- 1China Basic Education Quality Monitoring Collaborative Innovation Center, Beijing Normal University, Beijing, China
- 2Peabody College of Education and Human Development, Vanderbilt University, Nashville, TN, United States
- 3Beijing Key Laboratory of Applied Experimental Psychology, National Demonstration Center for Experimental Psychology Education, Faculty of Psychology, Beijing Normal University, Beijing, China
Introduction: Emotion and attention regulation significantly influence various aspects of human functioning and behavior. However, the interaction between emotion and attention in affecting performance remains underexplored. This study aims to investigate how individual differences in sustained attention, influenced by varying emotional states.
Methods: A total of 12 participants underwent emotion induction through Virtual Reality (VR) videos; completed an AX-CPT (continuous performance test) task to measure sustained attention, for which task performance is evaluated from two aspects, task accuracy and task reaction times; and reported their flow states. EEG and PPG data were collected throughout the sessions, as supporting evidence for sustained attention.
Results: Our findings suggest that emotional valence and arousal significantly influence task reaction times and sustained attention, when gender differences are accounted for, but do not significantly impact task accuracy. Specifically, males responded faster under high-arousal negative emotions, while females responded faster under high-arousal positive emotions. Additionally, we find that flow experience is not significantly impacted by emotions states or sustained attention.
Discussion: The study underscores the nuanced interplay between emotion, sustained attention, and task performance, suggesting that emotional states can differentially impact cognitive processes. Also, it support the use of VR, EEG, and PPG technologies in future research on related topics. Future research could expand upon this study by including larger sample sizes and a wider range of emotional inductions to generalize the findings.
1 Introduction
Emotion and attention regulation both play important roles in adaptive functioning and behavior (Pekrun et al., 2002; Westphal et al., 2018; Whitehill et al., 2014). In daily life, we are constantly faced by large amounts of information, which we use attention to filter and process (Brosch et al., 2013); emotions, in turn, pose effect upon our attention profiles, changing the portion of information that we attend to, thus modulating behavior (Mitchell, 2022). Recently, the interaction between emotion and attention have come into focus in the fields of education and psychology (Wass et al., 2021; Baykal, 2022), as the specific process in which they impact performance remains unclear. In related research, a commonly applied theory of emotion is the dimensional theory, which distinguishes emotions based on their positions in a continuous multi-dimensional space, characterized by two primary dimensions: valence (degree of positivity or negativity of the emotion) and arousal (physiological activation of the emotion, high to low) (Russell, 1980). Existing research have shown that emotional arousal and valence can modulate attention allocation and selection (Compton, 2003; Phelps et al., 2006). However, findings on the specific relationship between different emotional dimensions and attention have yet to concur. Some studies indicate that high-arousal emotions increase attention directed to high-priority stimuli, but decrease attention toward low-priority stimuli (Mather and Sutherland, 2011). Other studies have identified that negative emotions can lead to global attention, whereas positive emotions lead to more local attention (Gasper and Clore, 2002). Further complicating the picture, arousal and valence also interact to produce varied effects on task performance, possibly related to their moderation on attention. For instance, research found that low-arousal negative affect enhances target recognition accuracy, high-arousal negative affect lower target accuracy, while positive affect’s influence on target accuracy do not differ significantly with different level of arousal (Jefferies et al., 2008).
Hence, it seems necessary to unify these research outcomes into a more comprehensive picture. In this study, we use task-specific sustained attention, i.e., the ability to maintain focus on the experiment task, as a bridging factor between attention and task performance, while also taking individual differences into consideration, in hopes for explicating the influence of different emotional dimensions on attention and task performance. This is measured through reaction time and accuracy performance on an AX-CPT (continuous performance test) task. In order to further clarify the picture, we also take one step forward from existing research by employing new methodologies to improve validity. Immersive Virtual Reality (VR) technology is used to enhance the ecological validity of emotion induction, in comparison to traditional induction measures, while sustained attention is additionally assessed through objective measures including electroencephalogram (EEG) and photoplethysmography (PPG), which could likely reflect sustained attention more directly than common subjective/indirect measures. To this end, we also integrate prior findings on physiological response to task activation, to form a set of signals that could be representative of sustained attention. All in all, our primary goal for this study is to explore how different valence and arousal of emotions impact sustained attention, as demonstrated by EEG and PPG data. Along the way, we also validate the application of VR, EEG and PPG technology in future related studies.
In line with this, we test a secondary hypothesis as well. The flow state is a subjective experience of effortless concentration (Csikszentmihalyi, 1975). Research on its relationship with attention has yielded inconsistent results: some studies suggested that people who frequently experience the flow state show more sustained attention (Swann et al., 2012), while others found no significant relationship between flow and sustained attention, since flow is an automatic, unconscious process, while sustained attention requires effort (Marty-Dugas and Smilek, 2019; Schiefele and Raabe, 2011; Ullen et al., 2012). These conflicts bring up our secondary hypothesis: Is experiencing the flow state related to sustained attention and emotion valence/arousal? If participants tend to experience flow states more frequently during certain attention/emotion states, this may also lead to differential task performance, and thus produce confused results (Harris et al., 2021). This hypothesis serves to resolve one more factor that may confound the effect of different emotion states on attention and task performance.
2 Materials and methods
2.1 Stimuli, paradigms and equipment
This study aims to investigate the impact of different emotional states on sustained attention, with a side focus on flow. The necessary instruments are detailed below.
Immersive VR videos were utilized to induce different emotional states, presented in a HTC Vive Pro HMD. These were selected from the Stanford Immersive Virtual Reality Video Database (Li et al., 2017), which contains 73 VR clips categorized according to emotion valence and arousal (Russell, 1980). In the end, four videos with scores closest to the quadrant extremes of the valence and arousal dimensions were chosen to achieve optimal emotion induction, as shown in Figure 1.
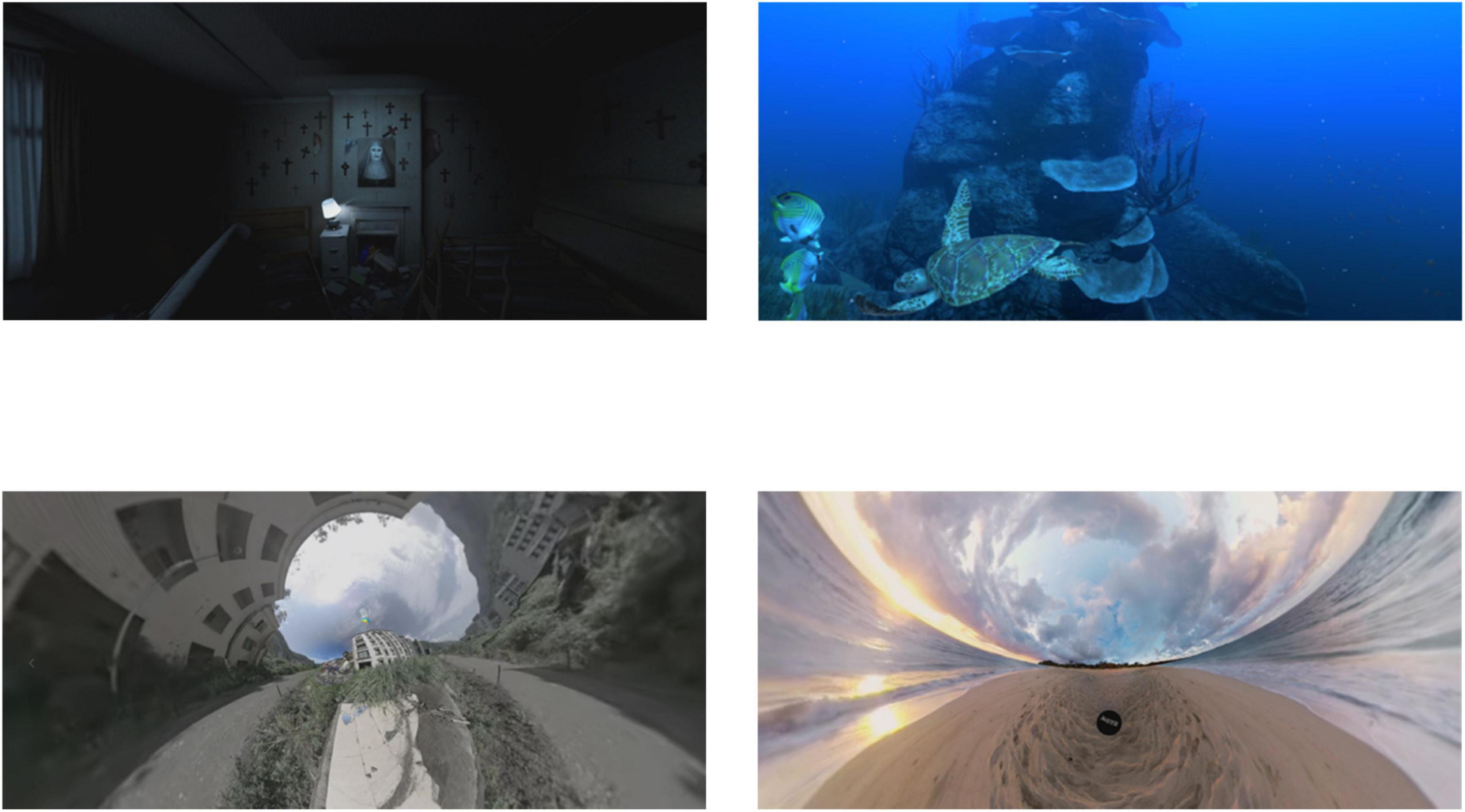
Figure 1. Screenshots of four VR videos used for emotion induction, including “negative-valence, high-arousal,” “positive-valence, high-arousal,” “negative-valence, low-arousal,” and “positive-valence, low-arousal.” Images reproduced from Li et al. (2017).
A subjective emotion self-report scale, the Self-Assessment Manikin (SAM; Bradley and Lang, 1994), was used to evaluate participants’ emotion arousal and valence. The AX-CPT (continuous performance test) paradigm was used to assess sustained attention. In AX-CPT, participants are instructed to respond to letter sequences, with “X” as the target stimulus, but only if preceded by the letter “A”. The sequences include four types: AX (target), AY, BX, and BY, where “B” can be any letter other than “A,” and “Y” can be any letter other than “X.” The classic AX-CPT paradigm comprised 70% AX sequences and 10% each of AY, BX, and BY sequences (Braver et al., 2007). Participants’ accuracy and reaction times were recorded during the task as performance criteria. The Flow Short Scale (Engeser and Rheinberg, 2008), a 10-item Likert scale, was used to measure flow experience during the AX-CPT task.
During the AX-CPT task, a Shimmer3 wearable device was used for ECG data collection, and an ANT Neuro system was used for EEG data collection, as shown in Figure 2.
2.2 Participants
A total of twelve participants were recruited for this study, 6 male and 6 female, aged 21 to 45 years (mean age = 31.2), right-handed and with normal or corrected-to-normal vision. Fields of expertise of the participants include computer science, education, psychology, foreign languages, marketing, and applied chemistry. All participants had no prior experience with similar experiments.
2.3 Procedure
The experiment was conducted one participant at a time without time limit, guided by a trained researcher in a quiet room and under constant screen brightness. Before the experiment, participants were introduced to the study, asked to sign a consent form, prepared for EEG and PPG collection, and instructed to fill out a demographic questionnaire covering gender, age, occupation, handedness, as well as previous VR experience. Then, participants were asked to stay stationary for 5 min, where a baseline SAM score was acquired. The experiment consists of four sessions, each with five steps, as shown in Figure 3. First, participants were randomly assigned to one of four conditions, each representing one of the four emotional dimensions: negative-valence high-arousal, negative-valence low-arousal, positive-valence high-arousal, or positive-valence low-arousal, to receive corresponding emotion induction through a VR video approximately 3 min and 30 s long. Afterwards, participants filled out the SAM scale, completed the AX-CPT attention task, and filled out the Flow Short Scale. Finally, participants take a 5-min rest before the next session.
2.4 Electroencephalogram (EEG) pre-processing
Before EEG data analysis, preprocessing was performed with EEGLAB (Delorme and Makeig, 2004), an open-source toolbox based on MATLAB, during which a band-pass FIR filter from 3 Hz to 47 Hz was applied.
2.5 Photoplethysmography (PPG) data
This study analyzed Heart Rate Variability (HRV), as calculated from PPG, to reflect autonomic responses that result from the sustained attention task. HRV analysis generally consists of two aspects, time-domain features and frequency-domain features.
Time-domain features reflect heart rate variability and autonomic regulation, including:
• MEANRR: Mean reciprocal of RR intervals, indicating heart rate stability, where RR interval is the time interval between two peaks.
• MEDIANRR: Median RR intervals, providing a robust measure against outliers.
• MEANHR: Mean heart rate, linked to cardiovascular health and autonomic function.
• SDNN: Standard deviation of NN intervals, reflecting overall HRV, where NN interval is the normal heartbeat interval.
• RMSSD: Root mean square of successive differences, indicating parasympathetic activity.
• pNN50: Percentage of NN intervals with differences over 50 ms, reflecting parasympathetic activity.
Frequency-domain features, which primarily reflects parasympathetic activity (Kumar et al., 2023), were analyzed using resampling, interpolation, and Fast Fourier Transform (FFT), to obtain Power Spectral Density (PSD). The final features includes:
• VLF: Very low frequency (0.0033–0.04 Hz), associated with long-term regulatory mechanisms.
• LF: Low frequency (0.04–0.15 Hz), reflecting sympathetic and parasympathetic activity.
• HF: High frequency (0.15–0.4 Hz), indicating parasympathetic (vagal) regulation.
• LF/HF Ratio: Evaluation on the balance between sympathetic and parasympathetic activity.
• Normalized LF (LF[n.u.]) and HF (HF[n.u.]): Relative power in their respective ranges, providing comparative importance via transforming absolute power to normalized units.
Absolute Power VLF (VLF[abs]), LF (LF[abs]), and HF (HF[abs]): Reflect the energy distribution in their respective frequency ranges, related to specific physiological mechanisms.
3 Results
3.1 Emotion induction
Due to the small sample size, we choose a non-parametric test, the Wilcoxon signed-rank test, to analyze differences in accuracy and reaction times in the AX-CPT task under different emotional states. Mean SAM scores among 12 participants are calculated for the four virtual reality videos. Firstly, we check to ensure that the emotion induction took effect. Results are presented in Table 1. Wilcoxon signed-rank tests showed significant differences in valence (Z = 3.07, p = 0.002) and arousal scores (Z = 3.01, p = 0.003) between corresponding videos. The virtual reality videos effectively elicited corresponding emotional responses.
3.2 Emotion and AX-CPT task data
Using the Wilcoxon signed-rank test, we analyze accuracy rates and reaction times during the AX-CPT task under the four emotional dimensions, and consider the influence of gender on the results.
As reported in Table 2, accuracy rates for positive emotions (M = 98.33, SD = 1.13, Mdn = 98.25) and negative emotions (M = 98.50, SD = 1.19, Mdn = 98.75) show no significant difference (Z = 0.49, p = 0.620). Accuracy rates for high-arousal emotions (M = 98.29, SD = 1.01, Mdn = 98.50) and low-arousal emotions (M = 98.54, SD = 1.16, Mdn = 98.75) also show no significant difference (Z = 1.08, p = 10.280). Also, reaction times for positive emotions (M = 30098.58, SD = 5758.64, Mdn = 29501.00) and negative emotions (M = 28465.17, SD = 5166.93, Mdn = 28273.00) show no significant difference (Z = 1.73, p = 0.084). Similarly, reaction times for high-arousal (M = 29061.67, SD = 4897.09, Mdn = 29341.00) and low-arousal emotions (M = 29502.08, SD = 5712.97, Mdn = 28618.00) show no significant difference (Z = 0.784, p = 0.433).
Further, as shown in Table 3, analyzing the influence of emotional valence, arousal, and gender on accuracy using the Wilcoxon signed-rank test revealed no significant differences (Z = 0.68, p = 0.500) in accuracy rates between males under high-arousal negative (M = 97.67, SD = 1.63, Mdn = 97.50) and high-arousal positive emotions (M = 98.33, SD = 1.37, Mdn = 98.00). Also, as shown in Table 4, for females, accuracy rates under high-arousal positive emotions (M = 98.00, SD = 1.55, Mdn = 98.00) and low-arousal positive emotions (M = 99.17, SD = 0.98, Mdn = 99.50) show no significant difference (Z = 1.63, p = 0.100). In comparison, for reaction time, males exhibit significantly shorter reaction times (Z = 2.20, p = 0.028, Cohen′sd = 1.16) under high-arousal negative emotions (M = 26449.17, SD = 4046.69, Mdn = 26046.00) compared to high-arousal positive emotions (M = 27511.17, SD = 3594.44, Mdn = 30373.00). For females, reaction times are significantly shorter (Z = 1.99, p = 0.046, Cohen′sd = 0.79) under high-arousal positive emotions (M = 30362.50, SD = 5326.55) compared to low-arousal positive emotions (M = 33004.17, SD = 8145.73).
There results suggest that emotional valence and arousal do not significantly affect task accuracy performance across different genders, but significantly impact reaction time performance. Males display shorter reaction times, i.e., better sustained attention, under high-arousal negative emotions than high-arousal positive emotions, while females display shorter reaction times under high-arousal positive emotions than low-arousal positive emotions. These results correspond with previous studies such as Bradley et al. (2001), that found males to show greater physiological reactivity toward negative emotions, and females to show greater reactivity toward positive emotions.
3.3 Emotion and flow experience data
Flow experience scores are displayed in Table 5. The Wilcoxon signed-rank test is used to assess differences in flow experience across the four sessions of emotional dimensions. Results indicate that emotional valence (positive: M = 52.46, SD = 9.17, Mdn = 50.75 and negative: M = 55.71, SD = 9.41, Mdn = 57.50) and arousal (high: M = 54.58, SD = 8.68, Mdn = 55.25 and low: M = 53.58, SD = 9.34, Mdn = 52.25) do not pose significant impact on flow experience during the AX-CPT (valence: Z = 1.49, p = 0.136 and arousal: Z = 0.45, p = 0.656). When accounting for gender, no significant difference (Z = 0.32, p = 0.750) in flow experience is found for males between high-arousal negative emotions (M = 50.67, SD = 10.29, Mdn = 50.50) and high-arousal positive emotions (M = 51.50, SD = 3.67, Mdn = 50.00). Neither is significant differences (Z = 0.68, p = 0.500) found for females between high-arousal positive emotion (M = 54.67, SD = 12.45, Mdn = 54.50) and low-arousal positive emotion (M = 56.00, SD = 11.35, Mdn = 56.00) conditions.
3.4 EEG data analysis
Differential brain activity due to variations in attention are commonly reflected in the frequency bands (Wang et al., 2011). Here, we extract and compare frequency domain characteristics, specifically Power Spectral Density (PSD), to reflect the changes of sustained attention in relation to emotion. PSD describes the distribution of signal power across frequencies. We calculate the PSD of α, β, and γ bands, as well as the sustained attention formula , using the Welch method from the Python-MNE toolkit (Gramfort et al., 2013). In detail, the Welch method divides the signal into n segments that allow overlap, which improves the signal’s variance properties (Solomon, 1991), windows the data, and computes the average PSD of multiple segments. In particular, the Hanning window is chosen for windowing to mitigate spectral distortion caused by rectangular windows (Harris, 1978). Additionally, baseline correction is performed by subtracting PSD values from the baseline phase, i.e. the first 5 min of each session, from the PSD values of each session. Finally, these PSD values are averaged over α (7–13 Hz), β (14–29 Hz), and γ (30–47 Hz) bands, to be used for analysis.
For the sake of analysis, high and low sustained attention emotion conditions are divided based on AX-CPT task performance, where only the groups with significant differences are retained, resulting in 12 samples with high sustained attention and 12 samples with low sustained attention. Meanwhile, normality tests are conducted for EEG power in α, β, γ bands, and ratio, for all subjects in each channel. The results suggest that the data does not have normality. Therefore, we use the Mann-Whitney U test to compare power differences between different sustained attention levels. We found the following patterns in EEG data that could represent sustained attention during the task, as shown in Figure 4, where the red circle represents the channels with significant differences between groups.
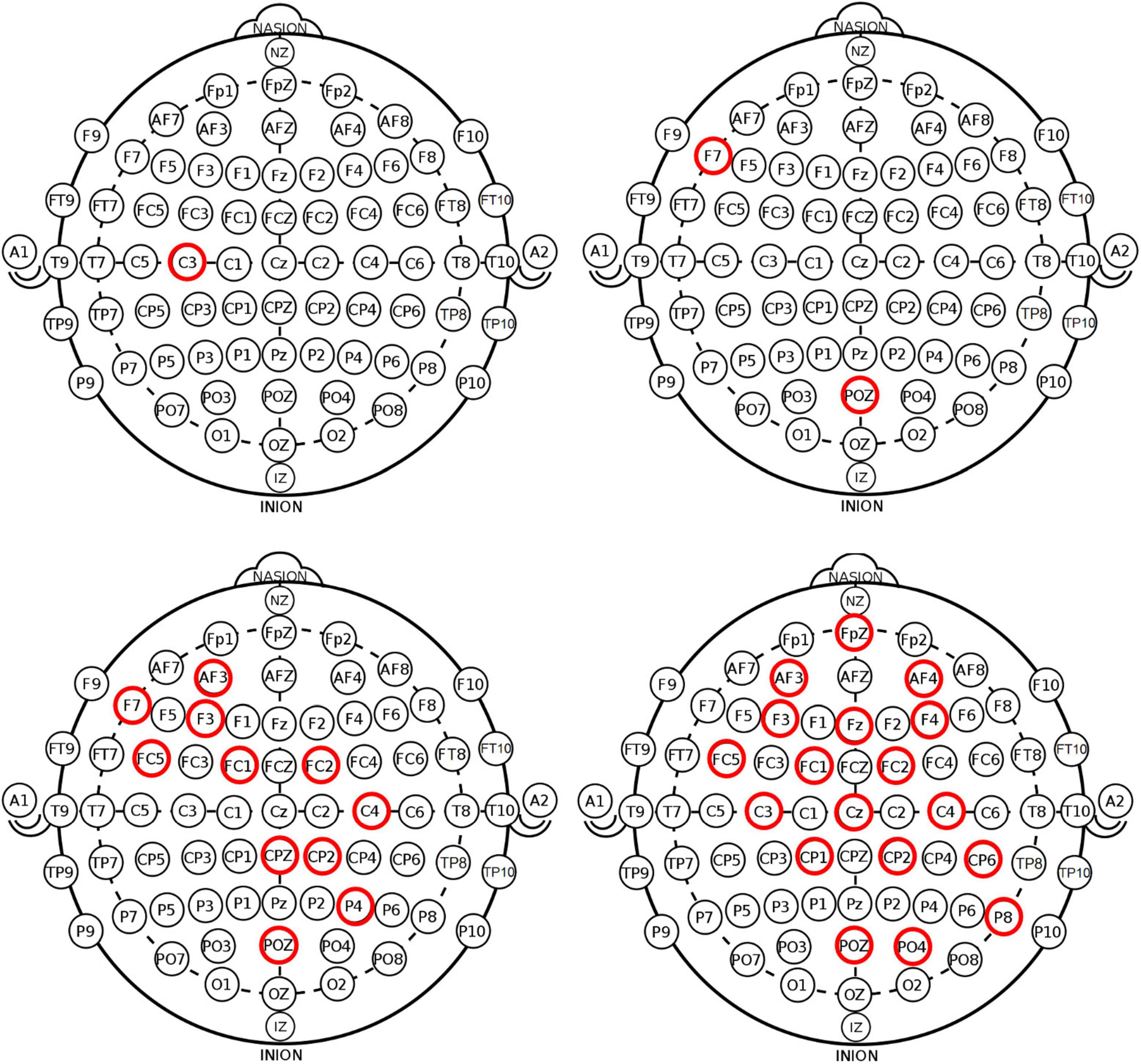
Figure 4. Patterns in EEG data that could represent sustained attention, for EEG power in α, β, γ bands, and ratio.
As shown in Table 6 and Figure 4a, the analysis of α band indicates that power in the C3 channel is significantly lower in high sustained attention state compared to low sustained attention state. This concurs with prior research, that found α wave activity in the C3 to be generally implicated in relaxation (Klimesch, 1999), meaning that decrease in activity can indicate heightened attention.
As shown in Table 7 and Figure 4b, for β band, analysis indicate that the power in the F7 and POZ channels is significantly higher in high sustained attention state compared to low sustained attention state. Correspondingly, β wave activity has been associated with attention and alertness (Rouhinen et al., 2013).
As shown in Table 8 and Figure 4c, for γ band, the analysis show that powers in the AF3, C4, CP2, CZ, F3, F7, FC1, FC2, FC5, P4, and POZ channels are significantly higher in high sustained attention state compared to low sustained attention state. In congruence with prior studies, γ wave activity is closely related to higher cognitive functions and information processing (Masuda, 2009; Rouhinen et al., 2013), which may be more active during high sustained attention.
For ratio, the analysis indicates that this ratio is significantly higher in high sustained attention state compared to low sustained attention state, as shown in Table 9 and Figure 4d. This is consistent with research, that suggested the ratio reflects changes in attention and alertness (Wang et al., 2011).
EEG results from this study indicate significant differences between high and low sustained attention conditions in the power of α, β, γ bands, as well as ratio. In high sustained attention state, EEG power in α band decreases in the C3 channel, β band power increases in the F7 and POZ channels, and both γ band power and increase in the parietal and frontal regions. These findings corroborate previous studies that found α wave power decrease, as well as β and γ wave power increase during high sustained attention states. This might be due to the increase in cognitive resources required for high sustained attention tasks (Başar et al., 2001; Klimesch, 1999).
3.5 PPG data analysis
We also examine heart rate variability (HRV) characteristics in participants under high and low sustained attention states, calculated from PPG data. Considering the sample size in general PPG data analysis, we divided each task into two halves, and HRV features are extracted from each half, resulting in 48 samples. After normality testing, HRV indices VLF[%], LF[%], HF[%], LF/HF, LF[n.u.], and HF[n.u.] follow a normal distribution, while MEANRR, MEDIANRR, MEANHR, SDNN, RMSSD, NN50, pNN50, VLF[abs], LF[abs], and HF[abs] do not.
Indices that adhere to normality are analyzed with t-test. Among these, HF[%] shows a significant decrease in high sustained attention state (M = 35.94, SD = 10.03) compared to low sustained attention state (M = 42.24, SD = 10.80), t(46)=2.09, p = 0.042, Cohen′sd = 0.60. Indices that do not adhere to normality are analyzed with the Mann-Whitney U test. Among them, VLF[abs] displays a significant increase in high sustained attention state (Md = 868.66) compared to low sustained attention state (Md = 500.26), U = 176.50, p = 0.021, Cohen′sd = 0.82. These findings align with previous research that found high sustained attention tasks to require more cognitive resources, which lead to changes in autonomic nervous system regulation: reduced parasympathetic activity, associated with decrease in HF[%], and increased sympathetic activity, associated with increase in VLF[abs] (Krygier et al., 2013; Thayer et al., 2012).
4 Discussion
As elaborated in previous sections, literature on emotions’ effect on attention has yielded mixed results. This study takes a step toward resolving existing contradictions by improving upon methodology: using more ecologically valid VR videos to induce emotions, and measuring sustained attention directly with EEG and PPG. Additionally, the study enhances the analysis by taking gender differences into consideration and using sustained attention as a factor to account for the quality of attention. Results show that for females, sustained attention levels (i.e. quality of attention) are significantly higher during high-arousal positive emotions compared to low-arousal positive emotions, while for males, sustained attention levels during high-arousal negative emotions are significantly higher than during high-arousal positive emotions. In particular, the findings of this study could be applied to educational settings to enhance learning outcomes. For example, understanding the impact of emotions on sustained attention could inform instructional design, suggesting that educators might tailor learning environments to evoke positive high-arousal states in students, potentially improving their engagement and performance. Similarly, in professional training and workplace settings, creating emotionally positive and stimulating environments could enhance employees’ focus and productivity.
Moreover, this study clarifies the relationship between flow experience and sustained attention, showing that there is no significant association under the context of timed AX-CPT tasks. This corresponds with previous research testing flow experience with timed tasks, such as Ullen et al. (2012), but points toward a possible link between flow experience and ecological validity of the experiment task. At the same time, our analysis on EEG and PPG provide insight into how heightened sustained attention is directly reflected in brain activity. Results from EEG data enables looking specifically at frequency bands related to attention and sustained attention, while raising a concern that may be related to the mixed results in previous research. That is, in subsequent studies, it may be worth considering using similar direct measurements to further distinguish between responses resulting from high sustained attention versus from emotional arousal.
This study uses a relatively small sample size. Future studies in related directions should consider using larger samples, while taking gender differences and quality of sustained attention into account when analyzing attention task performance. Potential future research directions can include exploring the application of these results to other types of attention tasks with more ecological validity, such as reading, writing, gaming, as well as untimed tasks. Also, researchers could investigate the adaptive contexts that brought forth these gender differences in emotion induction responses. In doing so, the effects of emotion on attention/engagement and flow experience in different contexts could be further explored, to point toward a more systematic, unified theory, that could be applied to improve performance in complex real-world contexts. This broader application could guide the development of more effective strategies in education, training, and therapy, ultimately enhancing individual performance and well-being.
Data availability statement
The raw data supporting the conclusions of this article will be made available by the authors, without undue reservation.
Ethics statement
The studies involving humans were approved by the Institutional Review Board of Beijing Normal University (No. BNU202212080135). The studies were conducted in accordance with the local legislation and institutional requirements. The participants provided their written informed consent to participate in this study.
Author contributions
YS: Conceptualization, Formal analysis, Funding acquisition, Writing – original draft. HZ: Visualization, Writing – original draft, Methodology. YL: Methodology, Software, Writing – original draft. XT: Conceptualization, Funding acquisition, Supervision, Writing – review and editing.
Funding
The author(s) declare financial support was received for the research, authorship, and/or publication of the article. This work was supported by the National Natural Science Foundation of China under Grants 62307003 and 62207002.
Conflict of interest
The authors declare that the research was conducted in the absence of any commercial or financial relationships that could be construed as a potential conflict of interest.
Publisher’s note
All claims expressed in this article are solely those of the authors and do not necessarily represent those of their affiliated organizations, or those of the publisher, the editors and the reviewers. Any product that may be evaluated in this article, or claim that may be made by its manufacturer, is not guaranteed or endorsed by the publisher.
References
Başar, E., Başar-Eroglu, C., Karakaş, S., and Schürmann, M. (2001). Gamma, alpha, delta, and theta oscillations govern cognitive processes. Int. J. Psychophysiol. 39, 241–248.
Baykal, B. (2022). Temporal effects of top-down emotion regulation strategies on affect, working memory load, and attentional deployment. Houston, TX: Faculty of The University of Houston-Clear Lake.
Bradley, M. M., and Lang, P. J. (1994). Measuring emotion: The self-assessment manikin and the semantic differential. J. Behav. Ther. Exp. Psychiatry 25, 49–59.
Bradley, M. M., Codispoti, M., Sabatinelli, D., and Lang, P. J. (2001). Emotion and motivation II: Sex differences in picture processing. Emotion 1, 300–319.
Braver, T. S., Gray, J. R., and Burgess, G. C. (2007). “Explaining the many varieties of working memory variation: Dual mechanisms of cognitive control,” in Variation in working memory, eds A. R. A. Conway, C. Jarrold, M. J. Kane, A. Miyake, and J. N. Towse (Oxford: Oxford University Press), 76–106.
Brosch, T., Scherer, K. R., Grandjean, D., and Sander, D. (2013). The impact of emotion on perception, attention, memory, and decision-making. Swiss Med. Wkly. 143:w13786.
Compton, R. J. (2003). The interface between emotion and attention: A review of evidence from psychology and neuroscience. Behav. Cogn. Neurosci. Rev. 2, 115–129. doi: 10.1177/1534582303255278
Csikszentmihalyi, M. (1975). Beyond boredom and anxiety, 1st Edn. Hoboken, NJ: Jossey-Bass Publishers.
Delorme, A., and Makeig, S. (2004). EEGLAB: An open-source toolbox for analysis of single-trial EEG dynamics. J. Neurosci. Methods 134, 9–21.
Engeser, S., and Rheinberg, F. (2008). Flow, performance and moderators of challenge-skill balance. Motiv. Emot. 32, 158–172.
Gasper, K., and Clore, G. L. (2002). Attending to the big picture: Mood and global versus local processing of visual information. Psychol. Sci. 13, 34–40. doi: 10.1111/1467-9280.00406
Gramfort, A., Luessi, M., Larson, E., Engemann, D. A., Strohmeier, D., Brodbeck, C., et al. (2013). MEG and EEG data analysis with MNE-Python. Front. Neurosci. 7:267. doi: 10.3389/fnins.2013.00267
Harris, D. J., Allen, K. L., Vine, S. J., and Wilson, M. R. (2021). A systematic review and meta-analysis of the relationship between flow states and performance. Int. Rev. Sport Exerc. Psychol. 16, 693–721.
Harris, F. J. (1978). On the use of windows for harmonic analysis with the discrete Fourier transform. Proc. IEEE 66, 51–83.
Jefferies, L. N., Smilek, D., Eich, E., and Enns, J. T. (2008). Emotional valence and arousal interact in attentional control. Psychol. Sci. 19, 290–295.
Klimesch, W. (1999). EEG alpha and theta oscillations reflect cognitive and memory performance: A review and analysis. Brain Res. Rev. 29, 169–195.
Krygier, J. R., Heathers, J. A. J., Shahrestani, S., Abbott, M., Gross, J. J., and Kemp, A. H. (2013). Mindfulness meditation, well-being, and heart rate variability: A preliminary investigation into the impact of intensive Vipassana meditation. Int. J. Psychophysiol. 89, 305–313. doi: 10.1016/j.ijpsycho.2013.06.017
Kumar, S. M., Vaishali, K., Maiya, G. A., Shivashankar, K. N., and Shashikiran, U. (2023). Analysis of time-domain indices, frequency domain measures of heart rate variability derived from ECG waveform and pulse-wave-related HRV among overweight individuals: An observational study. F1000Research 12:1229. doi: 10.12688/f1000research.139283.1
Li, B. J., Bailenson, J. N., Pines, A., Greenleaf, W. J., and Williams, L. M. (2017). A public database of immersive VR videos with corresponding ratings of arousal, valence, and correlations between head movements and self report measures. Front. Psychol. 8:2116. doi: 10.3389/fpsyg.2017.02116
Marty-Dugas, J., and Smilek, D. (2019). Deep, effortless concentration: Re-examining the flow concept and exploring relations with inattention, absorption, and personality. Psychol. Res. 83, 1760–1777. doi: 10.1007/s00426-018-1031-6
Masuda, N. (2009). Selective population rate coding: A possible computational role of gamma oscillations in selective attention. Neural Comput. 21, 3335–3362. doi: 10.1162/neco.2009.09-08-857
Mather, M., and Sutherland, M. R. (2011). Arousal-biased competition in perception and memory. Perspect. Psychol. Sci. 6, 114–133.
Pekrun, R., Goetz, T., Titz, W., and Perry, R. P. (2002). Academic emotions in students’ self-regulated learning and achievement: A program of qualitative and quantitative research. Educ. Psychol. 37, 91–105.
Phelps, E. A., Ling, S., and Carrasco, M. (2006). Emotion facilitates perception and potentiates the perceptual benefits of attention. Psychol. Sci. 17, 292–299.
Rouhinen, S., Panula, J., Palva, J. M., and Palva, S. (2013). Load dependence of β and γ oscillations predicts individual capacity of visual attention. J. Neurosci. 33, 19023–19033.
Schiefele, U., and Raabe, A. (2011). Skills-demands compatibility as a determinant of flow experience in an inductive reasoning task. Psychol. Rep. 109, 428–444. doi: 10.2466/04.22.PR0.109.5.428-444
Solomon, J. O. M. (1991). PSD computations using Welch’s method. [Power spectral density (PSD)] (SAND-91-1533). Albuquerque, NM: Sandia National Lab.
Swann, C., Keegan, R. J., Piggott, D., and Crust, L. (2012). A systematic review of the experience, occurrence, and controllability of flow states in elite sport. Psychol. Sport Exerc. 13, 807–819.
Thayer, J. F., Ahs, F., Fredrikson, M., Sollers, J. J., and Wager, T. D. (2012). A meta-analysis of heart rate variability and neuroimaging studies: Implications for heart rate variability as a marker of stress and health. Neurosci. Biobehav. Rev. 36, 747–756. doi: 10.1016/j.neubiorev.2011.11.009
Ullen, F., de Manzano, O., Almeida, R., Magnusson, P. K. E., Pedersen, N. L., Nakamura, J., et al. (2012). Proneness for psychological flow in everyday life: Associations with personality and intelligence. Pers. Individ. Differ. 52, 167–172.
Wang, X.-W., Nie, D., and Lu, B.-L. (2011). “EEG-based emotion recognition using frequency domain features and support vector machines,” in Neural information processing, Vol. 7062, eds B.-L. Lu, L. Zhang, and J. Kwok (Berlin Heidelberg: Springer), 734–743.
Wass, S. V., Smith, C. G., Stubbs, L., Clackson, K., and Mirza, F. U. (2021). Physiological stress, sustained attention, emotion regulation, and cognitive engagement in 12-month-old infants from urban environments. Dev. Psychol. 57, 1179–1194. doi: 10.1037/dev0001200
Westphal, A., Kretschmann, J., Gronostaj, A., and Vock, M. (2018). More enjoyment, less anxiety and boredom: How achievement emotions relate to academic self-concept and teachers’ diagnostic skills. Learn. Individ. Differ. 62, 108–117.
Keywords: sustained attention, emotion, virtual reality, electroencephalogram, photoplethysmography
Citation: Shen Y, Zheng H, Li Y and Tian X (2024) Understanding emotional influences on sustained attention: a study using virtual reality and neurophysiological monitoring. Front. Hum. Neurosci. 18:1467403. doi: 10.3389/fnhum.2024.1467403
Received: 23 July 2024; Accepted: 30 September 2024;
Published: 17 October 2024.
Edited by:
Tao Xu, Northwestern Polytechnical University, ChinaReviewed by:
Shihui Guo, Xiamen University, ChinaYi Feng, Central University of Finance and Economics, China
Copyright © 2024 Shen, Zheng, Li and Tian. This is an open-access article distributed under the terms of the Creative Commons Attribution License (CC BY). The use, distribution or reproduction in other forums is permitted, provided the original author(s) and the copyright owner(s) are credited and that the original publication in this journal is cited, in accordance with accepted academic practice. No use, distribution or reproduction is permitted which does not comply with these terms.
*Correspondence: Xuetao Tian, eHR0aWFuQGJudS5lZHUuY24=