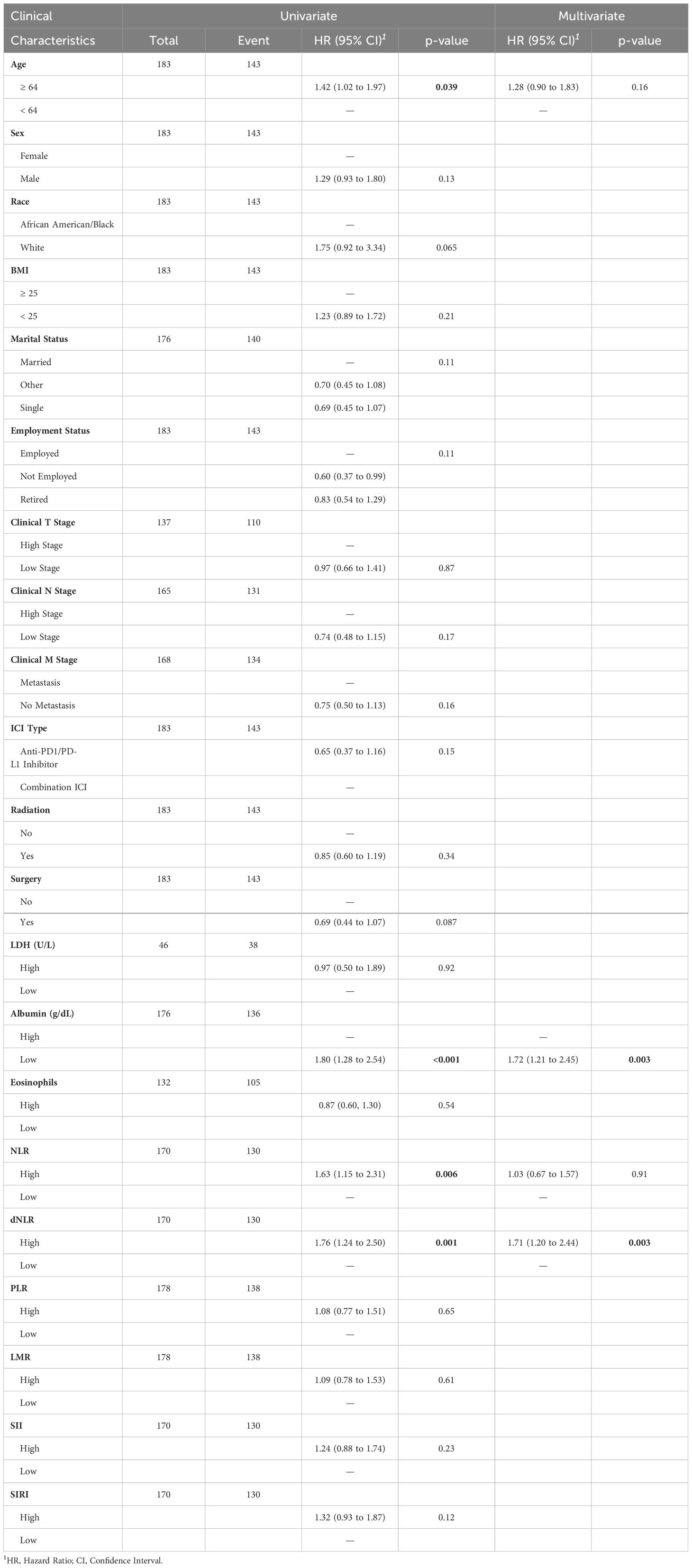
Table 3 Full patient cohort univariate and multivariate Cox proportional hazard regression model results.
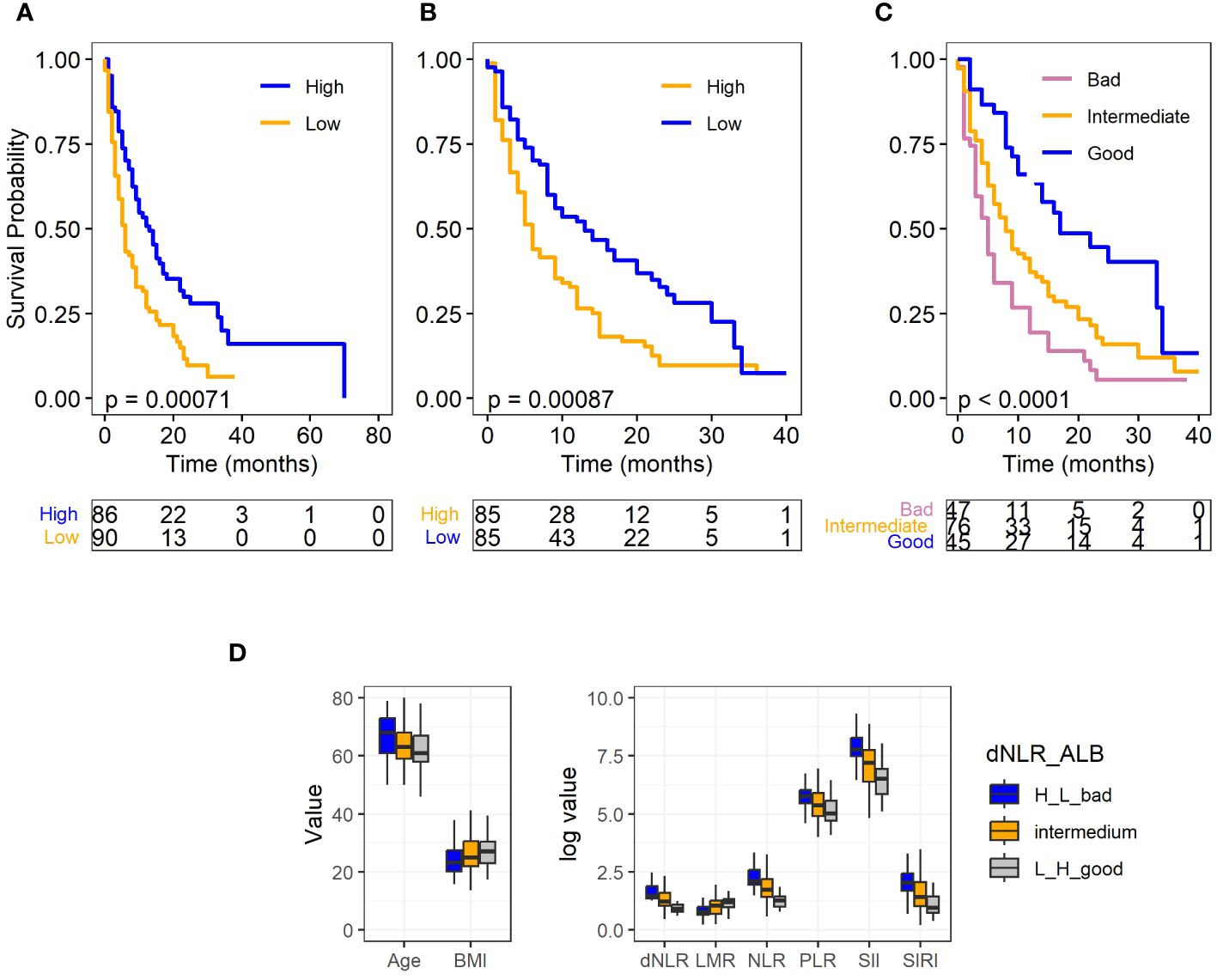
Figure 1 Survival plots for full SCLC cohort and levels of clinical factors in different prognostic groups. (A) Albumin level and OS. (B) dNLR level and OS. (C) Different prognostic groups and OS. (D) Levels of clinical factors including age, BMI, and hematological biomarkers in different prognostic groups. Albumin and dNLR was dichotomized to high and low according to their median values. Log-rank test was used for the analysis for the OS, and ANOVA was used for the comparison of the clinical factors and hematological biomarkers among different prognostic groups.
Prognostic scoring for full SCLC cohort
We proceeded to develop a prognostic scoring system that incorporates pretreatment serum albumin level and dNLR, and classified patients into three different prognostic groups. Patients with a low dNLR and high albumin levels received a score of 2 and were assigned into the “good prognosis group.” Conversely, those with high dNLR and low albumin levels were assigned a score of 0, designating them as the “bad prognosis group.” The rest of patients received a score of 1, placing them in the “intermediate group.” This group includes patients with either both high or both low levels of pretreatment albumin and dNLR.
Out of the 168 patients with complete data, 45 were categorized into the “good prognosis group,” 76 into the “intermediate group,” and 47 into the “bad prognosis group.” The median survival times for these groups were 14.91 months, 10.94 months, and 7.51 months, respectively. As demonstrated in Figure 1C, the log-rank test revealed a statistically significant difference in overall survival (P<.0001) among these three groups. The Cox model further confirmed that the good (p<.0001, HR: 0.33, 95% CI: 0.20–0.55) and intermediate groups (p=0.03, HR: 0.65, 95% CI: 0.44–0.96) exhibited a significantly reduced risk of death in comparison to the bad prognosis group. Furthermore, to test the robustness of our prognostic biomarker, a combination of dNLR and serum albumin level, we conducted an internal 3-fold cross validation. Despite each fold comprising only 33.3% of patient samples (n=56), our results demonstrated the stability of the prognostic power of the scoring system across different subsets of the data. The prognostic biomarker remained significantly associated with patient overall survival, with notable separation observed between the “good” and “bad” prognostic groups (Supplementary Figure S1).
Previous research has indicated that factors such as age, BMI and systemic inflammatory biomarkers are associated with patient responses to immunotherapy. To examine whether these variables are correlated with the prognostic groups, we conducted a comparison among the different prognostic groups using ANOVA. The results demonstrated that the good prognostic group had significantly younger age, higher BMI, and different level of systemic inflammatory biomarkers, including decreased level of PLR, NLR, SIRI, SII and increased level of LMR (P<.0001). Furthermore, as expected, this group also had higher albumin levels and lower dNLR (P<.0001) (Figure 1D). These findings reinforce the role of these factors in predicting patient outcomes following immunotherapy.
Subgroup OS analysis for metastatic patients
Considering that majority of SCLC patients are diagnosed at the extensive stage, we conducted a subgroup analyses of risk factors that could predict OS in individuals with advanced metastatic diseases (ED-SCLC cohort). In this subgroup study, 129 SCLC patients who presented with metastasis were included. Among them, 25 were alive at the end of the study. The median survival for this cohort was 6 months (95% CI: 8–11 months).
For this patient cohort, the results mirrored those of the overall cohort analysis (Table 4), which included patients with and without metastasis. Univariate Cox regression analysis revealed that low albumin levels (p=0.002, HR: 1.92, 95% CI: 1.27–2.91), high dNLR (p=0.005, HR: 1.80, 95% CI: 1.20–2.69), and high N-stage (p=0.046, HR: 0.85, 95% CI: 1.01–3.39), were significantly associated with an increased risk of death. The results were displayed with Kaplan-Meier survival curves as shown in Figure 2.
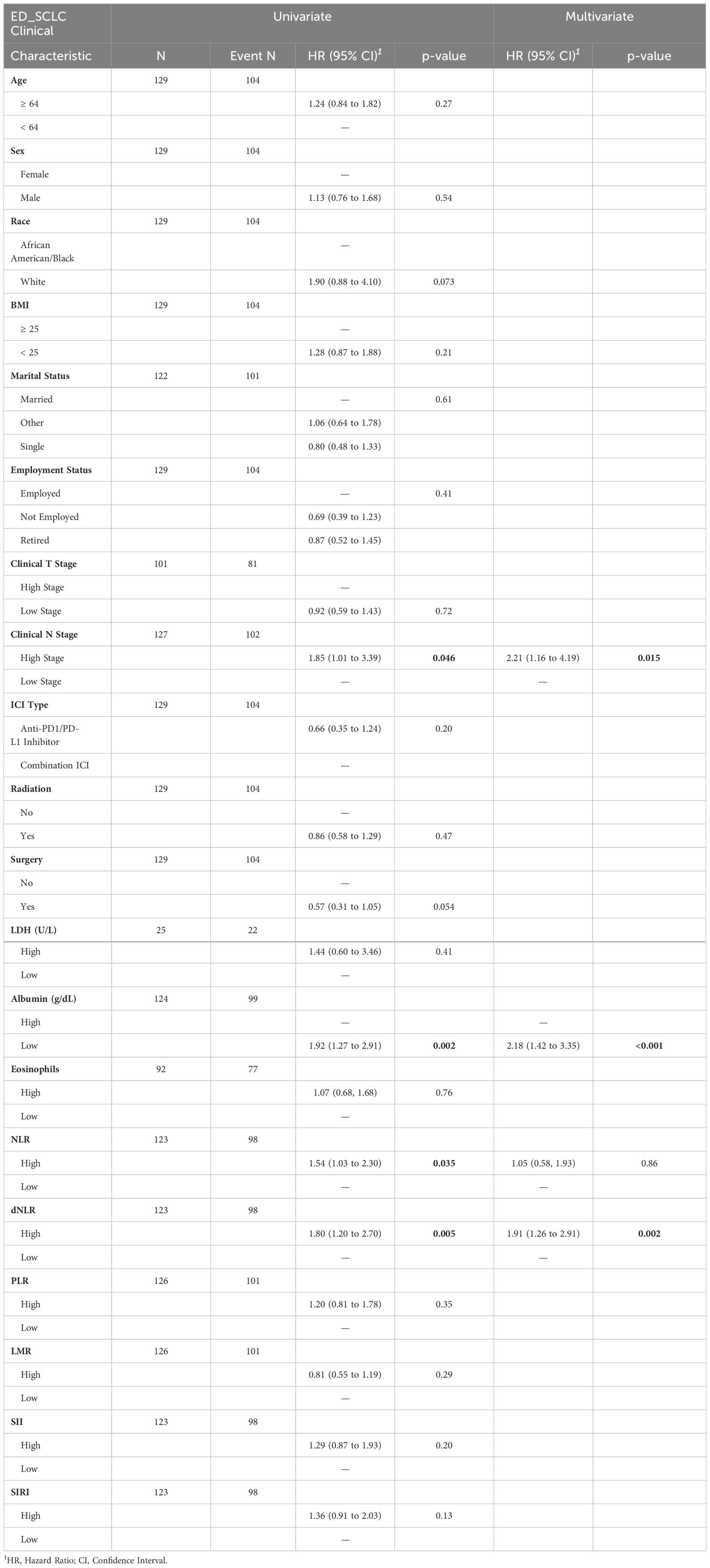
Table 4 Metastatic patient cohort univariate and multivariate Cox proportional hazard regression model results.
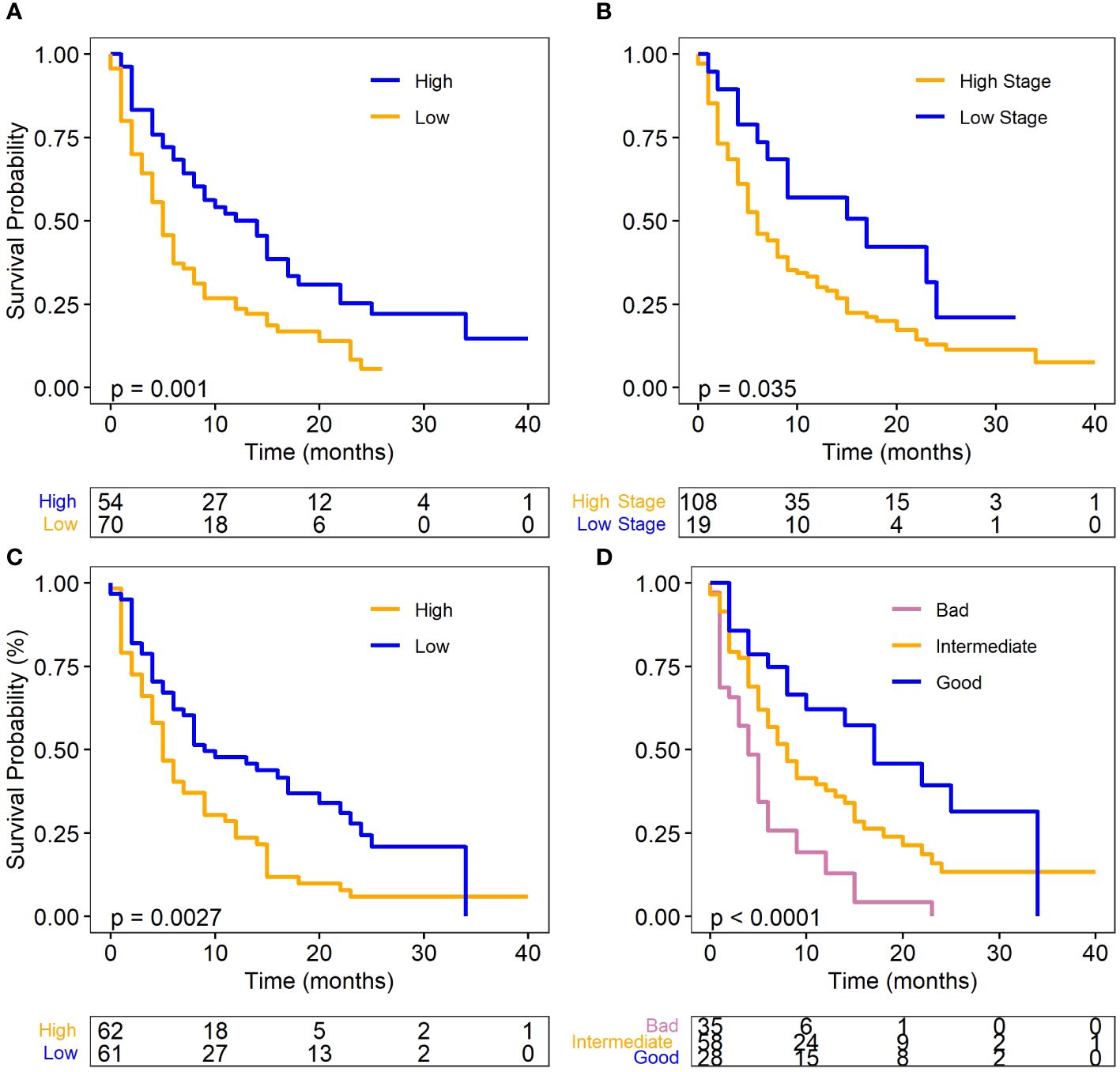
Figure 2 Survival plots for metastatic SCLC (ED-SCLC) cohort. (A) Albumin level and OS. (B) dNLR level and OS. (C) N stage and OS. (D) Different prognostic groups and OS. Log-rank test was used for the analysis for the OS. Albumin and dNLR was dichotomized to high and low according to their median values.
In the multivariate analysis, all three factors were identified as independent predictors of OS in the metastatic cohort (complete data: n=121, with 95 events). A decrease in albumin levels (p=0.0004, HR: 2.18, 95% CI: 1.42–3.35), an increase in N-stage (p=0.015, HR: 2.21, 95% CI: 1.16–4.19), and a higher dNLR (p=0.002, HR: 1.91, 95% CI: 1.26–2.91) were associated with an increased risk of death (Table 4). Moreover, the analysis of imputed data (n=129, with 104 events) also identified these three variables as independent factors predicting OS (Supplementary Data 3).
Similar to the full cohort, the prognostic scoring system also categorized the ED-SCLC patients into “good (n=28),” “intermediate (n=58),” and “bad (n=35)” groups. The median survival was 11, 8, and 4 months for the good, intermediate, and bad group respectively. The good prognostic group had significantly better OS than the bad prognostic group (P<.0001, HR=0.28, 95%CI: 0.15, 0.51) (Figure 2D). These findings further highlight the importance of these biomarkers in predicting SCLC patient outcomes in the context of ICI therapy.
Discussion
In our current study, we performed a comprehensive retrospective analysis on patient baseline risk factors to predict ICI treatment efficacy in SCLC. We placed a specific emphasis on evaluating a series of hematological biomarkers, including LDH, albumin, NLR, dNLR, PLR, LMR, SII, SIRI, as well as individual blood cell types eosinophils, neutrophils, WBC, lymphocytes, monocytes, and platelets on their significance in predicting the effectiveness of ICI treatment in SCLC. It’s noteworthy that such an investigation has not been previously explored in SCLC patients who have undergone ICI involved immunochemotherapy.
Through our study, we first highlighted the significance of the pretreatment blood albumin level as an independent prognostic marker for overall survival in SCLC patients with ICI treatment. The results demonstrated that patients with pretreatment low albumin levels (≤3.7 g/dL) exhibited significantly worse overall survival. This finding holds true not only in the entire SCLC patient cohort (p=0.003, HR 1.72, 95% CI: 1.21–2.45) but also in the subgroup analysis focused on the metastatic patients (p=0.0004, HR: 2.18, 95% CI: 1.42–3.35). Serum albumin has been widely used in assessing patients’ general nutritional health (41) and as a prognostic biomarker for cancer (30, 42, 43). Recently it has emerged as a potential biomarker for predicting the efficacy of ICI therapy (29, 44). In NSCLC, serum albumin has been identified as a potential biomarker for assessing the efficacy of ICI treatment alone or ICI involved immunochemotherapy, either alone or in combination with other markers (29, 40, 45–48). A recent pan-cancer study indicated that in SCLC, patients with elevated albumin levels had improved response and survival outcomes with ICI treatment (22). They determined an optimal cutoff of albumin >3.7 g/dL across different cancer types, and this same cutoff was utilized in our study. With a larger patient cohort, our study has further confirmed the significance of pretreatment serum albumin as a prognostic indicator for predicting the survival outcomes of SCLC patients undergoing ICI involved immunochemotherapy.
A second noteworthy finding in our study is the identification of the derived neutrophil-to-lymphocyte ratio (dNLR) at baseline as another independent risk predictor for the effectiveness of ICI treatment. In contrast to albumin, elevated dNLR level was associated with an increased risk of death, both in the overall SCLC patient cohort (p=0.003, HR 1.71, 95% CI: 1.20–2.44) and in those with ED-SCLC (p=0.002, HR: 1.91, 95% CI: 1.26–2.91). Both NLR and dNLR serve as indicators of systemic inflammation and are widely utilized as prognostic factors in predicting mortality, not only in the general population (28) but also across various disease types (23, 29). This includes a range of solid cancers such as colorectal cancer and melanoma, in both the broader cancer patient population and specifically among those receiving immunotherapy (30–32). Recently, low dNLR was reported to be associated with better outcomes in NSCLC patients who received ICI therapy (28, 29). However, to our best knowledge, our study is the first to demonstrate the critical prognostic significance of dNLR in predicting ICI treatment outcomes in SCLC.
Previous studies have shown that lung immune prognostic index (LIPI), a marker that combines dNLR and LDH, is a prognostic factor of ICI response for NSCLC and other solid tumors (36, 49, 50) as well as for survival outcomes of SCLC patients who received chemotherapy (39). However, due to the great missingness (~ 70%) of LDH values, we could not test the predictive power of LIPI in predicting ICI response in our SCLC cohort. In contrast, we developed a scoring system to evaluate the combined effects of pretreatment serum albumin and dNLR in predicting patient outcomes and identifying specific patient subgroups. For the full patient cohort, patients with a high dNLR and low albumin received a score of 0, and they experienced significantly worse overall survival compared to those with a low dNLR and high albumin who received a score of 2 (P<.0001). The median survival for the group with a favorable prognosis (score=2) was 14.91 months, whereas it was 7.51 months for the group with an unfavorable prognosis (score=0) (HR: 0.33, 95% CI: 0.20–0.55). Notably, the intermediate group, which included patients with either both high or both low albumin and dNLR (score=1), also exhibited significantly better survival than the unfavorable prognosis group (p=0.03, HR: 0.65, 95% CI: 0.44–0.96) but worse than the favorable group (p=0.005, HR: 0.1.98, 95% CI: 1.23–3.17), with a median survival of 10.94 months. To test the robustness of this prognostic scoring system, we did a 3-fold internal cross-validation. The results showed that the scoring system was significantly correlated with patient OS with great separation of the good from the bad prognostic group even with only 33.3% of patient samples (n=56), demonstrating the stability of the findings across different subsets of the data. This scoring system also effectively identified three distinct prognostic groups in patients with ED-SCLC, leading to significantly different survival outcomes (P<.0001). Again, the favorable prognosis group had significantly better survival than the unfavorable group (P<.0001, HR 0.28, 95% CI: 0.15–0.51) with a median survival of 11 months vs. 4 months. Furthermore, the good prognosis group had significantly younger age, higher BMI, and different levels of systemic inflammatory biomarkers, including significantly lower levels of PLR, NLR, SIRI, SII, higher LMR when compared to the unfavorable group. Although the mechanism remains unclear, these systemic inflammatory biomarkers have been reported to be associated with patient survival outcomes and responses to immunotherapy across various cancer types and are now considered as potential prognostic biomarkers (51–54). Our findings confirmed the relevance of these factors with patient outcomes following ICI therapy in the context of SCLC, even though they were not found to be independent factors in predicting patient survival. In summary, the developed scoring system serves as a valuable tool for stratifying SCLC patients into different prognostic categories. This approach has the potential to significantly enhance personalized clinical decision-making and improve the management of SCLC patients, ultimately leading to better patient care and outcomes. However, the validation of this scoring system and comparison with LIPI in predicting ICI response in SCLC still needs to be established in future studies with large independent data.
In addition to our main findings, we also observed that the metastasis status and a patient’s surgical history were significantly associated with the initial response to ICI treatment, but not necessary with patient OS, which is in line with previous findings (27). This is not surprising as patients without metastasis and/or with a history of surgery were likely at an early stage of their disease compared to others and were likely to have better response to the treatment. These factors should be considered in the overall treatment approach for SCLC patients. Due to the limited (n=15) cases as responders based on their first recurrence data, primarily due to the absence of information on partial response and stable disease, it is imperative for further research with a larger sample size to assess the impact of other clinical factors besides metastasis and surgical history on the response to ICI treatment in SCLC patients.
In summary, our study emphasizes the significance of pretreatment serum albumin and dNLR levels as significant potential predictors for patient survival in SCLC patients undergoing ICI therapy. Utilizing the combination of these markers can enhance the identification of patient subgroups most likely to benefit from ICI treatment. This contributes to the potential for more effective and personalized treatment strategies for SCLC patients.
Conclusion
To our knowledge, our study stands as the first to conduct an extensive examination of hematological biomarkers for predicting the effectiveness of ICIs in SCLC. Although our study was limited to the data from a single center and with missing values and variables that are critical for a more complete assessment, our findings highlight the importance of baseline serum albumin level and dNLR in predicting the overall survival of SCLC patients undergoing ICI-involved immunochemotherapy. They also shed light on the potential subgroups that may benefit from ICI therapy. Additionally, our study underscores the importance of factoring in clinical stages and treatment history when predicting initial patient responses. However, the translation of these results into practical guidance for clinical practices should be further evaluated through larger multicenter studies. This step is essential for confirming the utility of these biomarkers in the management of SCLC patients receiving ICI treatment.
Data availability statement
The data analyzed in this study is subject to the following licenses/restrictions: These are internally collected patient electronic medical records based on an approved Institutional Review Board (IRB) protocol IRB 2020C0145). Requests to access these datasets should be directed to the corresponding author (xiaoli.zhang@osumc.edu).
Ethics statement
The studies involving humans were approved by Institutional Review Board (IRB) protocol IRB 2020C0145. The studies were conducted in accordance with the local legislation and institutional requirements. The participants provided their written informed consent to participate in this study.
Author contributions
ZK: Formal analysis, Writing – review & editing, Data curation, Visualization. JM: Formal analysis, Writing – review & editing. XZ: Formal analysis, Writing – review & editing, Conceptualization, Funding acquisition, Investigation, Methodology, Supervision, Validation, Writing – original draft.
Funding
The author(s) declare financial support was received for the research, authorship, and/or publication of this article. This work was supported by the Department of Biomedical Informatics internal funding and Comprehensive Cancer Center Supporting Grant P30CA016058 at The Ohio State University.
Acknowledgments
We thank Drs. Lang Li, Aditi Shendre, and Mohamed Elsaid from the Department of Biomedical Informatics and Dr. Zihai Li from the Pelotonia Institute for Immuno-Oncology (PIIO) at The Ohio State University for collecting and providing the data for this project. Specifically, we want to thank Dr. Mohamed Elsaid for explaining the data
Conflict of interest
The authors declare that the research was conducted in the absence of any commercial or financial relationships that could be construed as a potential conflict of interest.
Publisher’s note
All claims expressed in this article are solely those of the authors and do not necessarily represent those of their affiliated organizations, or those of the publisher, the editors and the reviewers. Any product that may be evaluated in this article, or claim that may be made by its manufacturer, is not guaranteed or endorsed by the publisher.
Supplementary material
The Supplementary Material for this article can be found online at: https://www.frontiersin.org/articles/10.3389/fimmu.2024.1327449/full#supplementary-material
Supplementary Figure 1 | 3-fold cross validation validates the prediction power of the dNLR-Albumin scoring system for patient overall survival displayed with Kaplan-Meier survival curves.
Supplementary Table 1 | Patient Clinical Characteristics.
Supplementary Table 2 | Summary statistics of patient baseline individual hematological cell types for the full cohort and the metastatic subgroup.
Supplementary Table 3 | Logistic regression results showing the association of clinical factors with patient response to ICI therapy in SCLC.
Supplementary Table 4 | Univariate Cox proportional hazard regression testing the association of each individual cell types of hematological biomarkers and SCLC patient OS.
References
1. Nicholson AG, Chansky K, Crowley J, Beyruti R, Kubota K, Turrisi A, et al. The international association for the study of lung cancer lung cancer staging project: proposals for the revision of the clinical and pathologic staging of small cell lung cancer in the forthcoming eighth edition of the TNM classification for lung cancer. J Thorac Oncol. (2016) 11:300–11. doi: 10.1016/j.jtho.2015.10.008
2. Sabari JK, Lok BH, Laird JH, Poirier JT, Rudin CM. Unravelling the biology of SCLC: implications for therapy. Nat Rev Clin Oncol. (2017) 14:549–61. doi: 10.1038/nrclinonc.2017.71
3. Govindan R, Page N, Morgensztern D, Read W, Tierney R, Vlahiotis A, et al. Changing epidemiology of small-cell lung cancer in the United States over the last 30 years: analysis of the surveillance, epidemiologic, and end results database. J Clin Oncol. (2006) 24:4539–44. doi: 10.1200/JCO.2005.04.4859
4. Oronsky B, Reid TR, Oronsky A, Carter CA. What's new in SCLC? A review. Neoplasia. (2017) 19:842–7. doi: 10.1016/j.neo.2017.07.007
5. Lahiri A, Maji A, Potdar PD, Singh N, Parikh P, Bisht B, et al. Lung cancer immunotherapy: progress, pitfalls, and promises. Mol Cancer. (2023) 22:40. doi: 10.1186/s12943-023-01740-y
6. Frederickson AM, Arndorfer S, Zhang I, Lorenzi M, Insinga R, Arunachalam A, et al. Pembrolizumab plus chemotherapy for first-line treatment of metastatic nonsquamous non-small-cell lung cancer: a network meta-analysis. Immunotherapy. (2019) 11:407–28. doi: 10.2217/imt-2018-0193
7. Gandhi L, Rodríguez-Abreu D, Gadgeel S, Esteban E, Felip E, De Angelis F, et al. Pembrolizumab plus chemotherapy in metastatic non-small-cell lung cancer. N Engl J Med. (2018) 378:2078–92. doi: 10.1056/NEJMoa1801005
8. Paz-Ares L, Vicente D, Tafreshi A, Robinson A, Soto Parra H, Mazières J, et al. A randomized, placebo-controlled trial of pembrolizumab plus chemotherapy in patients with metastatic squamous NSCLC: protocol-specified final analysis of KEYNOTE-407. J Thorac Oncol. (2020) 15:1657–69. doi: 10.1016/j.jtho.2020.06.015
9. Soria JC, Tan DSW, Chiari R, Wu Y-L, Paz-Ares L, Wolf J, et al. First-line ceritinib versus platinum-based chemotherapy in advanced ALK-rearranged non-small-cell lung cancer (ASCEND-4): a randomised, open-label, phase 3 study. Lancet. (2017) 389:917–29. doi: 10.1016/S0140-6736(17)30123-X
10. Dingemans AC, Früh M, Ardizzoni A, Besse B, Faivre-Finn C, Hendriks LE, et al. Small-cell lung cancer: ESMO Clinical Practice Guidelines for diagnosis, treatment and follow-up(☆). Ann Oncol. (2021) 32:839–53. doi: 10.1016/j.annonc.2021.03.207
11. Xu Y, Fu Y, Zhu B, Wang J, Zhang B. Predictive biomarkers of immune checkpoint inhibitors-related toxicities. Front Immunol. (2020) 11:2023. doi: 10.3389/fimmu.2020.02023
12. Al-Salama ZT. Durvalumab: A review in extensive-stage SCLC. Target Oncol. (2021) 16:857–64. doi: 10.1007/s11523-021-00843-0
13. Zhang L, Hang Y, Liu M, Li N, Cai H. First-line durvalumab plus platinum-etoposide versus platinum-etoposide for extensive-stage small-cell lung cancer: A cost-effectiveness analysis. Front Oncol. (2020) 10:602185. doi: 10.3389/fonc.2020.602185
14. Liu SV, Reck M, Mansfield AS, Mok T, Scherpereel A, Reinmuth N, et al. Updated overall survival and PD-L1 subgroup analysis of patients with extensive-stage small-cell lung cancer treated with atezolizumab, carboplatin, and etoposide (IMpower133). J Clin Oncol. (2021) 39:619–30. doi: 10.1200/JCO.20.01055
15. Horn L, Mansfield AS, Szczęsna A, Havel L, Krzakowski M, Hochmair MJ, et al. First-line atezolizumab plus chemotherapy in extensive-stage small-cell lung cancer. N Engl J Med. (2018) 379:2220–9. doi: 10.1056/NEJMoa1809064
16. Marabelle A, Le DT, Ascierto PA, Di Giacomo AM, De Jesus-Acosta A, Delord J-P, et al. Efficacy of pembrolizumab in patients with noncolorectal high microsatellite instability/mismatch repair-deficient cancer: results from the phase II KEYNOTE-158 study. J Clin Oncol. (2020) 38:1–10. doi: 10.1200/jco.19.02105
17. Chung HC, Piha-Paul SA, Lopez-Martin J, Schellens JHM, Kao S, Miller WH Jr., et al. Pembrolizumab after two or more lines of previous therapy in patients with recurrent or metastatic SCLC: results from the KEYNOTE-028 and KEYNOTE-158 studies. J Thorac Oncol. (2020) 15:618–27. doi: 10.1016/j.jtho.2019.12.109
18. Owonikoko TK, Park K, Govindan R, Ready N, Reck M, Peters S, et al. Nivolumab and ipilimumab as maintenance therapy in extensive-disease small-cell lung cancer: checkMate 451. J Clin Oncol. (2021) 39:1349–59. doi: 10.1200/JCO.20.02212
19. Poirier JT, George J, Owonikoko TK, Berns A, Brambilla E, Byers LA, et al. New approaches to SCLC therapy: from the laboratory to the clinic. J Thorac Oncol. (2020) 15:520–40. doi: 10.1016/j.jtho.2020.01.016
20. Hellmann MD, Callahan MK, Awad MM, Calvo E, Ascierto PA, Atmaca A, et al. Tumor mutational burden and efficacy of nivolumab monotherapy and in combination with ipilimumab in small-cell lung cancer. Cancer Cell. (2019) 35:329. doi: 10.1016/j.ccell.2019.01.011
21. Yu H, Batenchuk C, Badzio A, Boyle TA, Czapiewski P, Chan DC, et al. PD-L1 expression by two complementary diagnostic assays and mRNA in situ hybridization in small cell lung cancer. J Thorac Oncol. (2017) 12:110–20. doi: 10.1016/j.jtho.2016.09.002
22. Ott PA, Bang Y-J, Piha-Paul SA, Razak ARA, Bennouna J, Soria J-C, et al. T-cell-inflamed gene-expression profile, programmed death ligand 1 expression, and tumor mutational burden predict efficacy in patients treated with pembrolizumab across 20 cancers: KEYNOTE-028. J Clin Oncol. (2019) 37:318–27. doi: 10.1200/JCO.2018.78.2276
23. Safa H, Abu Rous F, Belani N, Borghaei H, Gadgeel S, Halmos B. Emerging biomarkers in immune oncology to guide lung cancer management. Target Oncol. (2023) 18:25–49. doi: 10.1007/s11523-022-00937-3
24. Qiao M, Zhou F, Hou L, Li X, Zhao C, Jiang T, et al. Efficacy of immune-checkpoint inhibitors in advanced non-small cell lung cancer patients with different metastases. Ann Transl Med. (2021) 9:34. doi: 10.21037/atm
25. Sheng L, Gao J, Xu Q, Zhang X, Huang M, Dai X, et al. Selection of optimal first-line immuno-related therapy based on specific pathological characteristics for patients with advanced driver-gene wild-type non-small cell lung cancer: a systematic review and network meta-analysis. Ther Adv Med Oncol. (2021) 13:17588359211018537. doi: 10.1177/17588359211018537
26. Valero C, Lee M, Hoen D, Weiss K, Kelly DW, Adusumilli PS, et al. Pretreatment neutrophil-to-lymphocyte ratio and mutational burden as biomarkers of tumor response to immune checkpoint inhibitors. Nat Commun. (2021) 12:729. doi: 10.1038/s41467-021-20935-9
27. Montoya C, Spieler B, Welford SM, Kwon D, Pra AD, Lopes G, et al. Predicting response to immunotherapy in non-small cell lung cancer- from bench to bedside. Front Oncol. (2023) 13:1225720. doi: 10.3389/fonc.2023.1225720
28. Alessi JV, Ricciuti B, Alden SL, Bertram AA, Lin JJ, Sakhi M, et al. Low peripheral blood derived neutrophil-to-lymphocyte ratio (dNLR) is associated with increased tumor T-cell infiltration and favorable outcomes to first-line pembrolizumab in non-small cell lung cancer. J Immunother Cancer. (2021) 9:e003536. doi: 10.1136/jitc-2021-003536
29. Guo Y, Wei L, Patel SH, Lopez G, Grogan M, Li M, et al. Serum albumin: early prognostic marker of benefit for immune checkpoint inhibitor monotherapy but not chemoimmunotherapy. Clin Lung Cancer. (2022) 23:345–55. doi: 10.1016/j.cllc.2021.12.010
30. Gupta D, Lis CG. Pretreatment serum albumin as a predictor of cancer survival: a systematic review of the epidemiological literature. Nutr J. (2010) 9:69. doi: 10.1186/1475-2891-9-69
31. Jiang M, Peng W, Pu X, Chen B, Li J, Xu F, et al. Peripheral blood biomarkers associated with outcome in non-small cell lung cancer patients treated with nivolumab and durvalumab monotherapy. Front Oncol. (2020) 10:913. doi: 10.3389/fonc.2020.00913
32. Templeton AJ, McNamara MG, Šeruga B, Vera-Badillo FE, Aneja P, Ocaña A, et al. Prognostic role of neutrophil-to-lymphocyte ratio in solid tumors: a systematic review and meta-analysis. J Natl Cancer Inst. (2014) 106:dju124. doi: 10.1093/jnci/dju124
33. Wagner NB, Forschner A, Leiter U, Garbe C, Eigentler TK. S100B and LDH as early prognostic markers for response and overall survival in melanoma patients treated with anti-PD-1 or combined anti-PD-1 plus anti-CTLA-4 antibodies. Br J Cancer. (2018) 119:339–46. doi: 10.1038/s41416-018-0167-x
34. Zhang S, Pease DF, Kulkarni AA, Kumar M, Shanley RM, Xu B, et al. Real-world outcomes and clinical predictors of immune checkpoint inhibitor monotherapy in advanced lung cancer. Clin Med Insights Oncol. (2021) 15:11795549211004489. doi: 10.1177/11795549211004489
35. Aldea M, Benitez JC, Mezquita L. The Lung Immune Prognostic Index (LIPI) stratifies prognostic groups in advanced non-small cell lung cancer (NSCLC) patients. Transl Lung Cancer Res. (2020) 9:967–70. doi: 10.21037/tlcr
36. Mezquita L, Auclin E, Ferrara R, Charrier M, Remon J, Planchard D, et al. Association of the lung immune prognostic index with immune checkpoint inhibitor outcomes in patients with advanced non-small cell lung cancer. JAMA Oncol. (2018) 4:351–7. doi: 10.1001/jamaoncol.2017.4771
37. Li LL, Yu CF, Xie HT, Chen Z, Jia BH, Xie FY, et al. Biomarkers and factors in small cell lung cancer patients treated with immune checkpoint inhibitors: A meta-analysis. Cancer Med. (2023) 12:11211–33. doi: 10.1002/cam4.5800
38. Antonia SJ, López-Martin JA, Bendell J, Ott PA, Taylor M, Eder JP, et al. Nivolumab alone and nivolumab plus ipilimumab in recurrent small-cell lung cancer (CheckMate 032): a multicentre, open-label, phase 1/2 trial. Lancet Oncol. (2016) 17:883–95. doi: 10.1016/S1470-2045(16)30098-5
39. Sonehara K, Tateishi K, Komatsu M, Yamamoto H, Hanaoka M. Lung immune prognostic index as a prognostic factor in patients with small cell lung cancer. Thorac Cancer. (2020) 11:1578–86. doi: 10.1111/1759-7714.13432
40. Wu Y, Wu H, Lin M, Liu T, Li J. Factors associated with immunotherapy respond and survival in advanced non-small cell lung cancer patients. Transl Oncol. (2022) 15:101268. doi: 10.1016/j.tranon.2021.101268
41. Bharadwaj S, Ginoya S, Tandon P, Gohel TD, Guirguis J, Vallabh H, et al. Malnutrition: laboratory markers vs nutritional assessment. Gastroenterol Rep (Oxf). (2016) 4:272–80. doi: 10.1093/gastro/gow013
42. Fiala O, Pesek M, Finek J, Racek J, Minarik M, Benesova L, et al. Serum albumin is a strong predictor of survival in patients with advanced-stage non-small cell lung cancer treated with erlotinib. Neoplasma. (2016) 63:471–6. doi: 10.4149/318_151001N512
43. Zhou T, Zhan J, Hong S, Hu Z, Fang W, Qin T, et al. Ratio of C-reactive protein/albumin is an inflammatory prognostic score for predicting overall survival of patients with small-cell lung cancer. Sci Rep. (2015) 5:10481. doi: 10.1038/srep10481
44. Yoo SK, Chowell D, Valero C, Morris LGT, Chan TA. Pre-treatment serum albumin and mutational burden as biomarkers of response to immune checkpoint blockade. NPJ Precis Oncol. (2022) 6:23. doi: 10.1038/s41698-022-00267-7
45. Johannet P, Sawyers A, Qian Y, Kozloff S, Gulati N, Donnelly D, et al. Baseline prognostic nutritional index and changes in pretreatment body mass index associate with immunotherapy response in patients with advanced cancer. J Immunother Cancer. (2020) 8:e001674. doi: 10.1136/jitc-2020-001674
46. Roch B, Coffy A, Jean-Baptiste S, Palaysi E, Daures JP, Pujol JL, et al. Cachexia - sarcopenia as a determinant of disease control rate and survival in non-small lung cancer patients receiving immune-checkpoint inhibitors. Lung Cancer. (2020) 143:19–26. doi: 10.1016/j.lungcan.2020.03.003
47. Guven DC, Sahin TK, Erul E, Rizzo A, Ricci AD, Aksoy S, et al. The association between albumin levels and survival in patients treated with immune checkpoint inhibitors: A systematic review and meta-analysis. Front Mol Biosci. (2022) 9:1039121. doi: 10.3389/fmolb.2022.1039121
48. Zhang CL, Gao M-Q, Jiang X-C, Pan X, Zhang X-Y, Li Y, et al. Research progress and value of albumin-related inflammatory markers in the prognosis of non-small cell lung cancer: a review of clinical evidence. Ann Med. (2023) 55:1294–307. doi: 10.1080/07853890.2023.2192047
49. Meyers DE, Stukalin I, Vallerand IA, Lewinson RT, Suo A, Dean M, et al. The lung immune prognostic index discriminates survival outcomes in patients with solid tumors treated with immune checkpoint inhibitors. Cancers (Basel). (2019) 11:1713. doi: 10.3390/cancers11111713
50. Riudavets M, Auclin E, Mosteiro M, Dempsey N, Majem M, Prelaj A, et al. Association between lung immune prognostic index and durvalumab consolidation outcomes in patients with locally advanced non-small-cell lung cancer. Clin Lung Cancer. (2023) 25:233–43.E8. doi: 10.1016/j.cllc.2023.11.007
51. Bai R, Lv Z, Xu D, Cui J. Predictive biomarkers for cancer immunotherapy with immune checkpoint inhibitors. Biomark Res. (2020) 8:34. doi: 10.1186/s40364-020-00209-0
52. Brueckl WM, Ficker JH, Zeitler G. Clinically relevant prognostic and predictive markers for immune-checkpoint-inhibitor (ICI) therapy in non-small cell lung cancer (NSCLC). BMC Cancer. (2020) 20:1185. doi: 10.1186/s12885-020-07690-8
53. Chowell D, Yoo S-K, Valero C, Pastore A, Krishna C, Lee M, et al. Improved prediction of immune checkpoint blockade efficacy across multiple cancer types. Nat Biotechnol. (2022) 40:499–506. doi: 10.1038/s41587-021-01070-8
Keywords: SCLC, immune checkpoint inhibitors, albumin, derived neutrophil to lymphocyte ratio, response, overall survival, prognostic scoring system
Citation: Kuang Z, Miao J and Zhang X (2024) Serum albumin and derived neutrophil-to-lymphocyte ratio are potential predictive biomarkers for immune checkpoint inhibitors in small cell lung cancer. Front. Immunol. 15:1327449. doi: 10.3389/fimmu.2024.1327449
Received: 25 October 2023; Accepted: 21 May 2024;
Published: 07 June 2024.
Edited by:
Michael R. Shurin, University of Pittsburgh Medical Center, United StatesReviewed by:
Hisakazu Yamagishi, Kyoto Prefectural University, JapanIvaylo Mihaylov, University of Miami, United States
Copyright © 2024 Kuang, Miao and Zhang. This is an open-access article distributed under the terms of the Creative Commons Attribution License (CC BY). The use, distribution or reproduction in other forums is permitted, provided the original author(s) and the copyright owner(s) are credited and that the original publication in this journal is cited, in accordance with accepted academic practice. No use, distribution or reproduction is permitted which does not comply with these terms.
*Correspondence: Xiaoli Zhang, Xiaoli.zhang@osumc.edu