- 1New South Wales Department of Primary Industries, Biosecurity and Food Safety, Elizabeth Macarthur Agricultural Institute, Menangle, NSW, Australia
- 2Microbial Sciences, Pests and Diseases, Agriculture Victoria, AgriBio, Bundoora, VIC, Australia
- 3School of Applied Systems Biology (SASB), La Trobe University, Bundoora, VIC, Australia
High-throughput sequencing (HTS) technologies have revolutionized plant virology through simultaneous detection of mixed viral infections. HTS advances have uncovered and improved understanding of virus biology, ecology, and evolution which is vital for viral disease management. Plant viruses continue to threaten global agricultural productivity and strict quarantine measures are essential to prevent the introduction and spread of virulent viruses around the world. The gradual decrease in HTS operational costs, including improved computational systems and automation through robotics, has facilitated the adoption of this tool for plant diagnostics, including its use in surveillance and quarantine programs. However, the speed of technology advancements and distinct HTS chemistries, laboratory procedures, data management, and bioinformatic analyses have proven challenging. In addition, the lack of viral species reference sequences, compared with the estimated number of distinct viral taxa, makes classification and identification of novel viruses difficult. There is a need for standardized HTS testing, especially within plant health programs. In this review, we consider the application of HTS in plant virology, explore the technical challenges faced and the opportunities for HTS in plant health certification. We propose standards for overcoming current barriers and for ensuring reliable and reproducible results. These efforts will impact global plant health by reducing the risk of introduction and the spread of damaging novel viruses.
Introduction
As the world’s population continues to expand, global food security presents a fundamental challenge for the twenty-first century (Potapov et al., 2022; Campbell-Lendrum et al., 2023: Kimotho and Maina 2024). Minimizing crop loss to disease is pivotal for reaching the 60% increase in food production needed to satisfy future global nutritional requirements (Jones and Naidu, 2019). Comprehensive genome studies will inform the development of accurate diagnostic assays which in turn aids the development of effective and innovative management strategies for plant pathogens (Hardwick et al., 2019; Gullino et al., 2021). Plant viruses make up almost half of the pathogens responsible for emerging and re-emerging crop diseases worldwide, causing damaging disease in both natural vegetation and agricultural cultivated plants (Thresh, 2006; Anderson et al., 2004; Jones, 2009). Globally, plant viruses have an estimated economic impact of greater than $30 billion annually (Sastry et al., 2014). This may continue to rise due to climate change coupled with increasing globalization which facilitates crop pathogen movement and establishment (Gullino et al., 2021). With limited options available to control the damage caused by new viral pathogens, successful eradication or management relies on rapid identification of the threat.
Historically, plant viruses were identified by host symptoms, transmission to indicator plants and microscopy. However, the discovery of serological and molecular detection tools, coupled with Sanger sequencing, improved our understanding of plant viruses, including their host range and vectors (Timian, 1974). Integrating rapid innovations, such as cutting-edge high-throughput sequencing (HTS), offers unprecedented breakthroughs in the discovery and characterization of new damaging plant viruses (Wren et al., 2006; Roossinck et al., 2015; Song et al., 2019; Maina et al., 2021; Maina and Jones, 2023). HTS application in natural and none cultivated crop communities has been significantly explored to determine viral diversity, new variants, emerging future threat species, and identification of unknown hosts of known viruses (Wylie and Jones, 2011; Roossinck et al., 2015; Maina et al., 2016a, Maina et al., 2016b – Maina et al., 2016f; Massart et al., 2017; Wamaitha et al., 2018; Nancarrow et al., 2019a). Notably, HTS has contributed to a greater understanding of genetic diversity, molecular and biological interactions, hence informing disease management practices (Magliogka et al., 2018; You et al., 2021). The most effective way to limit the spread of pathogens, such as viruses, is by regulating the movement of plants and plant products through phytosanitary systems (Gullino et al., 2021). HTS has been applied extensively in seed and vegetative propagules virus testing, and its deployment in international phytosanitary border zones could prevent the introduction of damaging viruses into new geographic cropping regions or natural ecosystems (Maliogka et al., 2018; Maina et al., 2021; Bester et al., 2022, Maina et al., 2022, Maina et al., 2023).
The significant potential of HTS for plant health certification and targeted border surveillance has not yet been fully realized. Effective management of quarantine viral pathogens requires fast, sensitive, and cost-effective diagnostic tests that not only detect viruses but also distinguish between specific genotypes. Several studies have been published documenting efforts to improve and streamline HTS analysis in pursuit of a one-stop-shop solution for diagnosis (Yamashita et al., 2016; Barrero et al., 2017; Rott et al., 2017; Massart et al., 2019). Nevertheless, constant advancements in sequencing chemistry and technologies pose challenges for harmonizing wet lab procedures, data management and analysis, and subsequent biological interpretation. The technical aspects of generating reliable HTS data are described in this review, as well as the evolution of HTS, the challenges faced, and the opportunities for HTS in plant health certification.
Overview of high-throughput sequencing approaches in plant virus diagnostics
Plant virus diagnostic techniques have evolved with greater adoption of HTS, which involve sequencing of the total nucleic acid followed by bioinformatics (Adams et al., 2009; Maree et al., 2018). HTS has proven to be reliable and highly sensitive for virus detection and discovery (Al Rwahnih et al., 2009; Maina et al., 2016c; Maina et al., 2016d; Maina et al., 2016e; Maina et al., 2017d; Maina et al., 2017e; Maina and Jones, 2017g; Maina et al., 2019b), and when combined with biological information, has successfully resolved many disease complexes in agricultural and horticultural crops around the world (Maree et al., 2018; Wamaitha et al., 2018; Mbeyagala et al., 2019). In plant health diagnostics, the gold standards utilized by plant quarantine programs globally are biological indexing using indicator plants, serology, PCR, and electron microscopy. However, these detection methods require prior knowledge of viral pathogens for accurate detection, creating challenges for detecting novel viruses, emerging genetic variants and mixed infections. Over the last two decades, HTS technologies have evolved and are generally now defined as second and third generation. These platforms are categorized according to their detection technology and sequencing chemistry. When selecting a platform for plant virus research and diagnostics, it is critical to consider the size, genetic structure, genome complexity, and G+C content of the target or suspected virus, as well as the depth coverage and read accuracy needed. Past and available technologies are discussed below.
Roche 454
This was the first HTS platform, widely used after its release in 2005 by Life Sciences and later acquired by Roche in 2007 (Yee and Tapani, 2017). Its sequencing chemistry involves fixing nebulized and adapter-ligated DNA fragments to small DNA-capture beads in a water-in-oil emulsion, which are then loaded into the wells of a picotiter plate for amplification by emulsion PCR and finally sequenced through pyrosequencing (Rothberg and Leamon, 2008). The 454 chemistry gradually became noncompetitive and was discontinued. Nevertheless, this platform played a critical role in detecting virus outbreak, e.g. maize chlorotic mottle virus and a variant of sugarcane mosaic virus, a complex associated with the deadly disease of maize lethal necrosis (Adams et al., 2013).
Illumina
It is based on a sequencing by synthesis strategy that uses a fluorescently labeled dye-terminator and clonal amplification, where the adaptor ligates DNA fragments on a flow cell (Illumina, Bentley et al., 2008). The Illumina platform consists of a variety of instruments and is the most widely used technology today, providing high throughput with a low error rate. The MiSeq platform, for instance, is a small, benchtop platform that produces 1.5 Gbp per run in about 10 hours, and has a maximum paired-end read length of 300 bp. The latest widely adopted Illumina release (NovaSeq systems series) can deliver up to 20 billion reads per run, with a maximum paired read length of 150 bp and the ability to multiplex thousands of samples. The Illumina reversible terminator–based methods enable the parallel sequencing of billions of DNA fragments, detecting single bases as they are added to growing DNA strands (Illumina). This significantly reduces errors and biased calls associated with homopolymers. Due to its sufficient sequencing coverage and low error rates, Illumina has been widely used for viral research and diagnosis, leading to numerous research publications on its application for sensitive characterization of low and high-frequency variation within plant virus populations.
Solid
Solid (Applied Biosystems) was originally released in 2007 by Life Technologies. This system uses DNA ligases followed by repeating cycles of ligation with fluorescently labeled probes and clean-up of non-ligated probes prior to imaging (Valouev et al., 2008). Solid provides high throughput data but has a limitation of < 100 bp read lengths and a long sequencing time (Barba et al., 2014), which may create difficulties and increase the cost associated with subsequent virus bioinformatic analyses.
Ion Torrent
Ion Torrent Personal Genome Machine technology (Life Technologies) involves the amplification of an adaptor-ligated DNA fragment by exploiting emulsion PCR. It also incorporates a sequencing-by-synthesis approach, which uses native dNTP chemistry and relies on a modified silicon chip to detect hydrogen (Life Technologies). The machine measures pH changes resulting from the release of hydrogen ions during base incorporation by DNA polymerase (Loman et al., 2012). The analog signal is converted into a digital signal, which is then interpreted by the software (Rothberg et al., 2011). Ion Torrent instruments can generate reads up to 400 bp in read length, but there is reduced throughput and a higher rate of insertion and deletion (indel) error after long homopolymer stretches (Eid et al., 2009; Loman et al., 2012).
PacBio
This is the only sequencing platform that offers both second- and third-generation sequencing capability. Its core technology is termed SMRT sequencing (single molecule, real-time sequencing), and it can sequence single molecules in their native DNA or RNA form. In the SMRTbell, the template is created by hairpin adaptors, and then ligated to both ends of DNA targets (Shi et al., 2023). The templates are loaded on a chip, known as a SMRT cell, followed by diffusion into a sequencing unit called a zero-mode waveguide, which measures available light detection. For each zero-mode wave guides a single polymerase is immobilized, followed by binding to the hairpin adapt of the SMRTbell for onset replication (Eid et al., 2009). Four fluorescent nucleotides, which generate distinct emission, are added to the SMRT cell (Rhoads and Au, 2015). Once a base is held by the polymerase, a light pulse is produced to identify it. This produces much longer average read lengths of more than 10 kb, with a potential N50 of more than 20 kb. This platform was known to have a high (10%) intrinsic error rate and remains much more costly than other commonly used platforms (Rose et al., 2016), however, its advancement of HiFi sequencing has been proven to generate highly accurate long-reads (e.g. Baid et al., 2023; Hotaling et al., 2023).
Oxford Nanopore
Nanopore technology (ONT) is a single molecule sequencing technology that measures the changes in the electric current generated as nucleotide bases pass through a biological nanopore, followed by molecular motor protein anchoring https://nanoporetech.com/. ONT can use small flow cells (up to 2.8 Gb of data per run) with a Flongle adapter or MinION flow cells (50 Gb per run), GridION (250 Gb per run) or PromethION (14 Tb per run) for its applications. The smallest instruments, such as the MinION and Flongle, are portable and can provide cost-effective, real-time results when large data sets are not needed (Chen et al., 2023). MinION instruments are included with initial purchases of flow cells effectively meaning no upfront instrumentation costs, which helps low capital laboratories access sequencing technologies. The ONT intrinsic single molecule sequencing characteristic within third-generation platforms could mitigate the issue of low sequence coverage and depth observed in other sequencing platforms. Other advantages are the decreased turnaround time (24–48 hours) and the ability to analyze data in real time. Operators can also pause sequencing when sufficient data has accumulated meaning flow cells can be reused (Branton and Deamer, 2019). In contrast, other sequencing technologies process and save data at the end of the sequencing experiment lacking the same experimental flexibility. ONT also has no predefined library loading concentration, time, or output parameters. It is a long-read technology that greatly improves the assembly of virus contigs, especially when hybridized with other high-quality second-generation sequencing platforms (Maina et al., 2023). ONT MinION is a portable USB-type device, which makes it adaptable to any laboratory setting. Conversely, higher error rates than other technologies remain an issue with this sequencing technology (Laver et al., 2015; Sanderson et al., 2023). However, when investigations are based on a single or small number of samples and expensive sequencing platforms are not a practical option, ONT MinION can serve as an alternative tool for plant health diagnostics and virus research (Pecman et al., 2022). Additional applications of the MinION sequencer include, animal and human virology samples (Quick et al., 2016; Mackie et al., 2022), for example, genomic surveillance for severe acute respiratory syndrome-related coronavirus 2 (SARS-CoV-2), the causal agent of COVID-19 disease (Meredith et al., 2020), which supported the recent global pandemic and public health challenge.
Regardless of platform, using HTS as a metagenomics tool has revolutionized the identification and characterization of plant viruses including identifying and discovering novel plant viruses and viroids leading to better disease management (Maina et al., 2021; Malapi-Wight et al., 2021; Lebas et al., 2022; Alcalá Briseño et al., 2023; Fontdevila Pareta et al., 2023). HTS offers the capability to identify both known and unknown virus pathogens without requiring their prior knowledge of the biological sample (Villamor et al., 2019; Lebas et al., 2022; Alcalá Briseño et al., 2023; Fontdevila Pareta et al., 2023), and a reduced diagnostic timeframe (Al Rwahnih et al., 2015; Maina and Jones, 2023). It outperforms conventional detection methods given that, in a single test, it can capture the sequences of all viruses present in a host, including the co-existence of multiple viral species or strains of the same species. The advances in genomics technology have allowed HTS to remain versatile in comparison with traditional diagnostic tests (e.g. Rott et al., 2017; Villamor et al., 2019; Lebas et al., 2022; Alcalá Briseño et al., 2023; Fontdevila Pareta et al., 2023).
Metagenomics as a common RNA sequencing tool
As most plant viral genomes are composed of RNA, RNA sequencing (RNA-Seq) is an example of a highly used metagenomics tool that has gained momentum for both quantifying and mapping transcriptomes and the integrated mining of viral sequences. In plant virology, HTS has largely been adopted as a universal method for the detection and discovery of previously unknown viruses and viroids (Villamor et al., 2019; Maina et al., 2021; Whattam et al., 2021; Lebas et al., 2022; Maina et al., 2022; Alcalá Briseño et al., 2023; Fontdevila Pareta et al., 2023). The RNA-Seq approach involves converting RNA extracts to cDNA and then ligating adaptors to facilitate the pooling of multiple samples for sequencing of tagged DNA. Raw reads are assembled into contigs or scaffolds using an array of bioinformatic pipelines (e.g. Maina et al., 2017b; Maina et al., 2018a, Maina et al., 2018b, Maina et al., 2018c). However, various RNA-Seq approaches have been widely reported with different nucleic acid inputs, library preparation, and sequencing platforms for plant virus discovery and characterization. These approaches include using total RNA (totRNA), ribosomal RNA-depleted total RNA (rRNA-depleted totRNA), double-stranded RNA (dsRNA), small RNA (sRNA), or polyadenylated RNA (polyA) [as reviewed in Wu et al. (2015)]. Considering the genetic variability of viral genome structures, it is conceivable that the choice of sequencing chemistry directly impacts HTS output and downstream analyses. In the early days of virus HTS, viral genomic approaches focused on the use of sRNA and totRNA sequencing. Although both approaches can effectively detect viruses (Kreuze et al., 2009), they require an input of a high integrity RNA number (>7) (Schroeder et al., 2006), which is not always feasible, especially when dealing with degraded sample material. Another major drawback is the high ratio of host sequence data generated compared to that of the target sequence, leading to skewing of low abundance or low titer viral targets. sRNA gel purification steps are also laborious for routine screening of certification samples and may also lead to loss of template during the gel extraction procedure, consequently affecting the final data quality. Similarly, due to the shorter read lengths (21–24 nt) generated, sequence assembly and annotation of complex virus-segmented genomes may be complicated, making genome reconstruction and strain identification more difficult (Massart et al., 2017, Massart et al., 2019).
The total RNA sequencing of plant viruses was first described by Adams et al. (2009) and Al Rwahnih et al. (2009), with a later review by Boonham et al. (2014). Its merits include oligo (dT) primers consisting of 12–18 deoxythymidines that anneal to polyadenylated tails of mRNA, requiring low input requirements of nucleic acids, which makes it highly suited to the detection of polyadenylated RNA viruses. This totRNA sequencing has been explored as an RNA-based enrichment strategy for RNA viruses but is biased (only selects) polyadenylated viruses (Wu et al., 2015). The technique also suffers shortcomings when the viral RNA titer is low within a background of plant RNAs. This can also be an issue in plant species known to have abundant ribosomal RNA leading to fewer reads generated corresponding to virus RNA (Wamaitha et al., 2018; Havlová, and Fajkus, 2020). Additionally, a high ribosomal RNA background impacts the sequencing cost with higher sequence depth required (> 10 million reads) to ensure sufficient viral reads for virus genome assembly. To overcome the above issues, the viral detection threshold, and data quality can be improved by adopting enrichment strategies coupled with strand-specific library preparation procedures, such as depletion of the host (plant) ribosomal RNA (rRNA) and host messenger RNA (Nagano et al., 2015; Ndunguru et al., 2015; Maina et.al., 2017a, b). The Ribo-Zero chemistry (rRNA depletion) plant subtractive hybridization approach successfully reduced plant rRNA (e.g. Maina et al., 2021). It has also been found that the removal of plant ribosomal RNA by specific oligonucleotides resulted in a 10-fold enrichment of viral sequences (Adams and Fox, 2016). Importantly, a combination of rRNA depletion and random primer oligonucleotides for subsequent cDNA synthesis offers an opportunity for sequencing low-quality, degraded samples while also annealing to multiple RNA species, including phloem horizontally spread viruses (Maina et al., 2017c). For example, polyadenylated and non-polyadenylated RNA viruses has been efficiently detected from both high and low-integrity RNA using these approaches (Nagano et al., 2015; Maina et al., 2019a). During RNA extraction, omitting DNase treatments enhanced the parallel detection of DNA and RNA viruses (Zang et al., 2011). This approach has been widely exploited in plant transcriptome sequencing, generating accurate and reproducible datasets (e.g. Levin et al., 2010; Bailey et al., 2016). Studies have found sequences from strand-specific libraries to be more reliable than data from unstranded libraries and can accurately determine the expression of antisense RNA (asRNA) and other overlapping genes as well as the direction of intronic reads (Sigurgeirsson et al., 2014). Previous research shows plant virology research should strive to adopt strand-specific data to detect RNA viruses due to greater confidence derived from these data sets and fewer issues during bioinformatics analyses (Maina et al., 2017b). However, while this approach suits high-confidence results in plant health viral diagnostics, it remains relatively costly for adoption in routine testing. As such, innovative modifications are needed to develop cost-effective and high-quality RNA-Seq approaches for plant health certification programs.
Highly reliable, RNA-Seq traditionally employs recoding processes during library preparation, such as reverse transcription, second-strand synthesis, and PCR amplification. However, recoding can lead to errors or biases in the final sequence data, which could conceivably result in false negative detection of low abundance viruses. More recently, direct sequencing of RNA has been achieved using the ONT new chemistry. The direct RNA long-read sequencing has proven to be time efficient and robust for unraveling cell transcriptional features (Garalde et al., 2018; Bryrne et al., 2019; Cole et al., 2020). RNA viruses are notoriously variable (Briese et al., 2015), and ONT direct RNA-Seq offers an alternative cost-effective sequencing approach to reveal their native genetic structure since it directly sequences RNA without modifications (Fonzino et al., 2024). In addition, fewer amplification steps reduce bias, resulting in high-quality reads translating to quality assemblies (Gao et al., 2021). Its current drawback is the initial RNA required (500 ng) and the subsequent error rate, especially at the 5’ untranslated region (UTR) compared with Illumina sequencing (Wongsurawat et al., 2019; Maina et al., 2021). However, considering the recent substantial improvements in ONT DNA sequencing quality, this error rate for direct RNA sequencing is expected to improve in the next few years. Notably, incorporating an rRNA-depletion step in MinION direct totRNA sequencing significantly increases the data quality and sensitivity (Pecman et al., 2022). Another effective ONT virus detection strategy is cDNA-PCR sequencing of totRNA, which also provides high virus detection when rRNA depletion is incorporated within library preparation steps, although still associated with some errors. Nevertheless, when a rapid confirmation is needed, Illumina data or qPCR could be used for further verifications.
Targeted genome sequencing TG-Seq is an alternative method involving shotgun metagenomics in HTS diagnostics (Maina et al., 2021). The power of TG-Seq to generate many individual barcode sequences in a single reaction, with each sequence originally sourced from a single molecule, enables the simultaneous identification of individuals in a large mixed virome community (Maina et al., 2021; Mackie et al., 2022). Similar approach has been used in surveillance, monitoring and pathogen source tracing, such as metabarcoding various organism ecosystems in plant pathogen surveillance (Piper et al., 2019). For instance, TG-Seq is a simple tool that serves as an important means of passive surveillance and detects not only key pests but also other unanticipated species. This is because of the availability of conserved genetic regions, also known as barcode sequences or taxonomic markers which allow the design of a generic detection metabarcoding assay across many taxa. Several genetic regions have been proposed for identifying pathogens. For instance, fungal identification using the internal transcribed spacer regions, bacterial using 16S ribosomal RNA (Schoch et al., 2012; Mwaikono et al., 2016), mitochondrial cytochrome oxidase I for insects and animals (Piper et al., 2019), and the 18S RNA for nematodes (Ahmed et al., 2019). In contrast, viruses are well known to be highly variable, even in closely related strains and species, unlike bacteria and fungi (Maina et al., 2021). Additionally, viruses lack a universal conserved sequence that spans different families and genera. Given the high sequence genetic diversity within plant viruses, and the lack of a universally conserved barcode, the TG-Seq approach requires the use of a different marker for each group of viruses. Overall, regardless of the sequencing approach adopted in viral diagnostics. HTS laboratory protocols must be continuously updated and curated, including keeping up to date with library preparation and sequencing chemistry.
Technical considerations of HTS in plant health virus diagnostics
The accuracy of HTS data relies on stringent laboratory processes, such as extracting high-quality nucleic material and following strict nucleic template requirements for library preparation processes (Borgström et al., 2011; Villamor et al., 2019). In general, most HTS preparation protocols include common procedures such as initial mechanical or enzymatic shearing of nucleic acids to achieve a suitable insert size, end-repairing, A-tailing, ligation and random amplification to enrich libraries (Figure 1). The choice of library preparation method primarily depends on downstream applications. For example, targeting DNA or polyadenylated versus non-polyadenylated RNA viruses. There are several metagenomic protocols from various manufacturers, that are available as a one-stop-shop single kit, containing all required components for the detection of RNA and DNA viruses. The adoption and applicability of these protocols must consider the availability and compatibility of the sequencing platform, the genetic nature of the target and its host, and associated operational costs such as labor and consumables. It is also important to consider the chemical robustness of each selected chemistry, for instance, hybrid capture methods that enrich targets using oligonucleotides to eliminate abundant host nucleic acids or to specifically fish out target nucleic acids (Wamaitha et al., 2018).
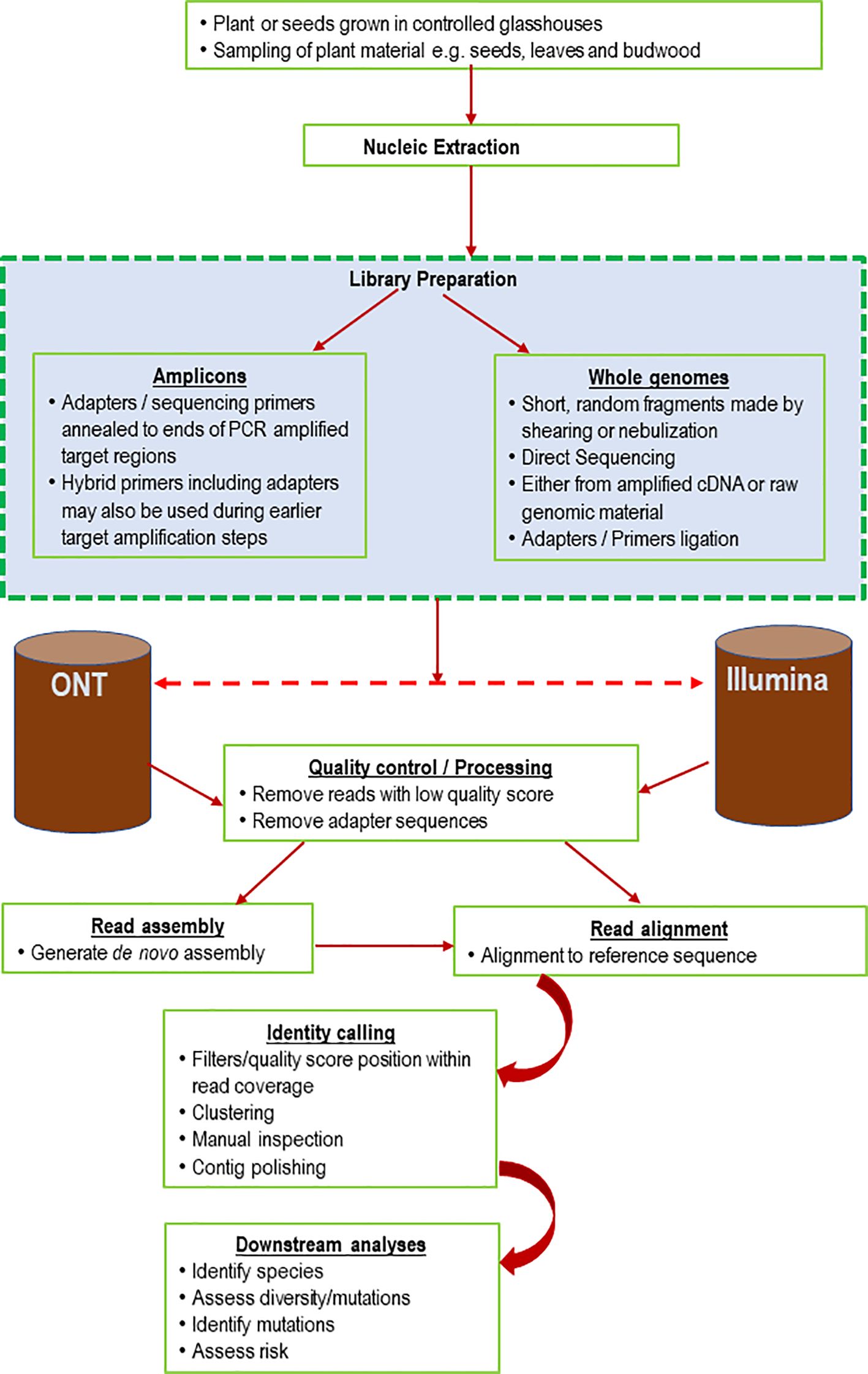
Figure 1 Schematic diagram representing amplicon, shotgun and bioinformatics pipeline for viral diagnostics using either Oxford Nanopore or Illumina sequencing technologies.
Nucleic acid extraction
Nucleic acid integrity is important for generating high-quality sequencing data to aid virus discovery and detection (Pecman et al., 2017). There is limited information describing the best practice in nucleic acid extraction for HTS detection of plant viruses. In most cases, extraction protocols generating nucleic acids with purity and integrity for conventional PCR or real-time PCR should also be suitable for HTS diagnostics (Johnson et al., 2012). The ability to obtain high-quality nucleic acids is affected by the sample type, such as freshness or biological nature (seed, leaf, stem, soil, water, or insects). Degraded RNA or DNA may result in poor-quality datasets (Bryant et al., 2012), leading to failed detection of low-titer RNA viruses. Therefore, HTS pathogen testing should adhere to the initial quality and quantity requirements described by the protocol manufacturer, for example, the RNA Integrity Number threshold (Mueller et al., 2004). Several methods can be adopted for nucleic acid extraction and often reveal disparities in the quality, quantity and specificity of the RNA obtained (Fitzpatrick et al., 2021). Considerations should be made to account for low-titer target(s) engulfed within a host expressing a high quantity of ribosomal RNA (Havlová and Fajkus, 2020) or additional organisms present at high levels, such as fungi or bacteria, that may inhibit actual target detection. The target organism concentration might be compromised by competition between the mixture of microbes in the population, as seen with environmental DNA (eDNA) samples (Goldberg et al., 2016). Different extraction methods should also be trialed for plant materials with high lignin or oil contents, such as seeds, to determine their suitability. This is particularly important when testing for low-titer viruses localized in the inner seed or phloem (Massart et al., 2019). Extraction methods may incorporate phenol and guanidine thiocyanate to deactivate RNases (Zepeda and Verdonk, 2022), to deter RNA degradation. However, using this combination of organic solvents may contaminate the final RNA extract (Tavares et al., 2011). RNA isolations can also adopt silica membrane-based spin column methods, which do not involve these organic solvents (Vennapusa et al., 2020).
Enrichment steps, such as ribosomal depletion or pre-cDNA synthesis to increase the detection of low-titer viruses or any potential DNA viruses integrated within the host, are essential to boost detection and reproducibility (Maina et al., 2017f; Maina et al., 2018a). The enrichment method should factor in the structure of the target genome (ssRNA, dsRNA) or potential host virus genome integration (Maina et al., 2018a, c). The nature and viability of the samples should be evaluated to establish unbiased protocol guidelines for HTS experiments (Budowle et al., 2014; Puchta et al., 2020). For example, negative-strand RNA viruses are found to exist in low-titer in many infected plants, and the use of dsRNA enrichment procedures will reduce the likelihood of detection of such viruses (Ho and Tzanetaki,s 2014). Detection of such targets can be improved significantly by ultracentrifugation of plant lysate followed by DNAse treatment during extraction and final host ribosomal deletion (Adams and Fox, 2016). The development of new HTS protocols should trial multiple HTS procedures, such as nucleic acid purification and library preparation chemistries, and the resultant HTS dataset should be analyzed using multiple bioinformatic pipelines. Once a high-sensitivity and reproducible nucleic acid extraction method is achieved, it should be documented for future reference. Although different laboratories have diverse nucleic acid processing preferences based on their experience, national and international laboratories should strive to share and retest standard operating procedures (SOPs), working toward standardization (Villamor et al., 2019).
Contamination
In molecular biology, contamination has remained pervasive (Ballenghien et al., 2017), which can result in false positive detections and scientific misinterpretation. HTS processes are prone to contamination due to the multiple steps involved in library preparation and the nature of handling multiple reagents or consumables. HTS has a broad range and high sensitivity detection capability, even at lower microbial concentration levels (Selitsky et al., 2020). Some of the known sources of contamination in HTS are from the field during sample collection and storage and from the laboratory environment, such as surfaces, equipment, and reagents. Mishandling of HTS laboratory reagents used for extraction and library preparation can significantly impact HTS results. Onboard sequencing and clustering have also remained problematic sources of carry-over contamination between sequencing runs (Nelson et al., 2014). Ballenghien et al. (2017) demonstrated significant cross-contamination in sequencing facilities. In addition, experimental design, such as multiplexing of multiple samples in a single sequencing lane, has been associated with cross-contamination between libraries, particularly due to index hopping and onboard clustering stochasticity (Buschmann et al., 2014).
Mitigation measures may include adopting and adhering to a best practice laboratory routine of using sterile reagents, consumables, tools, and equipment, frequently changing gloves after every bead cleanup step, particularly when handling multiple reagents, and frequent cleaning of benches, equipment and tools using 70% ethanol or RNAseZAp. When using bleach, safety must be considered because bleach is corrosive and reacts with guanidinium thiocyanate, which is found in many extraction kit lysis buffers, emitting a highly toxic compound (Goldberg et al., 2016). As extra measures, laboratories should also consider periodically assessing air pressure and filtration in the laboratory environment. UV treatment of benchtops or workstations should also be considered to prevent contamination of HTS experiments. HTS contamination may also arise from inadvertent sample jumping between tubes during laboratory sample handling or pipetting. While the risk of this type of contamination is less severe than for detection methods involving amplification, such as PCR, buffering barrier strategies should be considered, such as separation of samples suspected to contain a high viral titer from those suspected to contain a low viral titer (Larsson et al., 2018), and by establishing dedicated HTS laboratory work areas. Adopting the colored tracer dye AccuVue™ simplifies reaction assembly if used with clear plates or tubes and helps to minimize pipetting or mixing errors. Positive and negative controls should always be included and processed in parallel with actual sample library preparation to track any potential contamination in the final read analysis. Some sequencing platforms, such as Illumina, include maintenance washes between runs, followed by a template run wash to circumvent cross-contamination. More practices and recommendations for reducing contamination in HTS and molecular laboratories are described in Goldberg et al. (2016) and EPPO (2021).
Pooling of libraries
Multiplexing allows multiple diagnostic samples to be pooled together, reducing the cost, and significantly improving the turnaround time. In HTS, the process involves multiplexing a high number of diagnostic in parallel by tagging the library with a unique index sequence (barcode) that facilitates tracing after pooling, followed by computation deconvolution (Kircher et al., 2012; Budowle et al., 2014). For diagnostic sequencing to be cost-effective this is indispensable; however, its limitations include misassigning read sequences within the samples due to index hopping (van der Valk et al., 2020; Modi et al., 2021). Notably, read misassignments can also occur due to sequencing errors intrinsic to the sequencing platform. Also PCR-induced chimerism can result from incomplete library amplification steps, such as extension and reannealing, and sample cross contamination can carryover in the machine from the previous sequencing runs (Irish et al., 2018). These misassignments can be reduced by incorporating Illumina dual unique indices in library pools and using index blockers (Kircher et al., 2012; MacConaill et al., 2018). The application of unique dual indices within HTS libraries has been shown to reduce indexing crossover from multiplexed samples, especially when sequencing using patterned flow cells (Irish et al., 2018). This enhances accurate data generation and diagnosis, avoiding false positives (Costello et al., 2018; MacConaill et al., 2018). Normalization of each library should also be performed using relevant sequencing platform recommendations to reduce the library pooling bias that may lead to uneven data quantity of final raw read sequences (Hébrant et al., 2018). Finally, it is advisable to screen for index cross-contamination as a routine quality check of HTS datasets (Zavala et al., 2022).
Sequencing depth
Genome depth, coverage evenness, read length, and Q3 (score) quality are major factors that influence the accuracy of virus detection within HTS. As mentioned earlier, read length and quality are key considerations in plant virus diagnostics and research. A short <75 bp read length complicates sequence assembly, annotation, and strain identification. Illumina maximum read lengths of 300 bp for single or paired reads are preferable for virus detection. Longer reads generated by Pacific Biosciences and Oxford Nanopore technologies offer better genome reconstruction but with lower sequencing accuracy (Filloux et al., 2018), potentially causing indels within segmented viruses. Additionally, sequencing depth is important as it directly correlates to the titer of plant viruses present (Massart et al., 2019). Several variables, such as the viral titer, dictate the amount of coverage and sequencing reads that are needed to successfully detect specific viruses (Maina et al., 2017c). The discrepancy in sequencing depth between RNA and DNA viruses has been previously observed (Pecman et al., 2017). Previous findings have demonstrated that over.5 million reads were sufficient to cover the entire RNA virus genome (Visser et al., 2016; Malapi-Wight et al., 2021). However, the number of reads required to detect DNA viruses has been found to be higher and less consistent (Pecman et al., 2017). High sequencing depth may also increase the incidence of false positives due to over-sequencing of contaminants or lead to an increased cost per sample (Massart et al., 2014). Hence, it is important to validate new and existing HTS tests and datasets to evaluate the depth, and sequencing read requirements of different viruses.
Bioinformatics
Due to the broad adoption of HTS in pathogen research and diagnostics, and the substantial increase in dataset complexity. Bioinformatics has become an integral tool for routine analysis. Sequence-derived results are dependent on the quality of the input data, and the choice of correct analysis software. Additionally, software analysis parameters and the quality of the sequence databases used are critical (Pappas et al., 2021). For each application, there are often numerous options available for sequence analysis that can be run using a command line or graphical user interfaces (Table 1). Regardless of the choice selected for a particular analysis, an individual must understand the principle of the actual analysis performed and have an understanding of virus biology to credibly produce appropriately analyzed results and interpretation (Maree et al., 2018; Olmos et al., 2018).
Quality control
Before proceeding with any HTS data analysis, it is critical to determine the quality of the dataset. This might be done using the sequencing equipment by analyzing raw data metrics such as cluster densities, quality scores, translocation speeds, observed read lengths verses expected, and read quantity output. HTS platforms usually generate standard universal formats (such as FASTQ) after (or during) sequencing, which are compatible with open-source bioinformatic programs (Chen et al., 2018). Several heuristic methods can be used to identify spurious sequences sourced from unutilized primers, indices, or adapters, overrepresentation of specific nucleotide subsequences (k-mers), and the presence of duplicates in the sequencing dataset (Pappas et al., 2021). A range of bioinformatic tools have been developed to calculate these metrics and produce quality reports that summarize the results in useful, informative graphical interfaces, such as FastQC and PRINSEQ (Schmieder and Edwards, 2011; Krueger, 2012). Additional software are also available to perform quality control by trimming adapters and poor-quality sequences, including trim galore, trimmomatic, and cutadapt (Martin, 2011; Bolger et al., 2014, Krueger, 2015). Some software, such as fastp (Chen et al., 2018), can perform quality trimming and calculate quality control metrics in a single package. In second-generation sequencing instruments, base calling tends to drop at the end or near the end of each cycle, which translates to low-quality reads (Kwon et al., 2014; Lambert et al., 2018). Merging paired-end sequenced reads generated from both sense and antisense directions can significantly increase the overall data quality of the reads generated, resulting in high-quality assemblies (Magoč and Salzberg, 2011). Data should be cross-checked since there is potential for repeat regions to be misassembled if paired reads flank these regions. Quality trimming before read merging should be implemented to remove and discard extremely short reads, as they can occur multiple times within the target sequence and might contribute to misleading genomic information or identification. Typically, bases that fall below a set quality score threshold are trimmed along with any primer or barcode sequences (Maina et al., 2021). However, this can vary with application; for instance, it may be better to perform quality trimming within de novo analysis than in a reference aligning mapping process (Liao and Shi, 2020). Additional tools can be applied to perform error correction on the available short reads. After quality control, the trimmed reads can be queried for species identification via a database for initial draft data surveillance. This can be achieved through metagenomics databases such as nucleotide NCBI-blast, MG-RAST (Meyer et al., 2008; Keegan et al., 2016) or in-house database servers to characterize and confirm the viral species abundance. Nevertheless, for stringent confirmation of the virus species present within a sample, the trimmed raw data were assembled using available assemblers to generate contigs for subsequent comparison to sequence databases (Brinkmann et al., 2019). This can only be achieved by adopting high-quality score longer reads, since short reads have been associated with complexities during assembly, which might translate to a drawback when targeting a variant or novel virus (Massart et al., 2019).
De novo assembly and reference mapping
De novo assembly reconstructs a virus genome from fragmented sequencing reads without reference to a prior known sequence. The reads are assembled into contigs, and the quality of the resulting de novo assembly depends on the size and continuity of these contigs. A contiguous cross-check of the resulting contigs should be performed in every final de novo assembly to calculate QC metrics like depth and breadth of coverage, but also identify any potential errors in the assembly of the virus target contig. Reference mapping is the rapid alignment of reads to a reference sequence (Budowle et al., 2014). Preferably, this reference must be phylogenetically close to the suspected pathogen’s sequenced reads. Where multiple pathogens are suspected, the analysis must be done using multiple suspected target reference genomes or partial sequences performed in parallel (Maina et al., 2018a). This approach requires an understanding of the biological insight into the target virus rather than just an automated software approach.
The removal of host ribosomal sequences can be achieved using reference mapping, but other nontargets, such as fungi or bacterial reads, could also be present in samples at a higher percentage than viruses. In some cases, these background sequence reads could be filtered to increase the accuracy of detection (Lambert et al., 2018; Baizan-Edge et al., 2019). The reads that confidently map to the host genome must be investigated before being discarded to improve the identification of viral sequences such as badnaviruses and pararetroviruses that might have partially or completely integrated into the host genome (Hohn et al., 2008; Geering, 2014; Maina et al., 2019a). Since virus-host genome integration remains an ongoing bioinformatic challenge, it should be accounted for and carefully considered within plant virus diagnostic and certification activities to avoid the risk of misidentification (Massart et al., 2019). Such challenges could be mitigated by comparing pre-mined putative host reads to a reference database to identify specific integrated viruses. Importantly, it is vital to combine both de novo and reference mapping to improve the probability of detecting low viral titers or localized tissue-limited pathogens (Maina et al., 2017c; Maliogka et al., 2018).
Currently, there are few highly specialized tools or algorithms that have been written only to specialize in plant virus data analysis, especially virus HTS amplicon-derived datasets (Villamor et al., 2019). Web-based pipelines with GUIs have been designed and tested for plant virus detection and discovery, examples include VirFind (Ho and Tzanetakis, 2014), which uses Velvet (Zerbino and Birney, 2008; Zerbino, 2010; Table 1), SPADES (Bankevich et al., 2012) and Trinity programs (Grabherr et al., 2011) to generate de novo assembled contigs. Virus Detect (Zheng et al., 2017) and VSD toolkit (Barrero et al., 2017). In most cases, plant virologists have adopted software that was developed to analyze eukaryotic transcriptomic data. For instance, reference mapping can be performed effectively using command line-based tools such as Bowtie2 and Minimap2, which were originally designed and tested using human genomes (Langmead and Salzberg, 2012; Li, 2018). Viral genome assembly can also be achieved using stand-alone software pipelines such as Velvet, Trinity, metaSPAdes, Tadpole and Flye (Grabherr et al., 2011; Nurk et al., 2017; Zerbino and Birney, 2008; Bushnell, 2014; Li, 2018; Kolmogorov et al., 2020) and others (Table 1). Some of the above pipelines have proven to produce high-quality assemblies and mappings that have led to the identification of known and unknown viruses.
In addition to open-source pipelines, several commercial bioinformatic software packages have been developed for the analysis of SNPs, transcriptomics, reference mappings, and de novo assembly. This software includes CLC Genomics Workbench (Qiagen, USA), Geneious (Geneious, New Zealand) (Kearse et al., 2012), DNASTAR’s Lasergene (Burland, 1999), and Galaxy (Galaxy Community, 2022), which offer user-friendly interfaces and are integrated with core algorithms and programs. Nevertheless, the main drawback of these commercial software remains the cost. Additionally, GUI bioinformatics software is often limited to personal computers with lower processing power than what can be obtained from cloud computing or high-performance computing systems which can increase the time taken to analyze results, especially for larger datasets. Comprehensive descriptions of the analysis of several laboratory HTS-derived datasets can be found in Massart et al. (2019). Several attempts have also been made to streamline HTS sequence analysis into one-stop-shop solutions aiming to provide accurate diagnosis directly from sequencing reads (Yamashita et al., 2016; Barrero et al., 2017; Rott et al., 2017; Lebas et al., 2022). This constant advancement in distinct sequencing analysis methods may create a challenge in future bioinformatic analysis. Thus, a constant update of bioinformatics pipelines and the users is vital to cope with the vast range of new distinct datasets, and subsequent interpretations of detected virus sequences remain indispensable.
Prior to HTS, Sanger sequencing technology was the main available tool to generate viral sequence data followed by homology searches for virus detection. Its analysis is less complex since the chemistry involves deriving short fragments that could be manually analyzed by molecular biology-trained staff without extensive computing. However, HTS has greatly increased the size and complexity of biological sequences, which generate datasets that require specialized bioinformatics trained staff, and newer algorithms that require high-performance computing to execute and generate robust credible analyses. Below, we highlight a few major sequence analysis tools and critical steps vital to accurately analyze HTS-derived datasets within plant virology diagnostics and research.
HTS data and software management
Software management
Both laboratory and bioinformatic aspects of HTS technology are evolving rapidly. This can translate to the obsolescence of HTS protocols, sequence databases, sequence analysis software and the persistent need for better data management strategies. As such, viral diagnostic tests must continuously update and curate HTS laboratory protocols, including associated software. As discussed above, bioinformatic software can consist of published open-source pipelines or commercial analysis software. However, the dissemination of bioinformatics expertise has improved the ability of individual laboratories to generate their own custom “in-house” bioinformatics pipelines using programming languages such as python, R and bash. These pipelines and their parameters customized for the detection of distinct viruses need to be applied to follow quality coding practices (Wilson et al., 2014). This includes managing software dependencies that are used within the larger pipeline, for instance, to identify sequencing reads unique to a new virus. For example, an analysis pipeline read aligner may require a dependency (e.g. Bowtie2) to align reads to a reference sequence. Updating or upgrading a single dependency may cause incompatibilities or introduce errors that could lead to failure of the analysis or a false positive detection. As such, in PEQ programs, custom virus analysis pipelines must be validated prior to their widespread use. Furthermore, it is strongly recommended that diagnostic pipelines are run in separate software environments [such as Anaconda or Python virtual environments or using software containers (e.g. Docker or Singularity)]. These systems allow for control of dependency versions and the sharing of both pipelines and software environments between users and laboratories. To ensure that analyses are repeatable, analysis pipeline and pipeline dependency versions must be recorded for each analysis. A bioinformatics analysis can fail for numerous reasons, including input file corruption, excessive server load (especially memory) or network connection interruption, therefore, appropriate error handling should be included in custom pipelines. Custom software bioinformatics pipelines must be validated before use (Roy et al., 2018). However, validated pipelines, whether these are open-source or offered by commercial suppliers, may instead be verified by operators to ensure they work as indicated within the systems of the diagnostic laboratory. Validation or verification should involve testing with high-quality raw reads generated from previously collected virus reference control material. Such studies should also compared against other programs to allow the detection of any potential pipeline-specific artefacts. Validation studies must be documented to include the performance of the analysis pipeline. This must include metrics including the sensitivity, specificity, and accuracy of the pipeline to detect mixed virus species infections, virus variants, distinct genomic structures, and any limitation. To ensure repeatable results, validation studies also must be conducted in a system that closely resembles the expected operational environment. Finally, for custom pipelines, the use of version control software (such as Git, Concurrent Versions System, or Apache Subversion) must be adopted to track software and database releases and updates (Hall et al., 2013).
HTS data management
HTS data management is another key aspect that plant health diagnostic laboratories must consider to ensure result integrity and security, and to avoid the loss of raw data through accidental deletion or file corruption. Diagnostic HTS relies on the comparison of current and past datasets, so curation of previous datasets can be critical to test accuracy. For PEQ laboratories, data storage and retention policies need to be generated. Theses involve defining minimum data types and formats to be stored, including (e.g. fastq, bam and/or fasta formats). Data management policies must guide which data need to be kept and include the minimum storage time, location and accessibility. For instance, according to EPPO (2019), the minimum retention period for data is 5 years. Parameters may vary according to organizational and jurisdictional requirements and policies. HTS data backup must be developed to ensure data integrity and protection against any loss including ransomware or other cybersecurity threats. To ensure the integrity and confidentiality of HTS data sets, backups should be stored with multiple copies on servers, and it is best practice to maintain an off-site copy (e.g. using a secure cloud storage provider or second server within the same organization), and access should be limited to essential internal users (Hébrant et al., 2018). External storage, such as cloud storage (Grzesik et al., 2022), is readily scalable and can prevent the loss of datasets due to hardware failure, but bandwidth for access, cybersecurity, and confidentiality remain a major limitation. The cloud storage must establish the physical sovereignty of the data, and must ensure it complies with jurisdictional legal requirements. Where needed, a client agreement guiding data storage, data ownership, and deletion policies should be put in place. Where laboratories outsource cloud storage, it’s vital to consider provider data redundancy policies. Equally, once the datasets have undergone adequate quality control and have both internal and external approvals that consider the relevant country, state, territory and/or stakeholders. It is important to submit HTS datasets to appropriate curated externally shared databases. Analysis of HTS datasets involves the transfer between computing hardware, such as from the sequencer, to HPC. Data transfer using USB or external hard drives should be avoided. PEQ laboratories should establish a high-speed network with the ability to support the speed of 1 Gb/s for a successful transfer. This will reduce delays introduced by the transfer of large HTS dataset files. Importantly, the use of checksums for individual or compressed data files can mitigate data corruption during transfers.
Databases
The power of HTS for accurate identification relies completely on comparison to previously sequenced datasets. Consequently, the application of HTS methodologies as a routine diagnostic tool is dependent on databases of validated DNA and RNA sequences that represent the target organisms and genes (molecular markers). This database is critical for comparing sequences generated from the test plant (e.g. imported accessions tested in a phytosanitary program prior to release) with a database of sequences that represent target organisms. The validated sequences included in the database can be derived from known, trusted global databases, from sequences included in refereed scientific papers, or by the generation of sequences produced by the individual diagnostic laboratory, which can often be performed using vouchered specimens. There is a need to continuously expand and validate curated bioinformatics databases and their aligned algorithms to ensure robust identification of known and unknown plant viruses. It is also critical to have a centralized real-time curated plant virus sequence community database to solve the virus bioinformatics chasm. Databases such as the Barcode of Life Data System (BOLD), which is an online database that supports the identification of eukaryotic species such as insects, are lacking in the plant virus community globally. Currently, when constructing a virus sequence database for comparison purposes, scientists usually extract relevant viral sequences from GenBank. Many of these sequences remain unpublished and are not validated, which can lead to inaccurate diagnoses. As such, curation of a centralized virus sequence database is critical to avoid these issues.
Reference sequence databases
HTS is becoming increasingly mainstream for the detection of plant viruses. This creates opportunities to develop interoperable analysis standards to facilitate precise and accurate pathogen discovery and identification. Often, analysis standards rely on global sequence databases such as the National Center for Biotechnology Information (NCBI), the European Molecular Biology laboratory (EMBL), and DNA Data Bank of Japan (DDBJ) (Figure 2). The availability of quality raw and reference sequences within these free access global databases is core to HTS data interpretation and defining baseline knowledge for understanding pathogens, biological systems, phenotypes, and genomic variations (Yates et al., 2022). This also underpins the fundamental role of describing genomic variation and annotation, a prerequisite for new diagnostic development (Yates et al., 2022). In modern agriculture, access to quality sequence data is critical for scientific discoveries and improvements in disease management strategies to support agricultural productivity (Gaffney et al., 2022). More broadly, in the rise of COVID-19, public health has experienced one of the greatest challenges of mankind, but remarkably, open-access sequence databases revolutionized the development of effective vaccines (Winter, 2021). Likewise, in plant health certification, the availability of these data and the ability to interpret results are essential for the delivery of precision HTS crop diagnostics for global food security. While databases play an enormous role in modern science, there have been challenges such as the burgeoning growth of HTS sequence data (Siva, 2008; Figure 2). Although this is an opportunity, it has continued to create complexities in maintaining data accuracy and accessibility across databases. Efforts have attempted to correct and curate sequence data to harness such complexities (Pruitt et al., 2005). Additionally, some members of the scientific community have continued to update prior submitted sequences in global databases.
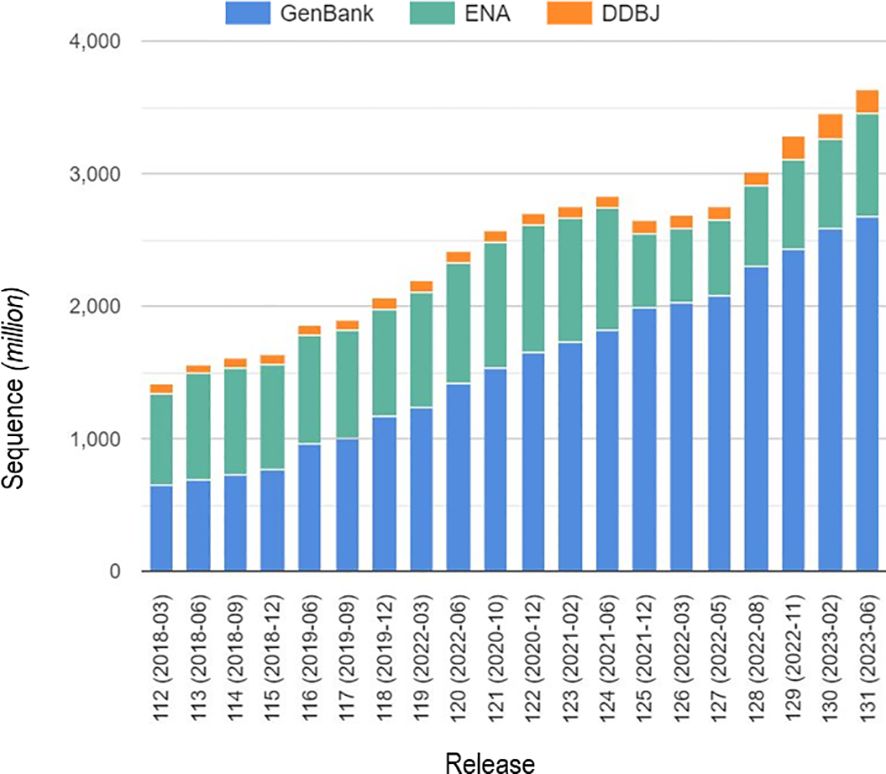
Figure 2 Number of sequences submitted in global sequence databases for the last five years. (Data from Bioinformation and DNA Data Bank of Japan, https://www.ddbj.nig.ac.jp/index-e.html).
Nevertheless, over the years, reference sequences have continued to suffer from not having standardized access, incomplete redundant datasets (Lathe et al., 2008), and duplications or errors in datasets. Reiterated sequence database accuracy is a valuable decision tool in target identification of both known and unknown viruses and their variants (Massart et al., 2019). The same study by Massart et al. (2019), describe a novel virus that was detected and incorrectly named the wrong species due to sequence database anomalies. Conversely, the success of accurate raw databases has a significant impact on virus identification and taxonomic classification. For example, watermelon crinkle leaf-associated virus 1 (WCLaV-1) genus Coguvirus, (family Phenuiviridae) was previously reported to have two different genomic segments (RNA2 and RNA3) (Xin et al., 2017), but the availability of high-quality deposited raw reads in the public database led to the determination that WCLaV-1 actually has a bipartite genome resembling other members of the genus Coguvirus (Zhang et al., 2021; Mulholland et al., 2023). This example reflects the importance of uploading high-quality HTS raw reads, processed sequences, and variant sequences coupled with accurately interlinked metadata. Additional comments or descriptions should be provided for incomplete or erroneous data highlighted in public sequence databases to inform database users and assist in quality control and decision-making. Within plant virus diagnostics, an HTS diagnostic test may be designed to target a range of known viral pathogens, for example, in post-entry quarantine or surveillance activities. In such scenarios, an in-house curated database could be designed and updated regularly to improve broad-spectrum detection. If accurate, this curated internal laboratory database would aid the interpretation of results and alleviate any confidentiality issues associated with early submission of results to public databases. Any in-house reference database must contain collected sequences of high quality with accurate annotation. Occasionally, where there is a disputed result or submission, it should be noted accordingly on the submission for consideration in subsequent and more-detailed analyses. The in-house database design model, as described above for custom analysis pipelines, should be updated regularly and documented for traceability and future improvements (Wilson et al., 2014). For unknown viral pathogens, global databases that have extensive sequence collections, but lower curation standards should be a starting point for detection and discovery. Researchers should strive to apply intertwined analyses to determine the accuracy of one database versus the other. An additional consideration is that possession of large in-house biological databases, that are critical to diagnostic pipelines, carries a potential cybersecurity risk such as a ransomware attack. Consequently, the PEQ laboratory must ensure that computing equipment used for HTS analysis is regularly updated and integrated with secure networking and cybersecurity policies.
High-throughput sequencing in routine plant health diagnostics
Most quarantine systems mainly test for known virus threats. This may pose an opportunity for introducing unknown viruses and their emerging genetic variants if they are not detected. Molecular, serological, and biological indexing methods are widely used in quarantine for virus detection. Molecular and serological tests are specific but may not detect a novel viral strain. Damaging exotic strains of endemic viruses can be missed using these methods and produce significant consequences to agricultural production. Additionally, some tests have low sensitivity, and core test reagents, such as virus-specific antisera, may be unavailable or difficult to source. Biological indexing provides the benefit of general screening, but these tests can take several months and are typically more labor-intensive (Villamor et al., 2019). Consequently, this prolongs the processing time in PEQ facilities, significantly adding to the cost of importation. Notably, for some commodities, the pathogen elimination process (i.e., via shoot-tip grafting), not the diagnostic process, typically dictates the time from importation to release. For example, in Australia, all imported citrus varieties are shoot tip grafted to remove all pathogens and are only released from post post-entry quarantine if no pathogens are detected, including strains endemic to Australia, to avoid the risk of importing exotic strains.
Resources can also be limited in PEQ facilities, restricting the number of varietal imports that can be processed at any given time (Al Rwahnih et al., 2015; Maina et al., 2021). This can impact the competitiveness, efficiency, and sustainability of the agricultural industries. The application of HTS in routine plant health screening is becoming more feasible, as the cost decreases and sequencing technology evolves, coupled with increased accessibility of bioinformatic tools (Villamor et al., 2019; Maina et al., 2021; Malapi-Wight et al., 2021; Mackie et al., 2022). There are enormous opportunities for adopting HTS for routine and surveillance diagnostic samples, which includes comprehensively identifying and understanding the status of pathogen genetics (Figure 3; Al Rwahnih et al., 2015; Barrero et al., 2017; Rott et al., 2017). HTS has been proven to expand our understanding of the diversity of complex virus species and is particularly useful for the development and validation of new detection assays and detect a wider range of viral variants (Malapi-Wight et al., 2021). Plants may be infected with multiple viruses and viroids (Martin and Tzanetakis, 2015; Dolja et al., 2017). Screening assays using PCR or immunological detection methods of such multiple viral populations are typically not highly successful (Maina et al., 2021). It may be more costly to run individual molecular or serological pathogen tests than the current cost of running HTS to screen for all pathogens present in each sample. Some HTS platforms are also portable and may be used in targeted border surveillance to evaluate and monitor imported agricultural commodities (Olmos et al., 2018; Velasco and Padilla, 2021). Studies have recommended the integration of HTS into plant health certification programs around the world to accelerate the availability of virus-tested material to the agriculture industry, particularly for commodities where the diagnostic process dictates the time spent in post entry quarantine (Gergerich et al., 2015; Martin and Tzanetakis, 2015; Maina et al., 2021; Velasco and Padilla, 2021; Lebas et al., 2022; Mackie et al., 2022). However, valid concerns and challenges remain when making decisions based on HTS results, as with all new technologies, and clear guidelines are needed to support the regulations.
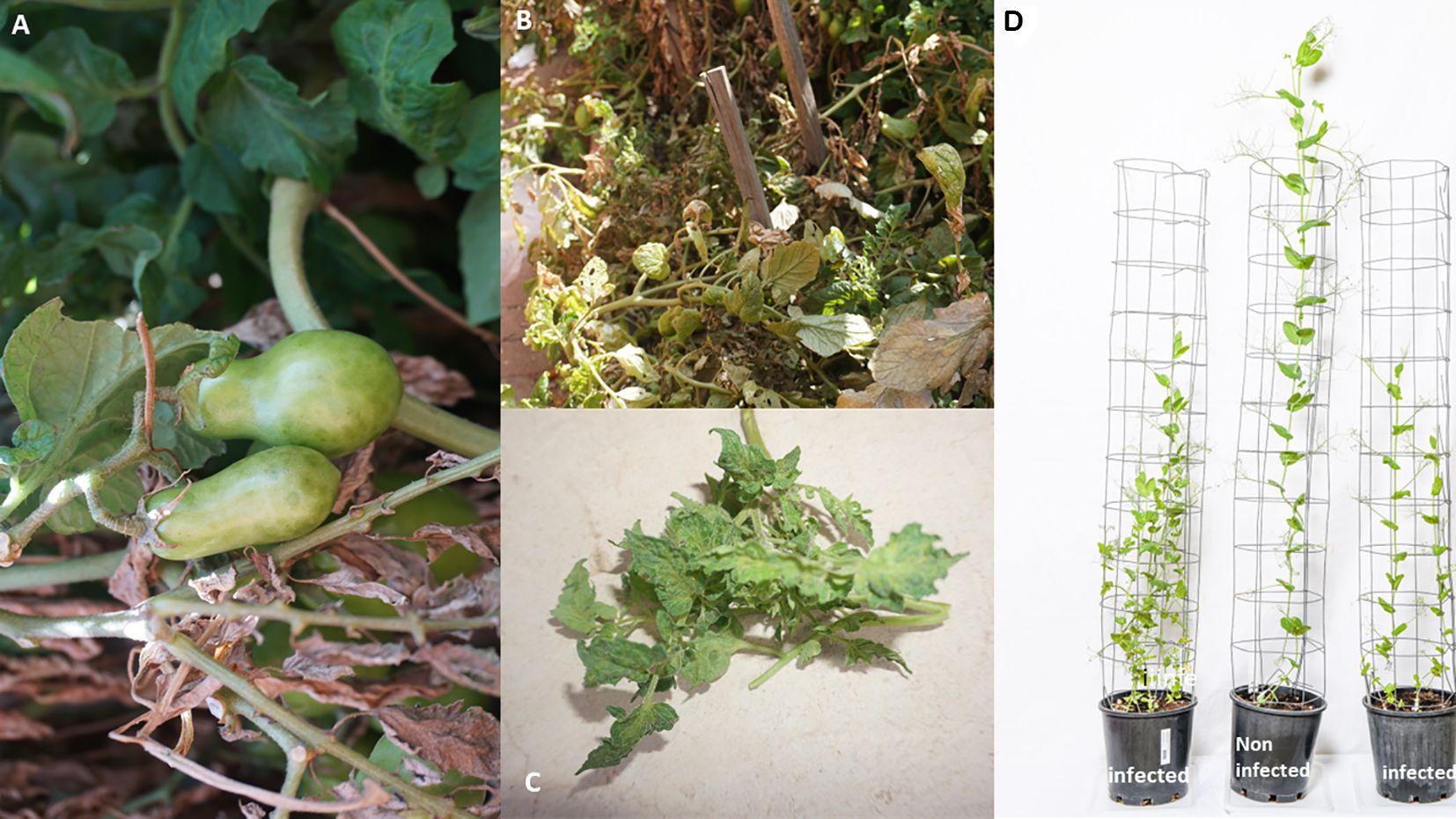
Figure 3 High throughput sequencing (HTS) detection of tomato fruit blotch virus in tomato plants in a home garden in Horsham, Australia, sown from commercial seeds (A–C). (D) Field pea plants were grown from asymptomatic field pea seeds in an insect-proof air-conditioned glasshouse maintained at 19-21°C. HTS detected pea seed-borne mosaic virus (PSbMV) in pots labeled infected - PSbMV, symptoms included stunting, reduced tendril size and pods with small-sized seeds.
Case studies
In 2015, the causal agent responsible for severe stunting and death of hop plants in Slovenia was identified by HTS to be citrus bark cracking viroid (CBCVd) (Jakse et al., 2015). This HTS discovery led to the inclusion of CBCVd on the European and Mediterranean Plant Protection Organization (EPPO) “Alert List” (Jakse et al., 2015). A further suggestion was made to adopt HTS in plant health detection of CBCVd in hops (Hadidi, 2019).
The National Germplasm Resources Laboratory, (United States Department of Agriculture, (USDA), United States (US) explored using HTS for the routine detection of sugarcane viruses that pose a threat to the US sugarcane industry (Roumagnac et al., 2018). Consequently, the US has adopted HTS for plant health certification of sugarcane varieties based on a provisional release category (Villamor et al., 2019). If no virus or viroid-like agents of quarantine significance are detected by HTS screening, the plant material is provisionally released. This baseline requirement allows for limited propagation of HTS-tested negative materials in designated areas approved by the U.S. Department of Agriculture Animal and Plant Health Inspection Service (USDA-APHIS), pending additional bioassays and laboratory tests prior to official release. Provisional release strategies enhance the multiplication of plant material for commercial production or agriculture industries in advance and can significantly reduce the time it takes for industry access to plant material.
In Australia, both industry and government have invested in innovative diagnostic approaches to improve the sensitivity, specificity, and efficiency of pathogen testing (e.g. Maina et al., 2021; Mackie et al., 2022). For example, the Australian Grains Genebank (AGG), a national biodigital resource center, is one of the largest collections of grain crop species globally and includes cultivated, landrace, and wild relative species of temperate and tropical grain crops. AGG provides informed access to grain genetic resources to accelerate cereal, oilseed, and pulse crop improvement for the benefit of Australian grain growers. AGG has been applying HTS for metagenomics and diagnostics to support germplasm certification programs and potential genomic surveillance activities (Figure 4; Maina et al., 2021).
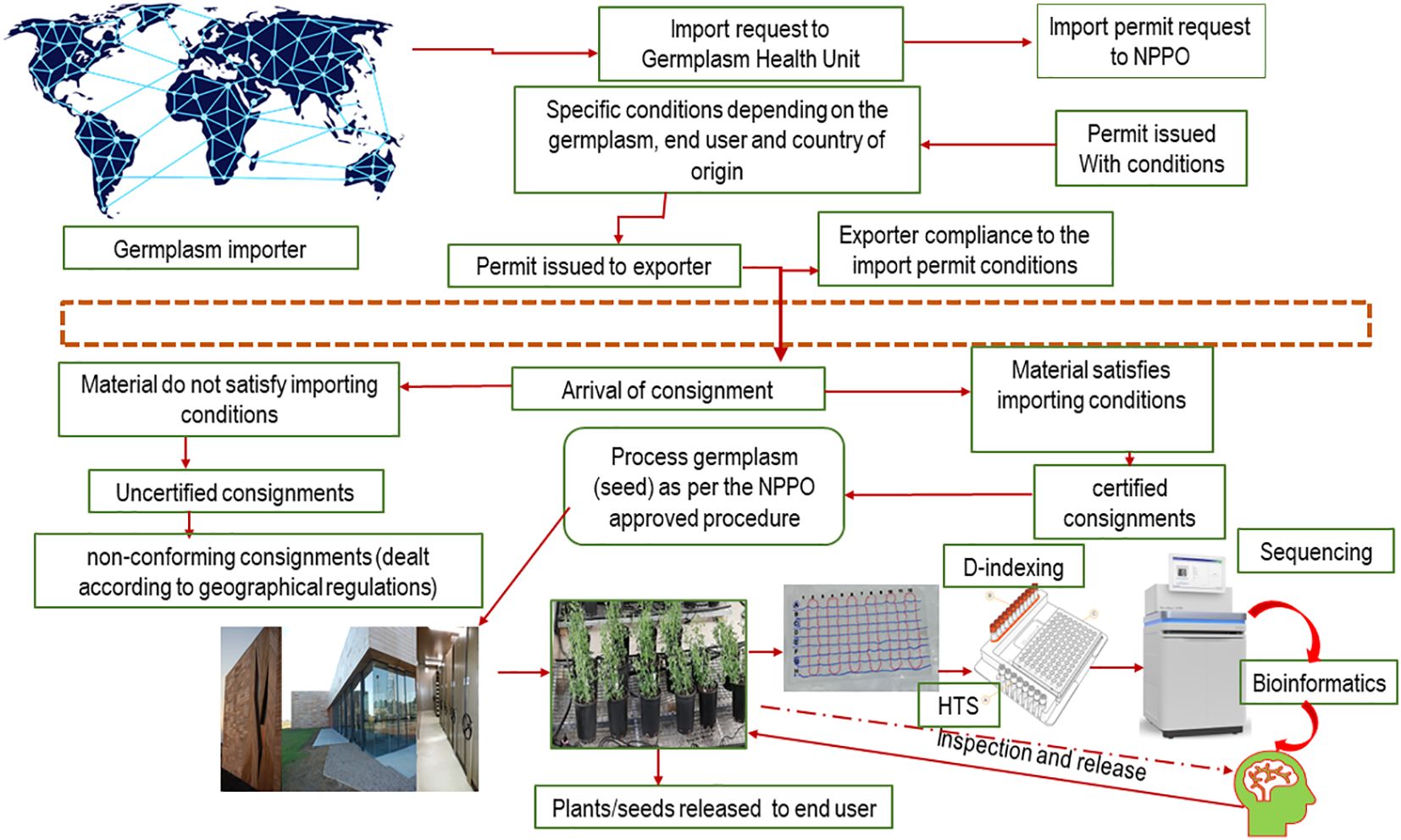
Figure 4 Flow chart representing future phytosanitary workflows integrating genomics innovative strategies to support virus interceptions within global seed movement.
Adoption of new technologies must include guidelines for technology implementation and analysis of the results. HTS has been routinely used for research for several years, but the regulatory space has struggled to keep up with technology development. However, in December 2022, HTS was adopted in Australian post entry quarantine, replacing PCR and biological indexing for pathogen screening of strawberries, stone fruit, almonds, raspberries, and blackberries. Currently, countries are at different stages of the adoption of HTS for routine screening in regulatory operations, and there is international pressure for a comprehensive standard governing the use of HTS in plant health certification (Lebas et al., 2022). Maree et al. (2018) proposed that HTS needs to be defined before its use in routine diagnostics and that appropriate controls need to be put in place to ensure the validity of the results. Collectively, in 2019, the Commission on Phytosanitary Measure, the governing body of the International Plant Protection Convention (IPPC), adopted a recommendation for preparing and benchmarking the use of HTS as a diagnostic tool for phytosanitary applications. This recommendation was expected to highlight the need for robust, HTS applications, including test design, validations, and quality assurance (FAO, 2019). Presently, a standard governing the use of HTS in plant health certification is of rising importance given the increase in global plant movements, both new variety introductions and agricultural trade products (Figure 4).
Perspective of HTS guideline results and interpretation
To achieve standardization of HTS in plant health, a range of practical factors need to be considered, including the limitations of laboratory and bioinformatic analyses, interpretation of results and their biological significance (Olmos et al., 2018). HTS-derived datasets should be thoroughly analyzed, followed by confirmation with molecular or serological methods and genomic data interpreted in a biological context, especially when a new infectious virus or viral strain is detected (Lebas et al., 2022). While not every HTS detection will indicate a new pathogen of biological significance. In a biosecurity context, biological examination of suspect material may include propagation of the material in controlled greenhouses and observation of symptoms. Uncommon biological symptoms or phenotypic traits may corroborate the presence of a new pathogen(s) (Wamaitha et al., 2018). Where there are no confirmatory tests available or any known indicator hosts, the sequence derived from the suspected pathogen should be used to design diagnostic primers followed by re-amplification of the original sample, or additional HTS prior to reporting. The subsequent amplicon can be sequenced, and where generic primers have been used for cloning, it should be used to deconvolute any mixed viral species (Zheng et al., 2010).
In rare circumstances, it might be impossible to confirm the identity of a virus detected in imported material. This could occur where very short counting sequences are detected in the raw data matching a suspected pathogen or if no known reference sequence is available in a global database to match the suspected sequence target. In such cases, the HTS results should be presented as provisional until confirmation, with further research work recommended. The results and subsequent interpretation should be carefully archived as a future confirmation reference. Where possible, the data should be shared in the local and global databases. Due to the universal nature of some HTS detection approaches, such as metagenomics (Budowle et al., 2014), its application in border plant health certification programs may create challenges such as false negatives or positives and the discovery of new unknown pathogens. Consequently, the detection of an unknown pathogen should be validated by designing new primers from the generated sequences and reamplifying the original nucleic acid extract followed by amplicon sequencing to confirm its specificity, repeatability, and reproducibility. Moreover, the bioinformatics analysis applied to a new unknown detection should also be validated using previously known datasets sequenced from a well-characterized virus (Massart et al., 2019) or a close species of the new threat detected. Within plant health certification diagnostic settings, a solid interpretation of virus-detected datasets should be sought in line with biological relevancy. The preliminary partial or full genome sequence information obtained must be kept, allowing reference predictions of future pathogens (Budowle et al., 2014). Massart et al. (2017) described critical risk assessment steps and consideration of unknown or less understood high-risk plant viruses. EPPO (2019) emphasizes archiving information such as prior provisional interpretations, identification approach, host species, source of the biological sample, and geographic origin.
Capacity-building initiatives
Despite rapid advances in genomics and computational science, there will likely be a lag time for HTS adoption in some regions, and for some commodities and viruses based on the cost and availability of resources and training. This was experienced during the early stages of the COVID-19 pandemic, when diagnostic and response capabilities were vastly different across the globe. Domestic and international frameworks are critical for the development, implementation, and coordination of robust plant health programs (Kumar et al., 2021). These frameworks could facilitate the establishment of programs in regions facing challenges, such as least developed countries, providing access to equipment, computing power and data storage capacity, and training in the interpretation of HTS datasets. An opportunity already exists via capacity-building initiatives of the Consortium of International Agricultural Research Centers Research Programs. Enhanced capability in new diagnostic technologies will facilitate the establishment and harmonization of plant health certification programs. Such efforts will enable quality standardization and harmonization of HTS protocols to avoid discrepancies in diagnostic results. In turn, this will reduce diagnostic complexities, potentially having significant implications for achieving sustainable viral disease management.
Recommendations
• Given the opportunities that have been realized by HTS in diagnostics, we cannot underestimate the importance of the systematic demand for quality library generation and data analysis. The plant health global community should adhere to standard operating procedures (SOPs) for data handling to prevent inconsistencies between plant certification programs and unnecessary obstruction of plant movement. This does not mean that everyone should adopt the same sequencing strategy using identical conditions, but their work should meet the minimum standardized conditions for sequence data collection to facilitate quality output and skill sharing. Such approaches could include online forum discussions through community repositories such as Zenodo. This would allow accessibility for both new users and less experienced laboratories to understand the development of individual HTS protocols, and the best way to utilize them. These efforts will contribute to achieving HTS global standards.
• The computing network capability of a diagnostic laboratory should allow easy transfer of HTS datasets from the sequencing provider or within intra-laboratory sequencing platforms. Systems to ensure successful and consistent data transfer processes and to mitigate potential downstream drawbacks should be carefully designed. Notably, technical issues arising from data transfer should be recorded thoroughly, transferred repeatedly, and factored in subsequent sequence analysis (Hébrant et al., 2018). The laboratory or center network should be safeguarded to mitigate data breaches and confidentiality threats by cyber-attacks or other online threats (Bertino and Sandhu, 2005; Alahmadi et al., 2022).
• When a new sequencing data analysis procedure is developed, it should be reviewed and approved by multiple quarantine or diagnostic agencies to meet international standards. This could be done by utilizing open-source platforms such as GitHub to collaborate, validate and share new informatics workflows.
• Before adopting HTS for plant health diagnostics, consideration should be made of operational costs and maintenance (Rehm et al., 2013), testing capacity, and technical bioinformatics capability coupled with skilled biological interpretation and decision-making surrounding positive detections, particularly of novel viruses or variants. Operational costs include the cost of cloud computing, for instance, the size of the HPC server and its associated data processing, data storage and computing systems administrators to support an ongoing routine service.
• Machine learning and other artificial intelligence (AI) approaches have unprecedented power to analyze these large datasets, offering remarkable opportunities for plant health diagnostics. Plant health programs and their stakeholders should prepare for both the benefits and potential challenges of new technologies.
Concluding remarks
In current modernization efforts, HTS has revolutionized plant, animal, and human virology by creating remarkable possibilities in virus and viroid discovery. In the future, HTS is envisaged to remain a backbone in crop disease management and safeguarding international transboundary movement of plants. As discussed in this review, it is prudent for the global research community to adopt a holistic international standard to harness this opportunity collaboratively, and support sustainable global disease management.
Author contributions
SM: Conceptualization, Writing – original draft, Writing – review & editing. ND: Writing – review & editing. KP: Writing – review & editing. DB: Writing – review & editing. BR: Writing – review & editing.
Funding
The author(s) declare financial support was received for the research, authorship, and/or publication of this article. We thank the Grains Research and Development Corporation for funding the National Grains Diagnostics and Surveillance Initiative (DPI2305-010RTX). The New South Wales Department of Primary Industries through the Centre for Invasive Species Solution for the additional funding.
Conflict of interest
The authors declare that the research was conducted in the absence of any commercial or financial relationships that could be construed as a potential conflict of interest.
The first author declared that he was an editorial board member of Frontiers, at the time of submission. This had no impact on the peer review process and the final decision.
Publisher’s note
All claims expressed in this article are solely those of the authors and do not necessarily represent those of their affiliated organizations, or those of the publisher, the editors and the reviewers. Any product that may be evaluated in this article, or claim that may be made by its manufacturer, is not guaranteed or endorsed by the publisher.
References
Adams I. P., Glover R. H., Monger W. A., Mumford R., Jackeviciene E., Navalinskiene M., et al. (2009). Next-generation sequencing and metagenomic analysis: a universal diagnostic tool in plant virology. Mol. Plant Pathol 10, 537–545. doi: 10.1111/j.1364-3703.2009.00545.x
Adams I. P., Miano D. W., Kinyua Z. M., Wangai A., Kimani E., Phiri N., et al. (2013). Use of next-generation sequencing for the identification and characterization of Maize chlorotic mottle virus and Sugarcane mosaic virus causing maize lethal necrosis in Kenya. Plant Pathol. 62, 741–749. doi: 10.1111/j.1365-3059.2012.02690.x
Ahmed M., Back M., Prior T., Karssen G., Lawson R., Adams I., et al. (2019). Metabarcoding of soil nematodes: the importance of taxonomic coverage and availability of reference sequences in choosing suitable marker (s). Metabarcoding Metagenomics 3, 77–99. doi: 10.3897/mbmg.3.36408
Alahmadi A. N., Rehman S. U., Alhazmi H. S., Glynn D. G., Shoaib H., Solé P. (2022). Cyber-security threats and side-channel attacks for digital agriculture. Sensors 22, 3520. doi: 10.3390/s22093520
Alcalá Briseño R. I., Batuman O., Brawner J., Cuellar W. J., Delaquis E., Etherton B. A., et al. (2023). Translating virome analyses to support biosecurity, on-farm management, and crop breeding. Front. Plant Sci. 14, 1056603. doi: 10.3389/fpls.2023.1056603
Al Rwahnih M., Daubert S., Golino D., Islas C., Rowhani A. (2015). Comparison of next-generation sequencing versus biological indexing for the optimal detection of viral pathogens in grapevine. Phytopathology 105, 758–763. doi: 10.1094/PHYTO-06-14-0165-R
Al Rwahnih M., Daubert S., Golino D., Rowhani A. (2009). Deep sequencing analysis of RNAs from a grapevine showing Syrah decline symptoms reveals a multiple virus infection that includes a novel virus. Virology 387, 395–401. doi: 10.1016/j.virol.2009.02.028
Anderson P. K., Cunningham A. A., Patel N. G., Morales F. J., Epstein P. R., Daszak P. (2004). Emerging infectious diseases of plants: pathogen pollution, climate change and agrotechnology drivers. Trends Ecol. Evol. 19, 535–544. doi: 10.1016/j.tree.2004.07.021
Baid G., Cook D. E., Shafin K., Yun T., Llinares-López F., Berthet Q., et al. (2023). Deep Consensus improves the accuracy of sequences with a gap-aware sequence transformer. Nat. Biotechnol. 41, 232–238.
Bailey P., Chang D. K., Nones K., Johns A. L., Patch A., Gingras M., et al. (2016). Genomic analyses identify molecular subtypes of pancreatic cancer. Nature 531, 47–52. doi: 10.1038/nature16965
Baizan-Edge A., Cock P., MacFarlane S., McGavin W., Torrance L., Jones S. (2019). Kodoja: A workflow for virus detection in plants using k-mer analysis of RNA-sequencing data. J. Gen. Virol. 100, 533–542. doi: 10.1099/jgv.0.001210
Ballenghien M., Faivre N., Galtier N. (2017). Patterns of cross-contamination in a multispecies population genomic project: detection, quantification, impact, and solutions. BMC Biol. 15, 1–16. doi: 10.1186/s12915-017-0366-6
Bankevich A., Nurk S., Antipov D., Gurevich A. A., Dvorkin M., Kulikov A. S., et al. (2012). SPAdes: A new genome assembly algorithm and its applications to single-cell sequencing. J. Comput. Biol. 19, 455–477. doi: 10.1089/cmb.2012.0021
Barba M., Czosnek H., Hadidi A. (2014). Historical perspective, development and applications of next-generation sequencing in plant virology. Viruses 6, 106–136. doi: 10.3390/v6010106
Barrero R. A., Napier K. R., Cunnington J., Liefting L., Keenan S., Frampton R. A., et al. (2017). An internet-based bioinformatics toolkit for plant biosecurity diagnosis and surveillance of viruses and viroids. BMC Bioinf. 18, 1–12. doi: 10.1186/s12859-016-1428-4
Bentley D. R., Balasubramanian S., Swerdlow H. P., Smith G. P., Milton J., Brown C. G., et al. (2008). Accurate whole human genome sequencing using reversible terminator chemistry. Nature 456, 53–59. doi: 10.1038/nature07517
Bertino E., Sandhu R. (2005). Database security-concepts, approaches, and challenges. IEEE Trans. Dependable secure computing 2, 2–19. doi: 10.1109/TDSC.2005.9
Bester R., Steyn C., Breytenbach J. H., de Bruyn R., Cook G., Maree H. J. (2022). Reproducibility and sensitivity of high-throughput sequencing (HTS)-based detection of citrus tristeza virus and three citrus viroids. Plants 11, 1939. doi: 10.3390/plants11151939
Bolger A. M., Lohse M., Usadel B. (2014). Trimmomatic: a flexible trimmer for Illumina sequence data. Bioinformatics 30, 2114–2120. doi: 10.1093/bioinformatics/btu170
Boonham N., Kreuze J., Winter S., van der Vlugt R., Bergervoet J., Tomlinson J., et al. (2014). Methods in virus diagnostics: from ELISA to next generation sequencing. Virus Res. 186, 20–31. doi: 10.1016/j.virusres.2013.12.007
Borgström E., Lundin S., Lundeberg J. (2011). Large scale library generation for high throughput sequencing. PloS One 6, e19119. doi: 10.1371/journal.pone.0019119
Branton D., Deamer D. W. (2019). Nanopore sequencing: an introduction (Singapore: World Scientific). doi: 10.1142/10995
Briese T., Kapoor A., Mishra N., Jain K., Kumar A., Jabado O. J., et al. (2015). Virome capture sequencing enables sensitive viral diagnosis and comprehensive virome analysis. MBio. 6 (5), 10–1128.
Brinkmann A., Andrusch A., Belka A., Wylezich C., Höper D., Pohlmann A., et al. (2019). Proficiency testing of virus diagnostics based on bioinformatics analysis of simulated in silico high-throughput sequencing data sets. J. Clin. Microbiol. 57 (8), 10–1128.
Bryant D. W., Priest H. D., Mockler T. C. (2012). “Detection and quantification of alternative splicing variants using RNA-seq,” in RNA abundance analysis (Humana Press, Totowa, NJ), 97–110).
Buschmann T., Zhang R., Brash D. E., Bystrykh L. V. (2014). Enhancing the detection of barcoded reads in high throughput DNA sequencing data by controlling the false discovery rate. BMC Bioinf. 15, 264.
Byrne A., Cole C., Volden R., Vollmers C. (2019). Realizing the potential of full-length transcriptome sequencing Phil. Trans. R. Soc. doi: 10.1098/rstb.2019.0097
Budowle B., Connell N. D., Bielecka-Oder A., Colwell R. R., Corbett C. R., Fletcher J., et al. (2014). Validation of high throughput sequencing and microbial forensics applications. Invest. Genet. 5, 1–18. doi: 10.1186/2041-2223-5-9
Burland T. G. (2000). DNASTAR’s Lasergene sequence analysis software. Methods Mol Biol. 132, 71–91. doi: 10.1385/1-59259-192-2:71
Bushnell B. (2014). BBMap: a fast, accurate, splice-aware aligner (No. LBNL-7065E) (Berkeley, CA (United States: Lawrence Berkeley National Lab.(LBNL).
Campbell-Lendrum D., Neville T., Schweizer C., Neira M. (2023). Climate change and health: three grand challenges. Nat. Med. 29, 1631–1638. doi: 10.1038/s41591-023-02438-w
Chen P., Sun Z., Wang J., Liu X., Bai Y., Chen J., et al. (2023). Portable nanopore-sequencing technology: Trends in development and applications. Front. Microbiol. 14, 1043967. doi: 10.3389/fmicb.2023.1043967
Chen S., Zhou Y., Chen Y., Gu J. (2018). fastp: an ultra-fast all-in-one FASTQ preprocessor. Bioinformatics 34, i884–i890. doi: 10.1093/bioinformatics/bty560
Cole C., Byrne A., Adams M., Volden R., Vollmers C. (2020). Complete characterization of the human immune cell transcriptome using accurate full-length cDNA sequencing. Genome Res. 30 (4), 589–601.
Costello M., Fleharty M., Abreu J., Farjoun Y., Ferriera S., Holmes L., et al. (2018). Characterization and remediation of sample index swaps by non-redundant dual indexing on massively parallel sequencing platforms. BMC Genomics 19, 1–10. doi: 10.1186/s12864-018-4703-0
Dolja V. V., Meng B., Martelli G. P. (2017). “Evolutionary aspects of grapevine virology,” in Grapevine viruses: molecular biology, diagnostics and management. Eds. Meng B., Martelli G., Golino D., Fuchs M. (Springer, Cham), 659–688.
Eid J., Fehr A., Gray J., Luong K., Lyle J., Otto G., et al. (2009). Real-time DNA sequencing from single polymerase molecules. Science 323, 133–138. doi: 10.1126/science.1162986
EPPO (2019). PM 7/77 (3). Documentation and reporting on a diagnosis Vol. 49 (EPPO Bulletin), 527–529.
EPPO. (2021). PM 7/98 (5). Specific requirements for laboratories preparing accreditation for a plant pest diagnostic activity. EPPO Bull. 51, 468–498.
F.A.O. (2019). Available at: https://www.ippc.int/static/media/files/publication/en/2019/05/R_08_En_2019-05-06_Post-CPM-14.pdf.
Filloux D., Fernandez E., Loire E., Claude L., Galzi S., Candresse T., et al. (2018). Nanopore-based detection and characterization of yam viruses. Sci. Rep. 8, 17879. doi: 10.1038/s41598-018-36042-7
Fitzpatrick A. H., Rupnik A., O’Shea H., Crispie F., Keaveney S., Cotter P. (2021). High throughput sequencing for the detection and characterization of RNA viruses. Front. Microbiol. 12, 621719. doi: 10.3389/fmicb.2021.621719
Fontdevila Pareta N., Khalili M., Maachi A., Rivarez M. P. S., Rollin J., Salavert F., et al. (2023). Managing the deluge of newly discovered plant viruses and viroids: an optimized scientific and regulatory framework for their characterization and risk analysis. Front. Microbiol. 14, 1181562. doi: 10.3389/fmicb.2023.1181562
Fonzino A., Manzari C., Spadavecchia P., Munagala U., Torrini S., Conticello S., et al. (2024). Unraveling C-to-U RNA editing events from direct RNA sequencing. RNA biology 21 (1), 1–14. doi: 10.1080/15476286.2023.2290843
Gaffney J., Girma D., Kane N. A., Llaca V., Mace E., Taylor N., et al. (2022). Maximizing value of genetic sequence data requires an enabling environment and urgency. Global Food Secur. 33, 100619. doi: 10.1016/j.gfs.2022.100619
Galaxy Community. (2022). The Galaxy platform for accessible, reproducible and collaborative biomedical analyses: 2022 update. Nucleic Acids Res. 50W1, W345–W351. doi: 10.1093/nar/gkac247
Garalde D. R., Snell E. A., Jachimowicz D., Sipos B., Lloyd J. H., Bruce M., et al. (2018). Highly parallel direct RNA sequencing on an array of nanopores. Nat. Methods 15, 201–206. doi: 10.1038/nmeth.4577
Gao Y., Liu X., Wu B., Wang H., Xi F., Kohnen M. V., et al. (2021). Quantitative profiling of N6-methyladenosine at single-base resolution in stem-differentiating xylem of Populus trichocarpa using nanopore direct RNA sequencing. Genome Biol. 22 (1), 22. doi: 10.1186/s13059-020-02241-7
Geering A. D. (2014). Caulimoviridae (plant pararetroviruses). Chichester, United Kingdom: John Wiley & Sons, Ltd.
Gergerich R. C., Welliver R. A., Gettys S., Osterbauer N. K., Kamenidou S., Martin R. R., et al. (2015). Safeguarding fruit crops in the age of agricultural globalization. Plant Dis. 99, 176–187. doi: 10.1094/PDIS-07-14-0762-FE
Goldberg C. S., Turner C. R., Deiner K., Klymus K. E., Thomsen P. F., Murphy M. A., et al. (2016). Critical considerations for the application of environmental DNA methods to detect aquatic species. Methods Ecol. Evol. 7, 1299–1307. doi: 10.1111/2041-210X.12595
Grabherr M. G., Haas B. J., Yassour M., Levin J. Z., Thompson D. A., Amit I., et al. (2011). Full-length transcriptome assembly from RNA-Seq data without a reference genome. Nature. Biotechnol. 29, 644–652. doi: 10.1038/nbt.1883
Grzesik P., Augustyn D. R., Wyciślik Ł., Mrozek D. (2022). Serverless computing in omics data analysis and integration. Briefings Bioinf. 23, bbab349. doi: 10.1093/bib/bbab349
Gullino M. L., Albajes R., Al-Jboory I., Angelotti F., Chakraborty S., Garrett K. A., et al. (2021). Scientific Review of the Impact of Climate Change on Plant Pests. Scientific review of the impact of climate change on plant pests-A global challenge to prevent and mitigate plant pest risks in agriculture, forestry and ecosystems (Secretariat of the International Plant Protection Convention: FAO).
Hadidi A. (2019). Next-generation sequencing and CRISPR/cas13 editing in viroid research and molecular diagnostics. Viruses 11, 120. doi: 10.3390/v11020120
Hall A., Hallowell N., Zimmern R. (2013). Managing incidental and pertinent findings from WGS in the 100,000 Genomes Project (Cambridge: PHG Foundation).
Hardwick K. M., Ojwang’ A. M. E., Stomeo F., Maina S., Bichang’a G., Calatayud P. A., et al. (2019). Draft genome of Busseola fusca, the maize stalk borer, a major crop pest in Sub-Saharan Africa. Genome Biol. Evol. 11, 2203–2207. doi: 10.1093/gbe/evz166
Havlová K., Fajkus J. (2020). G4 structures in control of replication and transcription of rRNA genes. Front. Plant Sci. 11, 593692. doi: 10.3389/fpls.2020.593692
Hébrant A., Froyen G., Maes B., Salgado R., Le Mercier M., D'Haene N., et al. (2018). The Belgian next generation sequencing guidelines for haematological and solid tumours. Belgian J. Med. Oncol. 11 (2), 56–67.
Hohn T., Richert-Pöggeler K. R., Staginnus C., Harper G., Schwarzacher T., Teo C. H., et al. (2008). Evolution of integrated plant viruses. Plant Virus Evol., 53–81. doi: 10.1007/978-3-540-75763-4_4
Hotaling S., Wilcox E. R., Heckenhauer J., Stewart R. J., Frandsen P. B. (2023). Highly accurate long reads are crucial for realizing the potential of biodiversity genomics. BMC Genomics 24, 1–9. doi: 10.1186/s12864-023-09193-9
Ho T., Tzanetakis I. E. (2014). Development of a virus detection and discovery pipeline using next generation sequencing. Virology 471, 54–60.
Irish J., Rose Figura J., Sandhu S., Lahann B., Kurihara L., Makarov V. (2018). “July. Improved sample indexing for high fidelity demultiplexing to increase confidence in low frequency variant calling,” in Cancer Research (Vol. 78, No. 13). 615 Chestnut st, 17th floor (American Association cancer research, Philadelphia, USA), 19106–14404.
Jakse J., Radisek S., Pokorn T., Matousek J., Javornik B. (2015). Deep-sequencing revealed Citrus bark cracking viroid (CBCV d) as a highly aggressive pathogen on hop. Plant Pathol. 64, 831–842. doi: 10.1111/ppa.12325
Johnson M. T., Carpenter E. J., Tian Z., Bruskiewich R., Burris J. N., Carrigan C. T., et al. (2012). Evaluating methods for isolating total RNA and predicting the success of sequencing phylogenetically diverse plant transcriptomes. PloS One 7, e50226. doi: 10.1371/journal.pone.0050226
Jones R. A. C. (2009). Plant virus emergence and evolution: origins, new encounter scenarios, factors driving emergence, effects of changing world conditions, and prospects for control. Virus Res. 141, 113–130. doi: 10.1016/j.virusres.2008.07.028
Jones R. A. C., Naidu R. A. (2019). Global dimensions of plant virus diseases: Current status and future perspectives. Annu. Rev. Virol. 6, 387–409.
Kearse M., Sturrock S., Meintjes P. (2012). The Geneious 6.0. 3 read mapper (Auckland, New Zealand: Biomatters, Ltd).
Keegan K. P., Glass E. M., Meyer F. (2016). “MG-RAST, a metagenomics service for analysis of microbial community structure and function,” in Microbial environmental genomics (MEG), 207–233.
Kimotho R. N., Maina S. (2024). Unraveling plant-microbe interactions using integrated omics approaches. J. Exp. Bot. 75, 1289–1313. doi: 10.1093/jxb/erad448
Kircher M., Sawyer S., Meyer M. (2012). Double indexing overcomes inaccuracies in multiplex sequencing on the Illumina platform. Nucleic Acids Res. 40, e3–e3. doi: 10.1093/nar/gkr771
Kolmogorov M., Bickhart D. M., Behsaz B., Gurevich A., Rayko M., Shin S. B., et al. (2020). metaFlye: scalable long-read metagenome assembly using repeat graphs. Nat. Methods 17, 1103–1110. doi: 10.1038/s41592-020-00971-x
Kreuze J. F., Perez A., Untiveros M., Quispe D., Fuentes S., Barker I., et al. (2009). Complete viral genome sequence and discovery of novel viruses by deep sequencing of small RNAs: A generic method for diagnosis, discovery and sequencing of viruses. Virology 388, 1–7. doi: 10.1016/j.virol.2009.03.024
Krueger F. (2012) Trim Galore: a wrapper tool around Cutadapt and FastQC to consistently apply quality and adapter trimming to FastQ files, with some extra functionality for MspI-digested RRBS-type (Reduced Representation Bisufite-Seq) libraries. Available online at: http://www.bioinformatics.babraham.ac.uk/projects/trim_galore/ (Accessed access: 28/04/2016).
Krueger F., Trim Galore (2015). Available at: http://www.bioinformatics.babraham.ac.uk/projects/trim_galore.
Kumar P. L., Cuervo M., Kreuze J. F., Muller G., Kulkarni G., Kumari S. G., et al. Phytosanitary Interventions for Safe Global Germplasm Exchange and the Prevention of Transboundary Pest Spread: The Role of CGIAR Germplasm Health Units. Plants 10 (2), 328. doi: 10.3390/plants10020328
Kwon S., Lee B., Yoon S. (2014). CASPER: context-aware scheme for paired-end reads from high-throughput amplicon sequencing. BMC Bioinf. 15 (Suppl. 9), S10.
Lambert C., Braxton C., Charlebois R. L., Deyati A., Duncan P., La Neve F., et al. (2018). Considerations for optimization of high-throughput sequencing bioinformatics pipelines for virus detection. Viruses 10, 528. doi: 10.3390/v10100528
Langmead B., Salzberg S. L. (2012). Fast gapped-read alignment with Bowtie 2. Nat. Methods 9, 357–359. doi: 10.1038/nmeth.1923
Larsson A. J., Stanley G., Sinha R., Weissman I. L., Sandberg R. (2018). Computational correction of index switching in multiplexed sequencing libraries. Nat. Methods 15, 305–307. doi: 10.1038/nmeth.4666
Lathe W., Williams J., Mangan M., Karolchik D. (2008). Genomic data resources: challenges and promises. Nat. Educ. 1, 2.
Laver T., Harrison J., O’Neill P. A., Moore K., Farbos A., Paszkiewicz K., et al. (2015). Assessing the performance of the Oxford Nanopore technologies minion. Biomolecular detection quantification 3, 1–8. doi: 10.1016/j.bdq.2015.02.001
Lebas B., Adams I., Al Rwahnih M., Baeyen S., Bilodeau G. J., Blouin A. G., et al. (2022). Facilitating the adoption of high-throughput sequencing technologies as a plant pest diagnostic test in laboratories: A step-by-step description. EPPO Bull. 52, 394–418. doi: 10.1111/epp.12863
Levin J. Z., Yassour M., Adiconis X., Nusbaum C., Thompson D. A., Friedman N., et al. (2010). Comprehensive comparative analysis of strand-specific RNA sequencing methods. Nat. Methods 7, 709–715. doi: 10.1038/nmeth.1491
Li H. (2018). Minimap2: pairwise alignment for nucleotide sequences. Bioinformatics 34, 3094–3100. doi: 10.1093/bioinformatics/bty191
Liao Y., Shi W. (2020). Read trimming is not required for mapping and quantification of RNA-seq reads at the gene level. NAR Genomics Bioinf. 2, lqaa068. doi: 10.1093/nargab/lqaa068
Loman N. J., Misra R. V., Dallman T. J., Constantinidou C., Gharbia S. E., Wain J., et al. (2012). Performance comparison of benchtop high-throughput sequencing platforms. Nat. Biotechnol. 30, pp.434–pp.439. doi: 10.1038/nbt.2198
MacConaill L. E., Burns R. T., Nag A., Coleman H. A., Slevin M. K., Giorda K., et al. (2018). Unique, dual-indexed sequencing adapters with UMIs effectively eliminate index cross-talk and significantly improve sensitivity of massively parallel sequencing. BMC Genomics 19, 1–10. doi: 10.1186/s12864-017-4428-5
Mackie J., Kinoti W. M., Chahal S. I., Lovelock D. A., Campbell P. R., Tran-Nguyen L. T., et al. (2022). Targeted whole genome sequencing (TWG-seq) of cucumber green mottle mosaic virus using tiled amplicon multiplex PCR and nanopore sequencing. Plants 11, 2716. doi: 10.3390/plants11202716
Magoč T., Salzberg S. L. (2011). FLASH: fast length adjustment of short reads to improve genome assemblies. Bioinformatics 27, 2957–2963. doi: 10.1093/bioinformatics/btr507
Maina S., Barbetti M. J., Edwards O., de Almeida L., Ximenes A., Jones R. A. C. (2018a). Sweet potato feathery mottle virus and Sweet potato virus C from East Timorese and Australian sweetpotato: Biological and molecular properties, and biosecurity implications. Plant Dis. 102, 589–599. doi: 10.1094/PDIS-08-17-1156-RE
Maina S., Barbetti M. J., Edwards O. R., Minemba D., Areke M. W., Jones R. A. C. (2018b). First complete genome sequence of Cucurbit aphid-borne yellows virus from Papua New Guinea. Genome Announcements 6, e00162–e00118. doi: 10.1128/genomeA.00162-18
Maina S., Barbetti M. J., Edwards O. R., Minemba D., Areke M. W., Jones R. A. C. (2019a). Zucchini yellow mosaic virus genomic sequences from Papua New Guinea: Lack of genetic connectivity with Northern Australian or East Timorese genomes, and new recombination findings. Plant Dis. 103, 1326–1336. doi: 10.1094/PDIS-09-18-1666-RE
Maina S., Barbetti M. J., Edwards O. R., Minemba D., Areke M. W., Jones R. A. C. (2019b). Genetic connectivity Papaya ringspot virus genomes from Papua New Guinea and northern Australia, and new recombination insights. Plant Dis. doi: 10.1094/PDIS-07-18-1136-RE
Maina S., Barbetti M. J., Martin D. P., Edwards O. R., Jones R. A. C. (2018c). New isolates of Sweet potato feathery mottle virus and Sweet potato virus C: Biological and molecular properties, and recombination analysis based on complete genomes. Plant Dis. 102, 1899–1914. doi: 10.1094/PDIS-12-17-1972-RE
Maina S., Coutts B. A., Edwards O. R., de Almeida L., Kehoe M. A., Ximenes A., et al. (2017a). Zucchini yellow mosaic virus populations from East Timorese and northern Australian cucurbit crops: Molecular properties, genetic connectivity and biosecurity implications. Plant Dis. 101, 1236–1245. doi: 10.1094/PDIS-11-16-1672-RE
Maina S., Coutts B. A., Edwards O. R., de Almeida L., Ximenes A., Jones R. A. C. (2017b). Papaya ringspot virus populations from East Timorese and northern Australian cucurbit crops: Biological and molecular properties, and absence of genetic connectivity. Plant Dis. 101, 985–993. doi: 10.1094/PDIS-10-16-1499-RE
Maina S., Edwards O. R., Barbetti M. J., de Almeida L., Ximenes A., Jones R. A. C. (2016a). Deep sequencing reveals complete genome of Sweet potato virus G from East Timor. Genome Announcements 4, e00957–e00916. doi: 10.1128/genomeA.00957-16
Maina S., Edwards O. R., de Almeida L., Ximenes A., Jones R. A. C. (2016b). Complete genome sequences of the Carlavirus Sweet potato chlorotic fleck virus from East Timor and Australia. Genome Announcements 4, e00414–e00416. doi: 10.1128/genomeA.00414-16
Maina S., Edwards O. R., de Almeida L., Ximenes A., Jones R. A. C. (2016c). Complete genome sequences of the Potyvirus Sweet potato virus 2 from East Timor and Australia. Genome Announcements 4, e00504–e00516. doi: 10.1128/genomeA.00504-16
Maina S., Edwards O. R., de Almeida L., Ximenes A., Jones R. A. C. (2016d). First complete genome sequence of Suakwa aphid-borne yellows virus from East Timor. Genome Announcements 4, e00718–e00716. doi: 10.1128/genomeA.00718-16
Maina S., Edwards O. R., de Almeida L., Ximenes A., Jones R. A. C. (2016e). First complete genome sequence of Bean common mosaic necrosis virus from East Timor. Genome Announcements 4, e01049–e01016. doi: 10.1128/genomeA.01049-16
Maina S., Edwards O. R., de Almeida L., Ximenes A., Jones R. A. C. (2017c). Metagenomic analysis of cucumber RNA from Timor-Leste reveals an Aphid lethal paralysis virus genome. Genome Announcements 5, e01445–e01416. doi: 10.1128/genomeA.01445-16
Maina S., Edwards O. R., de Almeida L., Ximenes A., Jones R. A. C. (2017d). RNA-seq strand specific library from East Timorese cucumber sample reveals complete Cucurbit aphid-borne yellows virus genome. Genome Announcements 5, e00320–e00317. doi: 10.1128/genomeA.00320-17
Maina S., Edwards O. R., de Almeida L., Ximenes A., Jones R. A. C. (2017e). First complete Squash leaf curl China virus genomic segment DNAA sequence from Timor-Leste. Genome Announcements 5, e00483–e00417. doi: 10.1128/genomeA.00483-17
Maina S., Edwards O. R., Jones R. A. C. (2016f). First complete genome sequence of Pepper vein yellows virus from Australia. Genome Announcements 4, e00450–e00416. doi: 10.1128/genomeA.00450-16
Maina S., Edwards O. R., Jones R. A. C. (2017f). Two complete genome sequences of Squash mosaic virus from 20-year-old cucurbit leaf samples from Australia. Genome Announcements 5, e00778–e00717. doi: 10.1128/genomeA.00778-17
Maina S., Jones R. A. C. (2017g). Analysis of an RNA-seq strand-specific library sample reveals a complete genome of Hardenbergia mosaic virus from native wisteria, an indigenous virus from Southwest Australia. Genome Announcements 5, e00599–e00517. doi: 10.1128/genomeA.00599-17
Maina S., Jones R. A. C. (2023). Enhancing biosecurity against virus disease threats to Australian grain crops: current situation and future prospects. Front. Horticulture 2, 1263604. doi: 10.3389/fhort.2023.1263604
Maina S., Norton S. L., McQueen V. L., King S., Rodoni B. (2022). Oxford Nanopore Sequencing Reveals an Exotic Broad bean mottle virus Genome within Australian Grains Post-Entry Quarantine. Microbiol. Resource Announcements 11, e00211–e00222. doi: 10.1128/mra.00211-22
Maina S., Norton S. L., Rodoni B. C. (2023). Hybrid RNA sequencing of broad bean wilt virus 2 from faba beans. Microbiol. Spectr., e02663–e02623. doi: 10.1128/spectrum.02663-23
Maina S., Zheng L., Kinoti W. M., Aftab M., Nancarrow N., Trębicki P., et al. (2019c). Metagenomic analysis reveals a nearly complete genome sequence of alfalfa mosaic virus from a field pea in Australia. Microbiol. Resource Announcements 8, pp.10–p1128. doi: 10.1128/MRA.00766-19
Maina S., Zheng L., Rodoni B. C. (2021). Targeted genome sequencing (TG-Seq) approaches to detect plant viruses. Viruses 13, 583. doi: 10.3390/v13040583
Malapi-Wight M., Adhikari B., Zhou J., Hendrickson L., Maroon-Lango C. J., McFarland C., et al. (2021). HTS-based diagnostics of sugarcane viruses: Seasonal variation and its implications for accurate detection. Viruses 13, 1627. doi: 10.3390/v13081627
Maliogka V. I., Minafra A., Saldarelli P., Ruiz-García A. B., Glasa M., Katis N., et al. (2018). Recent advances on detection and characterization of fruit tree viruses using high-throughput sequencing technologies. Viruses 10, 436. doi: 10.3390/v10080436
Maree H. J., Fox A., Al Rwahnih M., Boonham N., Candresse T. (2018). Application of HTS for routine plant virus diagnostics: State of the art and challenges. Front. Plant Sci. 9, 1082. doi: 10.3389/fpls.2018.01082
Martin M. (2011). Cutadapt removes adapter sequences from high-throughput sequencing reads. EMBnet J. 17, pp.10–pp.12. doi: 10.14806/ej.17.1
Martin R. R., Tzanetakis I. E. (2015). “Control of virus diseases of berry crops,” in Advances in virus research, vol. 91. (Academic Press), 271–309).
Massart S., Olmos A., Jijaki H., Candresse T. (2014). Current impact and future directions of high throughput sequencing in plant virus diagnostic. Virus Res. 188 (8), 90–96.
Massart S., Candresse T., Gil J., Lacomme C., Predajna L., Ravnikar M., et al. (2017). A framework for the evaluation of biosecurity, commercial, regulatory, and scientific impacts of plant viruses and viroids identified by NGS technologies. Front. Microbiol. 8, 45. doi: 10.3389/fmicb.2017.00045
Massart S., Chiumenti M., De Jonghe K., Glover R., Haegeman A., Koloniuk I., et al. (2019). Virus detection by high-throughput sequencing of small RNAs: Large-scale performance testing of sequence analysis strategies. Phytopathology 109, 488–497. doi: 10.1094/PHYTO-02-18-0067-R
Mbeyagala E. K., Maina S., Macharia M. W., Mukasa S. B., Holton T. (2019). Illumina sequencing reveals the first near-complete genome sequence of Ugandan passiflora virus. Microbiol. Resource Announcements 8, 10–1128. doi: 10.1128/MRA.00358-19
Meredith L. W., Hamilton W. L., Warne B., Houldcroft C. J., Hosmillo M., Jahun A. S., et al. (2020). Rapid implementation of SARS-CoV-2 sequencing to investigate cases of health-care associated COVID-19: a prospective genomic surveillance study. Lancet Infect. Dis. 20, 1263–1271. doi: 10.1016/S1473-3099(20)30562-4
Meyer F., Paarmann D., D’Souza M., Olson R., Glass E. M., Kubal M., et al. (2008). The Metagenomics RAST Server—A Public Resource for the Automatic Phylogenetic and Functional Analysis of Metagenomes. BMC Bioinf. 9.
Modi A., Vai S., Caramelli D., Lari M. (2021). “The Illumina sequencing protocol and the NovaSeq 6000 system,” in Bacterial pangenomics (Humana, New York, NY), 15–42.
Mueller O., Lightfoot S., Schroeder A. (2004). RNA integrity number (RIN)–standardization of RNA quality control. Agilent Appl. note Publ. 1, 1–8.
Mulholland S., Wildman O., Kinoti W. M., Constable F., Daly A., Tesoriero L., et al. (2023). First report of watermelon crinkle leaf associated virus-1 (WCLaV-1) in watermelon (Citrullus lanatus) in Australia. J. Plant Pathol. 105, 295–297. doi: 10.1007/s42161-022-01250-8
Mwaikono K. S., Maina S., Sebastian A., et al. (2016). High-throughput sequencing of 16S rRNA Gene Reveals Substantial Bacterial Diversity on the Municipal Dumpsite. BMC Microbiol. 16, 145. doi: 10.1186/s12866-016-0758-8
Nagano A. J., Honjo M. N., Mihara M., Sato M., Kudoh H. (2015). Detection of Plant Viruses in Natural Environments by Using RNA-Seq. Methods Mol. Biol. 1236, 89–98.
Nancarrow N., Aftab M., Zheng L., Maina S., Freeman A., Rodoni B., et al. (2019a). First report of barley virus G in Australia. Plant Dis. 103, 1799. doi: 10.1094/PDIS-01-19-0166-PDN
Ndunguru J., Sseruwagi P., Tairo F., Stomeo F., Maina S., Djinkeng A., et al. (2015). Analyses of twelve new whole genome sequences of cassava brown streak viruses and Ugandan cassava brown streak viruses from East Africa: diversity, supercomputing and evidence for further speciation. PloS One 10, e0139321.
Nelson M. C., Morrkathison H. G., Benjamino J., Grim S. L., Graf J. (2014). Analysis, optimization and verification of Illumina-generated 16S rRNA gene amplicon surveys. PloS One 9, e94249. doi: 10.1371/journal.pone.0094249
Nurk S., Meleshko D., Korobeynikov A., Pevzner P. A. (2017). metaSPAdes: New versatile metagenomic assembler. Genome Res. 27 (5), 824–834.
Olmos A., Boonham N., Candresse T., Gentit P., Giovani B., Kutnjak D., et al. (2018). High-throughput sequencing technologies for plant pest diagnosis: challenges and opportunities. EPPO Bull. 48, 219–224. doi: 10.1111/epp.12472
Pappas N., Roux S., Hölzer M., Lamkiewicz K., Mock F., Marz M., et al. (2021). “Virus bioinformatics,” in Encyclopedia of virology, 124–132. doi: 10.1016/B978-0-12-814515-9.00034-5
Pecman A., Adams I., Gutiérrez-Aguirre I., Fox A., Boonham N., Ravnikar M., et al. (2022). Systematic comparison of nanopore and Illumina sequencing for the detection of plant viruses and viroids using total RNA sequencing approach. Front. Microbiol. 13, 883921. doi: 10.3389/fmicb.2022.883921
Pecman A., Kutnjak D., Gutiérrez-Aguirre I., Adams I., Fox A., Boonham N., et al. (2017). Next generation sequencing for detection and discovery of plant viruses and viroids: Comparison of two approaches. Front. Microbiol. 8, 1998. doi: 10.3389/fmicb.2017.01998
Piper A. M., Batovska J., Cogan N. O., Weiss J., Cunningham J. P., Rodoni B. C., et al. (2019). Prospects and challenges of implementing DNA metabarcoding for high-throughput insect surveillance. GigaScience 8, giz092. doi: 10.1093/gigascience/giz092
Potapov P., Turubanova S., Hansen M. C., Tyukavina A., Zalles V., Khan A., et al. (2022). Global maps of cropland extent and change show accelerated cropland expansion in the twenty-first century. Nat. Food 3, 19–28. doi: 10.1038/s43016-021-00429-z
Pruitt K. D., Tatusova T., Maglott D. R. (2005). NCBI Reference Sequence (RefSeq): a curated non-redundant sequence database of genomes, transcripts and proteins. Nucleic Acids Res. 33, D501–D504.
Puchta M., Boczkowska M., Groszyk J. (2020). Low RIN value for RNA-seq library construction from long-term stored seeds: A case study of barley seeds. Genes 11, 1190. doi: 10.3390/genes11101190
Quick J., Loman N. J., Duraffour S., Simpson J. T., Severi E., Cowley L., et al. (2016). Real-time, portable genome sequencing for Ebola surveillance. Nature 530, 228–232. doi: 10.1038/nature16996
Rehm H. L., Bale S. J., Bayrak-Toydemir P., Berg J. S., Brown K. K., Deignan J. L., et al. (2013). ACMG clinical laboratory standards for next-generation sequencing. Genet. Med. 15, 733–747. doi: 10.1038/gim.2013.92
Rhoads A., Au K. F. (2015). PacBio sequencing and its applications. Genomics Proteomics Bioinf. 13, 278–289. doi: 10.1016/j.gpb.2015.08.002
Roossinck M. J., Martin D. P., Roumagnac P. (2015). Plant virus metagenomics: advances in virus discovery. Phytopathology 105, 716–727. doi: 10.1094/PHYTO-12-14-0356-RVW
Rose R., Constantinides B., Tapinos A., Robertson D. L., Prosperi M. (2016). Challenges in the analysis of viral metagenomes. Virus Evol. 2, vew022. doi: 10.1093/ve/vew022
Rothberg J. M., Hinz W., Rearick T. M., Schultz J., Mileski W., Davey M., et al. (2011). An integrated semiconductor device enabling non-optical genome sequencing. Nature 475, 348–352. doi: 10.1038/nature10242
Rothberg J. M., Leamon J. H. (2008). The development and impact of 454 sequencing. Nat. Biotechnol. 26, 1117–1124. doi: 10.1038/nbt1485
Rott M., Xiang Y., Boyes I., Belton M., Saeed H., Kesanakurti P., et al. (2017). Application of next generation sequencing for diagnostic testing of tree fruit viruses and viroids. Plant Dis. 101, 1489–1499. doi: 10.1094/PDIS-03-17-0306-RE
Roumagnac P., Mollov D. S., Daugrois J., Filloux D. (2018). “Viral metagenomics and sugarcane pathogens,” in Achieving sustainable cultivation of sugarcane. Volume 2: breeding, pests, and diseases. Ed. Rotte P. (Burleigh Dodds Science Publishing, Cambridge), 183–200.
Roy S., Coldren C., Karunamurthy A., Kip N. S., Klee E. W., Lincoln S. E., et al. (2018). Standards and guidelines for validating next-generation sequencing bioinformatics pipelines: a joint recommendation of the Association for Molecular Pathology and the College of American Pathologists. J. Mol. Diagnostics 20, 4–27. doi: 10.1016/j.jmoldx.2017.11.003
Sanderson N. D., Kapel N., Rodger G., Webster H., Lipworth S., Street T. L., et al. (2023). Comparison of R9. 4.1/Kit10 and R10/Kit12 Oxford Nanopore flowcells and chemistries in bacterial genome reconstruction. Microbial Genomics 9.
Sastry K. S., Zitter T. A., Sastry K. S., Zitter T. A. (2014). “Management of virus and viroid diseases of crops in the tropics,” in Plant virus and viroid diseases in the tropics: volume 2: epidemiology and management, 149–480. doi: 10.1007/978-94-007-7820-7
Schmieder R., Edwards R. (2011). Quality control and preprocessing of metagenomic datasets. Bioinformatics 27, 863–864. doi: 10.1093/bioinformatics/btr026
Schroeder A., Mueller O., Stocker S., Salowsky R., Leiber M., Gassmann M., et al. (2006). The RIN: an RNA integrity number for assigning integrity values to RNA measurements. BMC Mol. Biol. 7, 1–14. doi: 10.1186/1471-2199-7-3
Selitsky S. R., Marron D., Hollern D., Mose L. E., Hoadley K. A., Jones C., et al. (2020). Virus expression detection reveals RNA-sequencing contamination in TCGA. BMC Genomics 21, 1–11. doi: 10.1186/s12864-020-6483-6
Shi Z. X., Chen Z. C., Zhong J. Y., Hu K. H., Zheng Y. F., Chen Y., et al. (2023). High-throughput and high-accuracy single-cell RNA isoform analysis using PacBio circular consensus sequencing. Nat. Commun. 14, 2631. doi: 10.1038/s41467-023-38324-9
Schoch C. L., Seifert K. A., Huhndorf S., Robert V., Spouge J. L., Levesque C. A., et al. (2012). Nuclear ribosomal internal transcribed spacer (ITS) region as a universal DNA barcode marker for Fungi. Proceedings of the national academy of Sciences 109 (16), 6241–6246.
Sigurgeirsson B., Emanuelsson O., Lundeberg J. (2014). Analysis of stranded information using an automated procedure for strand specific RNA sequencing. BMC Genomics 15, 631. doi: 10.1186/1471-2164-15-631
Song B., Song Y., Fu Y., Kizito E. B., Kamenya S. N., Kabod P. N., et al. (2019). Draft genome sequence of Solanum aethiopicum provides insights into disease resistance, drought tolerance, and the evolution of the genome. Gigascience 8, giz115. doi: 10.1093/gigascience/giz115
Tavares L., Alves P. M., Ferreira R. B., Santos C. N. (2011). Comparison of different methods for DNA-free RNA isolation from SK-N-MC neuroblastoma. BMC Res. Notes 4, 1–5. doi: 10.1186/1756-0500-4-3
Thresh J. M. (2006). “Crop viruses and virus diseases: a global perspective,” in Virus diseases and crop biosecurity (Springer Netherlands, Dordrecht), 9–32.
Timian R. G. (1974). Barley stripe mosaic virus. Phytopathology 64, 342–345. doi: 10.1094/Phyto-64-342
Valouev A., Ichikawa J., Tonthat T., Stuart J., Ranade S., Peckham H., et al. (2008). A high-resolution, nucleosome position map of C. elegans reveals a lack of universal sequence-dictated positioning. Genome Res. 18, 1051–1063. doi: 10.1101/gr.076463.108
van der Valk T., Vezzi F., Ormestad M., Dalén L., Guschanski K. (2020). Index hopping on the Illumina HiseqX platform and its consequences for ancient DNA studies. Mol. Ecol. Resour. 20, 1171–1181. doi: 10.1111/1755-0998.13009
Velasco L., Padilla C. V. (2021). High-throughput sequencing of small RNAs for the sanitary certification of viruses in grapevine. Front. Plant Sci. 12, 682879. doi: 10.3389/fpls.2021.682879
Vennapusa A. R., Somayanda I. M., Doherty C. J., Jagadish S. K. (2020). A universal method for high-quality RNA extraction from plant tissues rich in starch, proteins and fiber. Sci. Rep. 10, 16887. doi: 10.1038/s41598-020-73958-5
Villamor D. E. V., Ho T., Al Rwahnih M., Martin R. R., Tzanetakis I. E. (2019). High throughput sequencing for plant virus detection and discovery. Phytopathology 109, 716–725. doi: 10.1094/PHYTO-07-18-0257-RVW
Visser M., Bester R., Burger J. T., Maree H. J. (2016). Next-generation sequencing for virus detection: covering all the bases. Virol. J. 13, 1–6. doi: 10.1186/s12985-016-0539-x
Wamaitha M. J., Nigam D., Maina S., Stomeo F., Wangai A., Njuguna J. N., et al. (2018). Metagenomic analysis of viruses associated with maize lethal necrosis in Kenya. Virol. J. 15, 1–19. doi: 10.1186/s12985-018-0999-2
Whattam M., Dinsdale A., Elliott C. E. (2021). Evolution of plant virus diagnostics used in Australian post entry quarantine. Plants 10, 1430. doi: 10.3390/plants10071430
Wilson G., Aruliah D. A., Brown C. T., Chue Hong N. P., Davis M., Guy R. T., et al. (2014). Best practices for scientific computing. PloS Biol. 12, e1001745. doi: 10.1371/journal.pbio.1001745
Winter L. (2021). Scientists Reverse Engineer mRNA Sequence of Moderna Vaccine [News & Opinion] (The Scientist). Available at: https://www.the-scientist.com/news-opinion/scientists-reverse-engineer-mrna-sequence-of-moderna-vaccine-68640.
Wongsurawat T., Jenjaroenpun P., Taylor M. K., Lee J., Tolardo A. L., Parvathareddy J., et al. (2019). Rapid sequencing of multiple RNA viruses in their native form. Front. Microbiol. 10, 260. doi: 10.3389/fmicb.2019.00260
Wren J. D., Roossinck M. J., Nelson R. S., Scheets K., Palmer M. W., Melcher U. (2006). Plant virus biodiversity and ecology. PloS Biol. 4, e80. doi: 10.1371/journal.pbio.0040080
Wu Q., Ding S. W., Zhang Y., Zhu S. (2015). Identification of viruses and viroids by next-generation sequencing and homology-dependent and homology-independent algorithms. Annu. Rev. Phytopathol. 53, 425–444. doi: 10.1146/annurev-phyto-080614-120030
Wylie S. J., Jones M. G. K. (2011). Characterization and quantitation of mutant and wild-type genomes of Hardenbergia mosaic virus isolates coinfecting a wild plant of Hardenbergia comptoniana. Arch. Virol. 156, 1251–1255. doi: 10.1007/s00705-011-0965-4
Xin M., Cao M., Liu W., Ren Y., Zhou X., Wang X. (2017). Two negative-strand RNA viruses identified in watermelon represent a novel clade in the order Bunyavirales. Front. Microbiol. 8, 1514. doi: 10.3389/fmicb.2017.01514
Yamashita A., Sekizuka T., Kuroda M. (2016). VirusTAP: viral genome-targeted assembly pipeline. Front. Microbiol. 7, 32. doi: 10.3389/fmicb.2016.00032
Yates A. D., Adams J., Chaturvedi S., Davies R. M., Laird M., Leinonen R., et al. (2022). Refget: Standardized access to reference sequences. Bioinformatics 38, 299–300. doi: 10.1093/bioinformatics/btab524
Yee L. L., Tapani T. M. (2017). Bioinformatics: A practical handbook of next generation sequencing and its applications (World Scientific Pub. Co. Inc), 1–252.
You M. P., Akhatar J., Mittal M., Barbetti M. J., Maina S., Banga S. S. (2021). Comparative analysis of draft genome assemblies developed from whole genome sequences of two Hyaloperonospora brassicae isolate samples differing in field virulence on Brassica napus. Biotechnol. Rep. 31, e00653. doi: 10.1016/j.btre.2021.e00653
Zavala E. I., Aximu-Petri A., Richter J., Nickel B., Vernot B., Meyer M. (2022). Quantifying and reducing cross-contamination in single-and multiplex hybridization capture of ancient DNA. Mol. Ecol. Resour. doi: 10.1111/1755-0998.13607
Zepeda B., Verdonk J. C. (2022). RNA extraction from plant tissue with homemade acid guanidinium thiocyanate phenol chloroform (AGPC). Curr. Protoc. 2, e351. doi: 10.1002/cpz1.351
Zerbino D. R. (2010). Using the velvet de novo assembler for short-read sequencing technologies. Curr. Protoc. Bioinf. 31 (1), 11–15.
Zerbino D. R., Birney E. (2008). Velvet: algorithms for de novo short read assembly using de Bruijn graphs. Genome Res. 18 (5), 821–829.
Zhang Y., Singh K., Kaur R., Qiu W. (2011). Association of a novel DNA virus with the grapevine vein-clearing and vine decline syndrome. Phytopathology 101, 1081–1090. doi: 10.1094/PHYTO-02-11-0034
Zhang S., Tian X., Navarro B., Di Serio F., Cao M. (2021). Watermelon crinkle leaf-associated virus 1 and watermelon crinkle leaf-associated virus 2 have a bipartite genome with molecular signatures typical of the members of the genus Coguvirus (family Phenuiviridae). Arch. Virol. 166, 2829–2834. doi: 10.1007/s00705-021-05181-0
Zheng L., Rodoni B. C., Gibbs M. J., Gibbs A. J. (2010). A novel pair of universal primers for the detection of potyviruses. Plant Pathol. 59, 211–220. doi: 10.1111/j.1365-3059.2009.02201.x
Keywords: high-throughput sequencing, plant virus, phytosanitation, diagnostic, genome
Citation: Maina S, Donovan NJ, Plett K, Bogema D and Rodoni BC (2024) High-throughput sequencing for plant virology diagnostics and its potential in plant health certification. Front. Hortic. 3:1388028. doi: 10.3389/fhort.2024.1388028
Received: 19 February 2024; Accepted: 10 April 2024;
Published: 23 May 2024.
Edited by:
Francis Onono Wamonje, National Institute of Agricultural Botany (NIAB), United KingdomReviewed by:
Leonardo Velasco, Andalusian Institute for Research and Training in Agriculture, Fisheries, Food and Ecological Production (IFAPA), SpainRakesh Kumar Verma, Mody University of Science and Technology, India
Copyright © 2024 Maina, Donovan, Plett, Bogema and Rodoni. This is an open-access article distributed under the terms of the Creative Commons Attribution License (CC BY). The use, distribution or reproduction in other forums is permitted, provided the original author(s) and the copyright owner(s) are credited and that the original publication in this journal is cited, in accordance with accepted academic practice. No use, distribution or reproduction is permitted which does not comply with these terms.
*Correspondence: Solomon Maina, c29sb21vbi5tYWluYUBkcGkubnN3Lmdvdi5hdQ==