- 1Department of Psychology, Psychology Clinical Neuroscience Center, University of New Mexico, Albuquerque, NM, United States
- 2The Mind Research Network of the Lovelace Biomedical Research Institute, University of New Mexico, Albuquerque, NM, United States
- 3DEVCOM Army Research Laboratory, Human Research, and Engineering Directorate, Aberdeen Proving Ground, MD, United States
Variable responses to transcranial direct current stimulation (tDCS) protocols across individuals are widely reported, but the reasons behind this variation are unclear. This includes tDCS protocols meant to improve attention. Attentional control is impacted by top-down and bottom-up processes, and this relationship is affected by state characteristics such as anxiety. According to Attentional Control Theory, anxiety biases attention towards bottom-up and stimulus-driven processing. The goal of this study was to explore the extent to which differences in state anxiety and related measures affect visual attention and category learning, both with and without the influence of tDCS. Using discovery learning, participants were trained to classify pictures of European streets into two categories while receiving 30 min of 2.0 mA anodal, cathodal, or sham tDCS over the rVLPFC. The pictures were classifiable according to two separate rules, one stimulus and one hypothesis-driven. The Remote Associates Test (RAT), Profile of Mood States, and Attention Networks Task (ANT) were used to understand the effects of individual differences at baseline on subsequent tDCS-mediated learning. Multinomial logistic regression was fit to predict rule learning based on the baseline measures, with subjects classified according to whether they used the stimulus-driven or hypothesis-driven rule to classify the pictures. The overall model showed a classification accuracy of 74.1%. The type of tDCS stimulation applied, attentional orienting score, and self-reported mood were significant predictors of different categories of rule learning. These results indicate that anxiety can influence the quality of subjects’ attention at the onset of the task and that these attentional differences can influence tDCS-mediated category learning during the rapid assessment of visual scenes. These findings have implications for understanding the complex interactions that give rise to the variability in response to tDCS.
Introduction
Attentional control is assumed to occur through bottom-up, implicit processes and top-down, consciously instigated processes, with this distinction codified via behavioral (Jonides, 1981; Wolfe et al., 1989) and neuroscience measures (Desimone and Duncan, 1995; Corbetta and Shulman, 2002; Mazaheri et al., 2011). While the usefulness of this distinction has been called into question, and processes outside this dichotomy have been proposed (Awh et al., 2012; Theeuwes, 2018), top-down and bottom-up attention remain the theoretical cornerstones of many cognitive models of attention and visual search (Posner, 1978; Duncan and Humphreys, 1989; Wolfe et al., 1989; Found and Müller, 1996; Ludwig and Gilchrist, 2002; Serences and Boynton, 2007). Part of the reason for their ubiquity is that this dichotomy provides ample room for interpretation (Wolfe et al., 2003; Lien et al., 2010; Theeuwes, 2010, 2018). Top-down and bottom-up represent broad directions for information flow that exist along an overlapping gradient. There is no discrete starting point for attention defined as top-down or bottom-up; rather, an attentional event begins somewhere along this gradient as dependent upon a host of state or trait influences (Katsuki and Constantinidis, 2014).
One factor known to bias attention towards implicit processing is anxiety (Bishop, 2007). Anxiety is often found to be detrimental to cognitive performance, with performance declining as complexity and attentional demands increase (Hembree, 1988; Eysenck and Calvo, 1992; Orem et al., 2008; Derakshan and Eysenck, 2009; Moran, 2016). Attentional Control Theory provides an account of how anxiety impacts attention and negatively impacts higher-level cognitive processing (Eysenck et al., 2007). This theory proposes that there are two competing systems of attention; a purpose-driven, top-down system, and a stimulus-driven, bottom-up system. Anxiety alters the balance of these competing systems in favor of bottom-up processing (Derryberry and Reed, 2002; Eysenck et al., 2007). This dichotomy is supported by neuroimaging, which has found partially overlapping substrates for these two systems (Corbetta and Shulman, 2002; Corbetta et al., 2008).
The antisaccade task has served as a behavioral analog for measuring changes in the balance of the competing systems in Attentional Control Theory (Miyake et al., 2000; Derakshan et al., 2009). In the antisaccade task, subjects are required to inhibit a reflexive saccade towards a sudden visual stimulus presented in the periphery and instead generate a purposeful saccade in the opposite direction. Purposeful and automatic saccades thus compete, with anxiety serving to suppress the former (Hunt et al., 2004; Massen, 2004). Administration of 7.5% CO2 has been used as a temporary way of increasing self-report anxiety and modeling generalized anxiety disorder in healthy volunteers (Bailey et al., 2007, 2011). Subjects given 7.5% CO2 have demonstrated a decreased ability to purposefully control eye movements in the antisaccade task (Garner et al., 2011). This ability is linked to the orienting network, which directs attention through space and is distinct from two other attention networks, executive and alerting. Appropriately, subjects given 7.5% CO2 exhibit greater orienting scores on the Attention Networks Task (ANT; Fan et al., 2002), demonstrating that this measure provides a way of quantifying attentional changes resulting from anxiety.
In viewing naturalistic scenes, the role of explicit attention is emphasized, where it has been proposed that attention falls more readily on pertinent rather than salient objects (Castelhano and Henderson, 2007; Henderson et al., 2009), with pertinence determined by explicit goals (Torralba et al., 2006; Neider and Zelinsky, 2008; Ehinger et al., 2009). However, while the factors that drive attention during Freeview of natural scenes have been described (Wolfe and Horowitz, 2017), the influence of baseline differences on these factors is less understood, especially in situations where preconscious knowledge and explicit goals are lacking. The trajectory of attention is then either driven by explicit ad-hoc goals, or by baseline differences, such as anxiety, that serve to influence the salience of items in the visual field (Itti and Koch, 2000; Wolfe and Horowitz, 2017). To explore these possibilities, the present study created a novel learning task where naturalistic stimuli could be categorized according to either top-down, hypothesis-driven, or bottom-up, stimulus-driven rules.
This study additionally employed transcranial direct current stimulation (tDCS), and the main effect of tDCS on learning is reported elsewhere (Gibson et al., 2020). Over the past several decades, tDCS, a form of non-invasive brain stimulation, has been coupled with behavioral interventions in an attempt to improve their efficacy (Clark and Parasuraman, 2014; Coffman et al., 2014). This includes functions classified under the umbrella of attention, with stimulation having been applied to areas across the cortex to try and improve different aspects of attention (Reteig et al., 2017). Some tDCS studies have found success in improving sustained attention by stimulating the right ventrolateral prefrontal cortex (rVLPFC; Clark et al., 2012; Coffman et al., 2012; Falcone et al., 2012; Nelson et al., 2014), a finding that is consistent with the association of the rVLPFC with the maintenance of attention and cognitive control (Coull et al., 1998, 2001; Aron et al., 2003, 2014; Hampshire et al., 2009, 2010). Besides attention, the rVLPFC is associated with other cognitive processes like convergent creativity, hypothesis testing, and rule learning (Seger et al., 2000; Bowden and Jung-Beeman, 2003a; Jung-Beeman et al., 2004; Seger and Cincotta, 2006; Goel et al., 2007; Mashal et al., 2007; Mihov et al., 2010; Crescentini et al., 2011; Cao et al., 2016). To examine how baseline differences in these constructs, as well as anxiety, influence tDCS-mediated category learning, subjects were tested on measures of convergent creativity, attention, and self-report state affect, before application of tDCS. The degree of interaction between these baseline measures and learning performance was then quantified. It was hypothesized that higher baseline anxiety would bias subjects to learn the stimulus-driven rather than hypothesis-driven rule.
Materials and Methods
Subjects
Subjects were recruited through the research portal of the University of New Mexico (UNM) and advertisements posted in and around the UNM campus. Subjects received either cash payment (approximately $30) or class credit for a single experimental visit that lasted around 2 h. Before enrollment, subjects were screened for typical tDCS inclusion criteria (Bikson et al., 2016). At the beginning of the experimental session, subjects were informed of the details and goals of the study, including the use of tDCS, and consented. Study materials and procedures were approved by the U.S. Army Research Laboratory’s Human Research Protection Program and by Chesapeake IRB.
Experimental Task
A novel paradigm using naturalistic visual stimuli was created for this study, where subjects were tasked with learning to classify pictures of European streets as belonging to one of two categories. These categories were associated with buttons 1 and 2 on the keyboard number pad. The stimuli were static street segment views accessed on Google Maps Street View1. During each trial a single static street view was presented for 2.5 s, followed by a fixation cross for 1.5 s. After an initial baseline block of 50 trials, there was a training portion consisting of four blocks of 60 trials each, where after each trial subjects received feedback on their classification accuracy. Feedback consisted of screens reading “Correct” or “Incorrect,” simultaneously accompanied by male voices with European accents reciting a range of feedback congruent with the written feedback. Following training, there were four test blocks of 50 trials each, all without feedback (Figure 1). The baseline block was framed as practice during which subjects were instructed to become accustomed to the timing of the stimuli and to begin thinking about criteria that might differentiate the two categories. Subjects were told that there were two regions but were not given any clues about possible ways to distinguish them, nor were they told that there might be more than one way to differentiate the regions. Instead, through discovery learning (Bruner, 1961), they were tasked with gleaning the identifying features via accuracy feedback in the training portion. The instruction screen read, “This task uses “discovery learning,” meaning that you do not know what separates one category from another at the start. All you know is that two categories exist. You will learn what separates the categories during feedback in the training portion. Any number of features could be critical to separating one category from another.”
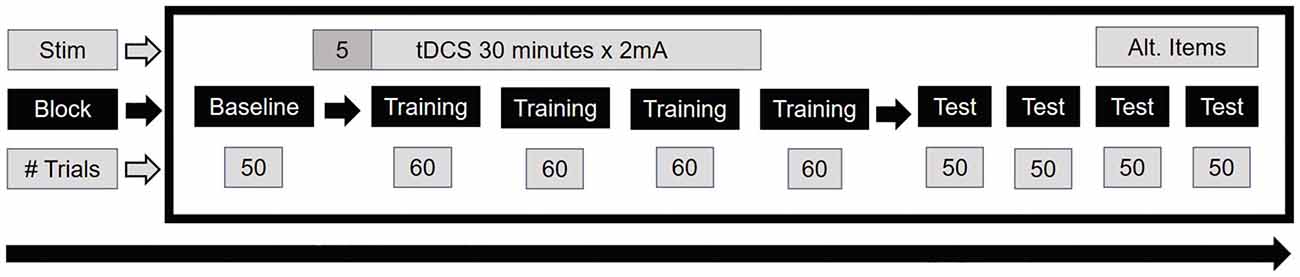
Figure 1. Timing of stimulation and design of the main task. A total of 54 subjects were run, with 18 each in the anodal, cathodal, and sham conditions.
Pictures could be correctly categorized through two arbitrary rules. The first rule differentiated regions based on how the picture was taken in relation to the road. In region 1, pictures were taken on the left-hand side of the road with traffic approaching, while in region 2, pictures were taken on the right-hand side of the road with traffic moving away (rule 1; Figure 2). The traffic pattern was on the right across all pictures. Rule 1 was created to model a bias towards explicit attention, as success in deciphering this rule does not depend on orienting towards items in the pictures, but rather gleaning the insight that rule 1 depends on the way the picture itself is taken. The second rule consisted of symbols within the pictures (i.e., hidden objects). Two side-by-side dots (umlaut) were in pictures for region 1, and a curved line (tilde) was in the pictures in region 2 (rule 2; Figure 3). The size of the two objects was standardized in pixels by height and width. Rule 2 was created to model a bias towards implicit attention driven by an orienting response. Hidden objects were only placed on human artifacts to accord with likely patterns of syntactic and semantic guidance (Biederman et al., 1982). Within the baseline, training, and first 2 test blocks, rule 1 (street direction) was present in all trials, while rule 2 (hidden objects) was present in 50% of the trials within each block. The last two test blocks contained trials designed to isolate and test learning of each rule individually. This consisted of 50 repeat images (all hidden object trials from the training portion where the hidden object had been removed) to preferentially isolate learning of rule 1, and 50 hidden object trials to preferentially isolate learning of rule 2 (where the street direction previously associated with each of the hidden objects was reversed). To ensure consistency throughout the task, the conspicuousness of each of the rules in individual pictures was rated on a 0–3 scale (with 0 being not present and 3 being very salient) by two researchers. These two ratings were then averaged, and the pictures were randomized to different blocks to ensure an even distribution of difficulty throughout the procedure. The criteria rated were: (1) saliency of written language (Cerf et al., 2009); (2) saliency of road direction rule; (3) saliency of hidden object rule; and (4) apparent temperature. All pictures were standardized to be 1,670 pixels wide and between 600 and 750 pixels tall.
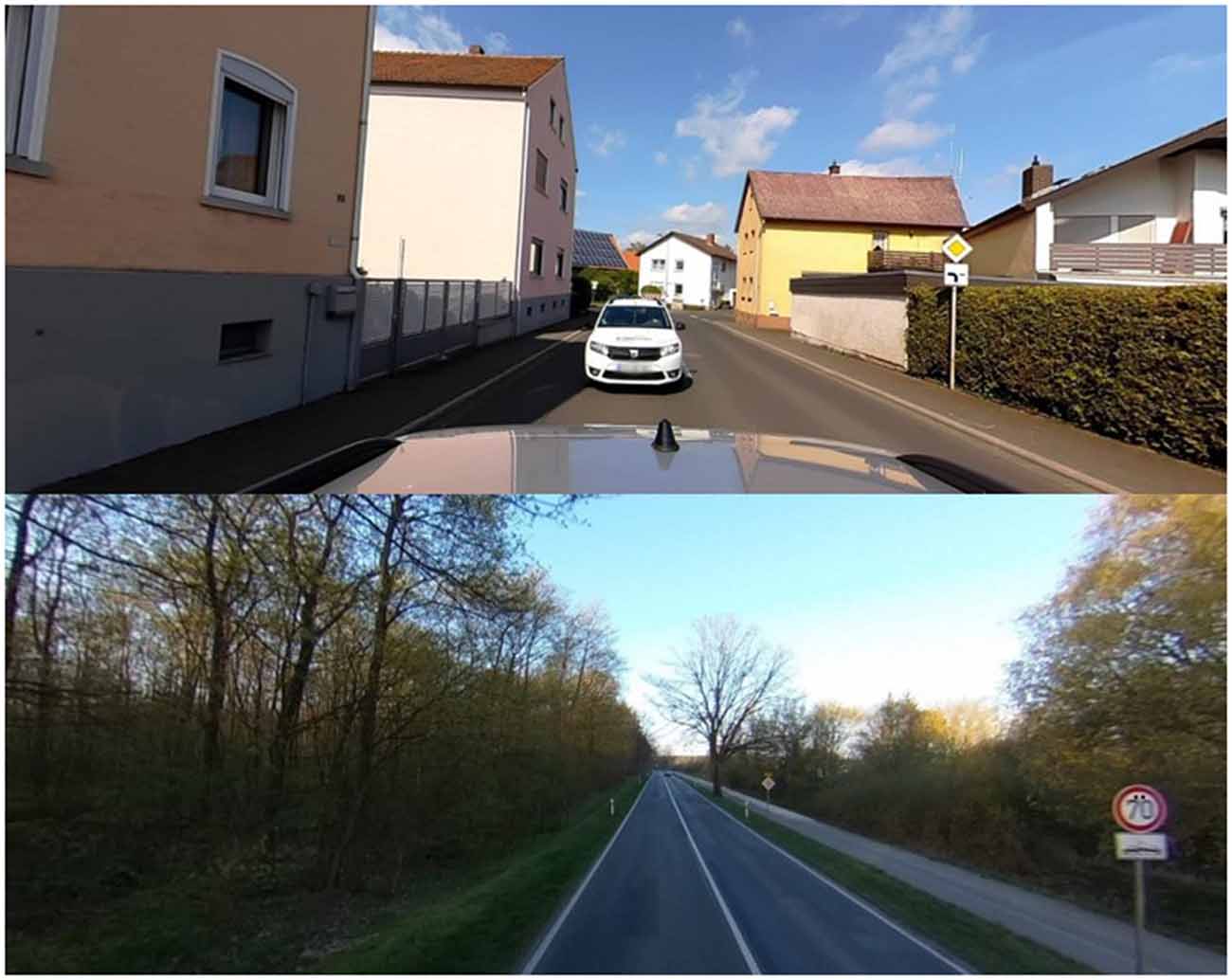
Figure 2. Example of rule 1 stimuli with approaching traffic (top picture) and rule 2 stimuli (bottom picture) with an umlaut (located on speed limit sign).
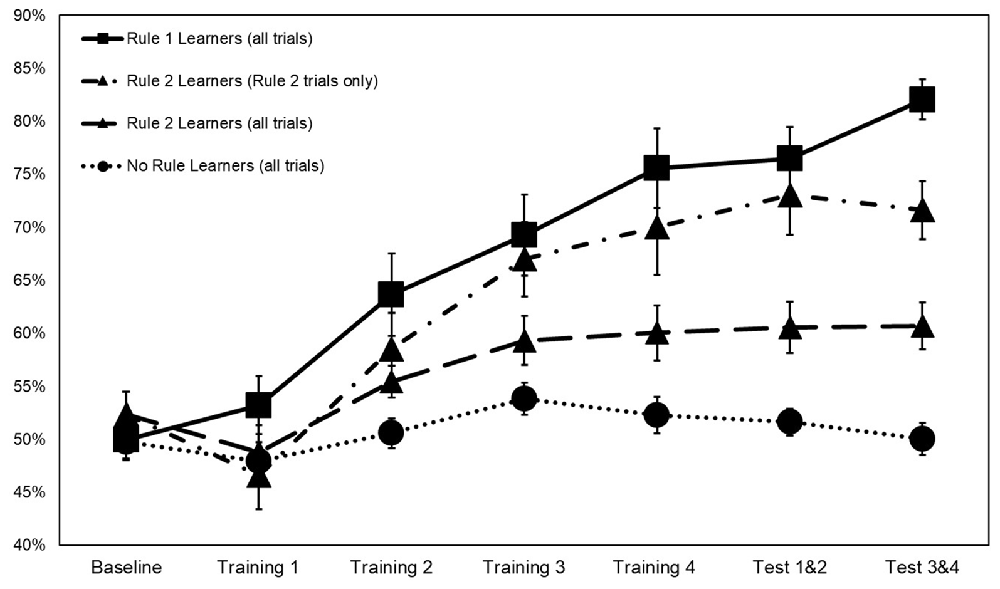
Figure 3. Categorization accuracy by rule group across training with rule 2 learners represented both on rule 2 accuracy only and on overall accuracy. Error bars ±1 SE.
tDCS
Subjects were randomized to receive anodal, cathodal, or sham stimulation over the left vlPFC. In each case, the return electrode was placed on the contralateral triceps. TDCS was administered by an ActivaDoseII Iontophoresis unit. Using a double-blind design, two of these units were connected to a blinding box, with 1 unit set to deliver an active dose of 2.0 mA and the other set to deliver a sham dose of 0.1 mA. Subjects were randomized to a specific switch on the blinding box, with the experimenter implementing the protocol unaware of the dosages associated with each switch. Two saline-soaked Amrex A5 (5 × 5 cm) sponges served as the electrodes, and these were attached to the subject’s arm with adhesive Coban wrap and to the subject’s head with an Amrex Velcro strap. Stimulation lasted 30 min and began after the baseline block. At 0 and 4 min after the start of stimulation, subjects completed a sensation questionnaire asking them to rate the degree of itching, heat and tingling on a 0–10 Likert-type scale. Subjects were informed that sensations rated 7 or above would prompt the termination of stimulation and end the experiment. After the first 5 min of stimulation, subjects began the 1st training block, with stimulation ending at the end of the 3rd training block.
Profile of Mood States
To explore possible interactions between self-reported affect and performance improvements during the categorization task, subjects completed the short form of the Profile of Mood States (POMS) before stimulation (Shacham, 1983; Grove and Prapavessis, 1992). The POMS includes seven unique subscales, tension, anger, fatigue, depression, esteem, vigor, and confusion. Subjects also completed the POMS at the end of the experimental visit to assess any possible affective changes induced by tDCS or the experimental task.
Remote Associates Test (RAT)
Before the experimental task, subjects performed the Remote Associates Test (RAT), a measure of convergent creativity (Mednick, 1962; Bowden and Jung-Beeman, 2003b). In the RAT the subject is presented with three words and is told to produce the 4th word that connects to the three presented words. For instance, a subject might be given the three words, “skate, pick, cream” where the appropriate answer would be “ice.” This test consisted of 15 unique items.
Attention Networks Task
As previous work has found improvements in the ANT following F10 anodal stimulation (Coffman et al., 2012), the current study implemented the ANT before the task to tie baseline ANT performance with task learning. The ANT (Fan et al., 2002) consists of a combination of the flanker and cued reaction time tasks, and yields scores corresponding to three attention networks, alerting, orienting, and executive control. The Orienting subscale is created by subtracting the average reaction time in trials where there is a spatial cue from trials in which no spatial cue is presented. Larger numbers for the Orienting subscale indicate a greater reaction time advantage when a spatial cue is included. The alerting subscale is created by subtracting reaction time on trials with a temporal cue from reaction time on trials without. The Executive subscale acts as a measure of inhibitory ability and is calculated by subtracting average response time on congruent flanker trials from average response time on incongruent flanker trials.
Data Analysis
To explicate learning of rules 1 and 2 within the main task, subjects were characterized as learners of rule 1, rule 2, both rules 1 and 2, or neither based on their categorization accuracy in test blocks 3 and 4. A subject was classified as a rule 1 learner if there was less than a 5% chance of having achieved their level of accuracy in the repeated images by chance alone. The value associated with a 5% chance was calculated from the distribution of categorization accuracy at baseline, such that scores above 60.5% were regarded as above chance. Rule 2 learners were similarly classified based on their performance in hidden object stimuli in test blocks 3 and 4. Based on these criteria, 19 subjects were classified as rule 1 learners, 14 as rule 2 learners, and 21 subjects as learners of neither rule. No subject had categorization accuracy above 60.5% for both rules 1 and 2.
Multinomial logistic regression was used to model the effect of performance on measures associated with the IFG and learning of the different rules in the main task. The full model contained one categorical variable, stimulation condition, and three continuous variables, Orienting subscale from the ANT, number of correct responses on the RAT, and the Tension subscale from the POMS.
Results
Subjects
Six subjects out of 60 were excluded from the final analysis. Two of these were excluded due to technical issues during data collection. An additional three subjects, one in each experimental group, were excluded for insufficient task engagement. Subjects were regarded as having insufficient task engagement if three criteria were met: classification accuracy was not appreciably above chance (60.5%) in at least one of the blocks, average response time was less than 1 s, and the pattern of response was indicative of disengagement. A response pattern was deemed as indicative of disengagement if responses were unidirectional (consistent 1’s or 2’s) or if the pattern of response consistently alternated across responses (1, 2, 1, 2, 1, 2…). One subject receiving cathodal stimulation reported a metallic taste and chose to leave the study during the first 5 min of stimulation. This left 54 subjects in the final analysis, equally distributed with 18 in each stimulation group. Rule group differences by demographic data and stimulation group are presented in Table 1.
Effects of tDCS on Category Learning
A mixed-model was run to explore the effect of stimulation conditions on overall learning (rules 1 and 2 combined through test block 2), the results of which are reported in detail elsewhere (Gibson et al., 2020). Both anodal and cathodal stimulation groups had significantly better improvement in categorization accuracy in comparison to sham, with anodal tDCS increasing categorization accuracy by 20.6% (SD = 16.1%) for an effect size of d = 1.71, and cathodal tDCS increasing categorization accuracy by 14.4% (SD = 11.8%) for an effect size of d = 1.16. In comparison, improvement in the sham stimulation group increased by 4.2% (SD = 11.7%) from baseline to test blocks 1 and 2.
Multinomial Logistic Regression
Interaction terms were created for the three continuous variables and dummy coded stimulation conditions. Continuous variables were mean-centered before the creation of interaction terms (Kraemer and Blasey, 2004). None of these interaction terms reached significance, so they were removed from the model. Means and standard deviations of continuous variables between rule groups are presented in Table 2. Categorization accuracy for the respective rule groups across the experimental task is presented in (Figure 3).
Given the variables included in the model, three criteria were found to significantly predict subjects being categorized as rule 1 as opposed to those who learned neither rule. Receiving anodal stimulation made it 97.5% more likely that a subject would belong to the rule 1 group rather than the no rule group (OR = 0.025, 95% CI = 0.002, 0.328). Two of the three continuous variables, orienting score (OR = 1.051, 95% CI = 1.014, 1.09) and tension sub-score (OR = 2.165, 95% CI = 1.144, 4.097), were significant predictors of belonging to the no rule learned group as opposed to rule 1 learners. The same two continuous variables (orienting score (OR = 1.041, 95% CI = 1.004, 1.079) and tension sub-score (OR = 2.928, 95% CI = 1.503, 5.702) were also significant predictors of belonging to the rule 2 group as opposed to the rule 1 group. Betas and odds ratios for both comparisons are presented in Table 3. The model had an overall classification accuracy of 74.1%, ranging from 64.3% accuracy for classifying learners of rule 2, 78.9% for learners of rule 1, and 76.2% for no rule learners.
Discussion
The results of this study support the interpretation that anodal tDCS over the rVLPFC is associated with an increased ability to learn rule 1, which requires an insight regarding the importance of traffic direction (Gibson et al., 2020). The results of the multinomial logistic regression additionally indicate that the quality of the attention subjects had as they began the task also selectively influenced category learning. Subjects who learned rule 2 featuring hidden objects had the largest tension sub-scores before stimulation at 5.14, compared to 1.16 in subjects who learned rule 1 featuring street directions. Rule 2 learners also had a gain in reaction time after receiving a spatial cue (Orienting) in the ANT, a difference of 44 ms compared to 29 ms in rule 1 learners. As predicted by Attentional Control Theory, the attention differences captured by the orienting subscale might themselves be the result of differences in state anxiety.
Differences in orienting driven by state anxiety possibly influenced subject learning during the experimental task. Rule 2 learners demonstrated a stronger reflexive saccade towards the positional cue in the ANT. Subsequently, rule 2 learners were more likely influenced by stimuli within the pictures presented during the experimental task, rather than by top-down goals (Schieber and Gilland, 2008; Allsop and Gray, 2014). Anxiety might have also disrupted working memory updating in rule 2 learners, further hindering systematic hypothesis testing (Eysenck and Calvo, 1992; Friedman and Miyake, 2004; Eysenck et al., 2007). In contrast, the lower relative anxiety of rule 1 learners allowed them to better control their attention, perhaps giving them an advantage in explicitly testing possible rules. Adding more complexity to an interpretation of the present results due to possible interactions with stimulation in the current study, changes in the relative activity of these attention systems are also associated with altered functioning of the prefrontal (Bishop, 2009; Eysenck and Derakshan, 2011) and ventrolateral prefrontal cortices (Ettinger et al., 2008; Fales et al., 2008).
Differences in convergent creativity, as measured by the RAT, may have also correlated with state anxiety, as previous research has shown that performing a convergent creativity task like the RAT is associated with decreases in mood (Chermahini and Hommel, 2012) and that this relationship is reciprocal (Bar, 2009), such that mood affects subsequent convergent creativity performance. Thus the same anxiety that promoted rule 2 learning could have also facilitated performance on the RAT. This may have also interacted with stimulation in the current study, as convergent creativity is associated with activity in the right hemisphere (Cerruti and Schlaug, 2009; Shah et al., 2013; Benedek et al., 2014; Hertenstein et al., 2019), and more subjects receiving anodal stimulation, rather than cathodal, gravitated towards rule 2 (8 vs. 4), while the number of rule 1 learners was even (8 vs. 8) between the anodal and cathodal groups, = 15.49, p = 0.004.
One debate between theoretical accounts of top-down and bottom-up processing involves the explanation of faster responses over time in stimuli that feature a single feature (Hillstrom, 2000; Wolfe et al., 2003; Wang et al., 2005; Awh et al., 2012). Do these faster reaction times indicate top-down processes (i.e., a tilde has been seen previously so attention is now purposefully being directed towards them), or do they indicate an unconscious priming effect that accrues over trials? The findings from the current study would seem to support top-down processes as 17 of 19 rule 1 learners mentioned street or traffic direction, and 12 of 14 rule 2 learners mentioned hidden objects as their main categorization criteria in a post-task debriefing. Subjects with higher baseline anxiety might have been more susceptible to an implicit orienting effect initially, but became fully aware of their search target as the training progressed. This awareness may have happened until later, however, in comparison to rule 1, learners are predisposed to top-down attentional control at the beginning of the task. While rule 1 learners appeared to identify rule 1 and begin improving in the first training block, as a group rule 2 learners did not begin to improve until the second training block. Subjects classified as no rule learners most often reported using architecture and written signage as categorization criteria.
Attentional control theory additionally posits that the detrimental effects of anxiety on performance can be overcome by compensatory strategies, with the employment of these strategies contingent upon motivation. In turn, motivation is thought to depend upon the clarity of task goals (Eysenck and Derakshan, 2011). In situations where goals are undefined, motivation and the use of compensatory strategies are likely to be low, leaving anxious individuals to fall back on an implicit attention system. This was demonstrated in what, to our knowledge, is the only other study to look at the effects of anxiety on category learning, where high anxiety was only detrimental to categorization performance when motivation was low (Hayes et al., 2009).
Limitations
While the results of the multinomial logistic regression speak to the first rule subjects gravitate towards (rule 1 vs. rule 2), they do not provide an answer to why no subjects learned more than 1 rule. Research has conceptualized this phenomenon as the satisfaction of search, originally defined in radiology, where the successful detection of 2nd specific target drastically decreases after identification of the first (Tuddenham, 1962). This effect is exacerbated by time constraints (Fleck et al., 2010), as were present in the current study. Future use of these stimuli should attempt to define the parameters necessary for learning multiple rules.
Additionally, while none of the variables included in the model had statistically significant interactions with stimulation conditions, the presence of tDCS is still a caveat for interpretation that bears on any implications these findings have for theories of attention. Attentional control measured by antisaccade performance has shown that activation of the right VLPFC predicts antisaccade control (Ettinger et al., 2008), and contrastingly, orienting also demonstrates a right hemisphere bias (Corbetta et al., 2000; Fan et al., 2005). Thus, stimulation of this area might have prompted these processes to work against each other or interact in an unknown way. Complex tDCS-mediated effects could have also occurred in other parts of the brain, as the current introduced by tDCS is not confined to the area underneath the electrode (Spreng et al., 2012; Fonteneau et al., 2018). It cannot be ruled out, and may even be likely, that trepidation about tDCS itself was the driving force behind individual differences in state anxiety and not preexisting differences. Several decades of recent research have established the safety of tDCS (Bikson et al., 2016; Nikolin et al., 2018), but the placement of electrodes on the head is still a novelty for most subjects, and some apprehension about the procedure is possible despite best practices in informed consent. While rule 2 learners in the current study had tension sub-scores that were typical in a college-aged population (Shacham, 1983; Nyenhuis et al., 1999), the influence of this baseline anxiety is a question that should be addressed by future tDCS studies. A cross-over design would have allowed for an exploration of anxiety, separating out those who were only anxious before receiving their first ever tDCS dose from those with preexisting differences in anxiety, but the stimuli used in the current study precluded this design as exposure to a second or third iteration of the learning task would not be comparable to the first. A final limitation is the novelty of the learning task used. Further validation of the ability of this task to differentiate rule learning based on self-reported anxiety is needed.
Conclusion
The current investigation demonstrates that individual differences can predict the trajectory of attention and that this trajectory in turn influences learning. While exploratory, these results fit within the structure of existing theories of attention and provide further evidence for the role anxiety plays within these theories. Importantly, these findings are relevant to real-world tasks that require effective orienting towards relevant stimuli, such as visual diagnosis, and piloting a car or plane, all of which can occur in situations of elevated anxiety or fatigue (Wilson et al., 2006; Allsop and Gray, 2014; Vine et al., 2016; Waite et al., 2019).
Data Availability Statement
The datasets generated for this study are available on request to the corresponding author.
Ethics Statement
The studies involving human participants were reviewed and approved by the U.S. Army Research Laboratory’s Human Research Protection Program, and by Advarra and Chesapeake Institutional Review Boards. The patients/participants provided their written informed consent to participate in this study.
Author Contributions
BG and VC: conceptualization and methodology. BG and TM: software. BG and MH: formal analysis. BG: investigation and writing—original draft preparation. JH, AY and VC: resources. All authors: writing—review and editing. BG and VC: supervision. VC: project administration and funding acquisition. All authors contributed to the article and approved the submitted version.
Funding
This Research was sponsored by the Army Research Laboratory and was accomplished under Cooperative Agreement Number W911NF-17-2-0001, and was also sponsored by NIGMS, NIH, P30GM122734. The views and conclusions contained in this document are those of the authors and should not be interpreted as representing the official policies, either expressed or implied, of the Army Research Laboratory or the U.S. Government. The U.S. Government is authorized to reproduce and distribute reprints for Government purposes not with standing any copyright notation herein.
Conflict of Interest
VC is a Scientific Advisor of NeuroGeneces LLC.
The remaining authors declare that the research was conducted in the absence of any commercial or financial relationships that could be construed as a potential conflict of interest.
Acknowledgments
We would like to thank our team at the Psychology Clinical Neuroscience Center for their efforts in making this research possible.
Footnotes
References
Allsop, J., and Gray, R. (2014). Flying under pressure: effects of anxiety on attention and gaze behavior in aviation. J. Appl. Res. Mem. Cogn. 3, 63–71. doi: 10.1016/j.jarmac.2014.04.010
Aron, A. R., Fletcher, P. C., Bullmore, E. T., Sahakian, B. J., and Robbins, T. W. (2003). Stop-signal inhibition disrupted by damage to right inferior frontal gyrus in humans. Nat. Neurosci. 6:115. doi: 10.1038/nn1003
Aron, A. R., Robbins, T. W., and Poldrack, R. A. (2014). Inhibition and the right inferior frontal cortex: one decade on. Trends Cogn. Sci. 18, 177–185. doi: 10.1016/j.tics.2013.12.003
Awh, E., Belopolsky, A. V., and Theeuwes, J. (2012). Top-down versus bottom-up attentional control: a failed theoretical dichotomy. Trends Cogn. Sci. 16, 437–443. doi: 10.1016/j.tics.2012.06.010
Bailey, J. E., Dawson, G. R., Dourish, C. T., and Nutt, D. J. (2011). Validating the inhalation of 7.5% CO2 in healthy volunteers as a human experimental medicine a model of generalized anxiety disorder (GAD). J. Psychopharmacol. 25, 1192–1198. doi: 10.1177/0269881111408455
Bailey, J. E., Kendrick, A., Diaper, A., Potokar, J. P., and Nutt, D. J. (2007). A validation of the 7.5% CO2 model of GAD using paroxetine and lorazepam in healthy volunteers. J. Psychopharmacol. 21, 42–49. doi: 10.1177/0269881106063889
Bar, M. (2009). A cognitive neuroscience hypothesis of mood and depression. Trends Cogn. Sci. 13, 456–463. doi: 10.1016/j.tics.2009.08.009
Benedek, M., Jauk, E., Fink, A., Koschutnig, K., Reishofer, G., Ebner, F., et al. (2014). To create or to recall? Neural mechanisms underlying the generation of creative new ideas. NeuroImage 88, 125–133. doi: 10.1016/j.neuroimage.2013.11.021
Biederman, I., Mezzanotte, R. J., and Rabinowitz, J. C. (1982). Scene perception: detecting and judging objects undergoing relational violations. Cogn. Psychol. 14, 143–177. doi: 10.1016/0010-0285(82)90007-x
Bikson, M., Grossman, P., Thomas, C., Zannou, A. L., Jiang, J., Adnan, T., et al. (2016). Safety of transcranial direct current stimulation: evidence based update 2016. Brain Stimul. 9, 641–661. doi: 10.1016/j.brs.2016.06.004
Bishop, S. J. (2007). Neurocognitive mechanisms of anxiety: an integrative account. Trends Cogn. Sci. 11, 307–316. doi: 10.1016/j.tics.2007.05.008
Bishop, S. J. (2009). Trait anxiety and impoverished prefrontal control of attention. Nat. Neurosci. 12, 92–98. doi: 10.1038/nn.2242
Bowden, E. M., and Jung-Beeman, M. (2003a). Aha! Insight experience correlates with solution activation in the right hemisphere. Psychon. Bull. Rev. 10, 730–737. doi: 10.3758/bf03196539
Bowden, E. M., and Jung-Beeman, M. (2003b). Normative data for 144 compound remote associate problems. Behav. Res. Methods Instrum. Comput. 35, 634–639. doi: 10.3758/bf03195543
Cao, B., Li, W., Li, F., and Li, H. (2016). Dissociable roles of medial and lateral PFC in rule learning. Brain Behav. 6:e00551. doi: 10.1002/brb3.551
Castelhano, M. S., and Henderson, J. M. (2007). Initial scene representations facilitate eye movement guidance in visual search. J. Exp. Psychol. Hum. Percept. Perform. 33, 753–763. doi: 10.1037/0096-1523.33.4.753
Cerf, M., Frady, E. P., and Koch, C. (2009). Faces and text attract gaze independent of the task: experimental data and computer model. J. Vis. 9, 10–10. doi: 10.1167/9.12.10
Cerruti, C., and Schlaug, G. (2009). Anodal transcranial direct current stimulation of the prefrontal cortex enhances complex verbal associative thought. J. Cogn. Neurosci. 21, 1980–1987. doi: 10.1162/jocn.2008.21143
Chermahini, S. A., and Hommel, B. (2012). Creative mood swings: divergent and convergent thinking affect mood in opposite ways. Psychol. Res. 76, 634–640. doi: 10.1007/s00426-011-0358-z
Clark, V. P., and Parasuraman, R. (2014). Neuroenhancement: enhancing brain and mind in health and in disease. NeuroImage 85, 889–894. doi: 10.1016/j.neuroimage.2013.08.071
Clark, V. P., Coffman, B. A., Mayer, A. R., Weisend, M. P., Lane, T. D. R., Calhoun, V. D., et al. (2012). TDCS guided using fMRI significantly accelerates learning to identify concealed objects. NeuroImage 59, 117–128. doi: 10.1016/j.neuroimage.2010.11.036
Coffman, B. A., Clark, V. P., and Parasuraman, R. (2014). Battery powered thought: enhancement of attention, learning and memory in healthy adults using transcranial direct current stimulation. NeuroImage 85, 895–908. doi: 10.1016/j.neuroimage.2013.07.083
Coffman, B. A., Trumbo, M. C., and Clark, V. P. (2012). Enhancement of object detection with transcranial direct current stimulation is associated with increased attention. BMC Neurosci. 13:108. doi: 10.1186/1471-2202-13-108
Corbetta, M., and Shulman, G. L. (2002). Control of goal-directed and stimulus-driven attention in the brain. Nat. Rev. Neurosci. 3, 201–215. doi: 10.1038/nrn755
Corbetta, M., Kincade, J. M., Ollinger, J. M., McAvoy, M. P., and Shulman, G. L. (2000). Voluntary orienting is dissociated from target detection in human posterior parietal cortex. Nat. Neurosci. 3, 292–297. doi: 10.1038/73009
Corbetta, M., Patel, G., and Shulman, G. L. (2008). The reorienting system of the human brain: from environment to theory of mind. Neuron 58, 306–324. doi: 10.1016/j.neuron.2008.04.017
Coull, J. T., Frackowiak, R. S. J., and Frith, C. D. (1998). Monitoring for target objects: activation of right frontal and parietal cortices with increasing time on task. Neuropsychologia 36, 1325–1334. doi: 10.1016/s0028-3932(98)00035-9
Coull, J. T., Nobre, A. C., and Frith, C. D. (2001). The noradrenergic α2 agonist clonidine modulates behavioural and neuroanatomical correlates of human attentional orienting and alerting. Cereb. Cortex 11, 73–84. doi: 10.1093/cercor/11.1.73
Crescentini, C., Seyed-Allaei, S., Pisapia, N. D., Jovicich, J., Amati, D., Shallice, T., et al. (2011). Mechanisms of rule acquisition and rule following in inductive reasoning. J. Neurosci. 31, 7763–7774. doi: 10.1523/JNEUROSCI.4579-10.2011
Derakshan, N., Ansari, T. L., Hansard, M., Shoker, L., and Eysenck, M. W. (2009). Anxiety, inhibition, efficiency and effectiveness. Exp. Psychol. 56, 48–55. doi: 10.1027/1618-3169.56.1.48
Derakshan, N., and Eysenck, M. W. (2009). Anxiety, processing efficiency and cognitive performance: new developments from attentional control theory. Eur. Psychol. 14, 168–176. doi: 10.1027/1016-9040.14.2.168
Derryberry, D., and Reed, M. A. (2002). Anxiety-related attentional biases and their regulation by attentional control. J. Abnorm. Psychol. 111, 225–236. doi: 10.1037//0021-843x.111.2.225
Desimone, R., and Duncan, J. (1995). Neural mechanisms of selective visual attention. Annu. Rev. Neurosci. 18, 193–222. doi: 10.1146/annurev.ne.18.030195.001205
Duncan, J., and Humphreys, G. W. (1989). Visual search and stimulus similarity. Psychol. Rev. 96, 433–458. doi: 10.1037/0033-295x.96.3.433
Ehinger, K. A., Hidalgo-Sotelo, B., Torralba, A., and Oliva, A. (2009). Modelling search for people in 900 scenes: a combined source model of eye guidance. Vis. Cogn. 17, 945–978. doi: 10.1080/13506280902834720
Ettinger, U., Ffytche, D. H., Kumari, V., Kathmann, N., Reuter, B., Zelaya, F., et al. (2008). Decomposing the neural correlates of antisaccade Eye movements using event-related FMRI. Cereb. Cortex 18, 1148–1159. doi: 10.1093/cercor/bhm147
Eysenck, M. W., and Calvo, M. G. (1992). Anxiety and performance: the processing efficiency theory. Cogn. Emot. 6, 409–434. doi: 10.1080/02699939208409696
Eysenck, M. W., and Derakshan, N. (2011). New perspectives in attentional control theory. Pers. Individ. Dif. 50, 955–960. doi: 10.1016/j.paid.2010.08.019
Eysenck, M. W., Derakshan, N., Santos, R., and Calvo, M. G. (2007). Anxiety and cognitive performance: attentional control theory. Emotion 7, 336–353. doi: 10.1037/1528-3542.7.2.336
Falcone, B., Coffman, B. A., Clark, V. P., and Parasuraman, R. (2012). Transcranial direct current stimulation augments perceptual sensitivity and 24-hour retention in a complex threat detection task. PLoS One 7:e34993. doi: 10.1371/journal.pone.0034993
Fales, C. L., Barch, D. M., Burgess, G. C., Schaefer, A., Mennin, D. S., Gray, J. R., et al. (2008). Anxiety and cognitive efficiency: differential modulation of transient and sustained neural activity during a working memory task. Cogn. Affect. Behav. Neurosci. 8, 239–253. doi: 10.3758/cabn.8.3.239
Fan, J., Mccandliss, B., Fossella, J., Flombaum, J., and Posner, M. (2005). The activation of attentional networks. NeuroImage 26, 471–479. doi: 10.1016/j.neuroimage.2005.02.004
Fan, J., McCandliss, B. D., Sommer, T., Raz, A., and Posner, M. I. (2002). Testing the efficiency and independence of attentional networks. J. Cogn. Neurosci. 14, 340–347. doi: 10.1162/089892902317361886
Fleck, M. S., Samei, E., and Mitroff, S. R. (2010). Generalized “satisfaction of search”: adverse influences on dual-target search accuracy. J. Exp. Psychol. Appl. 16, 60–71. doi: 10.1037/a0018629
Fonteneau, C., Redoute, J., Haesebaert, F., Le Bars, D., Costes, N., Suaud-Chagny, M.-F., et al. (2018). Frontal transcranial direct current stimulation induces dopamine release in the ventral striatum in human. Cereb. Cortex 28, 2636–2646. doi: 10.1093/cercor/bhy093
Found, A., and Müller, H. J. (1996). Searching for unknown feature targets on more than one dimension: investigating a “dimension-weighting” account. Percept. Psychophys. 58, 88–101. doi: 10.3758/bf03205479
Friedman, N. P., and Miyake, A. (2004). The relations among inhibition and interference control functions: a latent-variable analysis. J. Exp. Psychol. Gen. 133, 101–135. doi: 10.1037/0096-3445.133.1.101
Garner, M., Attwood, A., Baldwin, D. S., James, A., and Munafò, M. R. (2011). Inhalation of 7.5% carbon dioxide increases threat processing in humans. Neuropsychopharmacology 36, 1557–1562. doi: 10.1038/npp.2011.15
Gibson, B. C., Mullins, T. S., Heinrich, M. D., Witkiewitz, K., Yu, A. B., Hansberger, J. T., et al. (2020). Transcranial direct current stimulation facilitates category learning. Brain Stimul. 13, 393–400. doi: 10.1016/j.brs.2019.11.010
Goel, V., Tierney, M., Sheesley, L., Bartolo, A., Vartanian, O., Grafman, J., et al. (2007). Hemispheric specialization in human prefrontal cortex for resolving certain and uncertain inferences. Cereb. Cortex 17, 2245–2250. doi: 10.1093/cercor/bhl132
Grove, J. R., and Prapavessis, H. (1992). Preliminary evidence for the reliability and validity of an abbreviated profile of mood states. Int. J. Sport Psychol. 23, 93–109.
Hampshire, A., Chamberlain, S. R., Monti, M. M., Duncan, J., and Owen, A. M. (2010). The role of the right inferior frontal gyrus: inhibition and attentional control. NeuroImage 50, 1313–1319. doi: 10.1016/j.neuroimage.2009.12.109
Hampshire, A., Thompson, R., Duncan, J., and Owen, A. M. (2009). Selective tuning of the right inferior frontal gyrus during target detection. Cogn. Affect. Behav. Neurosci. 9, 103–112. doi: 10.3758/CABN.9.1.103
Hayes, S., MacLeod, C., and Hammond, G. (2009). Anxiety-linked task performance: dissociating the influence of restricted working memory capacity and increased investment of effort. Cogn. Emot. 23, 753–781. doi: 10.1080/02699930802131078
Hembree, R. (1988). Correlates, causes, effects and treatment of test anxiety. Rev. Educ. Res. 58, 47–77. doi: 10.3102/00346543058001047
Henderson, J. M., Malcolm, G. L., and Schandl, C. (2009). Searching in the dark: Cognitive relevance drives attention in real-world scenes. Psychon. Bull. Rev. 16, 850–856. doi: 10.3758/PBR.16.5.850
Hertenstein, E., Waibel, E., Frase, L., Riemann, D., Feige, B., Nitsche, M. A., et al. (2019). Modulation of creativity by transcranial direct current stimulation. Brain Stimul. 12, 1213–1221. doi: 10.1016/j.brs.2019.06.004
Hillstrom, A. P. (2000). Repetition effects in visual search. Percept. Psychophys. 62, 800–817. doi: 10.3758/bf03206924
Hunt, A. R., Olk, B., von Mühlenen, A., and Kingstone, A. (2004). Integration of competing saccade programs. Cogn. Brain Res. 19, 206–208. doi: 10.1016/j.cogbrainres.2003.12.004
Itti, L., and Koch, C. (2000). A saliency-based search mechanism for overt and covert shifts of visual attention. Vis. Res. 40, 1489–1506. doi: 10.1016/s0042-6989(99)00163-7
Jonides, J. (1981). Voluntary versus automatic control over the mind’s eye’s movement. Atten. Perform. 11, 187–203.
Jung-Beeman, M., Bowden, E. M., Haberman, J., Frymiare, J. L., Arambel-Liu, S., Greenblatt, R., et al. (2004). Neural activity when people solve verbal problems with insight. PLoS Biol. 2:e97. doi: 10.1371/journal.pbio.0020097
Katsuki, F., and Constantinidis, C. (2014). Bottom-Up and Top-Down attention: different processes and overlapping neural systems. Neuroscientist 20, 509–521. doi: 10.1177/1073858413514136
Kraemer, H. C., and Blasey, C. M. (2004). Centring in regression analyses: a strategy to prevent errors in statistical inference. Int. J. Methods Psychiatr. Res. 13, 141–151. doi: 10.1002/mpr.170
Lien, M.-C., Ruthruff, E., and Johnston, J. C. (2010). Attentional capture with rapidly changing attentional control settings. J. Exp. Psychol. Hum. Percept. Perform. 36, 1–16. doi: 10.1037/a0015875
Ludwig, C. J. H., and Gilchrist, I. D. (2002). Stimulus-driven and goal-driven control over visual selection. J. Exp. Psychol. Hum. Percept. Perform. 28, 902–912. doi: 10.1037/0096-1523.28.4.902
Mashal, N., Faust, M., Hendler, T., and Jung-Beeman, M. (2007). An fMRI investigation of the neural correlates underlying the processing of novel metaphoric expressions. Brain Lang. 100, 115–126. doi: 10.1016/j.bandl.2005.10.005
Massen, C. (2004). Parallel programming of exogenous and endogenous components in the antisaccade task. Q. J. Exp. Psychol. A 57, 475–498. doi: 10.1080/02724980343000341
Mazaheri, A., DiQuattro, N. E., Bengson, J., and Geng, J. J. (2011). Pre-stimulus activity predicts the winner of top-down vs. bottom-up attentional selection. PLoS One 6:e16243. doi: 10.1371/journal.pone.0016243
Mednick, S. (1962). The associative basis of the creative process. Psychol. Rev. 69, 220–232. doi: 10.1037/h0048850
Mihov, K. M., Denzler, M., and Förster, J. (2010). Hemispheric specialization and creative thinking: a meta-analytic review of lateralization of creativity. Brain Cogn. 72, 442–448. doi: 10.1016/j.bandc.2009.12.007
Miyake, A., Friedman, N. P., Emerson, M. J., Witzki, A. H., Howerter, A., Wager, T. D., et al. (2000). The Unity and diversity of executive functions and their contributions to complex “frontal lobe” tasks: a latent variable analysis. Cogn. Psychol. 41, 49–100. doi: 10.1006/cogp.1999.0734
Moran, T. P. (2016). Anxiety and working memory capacity: a meta-analysis and narrative review. Psychol. Bull. 142, 831–864. doi: 10.1037/bul0000051
Neider, M. B., and Zelinsky, G. J. (2008). Exploring set size effects in scenes: Identifying the objects of search. Vis. Cogn. 16, 1–10. doi: 10.1068/p6428
Nelson, J. T., McKinley, R. A., Golob, E. J., Warm, J. S., and Parasuraman, R. (2014). Enhancing vigilance in operators with prefrontal cortex transcranial direct current stimulation (tDCS). NeuroImage 85, 909–917. doi: 10.1016/j.neuroimage.2012.11.061
Nikolin, S., Huggins, C., Martin, D., Alonzo, A., and Loo, C. K. (2018). Safety of repeated sessions of transcranial direct current stimulation: a systematic review. Brain Stimul. 11, 278–288. doi: 10.1016/j.brs.2017.10.020
Nyenhuis, D. L., Yamamoto, C., Luchetta, T., Terrien, A., and Parmentier, A. (1999). Adult and geriatric normative data and validation of the profile of mood states. J. Clin. Psychol. 55, 79–86. doi: 10.1002/(sici)1097-4679(199901)55:1<79::aid-jclp8>3.0.co;2-7
Orem, D. M., Petrac, D. C., and Bedwell, J. S. (2008). Chronic self-perceived stress and set-shifting performance in undergraduate students. Stress 11, 73–78. doi: 10.1080/10253890701535103
Reteig, L. C., Talsma, L. J., van Schouwenburg, M. R., and Slagter, H. A. (2017). Transcranial electrical stimulation as a tool to enhance attention. J. Cogn. Enhanc. 1, 10–25. doi: 10.1007/s41465-017-0010-y
Schieber, F., and Gilland, J. (2008). Visual entropy metric reveals differences in drivers’ Eye Gaze complexity across variations in age and subsidiary task load. Proc. Hum. Factors Ergon. Soc. Annu. Meet. 52, 1883–1887. doi: 10.1037/e578302012-012
Seger, C. A., and Cincotta, C. M. (2006). Dynamics of frontal, striatal and hippocampal systems during rule learning. Cereb. Cortex 16, 1546–1555. doi: 10.1093/cercor/bhj092
Seger, C. A., Poldrack, R. A., Prabhakaran, V., Zhao, M., Glover, G. H., Gabrieli, J. D., et al. (2000). Hemispheric asymmetries and individual differences in visual concept learning as measured by functional MRI. Neuropsychologia 38, 1316–1324. doi: 10.1016/s0028-3932(00)00014-2
Serences, J. T., and Boynton, G. M. (2007). Feature-based attentional modulations in the absence of direct visual stimulation. Neuron 55, 301–312. doi: 10.1016/j.neuron.2007.06.015
Shacham, S. (1983). A shortened version of the profile of mood states. J. Pers. Assess. 47:305. doi: 10.1207/s15327752jpa4703_14
Shah, C., Erhard, K., Ortheil, H.-J., Kaza, E., Kessler, C., Lotze, M., et al. (2013). Neural correlates of creative writing: an FMRI Study. Hum. Brain Mapp. 34, 1088–1101. doi: 10.1002/hbm.21493
Spreng, R. N., Sepulcre, J., Turner, G. R., Stevens, W. D., and Schacter, D. L. (2012). Intrinsic architecture underlying the relations among the default, dorsal attention and frontoparietal control networks of the human brain. J. Cogn. Neurosci. 25, 74–86. doi: 10.1162/jocn_a_00281
Theeuwes, J. (2010). Top-down and bottom-up control of visual selection. Acta Psychol. 135, 77–99. doi: 10.1016/j.actpsy.2010.02.006
Theeuwes, J. (2018). Visual selection: usually fast and automatic; seldom slow and volitional. J. Cogn. 1:29. doi: 10.5334/joc.13
Torralba, A., Oliva, A., Castelhano, M. S., and Henderson, J. M. (2006). Contextual guidance of eye movements and attention in real-world scenes: the role of global features in object search. Psychol. Rev. 113, 766–786. doi: 10.1037/0033-295X.113.4.766
Tuddenham, W. J. (1962). Visual search, image organization and reader error in roentgen diagnosis. Radiology 78, 694–704. doi: 10.1148/78.5.694
Vine, S. J., Moore, L. J., and Wilson, M. R. (2016). An integrative framework of stress, attention and visuomotor performance. Front. Psychol. 7:1671. doi: 10.3389/fpsyg.2016.01671
Waite, S., Grigorian, A., Alexander, R. G., Macknik, S. L., Carrasco, M., Heeger, D. J., et al. (2019). Analysis of perceptual expertise in radiology-current knowledge and a new perspective. Front. Hum. Neurosci. 13:213. doi: 10.3389/fnhum.2019.00213
Wang, D., Kristjansson, A., and Nakayama, K. (2005). Efficient visual search without top-down or bottom-up guidance. Percept. Psychophys. 67, 239–253. doi: 10.1007/s13304-020-00968-3
Wilson, M., Smith, N. C., Chattington, M., Ford, M., and Marple-Horvat, D. E. (2006). The role of effort in moderating the anxiety-performance relationship: testing the prediction of processing efficiency theory in simulated rally driving. J. Sports Sci. 24, 1223–1233. doi: 10.1080/02640410500497667
Wolfe, J. M., and Horowitz, T. S. (2017). Five factors that guide attention in visual search. Nat. Human Behav. 1:0058. doi: 10.1038/s41562-017-0058
Wolfe, J. M., Butcher, S. J., Lee, C., and Hyle, M. (2003). Changing your mind: on the contributions of top-down and bottom-up guidance in visual search for feature singletons. J. Exp. Psychol. Hum. Percept. Perform. 29, 483–502. doi: 10.1037/0096-1523.29.2.483
Keywords: tDCS, brain stimulation, attention, learning, anxiety, individual differences, perception, visual learning
Citation: Gibson BC, Heinrich M, Mullins TS, Yu AB, Hansberger JT and Clark VP (2021) Baseline Differences in Anxiety Affect Attention and tDCS-Mediated Learning. Front. Hum. Neurosci.15:541369. doi: 10.3389/fnhum.2021.541369
Received: 08 March 2020; Accepted: 03 February 2021;
Published: 03 March 2021.
Edited by:
Björn H. Schott, Leibniz Institute for Neurobiology (LG), GermanyReviewed by:
Enrica Laura Santarcangelo, University of Pisa, ItalyDaniel Keeser, Ludwig Maximilian University of Munich, Germany
Copyright © 2021 Gibson, Heinrich, Mullins, Yu, Hansberger and Clark. This is an open-access article distributed under the terms of the Creative Commons Attribution License (CC BY). The use, distribution or reproduction in other forums is permitted, provided the original author(s) and the copyright owner(s) are credited and that the original publication in this journal is cited, in accordance with accepted academic practice. No use, distribution or reproduction is permitted which does not comply with these terms.
*Correspondence: Vincent P. Clark, dmNsYXJrQHVubS5lZHU=