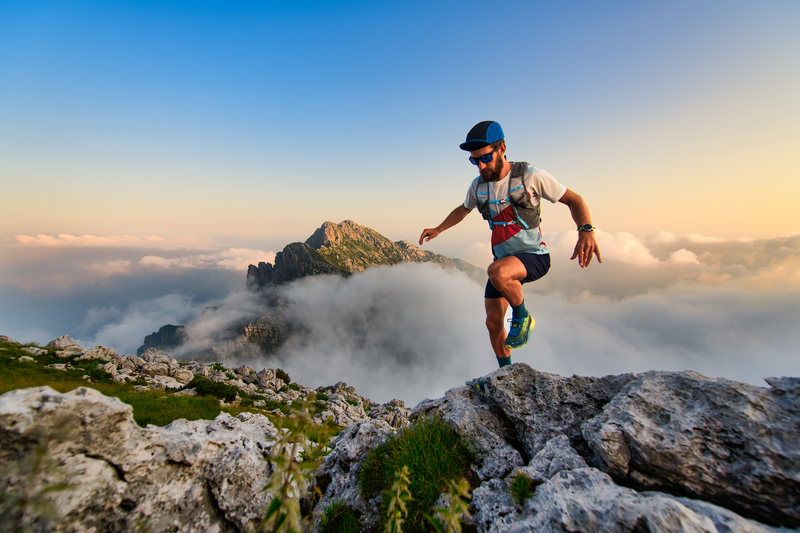
94% of researchers rate our articles as excellent or good
Learn more about the work of our research integrity team to safeguard the quality of each article we publish.
Find out more
BRIEF RESEARCH REPORT article
Front. Hum. Dyn. , 24 December 2024
Sec. Digital Impacts
Volume 6 - 2024 | https://doi.org/10.3389/fhumd.2024.1495270
This article is part of the Research Topic #breakthebias: Working Towards Alternative Ways of Being in a Digital World Through Conversations With Critical Friends, Texts, and Technologies View all 4 articles
Text generation by artificial intelligence became available to a broader public, latterly. This technology is based on machine learning and language models that need to be trained with input data. Many studies have focused on the distinction of human-written text. vs. generated texts but recent studies show that the underlying language models might be prone to reproduce gender bias in their output and, consequently, reinforcing gender roles and imbalances. In this paper, we give a perspective on this topic, considering both the generated text data itself and the machine learning models used for language generation. We present a case study of gender bias in generated text data and review recent literature addressing language models. Our results indicate that researching gender bias in the context of text generation faces significant challenges and that future work needs to overcome a lack of definitions as well as a lack of transparency.
With artificial intelligence becoming increasingly popular in recent times, their mainstream usage for writing texts has also increased. With large providers like ChatGPT and Gemini users have direct access to language models and machine learning methods for text generation and these have been objects of research in many fields, including natural language processing and computer science. Most studies focus on investigating the distinction of human-created and AI-generated texts (Bolukbasi et al., 2016). Measuring gender bias especially at large scale in output texts of generative AIs and their language models is still a rather new discipline. However, numerous studies have shown how gender bias in technology may lead to exclusion, making this topic worth of investigating (Stumpf et al., 2020). There are two views of perspective on gender bias in generated texts: First, the texts produced by artificial intelligence can be studied to shed light on the used language. Second, the language models that are used by artificial intelligence to generate texts can be studied to gain insight into the impact and predictability of gender bias.
In the literature, there are two different concepts of gender bias. The first understanding aims to establish a balance of gender-assigned terms in texts, meaning a similar amount of female-assigned and male-assigned words. The goal is that both genders are represented to a similar extent. The second understanding is the establishment of gender-neutral terms instead of gender-assigned terms. The goal is to use words that do not give an indication of gender. While a balance between male- and female-assigned terms may be assessed using counters or statistical methods, gender-neutral terms are less standardized and, thus, often excluded in studies on gender-assigned language (Vergoossen et al., 2020).
In this paper, we aim to give a two-fold perspective on gender bias in generated texts. First, we bring attention to the generated data itself and conduct a brief study on term occurrence. Second, we review literature on methods to measure and influence text generation models. Both views indicate a lack of standardized definitions and benchmarks, needed for thorough analyses. Nevertheless, promising approaches to reduce gender bias in generated texts exist.
Before shedding light on the models used for text generation, we investigate the generated text data itself regarding possible gender bias. For getting a first impression of the impact of gender bias in generated text data using artificial intelligence, we conduct a case study utilizing a frequency-based approach.
In this study, we recognize words that are defined as gender-assigned vs. gender-neutral in existing text corpora. Although there are numerous guidelines for gender-neutral language, e.g., by the European Parliament (Parliament, 2018) that began to encourage the use of inclusive and neutral language in 2008, these documents usually do not provide comprehensive lists of words that represent a specific gender and words that are deemed gender-neutral. Hence, authors who want to use gender-neutral language have to rely on different dictionaries and sources, and often on their intuition.
To lay a solid foundation for our case study, we utilize the following two sources of terms:
• The dictionary of gender-assigned and gender-neutral terms by Butler (2023a), including terms that are gender-assigned and gender-neutral terms.
• The dictionary of gender-neutral language published by Pronouns.page, also including gender-assigned and gender-neutral terms (Pronouns.page, 2024).
We scraped the websites of these dictionaries and extracted extracted the words together with a label describing each word as gender-neutral, female-assigned, or male-assigned. In combination, these dictionaries provide 764 gender-assigned words with almost the same share of male-assigned and female-assigned terms, and 1,415 neutral terms that do not represent a specific gender. The first dictionary also includes 431 terms that are neutral in terms of the Human Gender-Neutral Language (HGNE) standard and 181 terms that are discouraged by that standard, also known as Universal Gender-Neutral English (UGNE) standard (Butler, 2023b).
For the case study, we investigate three different corpora containing of AI-generated texts and compare these to a reference corpus of human-written texts. All texts we used are written in English.
Corpus A The first corpus that we used in the case study consists of 109 text generated by ChatGPT (Sotov, 2024a). It has been used to develop a detector able to classify texts that have been generated by ChatPGT (Sotov, 2024b). While distinguishing generated and human-written texts is a significant task, out study focuses on the language of these texts by investigating the use of gender-related terms.
Corpus B The second corpus contains generated essays and has been copmrised for a large-scale comparison of human-written and generated texts (Herbold et al., 2023). The 90 essays were rated by teachers as human experts and its data is available in the corresponding repository (Herbold, 2023). For our analysis, we use the generated texts of this corpus and analyze gender-related terms occurrences.
Corpus C The largest corpus holds answers generated by ChatGPT to questions ranging from open-domain, financial, medical, legal, and psychological areas. It has been built to tell apart human experts from artificial intelligence and is called the Human ChatGPT Comparison Corpus (HC3) (Guo et al., 2023). In total, it contains 26,903 AI-generated texts in English that we use for our case study.
Corpus H For reference, we also analyze a corpus of human-written texts. The Human ChatGPT Comparison Corpus (HC3) does not only contain generated texts but also answers to questions from open-domain, financial, medical, legal, and psychological areas that have been written by humans (Guo et al., 2023). We use the included 58,546 human-written texts for comparison in our analysis.
To gain insights into the use of gender-related terms in generated texts, we analyze the corpora on a term-level, in contrast to previous work that has targeted whole sentences to assign gender-labels (Kaneko et al., 2022). After lemmatization, gender-assigned terms are counted for male and female forms of these terms as well as gender-neutral terms. We then calculate the following scores:
Figure 1 shows the results of these calculations for each corpus as a colored heatmap, with the red end of the scale representing the use of gender-assigned terms and the green end of the scale representing the use of gender-neutral terms or the avoidance of gender-assigned terms. To enable a cross-corpus comparison, the colors scale is applied row-wise.
The two smaller corpora A and B both contain more female-assigned words than male-assigned words. In contrast, the larger corpora of generated texts (C) and human-written texts (H) both show a higher frequency of male-assigned terms over female-assigned terms.
All corpora have a higher neutral words share than gender-specific words share including both male-assigned and female-assigned terms. However, we cannot deduce that neutral terms are used to avoid gender-bias specifically. While all generated corpora contain “individuals” as a neutral term to describe persons of all genders, the dictionaries also list terms like “you” and “god” as neutral terms. Although these are deemed neutral, they are not meant as alternatives for a gender-specific term. Furthermore, the dictionaries define in total more neutral words than gender-assigned words. With this in mind, it seems plausible that the use of neutral terms outweighs the use of gender-assigned terms.
In a direct frequency comparison, there is no clear picture of the tendency of the occurrences of male-assigned terms in relation to female-assigned terms in the generated texts. For corpora A and B, the results indicate that ChatGPT might use more female-assigned words, e.g., in student essays. But corpus C shows a clear outweigh of male-assigned terms over female-assigned terms. The male-female ratio of corpus H, the human-written texts, lies in between the AI-generated texts corpora.
For male-to-neutral ratio, neutral terms are higher represented in the generated texts than male-assigned terms for all generated corpora. In comparison, the human-written texts seem to include more male-assigned terms than the generated texts, measured in the ratio to neutral terms. In contrast, female-assigned terms seem to be used more frequently measured in ratio to neutral terms in the smaller corpora A and B than in the larger corpus C.
Finally, the ratio of neutral terms to discouraged terms due to the HGNE/UGNE standard gives a mixed result. It is important to note that these defined terms occurred only rarely. The most often found term of that standard, servant, was found 8 times in all corpora. This raises the question whether the terms of this dictionary are suitable at all to describe gender-related tendencies in generated texts.
The overall results demonstrate the difficulties of measuring gender bias in generated texts. While the two smaller corpora show a tendency to include female-assigned terms, the larger corpus and the human-written texts tend to use more male-assigned terms. Measuring the representation of gender-neutral depends on the used dictionaries and definitions.
From this data, we cannot observe clear evidence of the presence or absence of a gender-bias in AI-generated texts. First, the data available is limited. Our case study included different corpora and especially the HC3 corpus is sufficiently large, but the dictionaries of gender-related and gender-neutral terms are not comprehensive and may follow different definitions. Especially the HGNE/UGNE dictionary underperformed in detecting relevant terms and includes terms that are not well-defined, e.g., mother nature as a female-assigned term. Furthermore, the impact of the texts' topics remains unclear. For further research, texts of specific topical areas should be compared to avoid a mix of topic-specific words throughout corpora.
Ultimately, the highest hurdle in the investigations of gender bias in generated texts is the absence of clear definitions and standards. In practice, guidelines describe the problem of gender bias bud do not give a well-defined dictionary or comprehensive list of terms that are assigned to one gender or terms that are commonly agreed upon to be gender-neutral. For investigating generated texts at a large scale, such a catalog is required but it seems challenging to achieve completeness.
The identified limitations of investigating gender bias in generated texts also seem to exist for the perspective on generative models. Nevertheless, the idea is to train the underlying language model of a generative AI in such way that gender-neutral terms are used as a default and gender-assigned terms should be avoided. This has been a research subject in several previous works that will be described in the following to give the perspective on gender bias in generative models.
Vig et al. (2020) introduce a causal mediation analysis framework for interpreting NLP models, which is then applied to analyze gender bias in pre-trained transformer language models. The used causal mediation analysis aims to understand how information flows through different model components, such as neurons, and how this affects the model's outputs. The authors test two types of interventions: set-gender, which replaces ambiguous professions with anti-stereotypical gender-specific words, and null, which leaves the input unchanged. To assess the impact, the total effect, natural direct effect, and natural indirect effect of the interventions on the model's gender bias are measured. These effects are described to understand how different model components mediate the gender bias. The study utilizes three datasets designed to gauge a model's sensitivity to gender bias in order to analyze gender bias in pre-trained transformer language models using causal mediation analysis.
Gupta et al. (2022) proposes an approach to mitigate gender bias in text generation from language models during knowledge distillation by using counterfactual role reversal, which involves modifying the teacher model's probabilities and augmenting the training set with counterfactual data. In a first step, counterfactual sequences are generated by swapping gendered words in the original text. Then, a combination of the original and counterfactual token probabilities is made in several ways, e.g., max, mean, expMean, swap, to obtain a more equitable teacher model. The training data is then enriched with the counterfactual sequences in addition to modifying the teacher probabilities and influence the language model used for text generation. The original GPT-2 language model was used as the starting point for the knowledge distillation and fine-tuning process.
Lu et al. (2020) examine gender bias in neural natural language processing systems and define a benchmark to quantify this bias. They propose counterfactual data augmentation (CDA) to mitigate the bias while preserving model accuracy. The introduced benchmark to quantify gender bias in neural NLP tasks is based on causal testing of matched pairs and could be used for language modeling tasks. Exploring CDA, word embedding debiasing (WED) is investigated as technique to mitigate gender bias. The authors also comprise a corpus that was augmented by the proposed CDA.
Kaneko et al. (2022) propose a multilingual bias evaluation (MBE) score to evaluate gender bias in masked language models (MLMs). As a strong benefit, this process does not require manual annotation of data. For this approach, the authors extract female-assigned and male-assigned sentences from a parallel corpus using a gender word lists and calculate the likelihoods of the extracted gender-assigned sentences using the target MLM. Then, the likelihoods of female- and male-assigned sentences are calculated using the all unmasked likelihood with attention weights (AULA) method, and compared to compute a bias score based on the percentage of male sentences with higher likelihoods.
Bordia and Bowman (2019) develop a metric to measure gender bias in a recurrent neural network language model and propose a regularization loss term that minimizes the projection of encoder-trained embeddings. These methods are based on word occurrences, requiring a dictionary of gender-related terms, and establishing a relation between male-assigned and female-assigned words in context windows. The authors evaluate the proposed method on three different text corpora: Penn Treebank, WikiText-2, and CNN/Daily Mail and make use of a standard LSTM-based language model architecture, the AWD-LSTM, as the base model for their experiments.
de Vassimon Manela et al. (2021) propose a new metrics to quantify gender bias in language models with a focus on male-assigned pronouns and stereotypical use of pronouns. The authors define two new metrics to quantify gender bias in language models: skew as the overall preference for male pronouns and stereotype as the assignment of pronouns to stereotypical professions. They measure the gender bias of various pre-trained language models (BERT, RoBERTa, ALBERT, etc.) using the WinoBias dataset. As approach for mitigation of gender bias effects, a data augmentation method to fine-tune BERT models is applied with the goal to reduce both skew and stereotype bias.
Qian et al. (2019) introduce a specialized loss function to mitigate gender bias in neural language models utilizing pre-trained GloVe word embeddings. The new loss term aims to equalize the predicted probabilities of gender-paired words, combined with the standard cross-entropy loss. Additionally, counterfactual data augmentation (CDA) is applied to expand the training corpus by swapping gender pairs, similar to the work done by Lu et al. (2020) The authors perform a tuning of the used LSTM language model with specific hyperparameters and use a sub-sample of 5% of the Daily Mail news article dataset for training. They also utilize the bias regularization method introduced by Bordia and Bowman (2019) which debiases the word embedding during language model training.
Vig et al. (2020) state that larger language models are more sensitive to gender bias, with the effect saturating at the largest model sizes. Gender bias effects could mainly be observed in a small subset of the model's middle layers and only a small fraction of the model is responsible for the majority of the gender bias effects. The authors acknowledge a limited setup and constructed templates due to the difficulties of assessing complete gender bias phenomena in language and language models.
Vig et al. (2020) find the approach of using counterfactual role reversal (CRR) during knowledge distillation capable of reducing gender disparity in the generated text. However, they acknowledge reducing gender disparity in text generation does not necessarily improve embedding fairness or overcomes gender bias in the generated data. Both introduced methods to mitigate gender bias in distilled language models reduced gender disparity in text generation, but did not consider gender-neutral terms.
Lu et al. (2020) attest that NLP systems exhibit significant gender bias, particularly regarding occupations. Optimization processes of language models could encourage the growth of gender bias in progressing training. Word embedding debiasing techniques is able to reduce this bias, but the residual bias is still considerable and may result in a drop in accuracy. In contrast, the counterfactual data augmentation (CDA) effectively decreases gender bias while preserving accuracy in their experiments, and outperforms word embedding debiasing.
The multilingual bias evaluation (MBE) method proposed by Kaneko et al. (2022) is able to measure gender bias in MLMs across multiple languages, showing high correlation with human bias annotations. MBE especially outperforms methods that use machine translation to evaluate bias in non-English languages. This shows that gender bias exists in MLMs across different languages studied.
The method to reduce gender bias in language models by Bordia and Bowman (2019) was found to be effective up to an optimal weight for the loss term, beyond which the model became unstable with increased perplexity. Furthermore, this approach also relies on word lists and dictionaries as additional limitation.
de Vassimon Manela et al. (2021) find in their study that there is a trade-off between gender stereotype and gender skew in pre-trained language models. The applied fine-tuning of BERT with a gender-balanced dataset can reduce both gender skew and stereotype compared to fine-tuning on the original dataset. This is in line with other mentioned papers, stating that countering an overweight of male-assigned terms, words or sentences may be countered by balancing the data, e.g., by adding counterfactual terms, words, or sentences. However, it is acknowledged that current gender bias benchmarks do not fully capture gender bias, especially regarding professions, as pronoun resolution may be influenced by other forms of gender prejudice beyond just professional stereotypes.
The developed loss function by Qian et al. is capable of influencing the output of a language model by equalizing the probabilities of male-assigned and female-assigned words. The authors show that their new loss function effectively reduces gender bias during model training without explicitly addressing the bias in the embedding layer. In the conducted experiments, the approach outperforms debiasing methods like data augmentation and word embedding debiasing and it is suggested to combine the approach with data augmentation for further improvement in reducing the bias. Still, the proposed method may have similar limitations as other debiasing strategies, especially in aspects beyond reducing gender bias in occupation words. The authors also acknowledge that the effectiveness of reducing gender bias may be limited to the specific bias evaluation metrics used, and that further research could explore other metrics (Qian et al., 2019).
In summary, several approaches have been made to benchmark and influence effects of gender bias in language models under certain limitations. There are strong indications of gender bias in language models, even for different languages. While balancing gender-assigned terms counterfactually may reduce the overbalance of a specific gender in generated texts, it fails encouraging the use of gender-neutral terms. Additionally, language models may be influenced, e.g., by loss functions, to favor a balance of gender-assigned words in a generated text but if such an influence is implemented in a model's training remains unclear in practice.
Both views on the generated text data and on the generation models shed light on certain gap in the research field. Measuring gender bias in generated texts is seems to be a significant challenge, especially due to missing consistent word lists and insufficient data of gender-neutral terms. Generally, there exists a lack of definitions and standards that needs to be overcome to enable thorough future studies and apply statistical methods for gender bias analyses beyond comparing female-assigned and male-assigned words. Furthermore, the majority of research investigates English texts while other languages, e.g., German, may be more challenging regarding gender-neutral terms. Future research might also dive into different corpora of texts with a dedicated feminist stance, for instance, Feminist HCI work (Bellini et al., 2018; Chivukula and Gray, 2020).
For language models used to generate texts, counterfactual methods enable a balancing of gender-assigned terms and seem to be a valid way of mitigating gender bias in terms of one gender outweighing another regarding used words. However, measuring the effect and outcome of methods aiming at reducing gender-assigned language and encouraging gender-neutral terms is still a significant challenge. This goal seems to be underrepresented in current research as it requires additional definitions and dictionaries that are yet to be made. Closing this gap is crucial for proceeding with future research on gender-neutral text generation. Nevertheless, approaches to improve generative AI models exist while their enforcement and implementation is unclear in practice and users may never know whether or not the language model of a text generator AI is set to mitigate gender bias. Hence, transparency and explainability of machine learning methods used for text generation is essential for future research of gender bias in language models while word-level analyses may generally neglect contextual gender bias like subtle reinforcement of traditional roles, imbalanced representation, or implicit expectations about gendered behavior.
The original contributions presented in the study are included in the article/supplementary material, further inquiries can be directed to the corresponding author/s.
TH: Conceptualization, Data curation, Investigation, Methodology, Validation, Visualization, Writing – original draft, Writing – review & editing.
The author(s) declare that no financial support was received for the research, authorship, and/or publication of this article.
The author declares that the research was conducted in the absence of any commercial or financial relationships that could be construed as a potential conflict of interest.
All claims expressed in this article are solely those of the authors and do not necessarily represent those of their affiliated organizations, or those of the publisher, the editors and the reviewers. Any product that may be evaluated in this article, or claim that may be made by its manufacturer, is not guaranteed or endorsed by the publisher.
Bellini, R., Strohmayer, A., Alabdulqader, E., Ahmed, A. A., Spiel, K., Bardzell, S., et al. (2018). “Feminist HCI: taking stock, moving forward, and engaging community,” in Extended Abstracts of the 2018 CHI Conference on Human Factors in Computing Systems, 1–4. doi: 10.1145/3170427.3185370
Bolukbasi, T., Chang, K.-W., Zou, J. Y., Saligrama, V., and Kalai, A. T. (2016). “Man is to computer programmer as woman is to homemaker? Debiasing word embeddings,” in Advances in Neural Information Processing Systems, 29.
Bordia, S., and Bowman, S. R. (2019). Identifying and reducing gender bias in word-level language models. arXiv preprint arXiv:1904.03035.
Butler, S. C. (2023a). Dictionary of gender-assigned and gender-neutral terms. Available at: https://shawncbutler.com/2023/02/07/gendered-english-words/#List_of_Gendered_English_Words (accessed August 28, 2024).
Butler, S. C. (2023b). Universal gender neutral english (the standard). Available at: https://shawncbutler.com/2023/01/29/humanist-gender-neutral-english/ (accessed August 28, 2024).
Chivukula, S. S., and Gray, C. M. (2020). “Bardzell's" feminist HCI" legacy: analyzing citational patterns,” in Extended Abstracts of the 2020 CHI Conference on Human Factors in Computing Systems, 1–8. doi: 10.1145/3334480.3382936
de Vassimon Manela, D., Errington, D., Fisher, T., van Breugel, B., and Minervini, P. (2021). “Stereotype and skew: quantifying gender bias in pre-trained and fine-tuned language models,” in Proceedings of the 16th Conference of the European Chapter of the Association for Computational Linguistics: Main Volume, 2232–2242. doi: 10.18653/v1/2021.eacl-main.190
Guo, B., Zhang, X., Wang, Z., Jiang, M., Nie, J., Ding, Y., et al. (2023). How close is CHATGPT to human experts? comparison corpus, evaluation, and detection. arXiv preprint arXiv:2301.07597.
Gupta, U., Dhamala, J., Kumar, V., Verma, A., Pruksachatkun, Y., Krishna, S., et al. (2022). Mitigating gender bias in distilled language models via counterfactual role reversal. arXiv preprint arXiv:2203.12574.
Herbold, S. (2023). sherbold/chatgpt-student-essay-study: v1.1. Available at: https://doi.org/10.5281/zenodo.8343644
Herbold, S., Hautli-Janisz, A., Heuer, U., Kikteva, Z., and Trautsch, A. (2023). A large-scale comparison of human-written versus chatgpt-generated essays. Sci. Rep. 13:18617. doi: 10.1038/s41598-023-45644-9
Kaneko, M., Imankulova, A., Bollegala, D., and Okazaki, N. (2022). Gender bias in masked language models for multiple languages. arXiv preprint arXiv:2205.00551.
Lu, K., Mardziel, P., Wu, F., Amancharla, P., and Datta, A. (2020). “Gender bias in neural natural language processing,” in Logic, Language, and Security: Essays Dedicated to Andre Scedrov on the Occasion of his 65th birthday, eds. V. Nigam, T. B. Kirigin, C. Talcott, J. Guttman, S. Kuznetsov, B. T. Loo et al. (Cham: Springer), 189–202. doi: 10.1007/978-3-030-62077-6_14
Parliament, E. (2018). Gender-neutral languagein the european parliament. Available at: https://www.europarl.europa.eu/cmsdata/151780/GNL_Guidelines_EN.pdf (accessed August 28, 2024).
Pronouns.page (2024). Dictionary of gender-neutral language. Available at: https://en.pronouns.page/dictionary (accessed August 28, 2024).
Qian, Y., Muaz, U., Zhang, B., and Hyun, J. W. (2019). Reducing gender bias in word-level language models with a gender-equalizing loss function. arXiv preprint arXiv:1905.12801.
Sotov, A. (2024a). Chatgpt corpus. Available at: https://github.com/roverbird/chatgpt_corpus (accessed August 28, 2024).
Sotov, A. (2024b). The intricate tapestry of chatgpt texts: why llm overuses some words at the expense of others? Available at: https://textvisualization.app/blog/intricate-tapesty-of-chatgpt-texts/ (accessed August 28, 2024).
Stumpf, S., Peters, A., Bardzell, S., Burnett, M., Busse, D., Cauchard, J., et al. (2020). Gender-inclusive HCI research and design: a conceptual review. Found. Trends Hum.-Comput. Inter. 13, 1–69. doi: 10.1561/1100000056
Vergoossen, H. P., Renström, E. A., Lindqvist, A., and Gustafsson Sendén, M. (2020). Four dimensions of criticism against gender-fair language. Sex Roles 83, 328–337. doi: 10.1007/s11199-019-01108-x
Keywords: gender bias, generative artificial intelligence, text generation, language models, machine learning
Citation: Hupperich T (2024) A perspective on gender bias in generated text data. Front. Hum. Dyn. 6:1495270. doi: 10.3389/fhumd.2024.1495270
Received: 12 September 2024; Accepted: 10 December 2024;
Published: 24 December 2024.
Edited by:
Debora De Castro Leal, University of Siegen, GermanyReviewed by:
Pragya Lodha, Lokmanya Tilak Municipal General Hospital, IndiaCopyright © 2024 Hupperich. This is an open-access article distributed under the terms of the Creative Commons Attribution License (CC BY). The use, distribution or reproduction in other forums is permitted, provided the original author(s) and the copyright owner(s) are credited and that the original publication in this journal is cited, in accordance with accepted academic practice. No use, distribution or reproduction is permitted which does not comply with these terms.
*Correspondence: Thomas Hupperich, dGhvbWFzLmh1cHBlcmljaEB1bmktbXVlbnN0ZXIuZGU=
Disclaimer: All claims expressed in this article are solely those of the authors and do not necessarily represent those of their affiliated organizations, or those of the publisher, the editors and the reviewers. Any product that may be evaluated in this article or claim that may be made by its manufacturer is not guaranteed or endorsed by the publisher.
Research integrity at Frontiers
Learn more about the work of our research integrity team to safeguard the quality of each article we publish.