- 1First Clinical College, Shandong University of Traditional Chinese Medicine, Jinan, Shandong, China
- 2Department of Oncology, Affiliated Hospital of Shandong University of Traditional Chinese Medicine, Jinan, Shandong, China
- 3Department of Oncology, Longhua Hospital Affiliated to Shanghai University of Traditional Chinese Medicine, Shanghai, China
Introduction: Cancer is a major global health concern, and immune checkpoint inhibitors (ICIs) offer a promising treatment option for cancer patients. However, the efficacy of ICIs can be influenced by various factors, including the use of concomitant medications.
Methods: We searched databases (PubMed, Embase, Cochrane Library, Web of Science) for systematic reviews and meta-analyses for systematic reviews and meta-analyses on the impact of concomitant medications on ICIs efficacy, published from inception to January 1, 2023. We evaluated the methodological quality of the included meta-analyses, and re-synthesized data using a random-effects model and evidence stratification.
Results: We included 23 publications, comprising 11 concomitant medications and 112 associations. Class II-IV evidence suggested that antibiotics have a negative impact on ICIs efficacy. However, ICIs efficacy against melanoma, hepatocellular carcinoma, and esophageal squamous cell carcinoma was not affected, this effect was related to the exposure window (class IV). Class III evidence suggested that proton pump inhibitors have a negative impact on ICIs efficacy; nevertheless, the efficacy against melanoma and renal cell carcinoma was not affected, and the effect was related to exposure before the initiation of ICIs therapy (class II). Although class II/III evidence suggested that steroids have a negative impact, this effect was not observed when used for non-cancer indications and immune-related adverse events (class IV). Class IV evidence suggested that opioids reduce ICIs efficacy, whereas statins and probiotics may improve ICIs efficacy. ICIs efficacy was not affected by histamine 2 receptor antagonists, aspirin, metformin, β-blockers, and nonsteroidal anti-inflammatory agents.
Conclusion: Current evidence suggests that the use of antibiotics, PPIs, steroids, and opioids has a negative impact on the efficacy of ICIs. However, this effect may vary depending on the type of tumor, the timing of exposure, and the intended application. Weak evidence suggests that statins and probiotics may enhance the efficacy of ICIs. Aspirin, metformin, β-blockers, and NSAIDs do not appear to affect the efficacy of ICIs. However, caution is advised in interpreting these results due to methodological limitations.
Systematic review registration: https://www.crd.york.ac.uk/PROSPERO,identifier, CRD42022328681.
1 Introduction
Cancer poses a major threat to human health, with increasing incidence and mortality rates imposing a heavy burden on societies worldwide (1). Immune checkpoint inhibitors (ICIs) have emerged as an important treatment option, bringing new hope to patients (2). However, the efficacy of ICIs is influenced by various factors (3), including the use of concomitant medications (4).
Antibiotics, proton pump inhibitors (PPIs), and steroids are widely used in clinical practice. Multiple clinical studies and meta-analyses have suggested that these concomitant medications may significantly reduce the efficacy of ICIs (5, 6). Nevertheless, other studies did not demonstrate this negative impact (7). Two almost simultaneously published meta-analyses investigating the effect of PPIs on ICIs efficacy yielded inconsistent results (8, 9). Additionally, a meta-analysis showed that the use of antibiotics during treatment with ICIs does not shorten progression-free survival (PFS), and may even prolong it (10). Moreover, the negative effect of antibiotics may also be related to the time window of use and the type of tumor (11), thereby complicating clinical decision-making.
Notably, besides the negative impact on ICIs efficacy, some concomitant medications (e.g., statins, metformin, β-blockers, and probiotics) may exert an enhancing effect on ICIs efficacy; however, the level of evidence remains poor and controversial (12). In recent years, the number of meta-analyses focusing on the impact of concomitant medications has surged. However, the quality of these analyses is uneven, and the results are inconsistent. Moreover, most meta-analyses only analyzed and evaluated a single type of concomitant medications, thus lacking comprehensiveness and systematicity. Therefore, it is necessary to comprehensively review and summarize published systematic reviews and meta-analyses, evaluate publication bias and evidence quality, explore the effects of different concomitant medications on ICIs efficacy, and present an overview of the available evidence for clinical application.
The objectives of this review were to: 1) comprehensively analyze and summarize the existing systematic reviews and meta-analyses; 2) use a random-effects model to re-synthesize the data; 3) evaluate publication bias and evidence quality; and 4) present an overview of the available evidence regarding the impact of concomitant medications on ICIs efficacy.
2 Methods
The protocol for this study has been submitted to and registered in PROSPERO (Registration number: CRD42022328681). This umbrella review was conducted in accordance with the PRISMA statement (13).
2.1 Search strategy
We used a pre-designed strategy to conduct a comprehensive and systematic search in the PubMed, Embase, Cochrane Library, and Web of Science databases from inception to January 1, 2023. The search was limited to articles published in English. In brief, the search terms included “concomitant medications”, “ICIs”, “systematic reviews”, and “meta-analyses”. Detailed search strategies and the results obtained from PubMed are presented in Table S1.
2.2 Inclusion and exclusion criteria
The detailed inclusion criteria were as follows: (1) study design was a systematic review or meta-analysis; (2) study population included patients with cancer receiving ICIs, with the observation and control groups receiving concomitant medications and no concomitant medications, respectively; (3) concomitant medications included antibiotics, steroids, PPIs, anticoagulants, lipid-lowering agents, antihypertensive agents, antidiabetic agents, probiotics, and analgesics; and (4) the study reported at least one outcome measure, namely overall survival (OS), PFS, objective response rate (ORR), progressive disease (PD), stable disease, complete response, partial response, and disease control rate.
Publications were excluded based on the following criteria: (1) animal experiments; (2) individual case reports; (3) network meta-analyses; (4) original clinical trials; (5) case reports; and (6) articles not published in English.
2.3 Literature screening and data extraction
Two reviewers (HLL and LZ) independently conducted literature screening based on the inclusion and exclusion criteria. After reading the title and abstract, full-text manuscripts were obtained for further evaluation. Any discrepancies were resolved through discussion with a third reviewer (HJL), reaching a consensus.
One reviewer (HLL) performed data extraction, and another reviewer (LZ) checked the data. The extracted data included the first author, year of publication, number of included studies, number of included patients, age, sex distribution, tumor type, co-medications type, follow-up duration, outcome measures, pooled effect size and 95% confidence interval (CI), quality assessment tool, conflict of interest, and funding information. For original studies included in the systematic review and meta-analysis, the extracted information included the first author, year of publication, sample size, effect size, and 95% CI. In addition, we also screened the reference lists of included studies to ensure that all available publications were included in the analysis.
2.4 Quality assessment
Two reviewers (HLL, LZ) independently conducted methodological quality assessments using Assessment of Multiple Systematic Reviews (AMSTAR) 2 (14), which evaluates 16 items sequentially (Table S2). Items 2, 4, 7, 9, 11, 13, and 15 are the critical domains. High quality is attributed to instances with no or just one non-critical weakness. Moderate quality applies to scenarios with multiple non-critical defects. Instances with one critical flaw, with or without non-critical weaknesses, are deemed of low quality. Critically low quality is assigned to situations featuring more than one critical flaw, with or without non-critical weaknesses. Any discrepancies were resolved through discussion with a third reviewer (HJL), reaching a consensus.
2.5 Removal of overlapping meta-analyses
In recent years, numerous meta-analyses on this topic have emerged. However, overlapping original studies for the same outcome measures may lead to bias. Therefore, we addressed this issue in our analysis. As in previously published umbrella reviews (15), we used the citation matrix and corrected covered area (CCA) to calculate the degree of overlap (16). If the CCA was >15%, we retained the most recent publication with the highest number of included studies and level of methodological quality. If the CCA was <15%, we retained both; however, in case of complete overlap, we retained the publication with the highest number of included studies.
2.6 Evidence grading
As previously described in an umbrella review (17), we categorized the evidence into five classes which are described below. Convincing evidence (class I) was characterized by a significant combined effect size (p < 10−6), significant effect size in the largest study (p < 0.05), low heterogeneity (I2 < 50%), 95% prediction interval (PI) that did not include the null value, no evidence of significant publication bias (p > 0.1) as indicated by Egger’s regression test, and >1,000 patients included in the meta-analysis. Highly suggestive evidence (class II) was characterized by a significant combined effect size (p < 10−6), significant effect size in the largest study (p < 0.05), and >1,000 patients included in the meta-analysis, but did not meet class I criteria. Suggestive evidence (class III) was characterized by a significant combined effect size (p < 10−3) and >1,000 patients included in the meta-analysis, but did not meet class I or II criteria. Weak evidence (class IV) was characterized by a significant combined effect size (p < 0.05), but did not meet class I–III criteria. Non-significant evidence (class ns) was characterized by no significant combined effect size (p > 0.05).
2.7 Statistical analysis
The DerSimonian–Laird (DL) method can underestimate the 95% CI when the number of included studies is small (18, 19). Therefore, we used the Hartung–Knapp–Sidik–Jonkman (HKSJ) method to analyze meta-data for fewer than five individual studies, and the DL method for more than five studies (20). Heterogeneity was assessed by calculating the I2 and 95% PI. An I2 >50% indicated significant heterogeneity, and the 95% PI predicted the potential range of true effects in the future.
For the assessment of publication bias, we first conducted Egger’s regression test. Subsequently, we evaluated all meta-analyses using contour-enhanced funnel plots. As in prior umbrella reviews (17, 21, 22), we assumed that small-study effects were present when Egger’s test yielded p-values <0.1. Therefore, we used the “trim-and-fill” method to re-estimate the effect size and 95% CI, thereby mitigating the potential impact of publication bias on the true results.
Furthermore, we conducted a test of excess significance to evaluate whether the number of observations of statistically significant results is greater than its expected number. In this test, p-values <0.1 indicated statistical significance (22). Additionally, we performed sensitivity analyses on meta-analyses with evidence grades I–III. This was achieved by excluding individual original studies with total sample sizes <100, re-synthesizing the data, and re-grading the evidence to test the robustness of the results and mitigate the bias introduced by studies with small samples. All data analyses were conducted using R software (version 4.1.1) using the ‘metaumbrella’ R package (23, 24), and p-values were two-tailed.
3 Results
3.1 Literature selection
As shown in Figure 1, we obtained a total of 1,057 publications from four electronic databases and reference lists. After removing duplicates, 631 publications were selected for preliminary screening based on their titles and abstracts. Subsequently, 545 and 42 publications were excluded by the two reviewers after title/abstract and full-text reading, respectively. Table S3 lists the excluded publications and exclusion criteria. We further removed overlapping studies by calculating the CCA (Table S4), resulting in a final set of 23 publications.
3.2 Basic characteristics
Table 1 summarizes the basic characteristics of the 23 included publications (10–12, 25–44). These publications were from China (n=19), the United States of America (n=2), Australia (n=1), and France (n=1).
The 112 associations of antibiotics (n = 40), PPIs (n = 23), histamine 2 receptor antagonists (H2RAs; n = 3), steroids (n = 21), statins (n = 4), aspirin (n = 2), metformin (n = 2), β-blockers (n = 2), probiotics (n = 9), nonsteroidal anti-inflammatory agents (NSAIDs; n = 3) and opioids (n=3) are presented in Table S5. The number of original studies included ranged 5–45; the median age of the patients ranged 52–75 years. In addition, we compiled a list of 110 associations (antibiotics [n =69], PPIs [n = 28], steroids [n = 2], β-blockers [n =4], NSAIDs [n = 2], opioids [n = 2], and probiotics [n = 3]) that were excluded due to overlap.
3.3 Quality assessment
Figure S1 shows the methodological quality assessment of the final 23 included publications and the 21 excluded publications due to overlap. Two articles on PPIs/H2RAs and one article on antibiotics were identified as low-quality publications due to the lack of a list of excluded literature. The remaining 41 publications were deemed to be of critically low quality, 27 (66%) were not registered with a protocol before conducting the meta-analysis, 35 (85%) did not discuss the sources of bias risk in detail, and 11 (27%) did not include tests and analyses on publication bias.
3.4 Overlapping associations
In the overlapping associations, 26 (24%) yielded inconsistent results with the included data. Regarding exposure to PPIs, 13 associations yielded inconsistent findings. Of note, two meta-analyses showed convincing evidence (class I) that PPIs reduced OS and PFS in any exposure window. Nevertheless, they were excluded due to the small number of included studies and overlapping. Regarding exposure to antibiotics, 13 associations yielded inconsistent results, mainly focusing on the initiation of ICIs therapy and RCC. Further details are provided in Table S6 and Figures S2, S3, S4.
3.5 Antibiotics
Figure 2 shows the effects of different exposure windows to antibiotics on the prognosis of different types of cancer and the level of evidence. Overall, based on all exposure windows and without distinguishing cancer types, suggestive evidence (class III) indicated that exposure to antibiotics reduced the OS of patients; highly suggestive evidence (class II) indicated reduced PFS; and weak evidence (class IV) showed reduced ORR and response rate. For non-small cell lung cancer (NSCLC), suggestive evidence (class III) showed that antibiotics reduced OS, and weak evidence (class IV) showed reduced PFS and ORR. For renal cell carcinoma (RCC), weak evidence (class IV) showed that antibiotics reduced OS, PFS, and ORR, but did not affect PD. For urothelial carcinoma (UC), weak evidence (class IV) showed that antibiotics reduced OS, but did not affect PFS. The prognosis of melanoma, hepatocellular carcinoma (HCC), and esophageal squamous cell carcinoma was not affected by antibiotics.
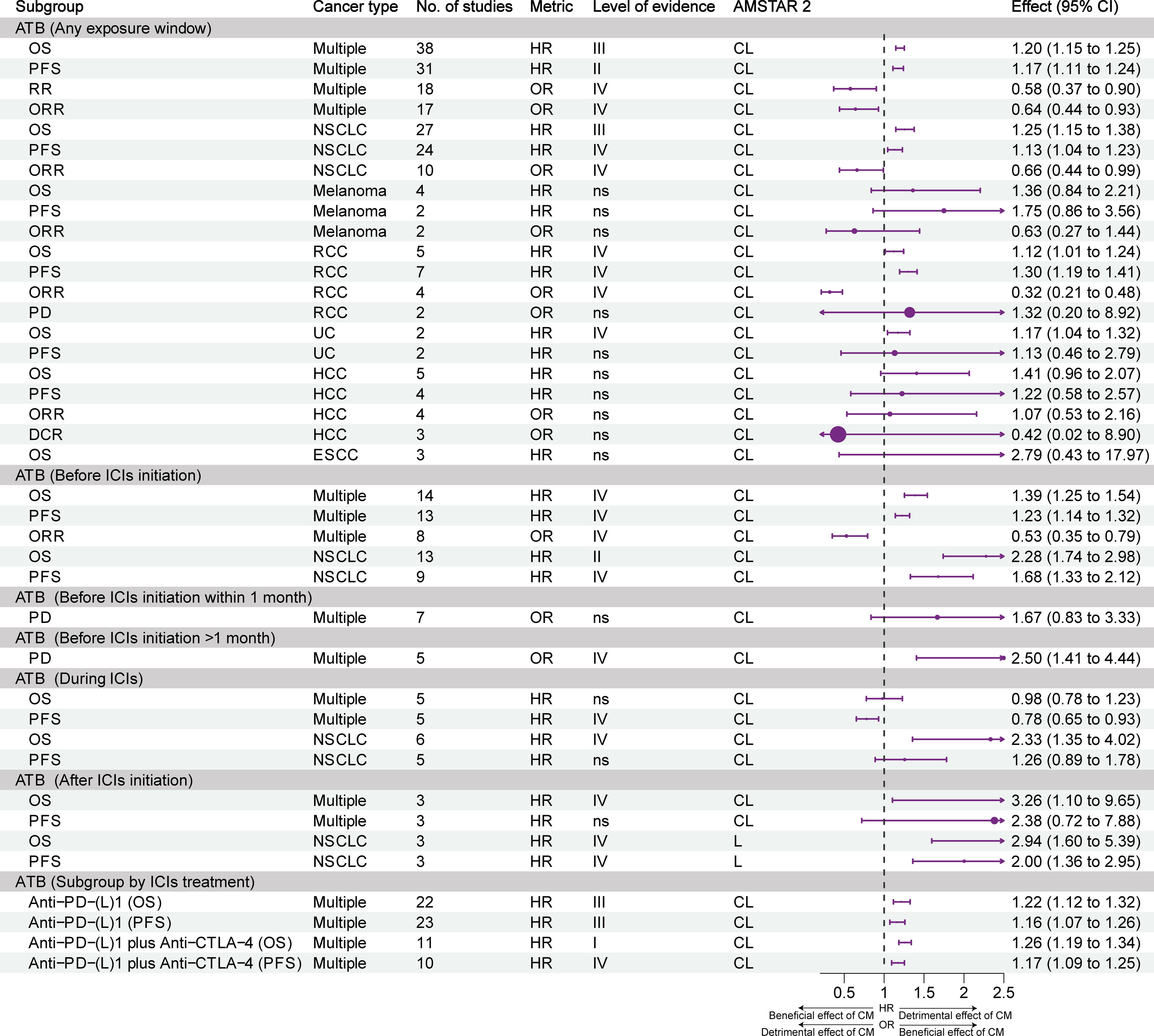
Figure 2 Forest plot of the prognosis of patients with cancer patients receiving ICIs and antibiotics, and subgroup analysis by cancer type and exposure window. ATB, antibiotic; CI, confidence interval; CL, critically low; DCR, disease control rate; ESCC, esophageal squamous cell carcinoma; HCC, hepatocellular carcinoma; HR, hazard ratio; ICIs, immune checkpoint inhibitors; L, low; NSCLC, non-small cell lung cancer; OR, odds ratio; ORR, objective response rate; OS, overall survival; PD, progressive disease; PFS, progression-free survival; RCC, renal cell carcinoma; UC, urothelial carcinoma; CM, concomitant medications.
The negative effects of exposure to antibiotics before the initiation of ICIs were evident. Without distinguishing tumor types, weak evidence (class IV) indicated that antibiotics reduced OS, PFS, and ORR. Highly suggestive evidence (class II) and weak evidence (class IV) showed that antibiotics reduced the OS and PFS of patients with NSCLC. Weak evidence (class IV) suggested that the use of antibiotics within 1 month before the initiation of ICIs promoted PD in patients with cancer, while the use of antibiotics >1 month prior to the initiation of ICIs did not affect PD.
Regarding exposure to antibiotics during ICIs therapy, weak evidence (class IV) showed that antibiotics prolonged the PFS of patients with cancer, but did not exert an effect on OS. When NSCLC was analyzed separately, weak evidence (class IV) showed that antibiotics shortened OS, but did not affect PFS.
Concerning exposure to antibiotics after the initiation of treatment with ICIs, weak evidence (class IV) showed that antibiotics reduced the OS of patients with cancer, but did not exert an effect on PFS. Weak evidence (class IV) showed that antibiotics reduced the OS and PFS of patients with NSCLC.
Subgroup analysis of ICIs treatment showed that antibiotics reduced OS and PFS, whether with PD-(L)1 inhibitors alone (suggestive evidence, class II) or in combination with CTLA-4 inhibitors (Convincing and weak evidence, class I and IV).
3.6 PPIs/H2RAs
Figure 3 illustrates the effect of different exposure windows to PPIs/H2RAs on the prognosis of different types of tumors and the stratification of evidence. Overall, based on all exposure windows, suggestive evidence (class III) showed that the use of PPIs reduced OS and PFS in multiple types of cancer; however, it did not affect the ORR. Suggestive evidence (class III) showed that PPIs reduced OS and PFS in NSCLC and UC; nevertheless, the OS and PFS of patients with melanoma and RCC were not affected. Highly suggestive evidence (class II) showed that the OS and PFS of patients with cancer were reduced in the subgroup that used PPIs within 60 days before the initiation of ICIs therapy. However, these effects were not observed in the subgroup that used PPIs after the initiation of treatment with ICIs. Exposure to H2RAs did not affect the efficacy of ICIs. Subgroup analysis of ICIs treatment showed that PPIs reduced OS and PFS in combination with PD-1 or PD-L1 inhibitors alone (weak evidence, class IV), but not CTLA-4 inhibitors alone.
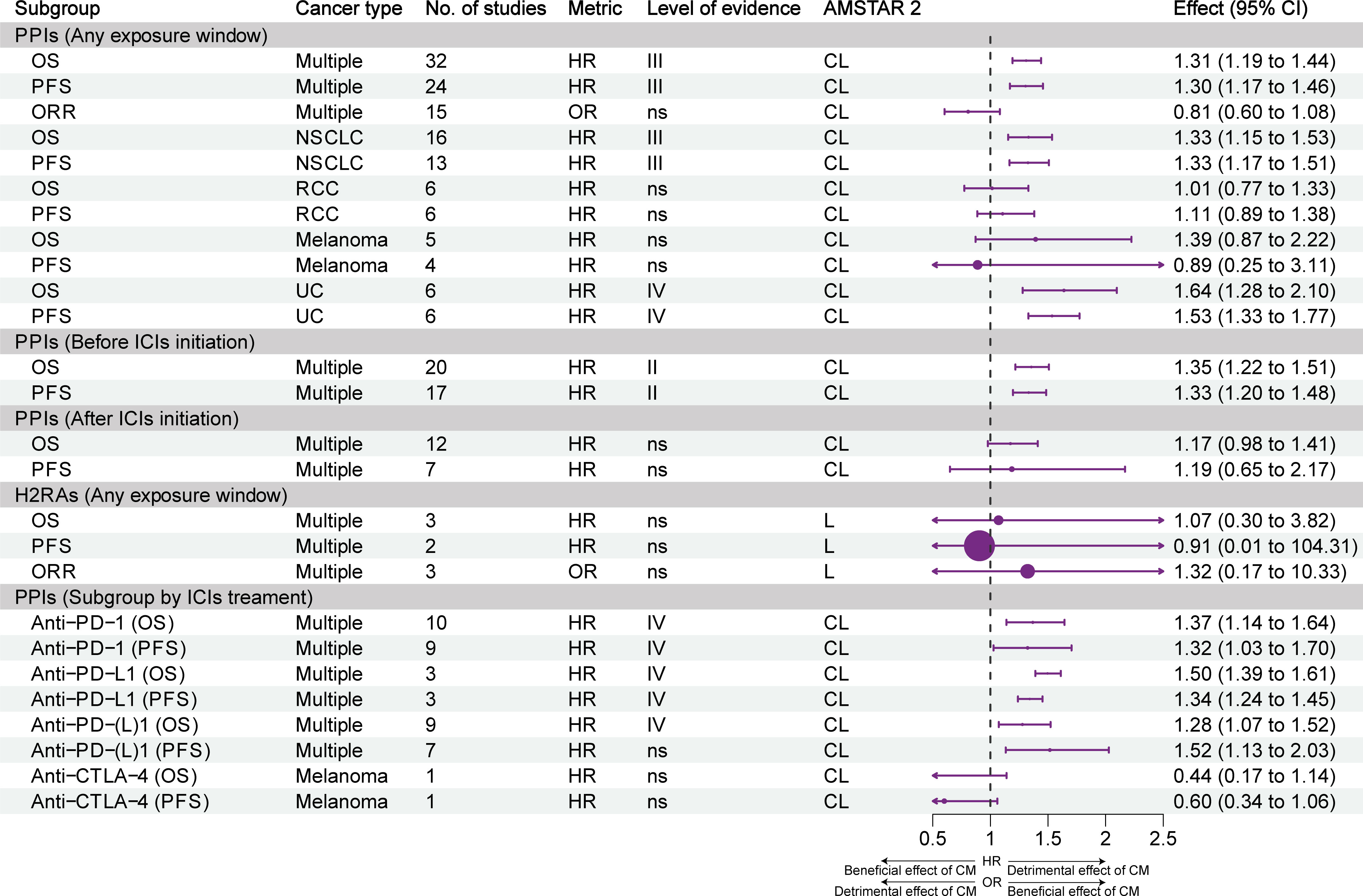
Figure 3 Forest plot of the prognosis of patients with cancer receiving ICIs and PPIs, and subgroup analysis by cancer type and exposure window. CI, confidence interval; CL, critically low; H2RAs, histamine 2 receptor antagonists; HR, hazard ratio; ICIs, immune checkpoint inhibitors; L, low; NSCLC, non-small cell lung cancer; OR, odds ratio; ORR, objective response rate; OS, overall survival; PFS, progression-free survival; PPI, proton pump inhibitor; RCC, renal cell carcinoma; UC, urothelial carcinoma; CM, concomitant medications.
3.7 Steroids
Figure 4 presents the effect of exposure to steroids on the prognosis of different types of tumors and the stratification of evidence. Overall, highly suggestive evidence (class II) and suggestive evidence (class III) showed that the use of steroids reduced OS and PFS in patients with cancer. Highly suggestive evidence (class II) and weak evidence (class IV) showed that steroids reduced the OS and PFS of patients with NSCLC. For cancer patients with brain metastasis, weak evidence (class IV) showed that steroids reduced OS and PFS, but not intracranial PFS. Moreover, steroids were associated with a reduction of OS in patients with brain metastasis who did not undergo stereotactic radiosurgery (SRS); however, the OS of patients who underwent SRS was not affected. Weak evidence (class IV) showed that steroids were associated with a reduction of OS in patients with melanoma but not in NSCLC patients with brain metastasis. Highly suggestive evidence (class II) and suggestive evidence (class III) showed that the use of steroids for cancer indications reduced the OS and PFS of patients. Nonetheless, the use of steroids for non-cancer indications and immune-related adverse events (irAEs) did not result in such effects. Subgroup analysis of ICIs treatment showed that steroids reduced OS and PFS in combination with PD-(L)1 inhibitors alone (highly suggestive and suggestive evidence, class II and III), reduced OS but not PFS in combination with CTLA-4 inhibitors alone (weak evidence, class IV).
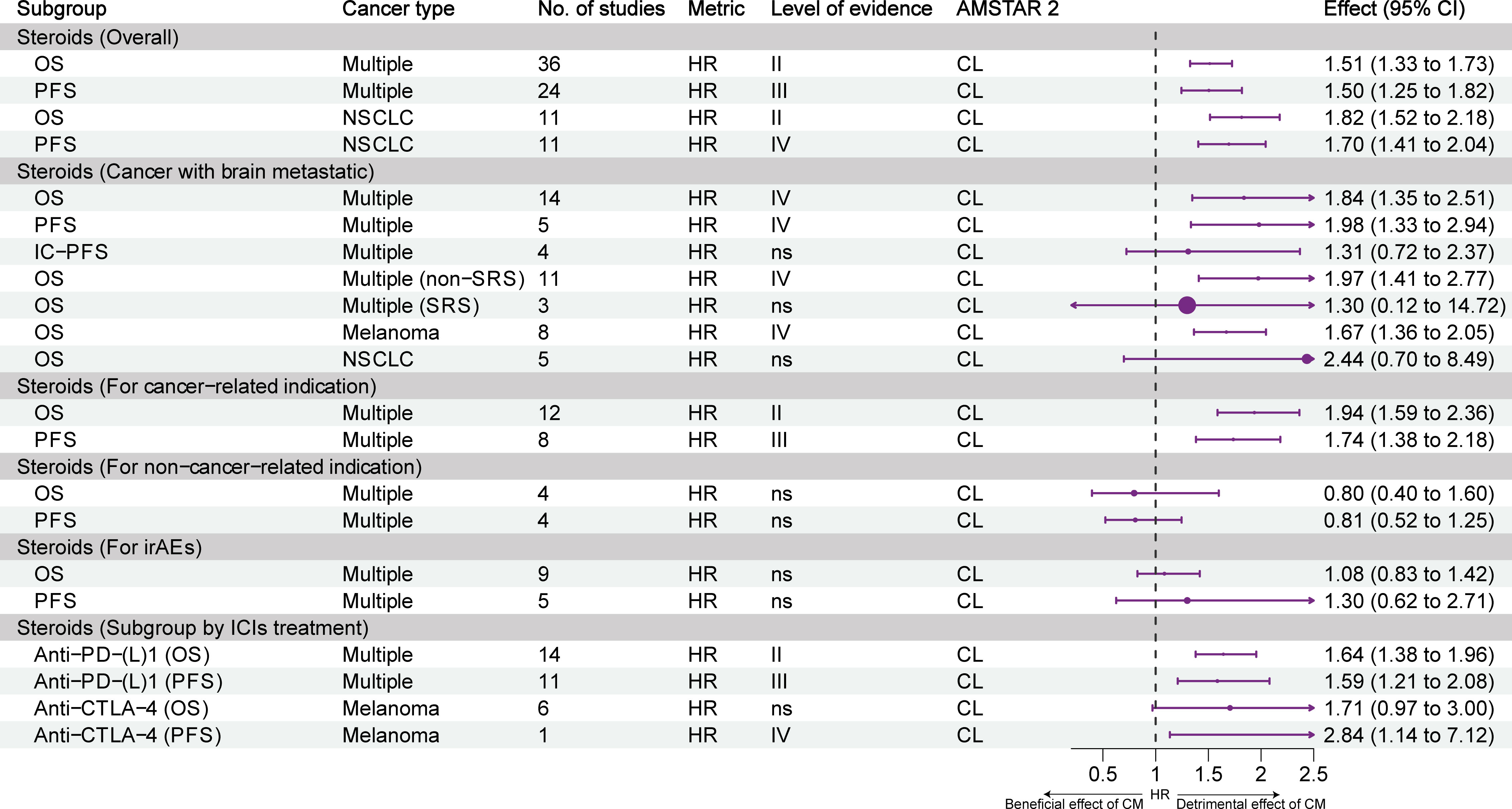
Figure 4 Forest plot of the prognosis of patients with cancer receiving ICIs and steroids, and subgroup analysis. CL, critically low; HR, hazard ratio; IC-PFS, intracranial progression-free survival; irAEs, immune-related adverse events; L, low; NSCLC, non-small cell lung cancer; OS, overall survival; PFS, progression-free survival; SRS, stereotactic radiosurgery; CM, concomitant medications.
3.8 Other concomitant medications
Figure 5 illustrates the impact and evidence grading of exposure to statins, aspirin, metformin, β-blockers, probiotics, opioids, and NSAIDs on the prognosis of different types of tumors. Weak evidence (class IV) suggested that statin use was associated with prolonged OS, but not PFS, in patients with cancer. Although statin use was linked to a trend for improvement in OS and PFS in NSCLC, the effect was not statistically significant. Weak evidence (class IV) indicated that probiotics increased the ORR, OS, and PFS of patients with NSCLC; however, they did not have an impact on the OS and ORR of patients with RCC. Although the use of probiotics was associated with a trend for improvement in OS and PFS in multiple types of cancer, the effect was not statistically significant. Weak evidence (class IV) suggested that the use of opioids was associated with a decrease in OS, PFS, and ORR. The use of aspirin, metformin, β-blockers, and NSAIDs did not have an impact on the efficacy of ICIs.
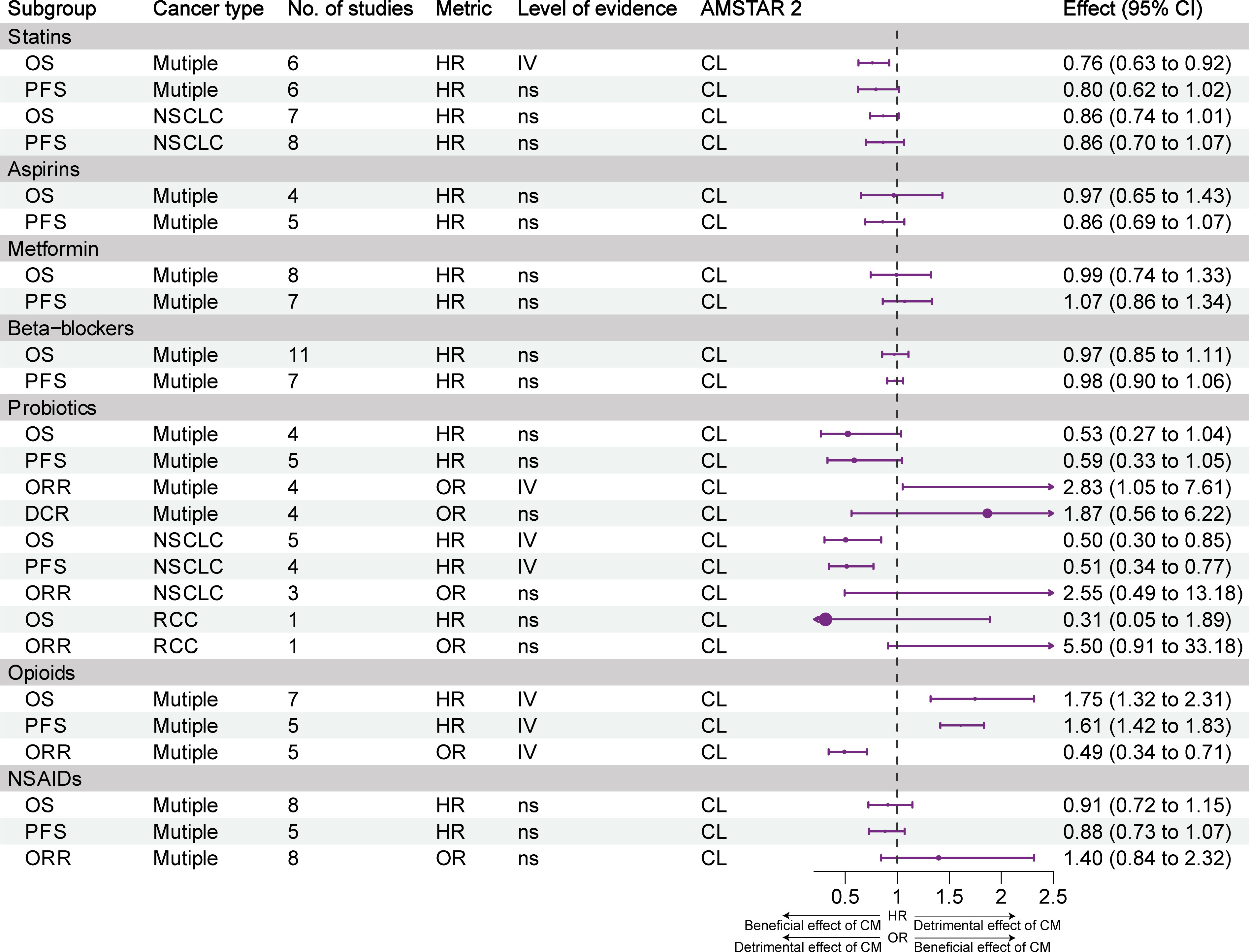
Figure 5 Forest plot of the prognosis of patients with cancer receiving ICIs and other concomitant medications (statins, aspirins, metformin, β-blockers, probiotics, opioids, NSAIDs), and subgroup analysis. CL, critically low; HR, hazard ratio; L, low; NSAIDs, nonsteroidal anti-inflammatory agents; NSCLC, non-small cell lung cancer; OR, odds ratio; OS, overall survival; PFS, progression-free survival; RCC, renal cell carcinoma; CM, concomitant medications. Supplementary Figure and table legends.
3.9 Publication bias
Figures S5, S6, S7, S9, S10 show an enhanced funnel plot of the meta-analyses that included more than three original studies. According to the results of the Egger’s regression test, 24 associations were characterized by a small sample effect (Table S7). The subsequent analysis using the ‘trim-and-fill’ method showed that the results of four associations lost statistical significance after adding studies. These included the effect of exposure to PPIs on OS in NSCLC, the effect of exposure to antibiotics on OS in multiple cancer types, the effect of exposure to antibiotics before the initiation of ICIs therapy on OS in NSCLC, and the effect of exposure to antibiotics on OS in RCC. The results of a associations gained statistical significance after adding studies (i.e., effect of exposure to PPIs on OS in melanoma.
3.10 Sensitivity analysis
Table S8 shows the sensitivity analysis of associations with evidence grading of II–III by removing small sample studies. The evidence grading for the effect of exposure to PPIs in any exposure window on PFS decreased from class II to III; the evidence grading for the effect of exposure to PPIs on OS in NSCLC decreased from class III to IV; regarding the effect on PFS, the evidence grading increased from class III to II. The evidence grading for the effect of exposure to antibiotics in any exposure window on PFS decreased from class II to III.
4 Discussion
The efficacy of ICIs is influenced by numerous factors, including the tumor mutational burden, programmed death- ligand 1 (PD-L1) expression, and DNA mismatch repair gene defects (45). As a potentially controllable external factor, the interaction between ICIs and concomitant medications should be considered to ensure treatment effectiveness.
To our knowledge, this is the first umbrella review of the effects of 11 concomitant medications on the efficacy of ICIs. We used rigorous quality assessment and exclusion of overlapping studies, included a total of 23 published articles, re-conducted meta-analysis synthesis and assessment of the certainty of evidence, and combed the available findings. Overall, exposure to antibiotics, PPIs, steroids, and opioids was identified as the main factor leading to a decrease in the efficacy of ICIs. Statins and probiotics may exert positive effects, while treatment with H2RAs, metformin, β-blockers, aspirin, and NSAIDs did not affect the efficacy.
Analyses demonstrated that antibiotics have a significant impact on the efficacy of ICIs against NSCLC, RCC, and UC, but not against melanoma, HCC, and esophageal squamous cell carcinoma. Recent studies have shown that the use of antibiotics in patients with HCC early in the course of treatment with ICIs can improve prognosis, possibly by reducing the abundance of bacteria with immune inhibitory functions (46). Exposure to antibiotics before or after the initiation of ICIs is associated with a decline in prognosis, while the negative effects of exposure to antibiotics during ICIs use are more limited. The study conducted by Hogue et al. (47), which was included in the meta-analysis, played a critical role in the results, nevertheless, this is an abstract article that provides insufficient information and may have significant bias. We found that three associations had significant publication bias. The statistical significance of the results of these associations were lost after re-analysis using the trim and fill method, indicating that the negative effects of antibiotics on ICIs may be overestimated.
The mechanism by which antibiotics affect the efficacy of ICIs includes changes in the gut microbiota and inhibition of immune cell responses (48). A retrospective study showed that antibiotics had a negative impact on the prognosis of treatment with ICIs, but not chemotherapy. This, to some extent, ruled out the interference of potential adverse prognostic factors on the results (5). Different types of antibiotics may exert different negative effects, and studies have shown that quinolones, carbapenems, and cephalosporins do not decrease OS or PFS (49). Additionally, studies have demonstrated that the negative effects of antibiotics vary depending on the expression of PD-L1; patients with high PD-L1 expression were more susceptible to the effects of antibiotics (50). However, subgroup analysis based on PD-L1 expression was not included in our meta-analysis. The possibility of infection in patients cannot be completely avoided. Consequently, there is an urgent need to develop antibiotics that do not affect the efficacy of ICIs.
We found that the effect of PPIs on ICIs efficacy is also related to the exposure window and tumor type. The use of PPIs prior to the initiation of ICIs therapy significantly affects the prognosis of patients. The mechanism that underlies the effects on efficacy may involve changes in gut microbiota diversity and immune suppression caused by PPIs (51). However, compared with the direct effect of antibiotics, PPIs may indirectly change the gut microbiota by inhibiting stomach acid (52). Hopkins et al. (53) found that the negative impact of PPIs on prognosis may be related to the decrease in CD19+ and CD16+ CD56+ immune cell counts caused by PPIs. Nonetheless, it is also possible that the indication of PPIs is a poor prognostic factor for patients. PPIs exert statistically significant effects on NSCLC and UC, whereas they do not affect melanoma and RCC. This difference may be due to the smaller number of studies included in the analysis. Nevertheless, preclinical studies have shown that PPIs have an inhibitory effect on melanoma, which may counteract their negative effect on the gut microbiota (54). In addition, studies have found that chronic use of PPIs in patients with RCC is associated with an increased risk of ICI-induced colitis (55). Currently available evidence suggests that H2RAs do not reduce the benefits of ICIs therapy. However, caution is needed due to the small number of studies included in the analysis.
Steroids are commonly used to treat serious adverse events caused by ICIs and relieve clinical symptoms (56). Evidence suggests that the use of steroids reduces the efficacy of ICIs; however, this effect must be analyzed in different settings. For patients with brain metastases, although steroids decrease OS and PFS, there is no significant impact on intracranial-PFS. Subgroup analysis of NSCLC patients with brain metastases showed that steroids did not significantly reduce the OS after treatment with ICIs. In addition, the use of steroids in patients treated with ICIs in combination with SRS did not have a negative effect on efficacy. This may be due to the protective effect of SRS on the central nervous system and the reversal of local immune suppression (57). Steroids are also used for non-cancer indications (e.g., autoimmune diseases, chronic obstructive pulmonary disease, hypersensitivity reactions) and irAEs, which do not reduce the efficacy of ICIs, possibly because cancer indications (e.g., brain metastases, respiratory distress, bone metastases, and anorexia) are poor prognostic factors for patients (58).
Although preclinical studies have found that metformin can synergize with programmed death-1 (PD-1) inhibitors (59), some retrospective studies have shown that metformin does not affect the efficacy of ICIs. A recent study suggested that metformin exerts a significant synergistic effect compared with non-metformin hypoglycemic drugs (60). Another study yielded similar results; however, the use of dipeptidyl peptidase-4 (DDP4) inhibitor was identified as a potential confounding factor (61). In addition, whether the adjuvant effect of metformin is particularly important for obese patients should be further investigated (62). In this umbrella review, only one meta-analysis on metformin was included, which did not show an effect on the efficacy of ICIs. Overall, currently available evidence supports that metformin does not reduce the efficacy of ICIs. However, prospective studies with larger sample sizes and the elimination of confounding factors may be required to evaluate the potential enhancing effect of metformin on ICIs efficacy.
Our study showed weak evidence supporting that opioids may reduce the efficacy of ICIs. The possible mechanisms by which opioids exert this effect include immunosuppression of the tumor microenvironment (63, 64) and changes in the gut microbiome (65–67). Svaton et al. suggested that NSAIDs may enhance the efficacy of ICIs (68). Nevertheless, the currently available evidence does not indicate statistically significant effects. Further investigation is required to determine whether this is related to the timing and duration of NSAID use. In addition, clinicians should avoid the overuse of opioid drugs in patients receiving treatment with ICIs.
The present results indicated that β-blockers do not affect the efficacy of ICIs. Notably, apart from studies on β-blockers, there are currently no meta-analyses on angiotensin-converting enzyme (ACE) inhibitors and angiotensin II receptor blockers. A study suggested that ACE inhibitors may have a positive effect; however, this effect did not reach statistical significance (69). Another study indicated that the use of ACE inhibitors promotes immune suppression and reduces efficacy (70), while angiotensin II receptor blockers do not have a significant impact (71). Prospective studies with large sample sizes are warranted to confirm these findings.
Weak evidence suggests that statins and probiotics can improve the OS of patients receiving treatment with ICIs. Cantini et al. showed that high-intensity statins were associated with improved ICIs efficacy (72). In patients with NSCLC, statins have been linked to a trend for improvement in the efficacy of ICIs; however, this effect did not reach statistical significance. Statins may also improve ICIs efficacy by modulating the gut microbiome (73). In addition, preclinical studies have found that statins can lower the expression of PD-1 and cytotoxic T-lymphocyte associated protein-4 (CTLA-4) on T cells, increase antigen uptake by dendritic cells, and synergize with PD-1 inhibitors in terms of anti-tumor activity (74, 75). Gandhi and colleagues’ study showed that β blockers, metformin, aspirin, and statins had no effect on the efficacy of ICIs (76). In a mixed analysis group of multiple cancer types, probiotics did not exert a statistically significant effect; nevertheless, a trend towards enhanced efficacy was observed. In patients with NSCLC, probiotics may prolong OS and PFS; nevertheless, the evidence supporting this conclusion is currently weak. Dysbiosis of the gut microbiota is widely considered a key mechanism for the decreased efficacy of ICIs when used in combination with other drugs. Preclinical studies have highlighted that manipulation of the gut microbiota may improve the efficacy of ICIs (77). Additionally, further research is required to examine whether probiotics or statins can reverse the negative effects of other co-medications on ICIs.
This study has several limitations that should be acknowledged. Firstly, the methodological quality of the included publications was considered low or critically low. This low quality was mainly due to insufficient reporting of bias risks or the lack of a list of excluded literature. We partially addressed this limitation by re-evaluating bias risks. Secondly, due to the difficulty in conducting randomized controlled trials for concurrent medications, the included original studies were retrospective or prospective cohort studies; thus, bias risks are inevitable. There may be reverse causation; the use of antibiotics, PPIs, steroids, and opioids may be necessary due to the presence of poor prognostic factors in patients. This reverse causation may have affected the results. Therefore, caution is needed when interpreting the results. Thirdly, the impact of concurrent medications on the incidence of irAEs was not included in the meta-analyses; hence, future studies should address this topic. Finally, in addition to the concurrent medications included in our study, there are retrospective studies suggesting that vitamin D may improve ICIs efficacy (78). However, these studies were not included in our review due to the lack of meta-analyses. Therefore, more high-quality prospective studies are warranted to support the currently available evidence and avoid the impact of confounding factors.
For primary clinical studies and meta-analyses, more comprehensive evaluations are needed in the future, such as distinguishing the specific type and dose of concomitant medications, the patient’s performance status, and past disease history, so as to draw more accurate conclusions. Variations in concomitant medication effects on ICIs efficacy across cancer types require recognition, likely due to limited studies and differing ICIs regimens. These intriguing findings necessitate elucidation via rigorous clinical trials and preclinical studies. Additionally, disparate effects on other cancers, such as breast and endometrial cancer, warrant further investigation (79, 80).
5 Conclusions
Current evidence suggests that the use of antibiotics, PPIs, steroids, and opioids has a negative impact on the efficacy of ICIs. However, this effect may vary depending on the type of tumor, the timing of exposure, and the indication. Weak evidence suggests that statins and probiotics may enhance the efficacy of ICIs. H2 receptor antagonists, aspirin, metformin, β-blockers, and NSAIDs do not appear to affect the efficacy of ICIs. However, due to publication bias and methodological limitations, caution is advised in interpreting these results.
Data availability statement
The original contributions presented in the study are included in the article/Supplementary Material. Further inquiries can be directed to the corresponding author.
Author contributions
HLL: Conceptualization, Methodology, Software, Formal analysis, Data Curation, Writing - Original Draft, Visualization. LZ: Conceptualization, Methodology, Software, Visualization, Writing - Original Draft. FY: Data Curation, Project administration. RZ: Software, Visualization. XL Conceptualization, Validation, Supervision, Project administration. HJL: Conceptualization, Validation, Supervision, Project administration, Formal analysis, Validation, Supervision.
Funding
The study was funded by the Natural Science Foundation of Shandong Province (ZR2021LZY029).
Acknowledgments
We are grateful to Professor Corentin J Gosling of Paris Nanterre University, one the author of ‘metaumbrella’ R package, for guidance in analyzing data using R software.
Conflict of interest
The authors declare that the research was conducted in the absence of any commercial or financial relationships that could be construed as a potential conflict of interest.
Publisher’s note
All claims expressed in this article are solely those of the authors and do not necessarily represent those of their affiliated organizations, or those of the publisher, the editors and the reviewers. Any product that may be evaluated in this article, or claim that may be made by its manufacturer, is not guaranteed or endorsed by the publisher.
Supplementary material
The Supplementary Material for this article can be found online at: https://www.frontiersin.org/articles/10.3389/fimmu.2023.1218386/full#supplementary-material
Supplementary Figure 1 | AMSTAR 2 quality appraisal scores. AMSTAR, assessment of multiple systematic reviews. CDW, critical domains weaknesses (negatively answered); CL, critically low; L, low.
Supplementary Figure 2 | Forest plot of the impact of exposure to antibiotics on ICIs efficacy, excluded due to overlap. CL, critically low; HR, hazard ratio; ICIs, immune checkpoint inhibitor; L, low; NSCLC, non-small cell lung cancer; OR, odds ratio; ORR, objective response rate; OS, overall survival; PFS, progression-free survival; RCC, renal cell carcinoma; CM, concomitant medications.
Supplementary Figure 3 | Forest plot of the impact of exposure to PPIs on ICIs efficacy, excluded due to overlap. CL, critically low; HR, hazard ratio; ICIs, immune checkpoint inhibitor; L, low; NSCLC, non-small cell lung cancer; OS, overall survival; PFS, progression-free survival; PPI, proton pump inhibitor; UC, urothelial carcinoma; CM, concomitant medications.
Supplementary Figure 4 | Forest plot of the impact of exposure to steroids, NSAIDs, β-blockers, probiotics, and opioids on ICIs efficacy, excluded due to overlap. CL, critically low; HR, hazard ratio; ICIs, immune checkpoint inhibitor; NSAIDs, nonsteroidal anti-inflammatory agents; NSCLC, non-small cell lung cancer; OR, odds ratio; ORR, objective response rate; OS, overall survival; PFS, progression-free survival; CM, concomitant medications.
Supplementary Figure 5 | Funnel plots for publication bias regarding the exposure of patients with multiple types of cancer to ATB. (A. OS of ATB with any exposure window; B. PFS of ATB with any exposure window; C. ORR of ATB with any exposure window; D. RR of ATB with any exposure window; E. OS of ATB before ICIs initiation; F. PFS of ATB before ICIs initiation; G. ORR of ATB before ICIs initiation; H. OS of ATB during ICIs; I. PFS of ATB during ICIs; J. OS of ATB after ICIs initiation; K. PFS of ATB after ICIs initiation; L. PD of ATB before ICIs initiation >1 month; M. PD of ATB before ICIs initiation <1 month).
Supplementary Figure 6 | Funnel plots for publication bias regarding the exposure of patients with NSCLC to ATB. (A. OS of ATB with any exposure window; B. PFS of ATB with any exposure window; C. OS of ATB before ICIs initiation; D. PFS of ATB before ICIs initiation; E. OS of ATB after ICIs initiation; F. PFS of ATB after ICIs initiation; G. OS of ATB during ICIs; H. PFS of ATB during ICIs).
Supplementary Figure 7 | Funnel plots for publication bias regarding the exposure of patients with RCC, HCC, ESCC, or melanoma to ATB. (A. OS of ATB with any exposure window in RCC patients; B. PFS of ATB with any exposure window in RCC patients; C. ORR of ATB with any exposure window in RCC patients; D. OS of ATB with any exposure window in HCC patients; E. PFS of ATB with any exposure window in HCC patients; F. ORR of ATB with any exposure window in HCC patients; G. DCR of ATB with any exposure window in HCC patients; H. OS of ATB with any exposure window in ESCC patients; I. OS of ATB with any exposure window in melanoma patients).
Supplementary Figure 8 | Funnel plots for publication bias regarding the exposure of patients with multiple types of cancer to PPIs/H2RAs. (A. OS of PPIs with any exposure window in multiple cancer types; B. PFS of PPIs with any exposure window in multiple cancer types; C. ORR of PPIs with any exposure window in multiple cancer types; D. OS of PPIs exposure [-60, NA] in multiple cancer types; E. PFS of PPIs exposure [-60, NA] in multiple cancer types; F. OS of PPIs exposure [0, NA] in multiple cancer types; G. PFS of PPIs exposure [0, NA] in multiple cancer types; H. OS of PPIs with any exposure window in NSCLC patients; I. PFS of PPIs with any exposure window in NSCLC patients; J. OS of PPIs with any exposure window in RCC patients; K. PFS of PPIs with any exposure window in RCC patients; L. OS of PPIs with any exposure window in melanoma patients; M. PFS of PPIs with any exposure window in melanoma patients; N. OS of PPIs with any exposure window in UC patients; O. PFS of PPIs with any exposure window in UC patients; P. ORR of H2RAs with any exposure window in multiple cancer types; Q. ORR of H2RAs with any exposure window in multiple cancer types).
Supplementary Figure 9 | Funnel plots for publication bias regarding the exposure of patients with multiple types of cancer to steroids. (A. OS of steroids exposure in multiple cancer types; B. PFS of steroids exposure in multiple cancer types; C. OS of steroids exposure in multiple cancer types with brain metastasis patients; D. PFS of steroids exposure in multiple cancer types with brain metastasis patients; E. IC-PFS of steroids exposure in multiple cancer types with brain metastasis patients; F. OS of steroids exposure in multiple cancer types with brain metastasis patients no-receiving SRS; G. OS of steroids exposure in multiple cancer types with brain metastasis patients receiving SRS; H. OS of steroids exposure for cancer related indication in multiple cancer types; I. PFS of steroids exposure for cancer related indication in multiple cancer types; J. OS of steroids exposure for irAEs in multiple cancer types; K. PFS of steroids exposure for irAEs in multiple cancer types; L. OS of steroids exposure for non-cancer related indication in multiple cancer types; M. PFS of steroids exposure for non-cancer related indication in multiple cancer types; N. OS of steroids exposure in melanoma with brain metastasis patients; O. OS of steroids exposure in NSCLC with brain metastasis patients; P. OS of steroids exposure in NSCLC patients; Q. PFS of steroids exposure in NSCLC patients).
Supplementary Figure 10 | Funnel plots for publication bias regarding the exposure of patients with multiple types of cancer to aspirin, β-blockers, metformin, NSAIDs, opioids, probiotics, and statins. NSAIDs, nonsteroidal anti-inflammatory agents. (A. OS of aspirins exposure in multiple cancer types; B. PFS of aspirins exposure in multiple cancer types; C. OS of β-blockers exposure in multiple cancer types; D. PFS of β-blockers exposure in multiple cancer types; E. OS of metformin exposure in multiple cancer types; F. PFS of metformin exposure in multiple cancer types; G. OS of NSAIDs exposure in multiple cancer types; H. PFS of NSAIDs exposure in multiple cancer types; I. ORR of NSAIDs exposure in multiple cancer types; J. OS of opioids exposure in multiple cancer types; K. PFS of opioids exposure in multiple cancer types; L. ORR of opioids exposure in multiple cancer types; M. OS of probiotics exposure in NSCLC patients; N. PFS of probiotics exposure in NSCLC patients; O. ORR of probiotics exposure in multiple cancer types; P. DCR of probiotics exposure in multiple cancer types; Q. OS of statins exposure in NSCLC patients; R. PFS of statins exposure in NSCLC patients; S. OS of statins exposure in multiple cancer types; T. PFS of statins exposure in multiple cancer types).
References
1. Sung H, Ferlay J, Siegel RL, Laversanne M, Soerjomataram I, Jemal A, et al. Global cancer statistics 2020: globocan estimates of incidence and mortality worldwide for 36 cancers in 185 countries. CA Cancer J Clin (2021) 71(3):209–49. doi: 10.3322/caac.21660
2. Robert C. A decade of immune-checkpoint inhibitors in cancer therapy. Nat Commun (2020) 11(1):3801. doi: 10.1038/s41467-020-17670-y
3. Bai R, Lv Z, Xu D, Cui J. Predictive biomarkers for cancer immunotherapy with immune checkpoint inhibitors. Biomark Res (2020) 8:34. doi: 10.1186/s40364-020-00209-0
4. Rossi G, Pezzuto A, Sini C, Tuzi A, Citarella F, McCusker MG, et al. Concomitant medications during immune checkpoint blockage in cancer patients: Novel insights in this emerging clinical scenario. Crit Rev Oncol Hematol (2019) 142:26–34. doi: 10.1016/j.critrevonc.2019.07.005
5. Cortellini A, Di Maio M, Nigro O, Leonetti A, Cortinovis DL, Aerts JG, et al. Differential influence of antibiotic therapy and other medications on oncological outcomes of patients with non-small cell lung cancer treated with first-line pembrolizumab versus cytotoxic chemotherapy. J Immunother Cancer (2021) 9(4):e002421. doi: 10.1136/jitc-2021-002421
6. Chalabi M, Cardona A, Nagarkar DR, Dhawahir Scala A, Gandara DR, Rittmeyer A, et al. Efficacy of chemotherapy and atezolizumab in patients with non-small-cell lung cancer receiving antibiotics and proton pump inhibitors: pooled post hoc analyses of the oak and poplar trials. Ann Oncol (2020) 31(4):525–31. doi: 10.1016/j.annonc.2020.01.006
7. Iglesias-Santamaria A. Impact of antibiotic use and other concomitant medications on the efficacy of immune checkpoint inhibitors in patients with advanced cancer. Clin Transl Oncol (2020) 22(9):1481–90. doi: 10.1007/s12094-019-02282-w
8. Rizzo a, cusmai a, giovannelli f, acquafredda s, rinaldi l, misino a, et al. impact of proton pump inhibitors and histamine-2-receptor antagonists on non-small cell lung cancer immunotherapy: a systematic review and meta-analysis. Cancers (Basel) (2022) 14(6):1404. doi: 10.3390/cancers14061404
9. Liu C, Guo H, Mao H, Tong J, Yang M, Yan X. An up-to-date investigation into the correlation between proton pump inhibitor use and the clinical efficacy of immune checkpoint inhibitors in advanced solid cancers: a systematic review and meta-analysis. Front Oncol (2022) 12:753234. doi: 10.3389/fonc.2022.753234
10. Huang L, Chen X, Zhou L, Xu Q, Xie J, Zhan P, et al. Antibiotic exposure windows and the efficacy of immune checkpoint blockers in patients with cancer: a meta-analysis. Ann Palliat Med (2021) 10(3):2709–22. doi: 10.21037/apm-20-2076
11. Wu Q, Liu J, Wu S, Xie X. The impact of antibiotics on efficacy of immune checkpoint inhibitors in malignancies: A study based on 44 cohorts. Int Immunopharmacol (2021) 92:107303. doi: 10.1016/j.intimp.2020.107303
12. Zhang L, Wang H, Tian J, Sui L, Chen X. Concomitant statins and the survival of patients with non-small-cell lung cancer treated with immune checkpoint inhibitors: a meta-analysis. Int J Clin Pract (2022) 2022:3429462. doi: 10.1155/2022/3429462
13. Page MJ, McKenzie JE, Bossuyt PM, Boutron I, Hoffmann TC, Mulrow CD, et al. The PRISMA 2020 statement: an updated guideline for reporting systematic reviews. Rev Esp Cardiol (Engl Ed). (2021) 74(9):790–9. doi: 10.1016/j.rec.2021.07.010
14. Shea BJ, Reeves BC, Wells G, Thuku M, Hamel C, Moran J, et al. AMSTAR 2: a critical appraisal tool for systematic reviews that include randomised or non-randomised studies of healthcare interventions, or both. BMJ (2017) 358:j4008. doi: 10.1136/bmj.j4008
15. Okoth K, Chandan JS, Marshall T, Thangaratinam S, Thomas GN, Nirantharakumar K, et al. Association between the reproductive health of young women and cardiovascular disease in later life: umbrella review. BMJ (2020) 371:m3502. doi: 10.1136/bmj.m3502
16. Hennessy EA, Johnson BT. Examining overlap of included studies in meta-reviews: Guidance for using the corrected covered area index. Res Synth Methods (2020) 11(1):134–45. doi: 10.1002/jrsm.1390
17. Howes OD, Whitehurst T, Shatalina E, Townsend L, Onwordi EC, Mak TLA, et al. The clinical significance of duration of untreated psychosis: an umbrella review and random-effects meta-analysis. World Psychiatry (2021) 20(1):75–95. doi: 10.1002/wps.20822
18. Mathes T, Kuss O. A comparison of methods for meta-analysis of a small number of studies with binary outcomes. Res Synth Methods (2018) 9(3):366–81. doi: 10.1002/jrsm.1296
19. Veroniki AA, Jackson D, Viechtbauer W, Bender R, Bowden J, Knapp G, et al. Methods to estimate the between-study variance and its uncertainty in meta-analysis. Res Synth Methods (2016) 7(1):55–79. doi: 10.1002/jrsm.1164
20. Li X, Celotto S, Pizzol D, Gasevic D, Ji MM, Barnini T, et al. Metformin and health outcomes: An umbrella review of systematic reviews with meta-analyses. Eur J Clin Invest (2021) 51(7):e13536. doi: 10.1111/eci.13536
21. Sterne JA, Gavaghan D, Egger M. Publication and related bias in meta-analysis: power of statistical tests and prevalence in the literature. J Clin Epidemiol (2000) 53(11):1119–29. doi: 10.1016/s0895-4356(00)00242-0
22. Kalliala I, Markozannes G, Gunter MJ, Paraskevaidis E, Gabra H, Mitra A, et al. Obesity and gynaecological and obstetric conditions: umbrella review of the literature. BMJ (2017) 359:j4511. doi: 10.1136/bmj.j4511
23. Gosling CJ, Solanes A, Fusar-Poli P, Radua J. Metaumbrella: the first comprehensive suite to perform data analysis in umbrella reviews with stratification of the evidence. BMJ Ment Health (2023) 26(1):e300534. doi: 10.1136/bmjment-2022-300534
24. Gosling CJ, Cartigny A, Mellier BC, Solanes A, Radua J, Delorme R. Efficacy of psychosocial interventions for Autism spectrum disorder: an umbrella review. Mol Psychiatry (2022) 27(9):3647–56. doi: 10.1038/s41380-022-01670-z
25. Chen B, Yang C, Dragomir MP, Chi D, Chen W, Horst D, et al. Association of proton pump inhibitor use with survival outcomes in cancer patients treated with immune checkpoint inhibitors: a systematic review and meta-analysis. Ther Adv Med Oncol (2022) 14:17588359221111703. doi: 10.1177/17588359221111703
26. Zhang L, Chen C, Chai D, Li C, Kuang T, Liu L, et al. Effects of PPIs use on clinical outcomes of urothelial cancer patients receiving immune checkpoint inhibitor therapy. Front Pharmacol (2022) 13:1018411. doi: 10.3389/fphar.2022.1018411
27. Deng R, Zhang H, Li Y, Shi Y. Effect of antacid use on immune checkpoint inhibitors in advanced solid cancer patients: a systematic review and meta-analysis. J Immunother (2023) 46(2):43–55. doi: 10.1097/CJI.0000000000000442
28. Chen H, Han KD, He ZJ, Huang YS. How to choose a survival period? the impact of antibiotic use on os or pfs in nsclc patients treated with immune checkpoint inhibitors: a systematic review and meta-analysis. Technol Cancer Res Treat (2021) 20:15330338211033498. doi: 10.1177/15330338211033498
29. Crespin A, Bandinelli P-A, Bescop CL, Buffet R, Gunzburg JD, Vitry F, et al. 278 Systematic review and meta-analysis evaluating the impact of antibiotic use on clinical outcomes of non-small-cell lung cancer patients treated with immune checkpoint inhibitors. J ImmunoTher Cancer (2021) 9(Suppl 2):A301–2. doi: 10.1136/jitc-2021-SITC2021.278
30. Lurienne L, Cervesi J, Duhalde L, de Gunzburg J, Andremont A, Zalcman G, et al. NSCLC immunotherapy efficacy and antibiotic use: a systematic review and meta-analysis. J Thorac Oncol (2020) 15(7):1147–59. doi: 10.1016/j.jtho.2020.03.002
31. Tsikala-Vafea M, Belani N, Vieira K, Khan H, Farmakiotis D. Use of antibiotics is associated with worse clinical outcomes in patients with cancer treated with immune checkpoint inhibitors: A systematic review and meta-analysis. Int J Infect Dis (2021) 106:142–54. doi: 10.1016/j.ijid.2021.03.063
32. Wilson BE, Routy B, Nagrial A, Chin VT. The effect of antibiotics on clinical outcomes in immune-checkpoint blockade: a systematic review and meta-analysis of observational studies. Cancer Immunol Immunother (2020) 69(3):343–54. doi: 10.1007/s00262-019-02453-2
33. Luo Z, Hao S, Li Y, Cheng L, Zhou X, Gunes EG, et al. The negative effect of antibiotics on RCC patients with immunotherapy: A systematic review and meta-analysis. Front Immunol (2022) 13:1065004. doi: 10.3389/fimmu.2022.1065004
34. Zhang L, Chen C, Chai D, Li C, Guan Y, Liu L, et al. The association between antibiotic use and outcomes of HCC patients treated with immune checkpoint inhibitors. Front Immunol (2022) 13:956533. doi: 10.3389/fimmu.2022.956533
35. Zhou J, Huang G, Wong WC, Hu DH, Zhu JW, Li R, et al. The impact of antibiotic use on clinical features and survival outcomes of cancer patients treated with immune checkpoint inhibitors. Front Immunol (2022) 13:968729. doi: 10.3389/fimmu.2022.968729
36. Jessurun CAC, Hulsbergen AFC, de Wit AE, Tewarie IA, Snijders TJ, Verhoeff JJC, et al. The combined use of steroids and immune checkpoint inhibitors in brain metastasis patients: a systematic review and meta-analysis. Neuro Oncol (2021) 23(8):1261–72. doi: 10.1093/neuonc/noab046
37. Wang Y, Yang M, Tao M, Liu P, Kong C, Li H, et al. Corticosteroid administration for cancer-related indications is an unfavorable prognostic factor in solid cancer patients receiving immune checkpoint inhibitor treatment. Int Immunopharmacol (2021) 99:108031. doi: 10.1016/j.intimp.2021.108031
38. Zhang H, Li X, Huang X, Li J, Ma H, Zeng R. Impact of corticosteroid use on outcomes of non-small-cell lung cancer patients treated with immune checkpoint inhibitors: A systematic review and meta-analysis. J Clin Pharm Ther (2021) 46(4):927–35. doi: 10.1111/jcpt.13469
39. Zhang Y, Chen H, Chen S, Li Z, Chen J, Li W. The effect of concomitant use of statins, NSAIDs, low-dose aspirin, metformin and beta-blockers on outcomes in patients receiving immune checkpoint inhibitors: a systematic review and meta-analysis. Oncoimmunology (2021) 10(1):1957605. doi: 10.1080/2162402X.2021.1957605
40. Yan X, Liu P, Li D, Hu R, Tao M, Zhu S, et al. Novel evidence for the prognostic impact of beta-blockers in solid cancer patients receiving immune checkpoint inhibitors. Int Immunopharmacol (2022) 113(Pt A):109383. doi: 10.1016/j.intimp.2022.109383
41. Zhang L, Jin Q, Chai D, Kuang T, Li C, Guan Y, et al. The correlation between probiotic use and outcomes of cancer patients treated with immune checkpoint inhibitors. Front Pharmacol (2022) 13:937874. doi: 10.3389/fphar.2022.937874
42. Wan L, Wu C, Wu Q, Luo S, Liu J, Xie X. Impact of probiotics use on clinical outcomes of immune checkpoint inhibitors therapy in cancer patients. Cancer Med (2023) 12(2):1841–9. doi: 10.1002/cam4.4994
43. Mao Z, Jia X, Jiang P, Wang Q, Zhang Y, Li Y, et al. Effect of concomitant use of analgesics on prognosis in patients treated with immune checkpoint inhibitors: a systematic review and meta-analysis. Front Immunol (2022) 13:861723. doi: 10.3389/fimmu.2022.861723
44. Ju M, Gao Z, Liu X, Zhou H, Wang R, Zheng C, et al. The negative impact of opioids on cancer patients treated with immune checkpoint inhibitors: a systematic review and meta-analysis. J Cancer Res Clin Oncol (2022) 20:2699–708. doi: 10.1007/s00432-022-04513-0
45. Bagchi S, Yuan R, Engleman EG. Immune checkpoint inhibitors for the treatment of cancer: clinical impact and mechanisms of response and resistance. Annu Rev Pathol (2021) 16:223–49. doi: 10.1146/annurev-pathol-042020-042741
46. Fessas P, Naeem M, Pinter M, Marron TU, Szafron D, Balcar L, et al. Early antibiotic exposure is not detrimental to therapeutic effect from immunotherapy in hepatocellular carcinoma. Liver Cancer (2021) 10(6):583–92. doi: 10.1159/000519108
47. Hogue C, Kuzel T, Borgia J, Marwaha G, Bonomi P, Fidler M, et al. P2. 04-69 Impact of antibiotic usage on survival during checkpoint inhibitor treatment of non-small cell lung cancer (NSCLC). J Thorac Oncol (2019) 14(10):S735. doi: 10.1016/j.jtho.2019.08.1574
48. Routy B, Le Chatelier E, Derosa L, Duong CPM, Alou MT, Daillere R, et al. Gut microbiome influences efficacy of PD-1-based immunotherapy against epithelial tumors. Science (2018) 359(6371):91–7. doi: 10.1126/science.aan3706
49. Qiu H, Ma QG, Chen XT, Wen X, Zhang N, Liu WM, et al. Different classes of antibiotics exhibit disparate negative impacts on the therapeutic efficacy of immune checkpoint inhibitors in advanced non-small cell lung cancer patients. Am J Cancer Res (2022) 12(7):3175–84.
50. Ochi N, Ichihara E, Takigawa N, Harada D, Inoue K, Shibayama T, et al. The effects of antibiotics on the efficacy of immune checkpoint inhibitors in patients with non-small-cell lung cancer differ based on PD-L1 expression. Eur J Cancer (2021) 149:73–81. doi: 10.1016/j.ejca.2021.02.040
51. Maier L, Pruteanu M, Kuhn M, Zeller G, Telzerow A, Anderson EE, et al. Extensive impact of non-antibiotic drugs on human gut bacteria. Nature (2018) 555(7698):623–8. doi: 10.1038/nature25979
52. Imhann F, Bonder MJ, Vich Vila A, Fu J, Mujagic Z, Vork L, et al. Proton pump inhibitors affect the gut microbiome. Gut (2016) 65(5):740–8. doi: 10.1136/gutjnl-2015-310376
53. Hopkins AM, Badaoui S, Kichenadasse G, Karapetis CS, McKinnon RA, Rowland A, et al. Efficacy of atezolizumab in patients with advanced nsclc receiving concomitant antibiotic or proton pump inhibitor treatment: pooled analysis of five randomized control trials. J Thorac Oncol (2022) 17(6):758–67. doi: 10.1016/j.jtho.2022.02.003
54. Peppicelli S, Bianchini F, Toti A, Laurenzana A, Fibbi G, Calorini L. Extracellular acidity strengthens mesenchymal stem cells to promote melanoma progression. Cell Cycle (2015) 14(19):3088–100. doi: 10.1080/15384101.2015.1078032
55. Yin J, Elias R, Peng L, Levonyak N, Asokan A, Christie A, et al. Chronic use of proton pump inhibitors is associated with an increased risk of immune checkpoint inhibitor colitis in renal cell carcinoma. Clin Genitourin Cancer (2022) 20(3):260–9. doi: 10.1016/j.clgc.2022.01.017
56. Aldea M, Orillard E, Mansi L, Marabelle A, Scotte F, Lambotte O, et al. How to manage patients with corticosteroids in oncology in the era of immunotherapy? Eur J Cancer (2020) 141:239–51. doi: 10.1016/j.ejca.2020.09.032
57. Zhu Z, Ni J, Cai X, Su S, Zhuang H, Yang Z, et al. International consensus on radiotherapy in metastatic non-small cell lung cancer. Transl Lung Cancer Res (2022) 11(9):1763–95. doi: 10.21037/tlcr-22-644
58. Ricciuti B, Dahlberg SE, Adeni A, Sholl LM, Nishino M, Awad MM. Immune checkpoint inhibitor outcomes for patients with non-small-cell lung cancer receiving baseline corticosteroids for palliative versus nonpalliative indications. J Clin Oncol (2019) 37(22):1927–34. doi: 10.1200/JCO.19.00189
59. Scharping NE, Menk AV, Whetstone RD, Zeng X, Delgoffe GM. Efficacy of PD-1 blockade is potentiated by metformin-induced reduction of tumor hypoxia. Cancer Immunol Res (2017) 5(1):9–16. doi: 10.1158/2326-6066.CIR-16-0103
60. Chiang CH, Chen YJ, Chiang CH, Chen CY, Chang YC, Wang SS, et al. Effect of metformin on outcomes of patients treated with immune checkpoint inhibitors: a retrospective cohort study. Cancer Immunol Immunother (2023) 72(6):1951–6. doi: 10.1007/s00262-022-03363-6
61. Yang J, Kim SH, Jung EH, Kim SA, Suh KJ, Lee JY, et al. The effect of metformin or dipeptidyl peptidase 4 inhibitors on clinical outcomes in metastatic non-small cell lung cancer treated with immune checkpoint inhibitors. Thorac Cancer (2023) 14(1):52–60. doi: 10.1111/1759-7714.14711
62. Yendamuri S, Barbi J, Pabla S, Petrucci C, Punnanitinont A, Nesline M, et al. Body mass index influences the salutary effects of metformin on survival after lobectomy for stage I NSCLC. J Thorac Oncol (2019) 14(12):2181–7. doi: 10.1016/j.jtho.2019.07.020
63. Borner C, Warnick B, Smida M, Hartig R, Lindquist JA, Schraven B, et al. Mechanisms of opioid-mediated inhibition of human T cell receptor signaling. J Immunol (2009) 183(2):882–9. doi: 10.4049/jimmunol.0802763
64. Beagles K, Wellstein A, Bayer B. Systemic morphine administration suppresses genes involved in antigen presentation. Mol Pharmacol (2004) 65(2):437–42. doi: 10.1124/mol.65.2.437
65. Banerjee S, Sindberg G, Wang F, Meng J, Sharma U, Zhang L, et al. Opioid-induced gut microbial disruption and bile dysregulation leads to gut barrier compromise and sustained systemic inflammation. Mucosal Immunol (2016) 9(6):1418–28. doi: 10.1038/mi.2016.9
66. Acharya C, Betrapally NS, Gillevet PM, Sterling RK, Akbarali H, White MB, et al. Chronic opioid use is associated with altered gut microbiota and predicts readmissions in patients with cirrhosis. Aliment Pharmacol Ther (2017) 45(2):319–31. doi: 10.1111/apt.13858
67. Prasetya RA, Metselaar-Albers M, Engels F. Concomitant use of analgesics and immune checkpoint inhibitors in non-small cell lung cancer: A pharmacodynamics perspective. Eur J Pharmacol (2021) 906:174284. doi: 10.1016/j.ejphar.2021.174284
68. Svaton M, Zemanova M, Zemanova P, Kultan J, Fischer O, Skrickova J, et al. Impact of Concomitant Medication Administered at the Time of Initiation of Nivolumab Therapy on Outcome in Non-small Cell Lung Cancer. Anticancer Res (2020) 40(4):2209–17. doi: 10.21873/anticanres.14182
69. Buti S, Bersanelli M, Perrone F, Tiseo M, Tucci M, Adamo V, et al. Effect of concomitant medications with immune-modulatory properties on the outcomes of patients with advanced cancer treated with immune checkpoint inhibitors: development and validation of a novel prognostic index. Eur J Cancer (2021) 142:18–28. doi: 10.1016/j.ejca.2020.09.033
70. Medjebar S, Truntzer C, Perrichet A, Limagne E, Fumet JD, Richard C, et al. Angiotensin-converting enzyme (ACE) inhibitor prescription affects non-small-cell lung cancer (NSCLC) patients response to PD-1/PD-L1 immune checkpoint blockers. Oncoimmunology (2020) 9(1):1836766. doi: 10.1080/2162402X.2020.1836766
71. Miura K, Sano Y, Niho S, Kawasumi K, Mochizuki N, Yoh K, et al. Impact of concomitant medication on clinical outcomes in patients with advanced non-small cell lung cancer treated with immune checkpoint inhibitors: A retrospective study. Thorac Cancer (2021) 12(13):1983–94. doi: 10.1111/1759-7714.14001
72. Cantini L, Pecci F, Hurkmans DP, Belderbos RA, Lanese A, Copparoni C, et al. High-intensity statins are associated with improved clinical activity of PD-1 inhibitors in malignant pleural mesothelioma and advanced non-small cell lung cancer patients. Eur J Cancer (2021) 144:41–8. doi: 10.1016/j.ejca.2020.10.031
73. Vieira-Silva S, Falony G, Belda E, Nielsen T, Aron-Wisnewsky J, Chakaroun R, et al. Statin therapy is associated with lower prevalence of gut microbiota dysbiosis. Nature (2020) 581(7808):310–5. doi: 10.1038/s41586-020-2269-x
74. Xia Y, Xie Y, Yu Z, Xiao H, Jiang G, Zhou X, et al. The Mevalonate Pathway Is a Druggable Target for Vaccine Adjuvant Discovery. Cell (2018) 175(4):1059–1073.e21. doi: 10.1016/j.cell.2018.08.070
75. Elahi S, Weiss RH, Merani S. Atorvastatin restricts HIV replication in CD4+ T cells by upregulation of p21. AIDS (2016) 30(2):171–83. doi: 10.1097/QAD.0000000000000917
76. Gandhi S, Pandey M, Ammannagari N, Wang C, Bucsek MJ, Hamad L, et al. Impact of concomitant medication use and immune-related adverse events on response to immune checkpoint inhibitors. Immunotherapy (2020) 12(2):141–9. doi: 10.2217/imt-2019-0064
77. Lu Y, Yuan X, Wang M, He Z, Li H, Wang J, et al. Gut microbiota influence immunotherapy responses: mechanisms and therapeutic strategies. J Hematol Oncol (2022) 15(1):47. doi: 10.1186/s13045-022-01273-9
78. Luo J, Chen H, Ma F, Xiao C, Sun B, Liu Y, et al. Vitamin D metabolism pathway polymorphisms are associated with efficacy and safety in patients under anti-PD-1 inhibitor therapy. Front Immunol (2022) 13:937476. doi: 10.3389/fimmu.2022.937476
79. Chambers LM, Michener CM, Rose PG, Reizes O, Yao M, Vargas R. Impact of antibiotic treatment on immunotherapy response in women with recurrent gynecologic cancer. Gynecol Oncol (2021) 161(1):211–20. doi: 10.1016/j.ygyno.2021.01.015
Keywords: concomitant medications, immune checkpoint inhibitors, efficacy, umbrella review, meta-analysis
Citation: Li H, Zhang L, Yang F, Zhao R, Li X and Li H (2023) Impact of concomitant medications on the efficacy of immune checkpoint inhibitors: an umbrella review. Front. Immunol. 14:1218386. doi: 10.3389/fimmu.2023.1218386
Received: 07 May 2023; Accepted: 13 September 2023;
Published: 29 September 2023.
Edited by:
Dallas Flies, NextCure, Inc., United StatesReviewed by:
Shipra Gandhi, University at Buffalo, United StatesShui Liu, Emergency General Hospital, China
Copyright © 2023 Li, Zhang, Yang, Zhao, Li and Li. This is an open-access article distributed under the terms of the Creative Commons Attribution License (CC BY). The use, distribution or reproduction in other forums is permitted, provided the original author(s) and the copyright owner(s) are credited and that the original publication in this journal is cited, in accordance with accepted academic practice. No use, distribution or reproduction is permitted which does not comply with these terms.
*Correspondence: Huijie Li, 2008lihuijie@163.com
†These authors have contributed equally to this work and share first authorship