- 1Department of Agricultural Production, College of Agriculture and Environmental Sciences, Makerere University, Kampala, Uganda
- 2Makerere University Regional Center for Crop Improvement, Makerere University, Kampala, Uganda
- 3Savanna Agricultural Research Institute, Council for Scientific and Industrial Research, Tamale, Ghana
- 4National Crops Resources Research Institute, Kampala, Uganda
- 5International Institute of Tropical Agriculture, Oyo, Nigeria
- 6Department of Plant Science, Microbiology and Biotechnology, School of Bioscience, College of Natural Science, Makerere University, Kampala, Uganda
Introduction: Yam is an important crop for food security in East and West Africa due to its high market value and customer demand. High tuber quality with yield and disease resistance are the main traits for acceptability of yam cultivars across the tropical zone. There has been limited progress in enhancing the production and quality traits of yams, despite the significant socio-economic significance of this crop.
Method: To expedite the development of high-quality yam cultivars in Uganda, traits association study was conducted to identify genomic regions associated with key traits such as disease resistance, high yields, and dry matter content. The association mapping was conducted with multi-random mixed linear model (mrMLM) to compute the associations using five genetic models.
Results: A total of 16 significant single nucleotide polymorphisms (SNPs) markers were identified to be associated with the traits studied. Gene identification analysis revealed the presence of key putative genes such as Vicilin-like seed storage protein At2g28490 (ARATH)and Growth-regulating factor 1 involved in a variety of functions ranging from storage and gene regulation for disease resistance.
Discussion: The results obtained from this work have significant implications for the in-depth analysis of the genetic structure underlying key traits in yam. Additionally, this study emphasizes the identification of SNP variants and genes that may be utilized for genomic-informed selection in order to enhance yield and disease resistance in yams.
1 Introduction
Yam belongs to the Dioscorea species and plays a crucial role in ensuring food security in East and West Africa (Kilimo Trust, 2013). It is well-suited for market-driven production intensification because of its high market value and strong consumer demand (Haneishi et al., 2013). Dioscorea rotundata, often known as white yam or Guinea yam is a vital tuber crop with significant economic and dietary importance in several regions worldwide. The crop often known as the “old man” crop is cultivated by older individuals in Uganda (Adjei et al., 2022a). It is sometimes confused with the Colocasia species due to their similar tuber-producing nature (Kagoda et al., 2005). Over the past two decades, there has been limited progress in enhancing the production and quality of yams compared to other roots and tuber crops. This is particularly evident in East Africa, despite the significant socio-economic importance of yams. Nevertheless, in Uganda, yam breeding endeavors have not adequately explored the genetic foundation of valuable characteristics such as tuber quality and yield. This has hindered the rapid development of improved cultivars (Adjei et al., 2022a).. The improvement of several characteristics of yam such as resistance to pests and diseases, tuber production and quality is a challenging task due to their quantitative inheritance (Mignouna et al., 2001; Nemorin et al., 2012). While conventional breeding methods (Mass selection, phenotypic classification and hybridization) have been successful in improving many crop traits, there are certain traits that are not easily manipulated using these techniques. These traits often involve complex genetic traits influenced by multiple genes and environmental interactions (Acquaah, 2012).
The behavior of yams specifically in relation to their dry matter content (DMC), yield (TWY), and susceptibility to yam mosaic virus (YMV) has captured the attention of scholars, farmers, and breeders alike. To achieve sustainable yam production, provide food security and effectively manage diseases, it is critical to have a thorough understanding of these traits. The agricultural relevance and socio-economic effect of D. rotundata are greatly influenced by its dry matter content, yield, and sensitivity to yam mosaic virus (Mignouna et al., 2001). Continued research and innovative approaches in yam production are crucial for fully utilizing the potential of this important commodity. This is especially important as global concerns in food security and sustainable agriculture persist and evolve over time (Adeniji et al., 2012).
Dry matter content is a determinant of yam quality representing the remaining portion of the yam tuber after the removal of water content. The trait has a direct influence on the taste, consistency and nutritional composition of the yam. Due to its indication of a greater abundance of essential nutrients such as carbohydrates, a larger dry matter content is sometimes favored (Gatarira et al., 2020). To improve tuber quality and increase consumer satisfaction, researchers have been investigating the traits that influence dry matter content in Dioscorea rotundata. These traits include environmental conditions, cultivation methods, and genetic factors (Adjei et al., 2022b).. Yield, a significant trait of Dioscorea rotundata which constitutes the quantity of tubers generated within a given area (Wu et al., 2015). Optimizing yam output is important for meeting the food needs of growing populations and ensuring the economic prosperity of farmers (Adeniji et al., 2012). Yam productivity is influenced by crucial elements such as soil quality, planting density, pest and disease control, and proper watering. Scientists and agricultural experts are still exploring efficient methods to enhance yam production while maintaining environmental sustainability.
The cultivation of Dioscorea rotundata is significantly threatened by Yam mosaic virus (Mignouna et al., 2001). It is a viral disease that results in the formation of unique mosaic patterns on the leaves of yam plants. This leads to a decrease in the plant’s ability to carry out photosynthesis, resulting in stunted growth and reduced output of yam tubers (Sorho Fatogoma Brahima Kone YKA& GOEDJB and Ettien Djecthi Jean Baptiste SFBKYKA& GO, 2014). Effectively managing YMV requires implementing a range of preventative strategies. These include using virus-free planting materials, adhering to proper sanitation practices, and employing cultivars that are resistant to the virus (Sorho Fatogoma Brahima Kone YKA& GOEDJB and Ettien Djecthi Jean Baptiste SFBKYKA& GO, 2014). Gaining insight into the interplay between Dioscorea rotundata and YMV is essential for devising efficient approaches to alleviate the adverse effects of this disease on yam cultivation (Adjei et al., 2022b).
The use of advanced breeding methods, such as genomic selection into yam improvement initiatives is anticipated to address complex challenges by enhancing the pace and effectiveness of breeding (Sugihara et al., 2020). The development of next-generation sequencing (NGS) technology has allowed for the discovery and utilization of single nucleotide polymorphism (SNP) markers that are associated with certain traits. These markers may be used to aid in breeding programs for important crops (Bhattacharjee et al., 2013). A genome sequence assembly for Dioscorea rotundata has been recently published (Siadjeu et al., 2020). This development enables the utilization of high-density markers for Genome-Wide Association Studies (GWAS) to identify genomic regions associated with specific traits (Tamiru et al., 2017). Additionally, it allows for the accurate placement of SNPs at these specific locations. GWAS has been extensively employed to identify the intricate genes responsible for economic traits in crops. These qualities encompass disease resistance and quality improvement which are crucial for efficient breeding and genetic analysis (Cuevas et al., 2018; Ahn et al., 2019).
SNP Markers have been utilized in yams for a range of research, encompassing genetic analysis of tuber yield and yam mosaic tolerance (Agre et al., 2021), analysis of sex determination and cross-compatibility (Mondo et al., 2021), determination of paternity in yams (Norman et al., 2020), development of molecular markers (Girma et al., 2019), examination of yam hybrid origin at the genome level (Sugihara et al., 2020), and exploration of yam diversity (Loko et al., 2016; Agre et al., 2019; Bhattacharjee et al., 2020; Sugihara et al., 2020; Amponsah Adjei et al., 2023). The identification of favorable SNP alleles through QTL discovery is relevant for enhancing key traits via marker-assisted selection (MAS). Incorporating genomic-assisted breeding tools into yam breeding programs is anticipated to expedite genetic improvements for prioritized traits. This objective can be realized by elucidating the genetic mechanisms governing these traits, thereby enabling the systematic application of MAS for forward breeding.
Thus, this study aimed at identifying genomic regions associated with yield, YMV and dry matter content in Dioscorea rotundata species. The yam breeding program will benefit from the identified genomic areas in the marker-assisted selection process for the important traits under investigation. Furthermore, this study will establish a basis for genetic improvement and variety breeding for yams in Uganda by offering information for the investigation of high yielding, disease resistance-related, and high dry matter genes.
2 Materials and methods
2.1 Genetic materials
A total of 207 D. rotundata genotypes were used in this study. These populations consisted of breeding lines used in routine activities in Ghana, Nigeria, and landraces from Uganda. The genetic materials were part of the collection held at the National Crop Resource Research Institute (NaCRRI), which is an entity of the National Agricultural Research Organization (NARO) in Uganda (Supplementary Table 1).
2.2 Trials establishment and leaf sampling
The study was carried out in NaCRRI, Namulonge, Uganda, over two consecutive cropping seasons in 2020 and 2021. The location is situated at a latitude of 0°5` N and a longitude of 32°61` E. It has an elevation of 1,120 meters above sea level (masl) and experiences an annual rainfall of 1,170 mm (Nsubuga et al., 2011). The experiment was conducted using an augmented design, where each block (8 blocks) had 26 genotypes, three local checks, and three plants per genotype. The Genotypes were planted on mounds with inter-row and intra-row spacings of 1.2 m x 1.2 m, respectively. For each genotype, three pre-sprouted sets weighing between 400 and 500 g on average were planted in each mound. The plots were tagged for data collection. Yam leaf samples were gathered using the designated plant sample collection kit (KBS-9370-001) procedure. Using the BioArk Leaf sampling technique. Leaf samples were obtained from labeled plants and placed into 96-well tube plates 16 weeks following planting, utilizing a leaf puncher. Subsequently, the leaves were subjected to oven-drying at a temperature of 80°C.
2.3 Trait measurements
Data were collected for disease-related traits (Yam mosaic virus severity), yield-related traits (Yam tuber yield expressed as kg/plot) and dry matter content (%) (Table 1). All measurements were taken based on the standard operating protocol for the yam varietal performance evaluation trial (Asfaw, 2016) and the trait ontology dictionary described in YamBase (https://yambase.org/(accessed on: 18/12/2020) (Table 1).
2.3.1 Dry matter content
Dry matter content was determined seven days after harvesting by oven-dry method using a single tuber. Each tuber was sliced into chips, the fresh weight (wet sample) was taken and then oven dried. The oven for the experiment was preheated to a temperature of 80 ° C for 2 hours. After, the envelopes containing the sliced tubers were placed in the preheated oven for drying at 80 ° C for 48 hours. The dried sliced tubers were then weighed to determine the dry weight used as the dry matter content and expressed as a percentage (Equation 1).
2.3.2 Yam mosaic virus estimates and area under disease progress calculation
The virus severity scores (Table 1) were averaged from three plant stands per plot. These averages were then used to calculate the area under the disease progress curve (AUDPC) values (Equation 2), following the method described by Forbes et al (Forbes et al., 2014)
The virus symptioms description in leaf included; 1 = No visible symptoms (virus negative), 2 = Mosaic on most leaves (symptoms recovery with time), 3 = Mild symptoms on few leaves (No leaf distortion), 4 = Severe mosaic on most leaves (leaf distortion) and 5 = Severe mosaic (bleaching/severe leaf distortion and stunting).
Where;
● yi = disease severity at the ith observation
● ti = time (days) at the ith observation
● n = total number of observations
2.4 Genotyping and quality assessment
The dried leaf samples of each yam genotype were sent to SEQART AFRICA at the International Livestock Research Institute (ILRI) in Nairobi for genotyping. The process of DNA extraction was performed utilizing the Nucleomag Plant DNA extraction kit, specifically the Mag-Bind® Plant DNA DS 96 Kit. The isolated genomic DNA had a concentration ranging from 50 to 100 ng/μl. The quality and amount of DNA were assessed using 0.8% agarose gel. The libraries were generated utilizing the DArTSeq complexity reduction methodology (Kilian et al., 2016) by the digestion of genomic DNA using PstI and MseI enzymes. Subsequently, the barcoded adapters and common adapters were joined together, and then the resulting fragments were amplified by PCR. The libraries were subsequently subjected to single-read sequencing runs, with each run generating sequences of seventy-seven bases.
The Hiseq2500 platform was utilized to do next-generation sequencing (Kilian et al., 2016). The SEQART AFRICA platform utilizes genotyping by sequencing (GBS) DArTseqTM technology, which allows for rapid, high-quality, and cost-efficient genome profiling of complex polyploid genomes. The scoring of DArTseq markers was accomplished using DArTsoft14, an internal pipeline for marker scoring developed by Kilian et al (Kilian et al., 2016). The SilicoDArT markers and SNP markers were assessed using a binary scoring system. A value of 1 was assigned if the restriction fragment containing the marker sequence was present in the genomic representation of the sample, and a value of 0 was assigned if it was absent.
The SilicoDArT markers and SNP markers were mapped to the D. rotundata reference genome (TDr96_F1_v2_PseudoChromosome.rev07) in order to determine their placements on the chromosomes. The process of ensuring data quality and removing unwanted data was carried out using TASSEL (v5.2.52) (Bradbury et al., 2007). SNP markers exhibiting more than 20% missing data, a minor allele frequency (MAF) below 0.05, and places on the genome that are unknown were excluded. The SNP data underwent further imputation using the LD k-nearest neighbor genotype imputation (LD KNNI) approach (Troyanskaya et al., 2001), as described by Bradbury et al (Bradbury et al., 2007). This approach was selected because of Its ability to impute missing values by finding the closest neighbors in multidimensional space ensuring robustness considering the datasets used. Moreover, KNNI does not rely on assumptions about the underlying linkage disequilibrium (LD) structure, making it a flexible choice when the LD patterns are complex. In the end, a grand total of 4,957 Single Nucleotide Polymorphisms (SNPs) were chosen for subsequent examination.
2.5 Statistical analysis
2.5.1 Phenotypic data analysis
The phenotypic data collected from the two cropping seasons were analyzed using the “augmentedRCBD” function from the R package “agricolae” for the DAU test function (De Mendiburu, 2015). In the model, checks were treated as fixed while block and treatments were considered random. The adjusted means for the various genotypes in the two cropping seasons were derived through mixed model analysis (Equation 3) and were subsequently utilized in GWAS analysis (R Core Team, 2022).
Where;
● Y: The phenotypic trait value.
● β₀: The intercept of the model.
● βᵢ: The effect size (regression coefficient) of the ith SNP genotype (typically coded as 0, 1, or 2 for the number of minor alleles).
● Xi: The genotype score for the ith SNP.
● γj: The effect size of the jth covariate.
● Cj: The value of the jth covariate for each individual (polulation structure).
● ϵ: The error term, representing all other unmeasured factors influencing the trait.
Σ represents summation across all SNPs (i) and covariates (j) included in the model.
In this case, we have as well the kinship matrix which is implemented in the mrMLM
2.5.2 GWAS Analysis and gene identification
A mixed linear model implemented in the multi-random mixed linear model (mrMLM) was used to compute the associations using five genetic models (Zhang et al., 2017). These models included: multi-locus random-SNP-effect Mixed Linear Model (Wang et al., 2016), fast multi-locus random-SNP-effect EMMA (FASTmrEMMA) (Zhang et al., 2020), polygenic-background-control- based least angle regression plus empirical Bayes (pLARmEB) (Zhang et al., 2017), fast mrMLM (FASTmrMLM) (Tamba and Zhang, 2018) and pKWmEB (Wen et al., 2018).
The observed logarithms (-log10) of the p-values were plotted against the expected p-values to assess the adequacy of the GWAS model to examine how effectively the models compensated for population structure. The Manhattan plot was created for visualizing GWAS on the entire genome and zoom mapping was performed on a particular chromosome after identifying a significant SNP marker.
For gene identification, we made use of the generic feature format (GFF3) for searching for genes in the nearest associated marker (Hunter et al., 2012). Public database Interpro, European Molecular Biology Laboratory-European Bioinformatics Institute (EMBL-EBI) was used to determine the functions of the genes associated with the different SNPs identified (Shin et al., 2006).
2.6 Results
2.6.1 Genotypic variability for studied traits
The variance estimate for the traits under study was significant. For season one, the DMC varied from 15.9% to 45.5% with a mean of 30.9%, and for season two, it varied from 17.1% to 38% with a mean of 28.9% (Table 2; Figures 1, 2). When compared to the mean of 5.5 (kg/ha) for season one, the mean yield was highest in season two (13.1 kg/plot). Additionally, season one had the lowest YMV mean incidence (175), ranging from 121.9 to 393.3, whereas season two had a mean of 217 and a range of 102 to 332.4 for YMV incidence. Heritability was highest for YMV in season one followed by DMC for the same season. The lowest was identified for tuber yield for both seasons respectively (Table 2).
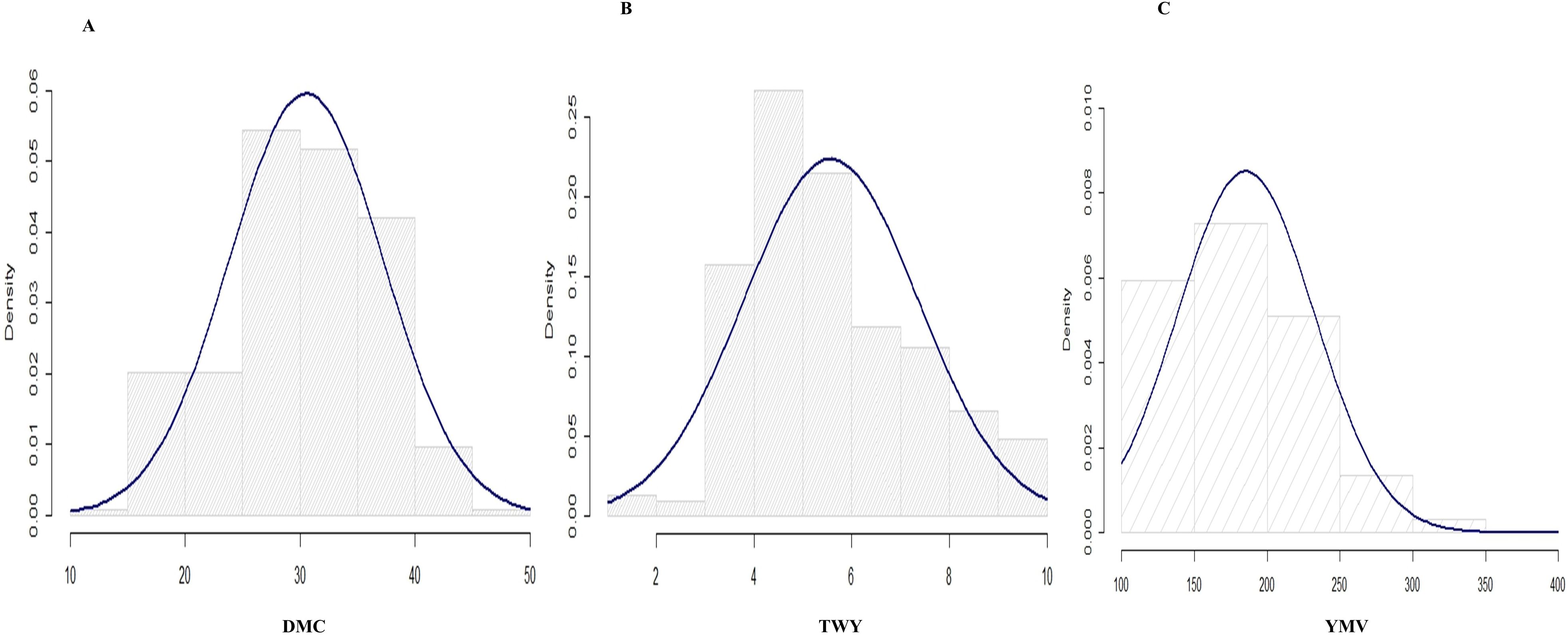
Figure 1. Histogram for the distributions for the studied traits in 2020 cropping season (A) tuber dry matter content, (B) tuber yield and (C) yam mosaic virus.
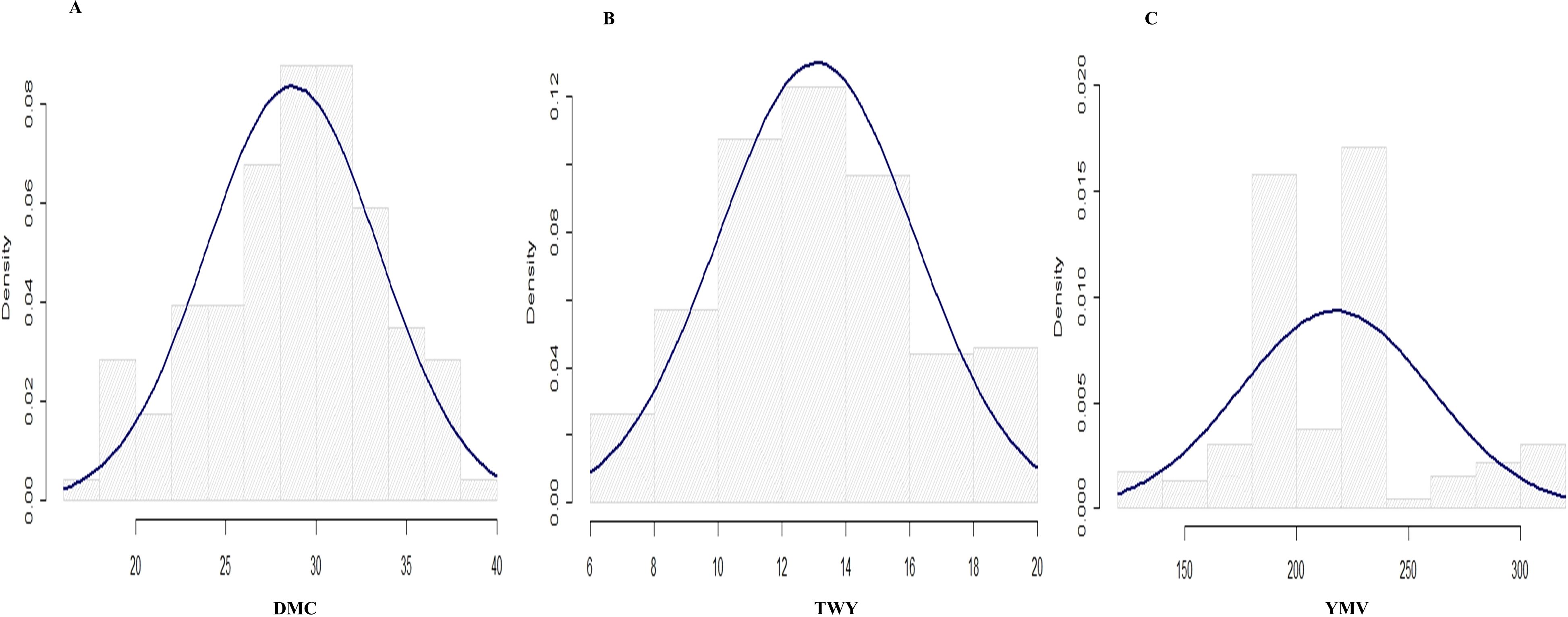
Figure 2. Histogram for the distributions for the traits studied in 2021 cropping season (A) tuber dry matter content, (B) tuber yield and (C) yam mosaic virus.
2.6.2 Marker coverage, population structure and linkage disequilibrium
Table 3 provides the comprehensive count of single nucleotide polymorphisms (SNPs) detected on the chromosomes of Dioscorea rotundata, taking into consideration the absence of data, allele frequency, and heterozygosity. A total of 4,957 single nucleotide polymorphisms (SNPs) were retained and unequally distributed across the 20 chromosomes. Chromosome 5 had the highest number of SNPs, with 524 SNPs accounting for 10.6% of the total while chromosome 13 had the lowest SNPs, with 91 SNPs, making up 1.8% of the total. When comparing the observed and predicted heterozygosity, the observed heterozygosity varied between 0.097 and 0.153, with an average of 0.115. On the other hand, the expected heterozygosity ranged from 0.256 to 0.314, with an average of 0.280 (Table 3).
A principal component analysis (PCA) was conducted utilizing the pairwise Euclidean genetic distance matrix of the genotypes to illustrate the genetic divergence in the yam genotypes. The PCA findings demonstrated that the first two axes accounted for 99.7% of the overall genetic variance (Figure 3A). The first axis (Dim 1) accounted for 94.9% of the genetic variance, while the second axis (Dim 2) accounted for 4.8% of the overall genetic variation. The genotypes, as determined by SNP markers and principal component analysis, exhibited limited diversity with their geographical origins. The graph displayed three prominent clusters, revealing that the genotypes obtained from Nigeria and Ghana formed a cohesive group, whereas the genotypes from Uganda were dispersed among the genotypes from Nigeria and Ghana in various quadrants (Figure 3A).
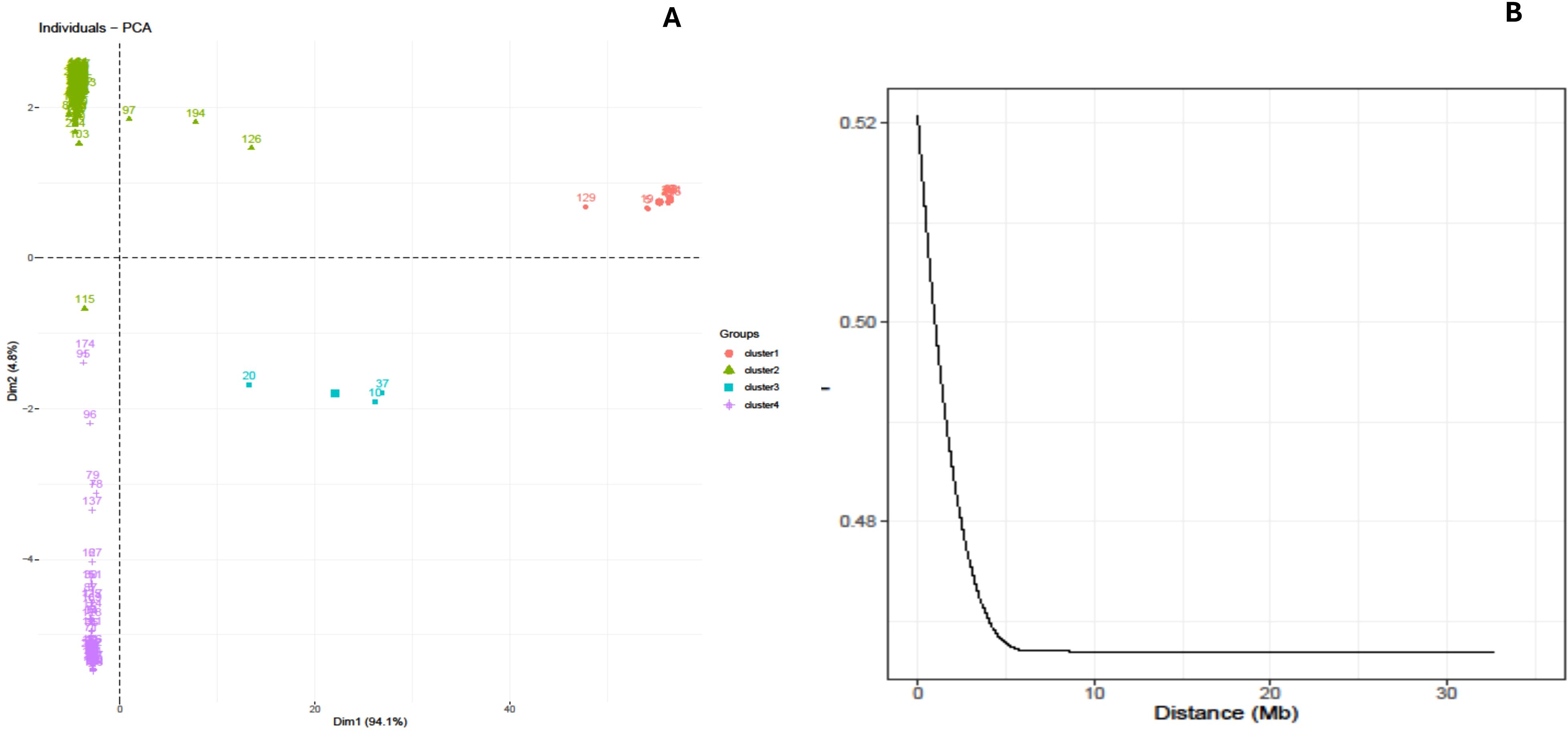
Figure 3. (A) PCA-biplot based clustering displaying the relationship between and among the genotypes. (B) Linkage disequilibrium (LD) of yam genome used for the study.
The LD plot indicated a significant association between linkage disequilibrium (R2) and physical distance (bp) (r = −0.035), as well as between p-value and R2 (r = −0.40), suggesting the presence of linkage decay. The decrease of linkage disequilibrium (LD) varied among the chromosomes, with a range of 8,289 base pairs for chromosome 1 to 58,562 base pairs for chromosome 20 (Figure 3B).
2.7 Genome-wide association scans for studied traits
2.7.1 Dry matter content
Based on the methods used for the study, we identified two significant SNP markers associated with the dry matter content, with all the SNP markers located on Chromosome 18 (Table 4; Figures 4, 5). The SNP loci exhibited marker effect ranging between 1.74 and 3.49 and together accounted for an average of 25.9% of the overall phenotypic variance with an average minor allele frequency of 0.212 for the two seasons. Additional analysis of the two SNP loci linked to the tuber DMC on chromosome 18 revealed three linkage alleles (AA, AT and TT). The association between loci AT and AA for marker was observed significant at p<0.45 compared to linkage between the AA and TT which was at p< 0.054. These alleles were observed in clones that possess the homozygous allele TT and AA exhibited high DMC compared to those with the heterozygous allele AT.
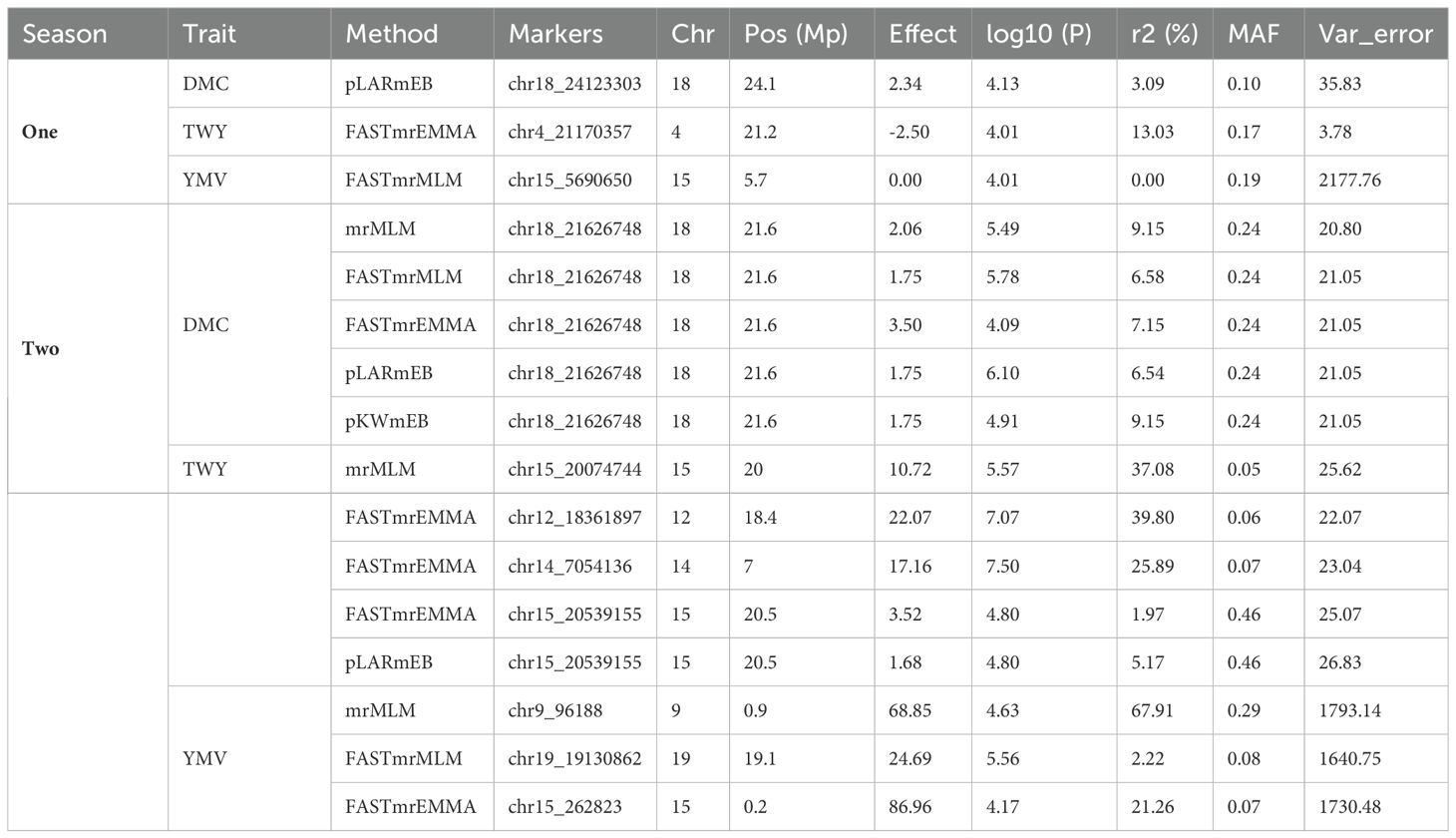
Table 4. Summary of significant single nucleotide polymorphism describing different genomic regions associated with studied traits for two cropping seasons in a panel of 207 Dioscorea rotundata genotypes.
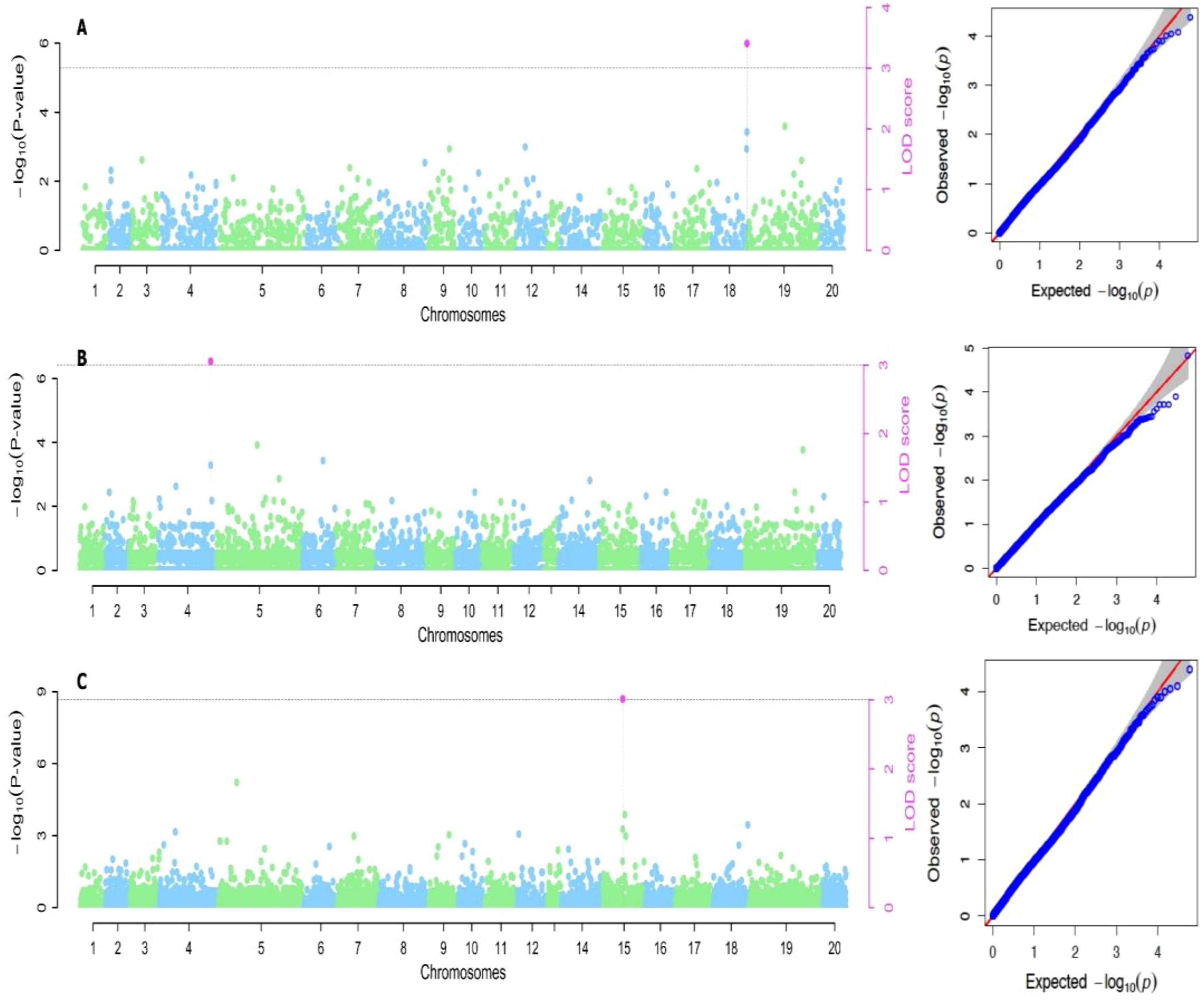
Figure 4. Manhattan plots for genome-wide diagnosis of association signals for studied traits of cropping season one [(A) Dry matter content, (B) Total weight of yam and (C) Yam mosaic virus]. [The y-axis represents the p-value of the marker-trait association on a -log10 scale and the x-axis relates to the 20 yam chromosomes, green dots above the blue dotted line and red dot above red line indicate SNPs associated with QTL with influence on yam mosaic virus disease. Horizontal blue line is 5% Bonferroni threshold line].
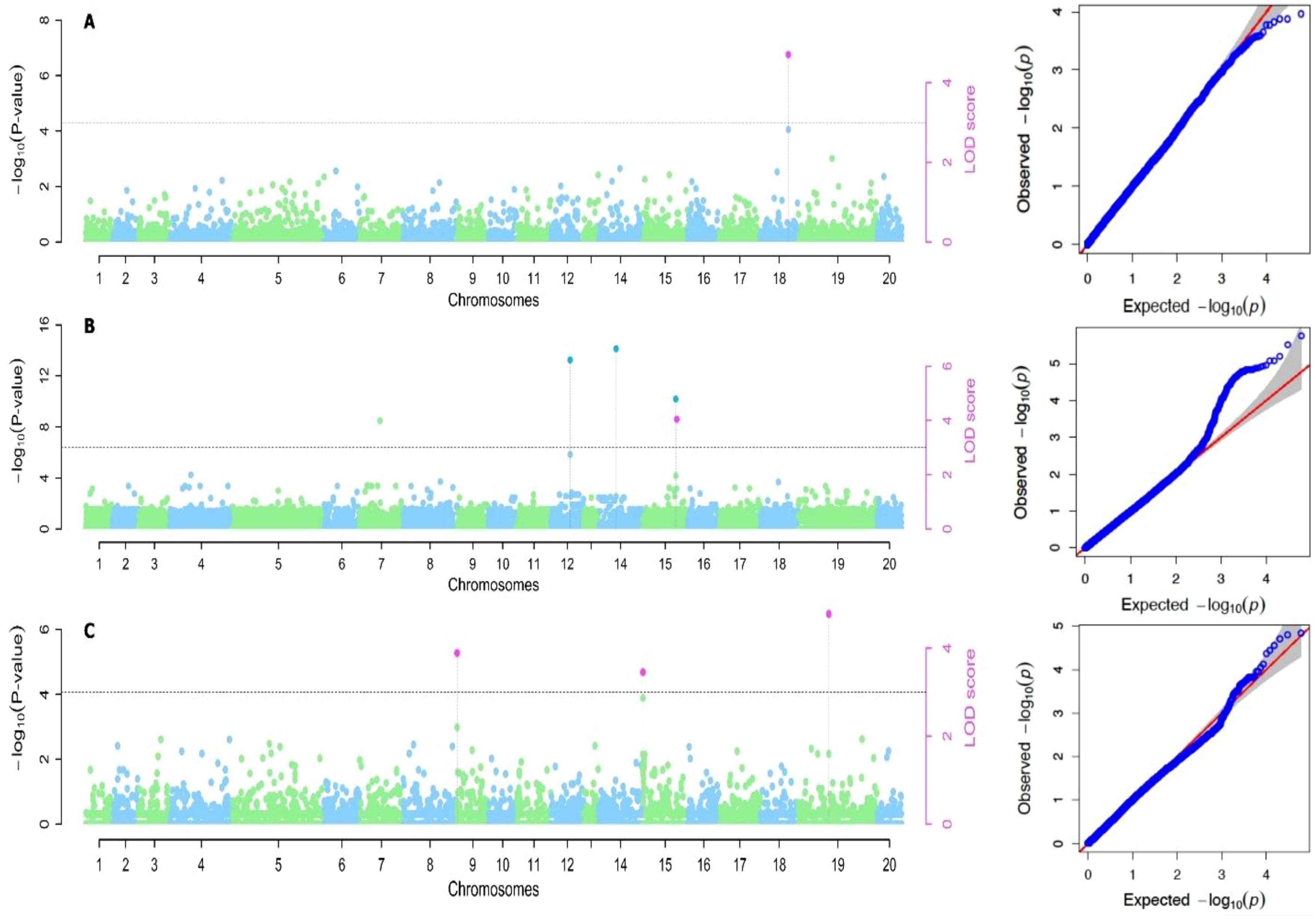
Figure 5. Manhattan plots for genome-wide diagnosis of association signals for studied traits of cropping season two [(A) Dry matter content, (B) Total weight of yam and (C) Yam mosaic virus]. [The y-axis represents the p-value of the marker-trait association on a -log10 scale and the x-axis relates to the 20 yam chromosomes, green dots above the blue dotted line and red dot above red line indicate SNPs associated with QTL with influence on yam mosaic virus disease. Horizontal blue line is 5% Bonferroni threshold line].
2.7.2 Total weight of yam
Six significant single nucleotide polymorphism (SNP) indicators related to yam tuber yield were discovered on four chromosomes (4, 12, 14 and 15). The following Markers “chr4_21170357”, “chr12_18361897”, “chr14_7054136” and “chr15_20539155” located on chromosome 4, 12 14 and 15 showed a significant association using the FASTmrEMMA method (Table 4). The MAF ranged between 0.05 and 0.45, and they were detected with marker effect ranging between -2.50 and 22.06. These markers explained approximately 32.2% of the total phenotypic variation. For TWY, a total of 5 alleles (CC, CT, TT, AA and AT) were identified and alleles CC and TT are associated with high tuber yield (TWY) in the population. Conversely, the alleles AA, CT, and AT were found to be associated with low TWY.
2.7.3 Yam mosaic virus
A total of four significant SNPs were found to be linked with YMV. Among these, two SNP loci were located on chromosome 15, and one was located on chromosome 19 at a physical position of 19,130,862 Bp. With FASTmrEMMA method, we identified three SNPs (chr15_5690650, chr15_262823 and chr19_19130862). Additionally, through the mrMLM method, we observed one SNP (chr9_96188) (Figures 4, 5). The SNP loci exhibited marker effect ranging from 24.68 to 86.96, and together accounted for an average of 21.12% (Table 4). The Quantile-Quantile (QQ) plot confirmed a decrease in the -log10 (p-value) toward the predicted level for YMV. In the case of YMV, the presence of the homozygous alleles CC and AA resulted in lower resistance compared to the heterozygous allele AT.
2.8 Gene identification
2.8.1 Dry matter content
Putative genes associated with dry matter content were mainly identified on chromosomes 18 (Table 5). Through the annotation process, two candidate genes linked to DMC were identified. The genes mentioned include Transcription initiation factor TFIID subunit 12b, and Growth-regulating factor 1. The gene Transcription initiation factor TFIID subunit 12b was found at position 24Mb on chromosome 18. The growth-regulating factor 1 gene was found at positions 21.6 Mbs on chromosome 18 for five methods used for the study (Table 5).
2.8.2 Total weight of yams
We identified putative genes associated with total tuber yield of which were identified on 6 chromosomes (Table 5). Among the 6 chromosomes, 5 potential candidate genes were found near the peak SNPs. These genes include Phoenix dactylifera coatomer subunit delta-2-like observed on chromosome 4 for positions 21.2 Mbs, COMPASS-like H3K4 histone methylase component WDR5AARATH (Chromosome 15; position 20.0 Mbs), Vicilin-like seed storage protein At2g28490ARATH (Chromosome 12; position 18.4 Mbs), Ananas comosus IAA-amino acid hydrolase ILR1-like 3 (Chromosome 14; position 7.1 Mbs) and Late exocytosis, associated with Golgi transport (Chromosome 15; position 20.5 Mbs), (Table 5).
2.8.3 Yam mosaic virus
However, five potential genes were identified for YMV, specifically the Glucan endo-1,3-beta-glucosidase 8 which is observed on chromosome 15 at 5.7 b. The second association was identified as chromosome 9 at 9Mb for nuclear pores complex protein NUP62 gene. The two genes (Cold-responsive protein kinase 1 and Probable LRR receptor-like serine/threonine-protein) were linked to chromosome 19 at 19.1Mb. The last gene observed to be associated with YMV was G-type lectin S-receptor-like serine/threonine-protein for chromosome 15 at 0.2 Mb. The heatmaps display the linkage disequilibrium (LD) of each detected SNP locus. Regarding DMC, the LD analysis of the loci (traits under study) indicated that these markers exhibited a moderately to highly significant LD parameter (R2 > 0.6), indicating a rather strong connection (Supplementary Figure 1). The LD analysis conducted on two loci, TWY and YMV, revealed that the markers on chromosome 5 exhibited very low LD characteristics (R2 < 0.7) (Supplementary Figure 1).
3 Discussion
The study revealed an extensive genome-wide association analysis to pinpoint quantitative trait nucleotides associated with key agronomic traits in yam, such as Yam Mosaic Virus resistance, yield, and dry matter content. The analysis uncovered significant and informative variances among these traits. Employing multi-random mixed linear model approaches in mrMLM, five different gene action models were utilized. Across two seasons, a total of 16 SNP markers were identified. Similar chromosomal regions linked to yield and dry matter content were reported in previous studies (Agre et al., 2021).
This study identified four gene/protein families linked to yam mosaic virus disease: Glucan endo-1,3-beta-glucosidase 8 (Henrissat and Davies, 2000; Mouyna et al., 2000; Barral et al., 2004), Nuclear pore complex protein NUP62 (Bailer et al., 2001), Cold-responsive protein kinase 1; Probable LRR receptor-like (Manning et al., 2002; Li et al., 2004; Stout et al., 2004) and G-type lectin S-receptor-like serine/threonine-protein (Hanks and Quinn, 1991). These genes are located on chromosomes 9, 15 and 19, respectively.
According to Barral (Barral et al., 2004), the X8 domain contains at least 6 conserved cysteine residues that presumably form three disulphide bridges. The domain is found in an Olive pollen allergen as well as at the C-terminus of several families of glycosyl hydrolases and observed to be involved in carbohydrate binding. It is characteristic of GPI-anchored domains. Moreover, some of the identified genes such as protein phosphorylation play a key role in most cellular activities. Additionally they serve as a reversible process mediated by protein kinases and phosphoprotein phosphatases (Hanks and Quinn, 1991). Protein kinases catalyse the transfer of the gamma phosphate from nucleotide triphosphates (often ATP) to one or more amino acid residues in a protein substrate side chain, resulting in a conformational change affecting protein function (Hanks and Quinn, 1991). In contrast to the findings of Agre et al (Agre et al., 2021), they identified single nucleotide polymorphisms (SNPs) on chromosome 3 that are linked to yam mosaic virus. These SNPs are located close proximity to genes encoding the AP2/ERF domain, AUX/IAA protein, major facilitator, and sugar transporter-like proteins. The findings of these studies might expedite the use of SNP variations to assist selection decisions in breeding white Guinea yam for genotype selection with resistance to mosaic virus in Uganda.
For the yam tuber yield we identified several important genes related to total tuber weight, including Phoenix dactylifera coatomer subunit delta-2-like, COMPASS-like H3K4 histone methylase component WDR5AARATH, Vicilin-like seed storage protein At2g28490ARATH, Ananas comosus IAA-amino acid hydrolase ILR1-like 3 and Late exocytosis, associated with Golgi transport. According to Li and Roberts (2001), the Vicilin-like seed storage protein At2g28490 protein family is a representation of the conserved barrel domain of the ‘cupin’ superfamily. This family contains 11S and 7S plant seed storage proteins, and germins. Plant seed storage proteins provide the major nitrogen source for the developing plant (Dunwell, 1998). Also, Ananas comosus IAA-amino acid hydrolase gene consisted of 4 beta strands and two alpha helices which make up the dimerization surface of members of the M20 family of peptidases. This family includes a range of zinc metallopeptidases belonging to several families in the peptidase classification. Family M20 are Glutamate carboxypeptidases. Peptidase family M25 contains X-His dipeptidases (Rowsell et al., 1997).
The identification of protein function led to the discovery of two putative genes related to dry matter content. The potential genes identified on chromosome 18 including Transcription initiation factor TFIID subunit 12b and Growth-regulating factor 1 at 24.1 Mb and 21.6 Mb respectively. The TFIID is one of several General Transcription Factors (GTFs), which also include TFIIA, TFIIB, TFIIE, TFIIF and TFIIH, that are involved in the accurate initiation of transcription by RNA polymerase II in eukaryotes (Gazit et al., 2009). TFIID plays an important role in the recognition of promoter DNA and assembly of the pre-initiation complex (Gazit et al., 2009). In addition, Kim (Kim et al., 2003) indicated that the WRC domain, named after the conserved Trp-Arg-Cys motif, contains two distinctive features: a putative nuclear localization signal and a zinc-finger motif (C3H). It is suggested that the WRC domain functions in DNA binding. In their study on water yams, (Gatarira et al., 2020) presented findings that found QTLs correlated with tuber dry matter at different sites. (Kayondo et al., 2018) discovered similar results and revealed that traits such as panel size, harvest time, and environment influence the discovery of quantitative trait loci (QTLs) related to virus disease. To address this issue, the most effective approach for enhancing the connections between traits and (QTLs) is to combine phenotypic scores from numerous locations with genotypic data utilizing diverse panels of individuals (Jing et al., 2018).
The study revealed that loci with varying effects can influence the variability of the traits examined in Dioscorea rotundata. This study identified distinct genomic regions harboring genes related to flowering development and disease resistance in yam germplasm. These findings should be further confirmed and evaluated. To do this, the QTLs may be converted into cost-effective Kompetitive Allele-Specific PCR (KASPs) markers, which can then be efficiently utilized to transfer alleles into high-quality yam genotypes. The results of this work might potentially aid in the development of novel breeding strategies for preserving advantageous genetic traits related to disease resistance and tuber production in certain yam genotypes, with the aim of enhancing future marker-based breeding efforts. The chromosomal regions responsible for these analyzed traits might be utilized for the purpose of selecting and efficiently combining advantageous alleles in order to enhance the population of Dioscorea rotundata. Certain roles and characteristics of the identified genes remain unexplored. Future studies on investigating the action mode of these genes in yam will help to elucidate the expression/regulation of these
4 Conclusion
Using five models, we identified 12 SNP markers associated with the three important traits. Through the allele’s analysis, we identified as well promising alleles to be used for markers-assisted selection. Several genes linked to plant defense mechanism, plant growth, to tuber accumulation were reported. Future investigation such as gene expression may be required for gene profiling to understand the genetic basis in Uganda’s D. rotundata gene pool. Moreover, the discoveries might prove valuable for the verification and integration of markers in the process of yam breeding.
Data availability statement
The original contributions presented in the study are publicly available. This data can be found here: FigShare, https://figshare.com/articles/dataset/good_vcf_uganda_emma_vcf/24957663?file=43950204.
Author contributions
EA: Conceptualization, Data curation, Formal analysis, Funding acquisition, Investigation, Methodology, Project administration, Resources, Software, Supervision, Validation, Visualization, Writing – original draft, Writing – review & editing. TO: Conceptualization, Supervision, Validation, Visualization, Writing – review & editing. WE: Conceptualization, Supervision, Validation, Visualization, Writing – review & editing. RB: Validation, Visualization, Writing – review & editing. PAr: Formal analysis, Software, Validation, Visualization, Writing – review & editing. PAd: Resources, Visualization, Writing – review & editing. EC: Visualization, Writing – review & editing. AA: Formal analysis, Validation, Visualization, Writing – review & editing. ID: Validation, Visualization, Writing – review & editing. SM: Visualization, Writing – review & editing. RE: Funding acquisition, Visualization, Writing – review & editing. AO: Validation, Visualization, Writing – review & editing. MO-S: Visualization, Writing – review & editing. TA: Funding acquisition, Visualization, Writing – review & editing.
Funding
The author(s) declare financial support was received for the research, authorship, and/or publication of this article. This study was jointly supported by funding from the Bill and Melinda gate foundation project through African yam project of the international Institute of Tropical Agriculture (IITA) (OPP1052998), World Bank project through Makerere Regional Center for Crop Improvement, Makerere University (MaRCCI) (Grant: IDA Credit No. 57970 UG), the European Union-funded academic mobility project—Scientists in Crop Improvement for Security in Africa (SCIFSA), post-doctoral funding [RU/2022/R1/03] through the Regional Universities Forum for Capacity Building in Agriculture (RUFORUM) and kind support from individuals in the National Agricultural Research Resource Institute, Uganda and Savanna Agriculture Research Institute, Tamale - Ghana.
Acknowledgments
We acknowledge support and resources from Scientists in Crop Improvement for Food Security in Africa (SCIFSA), National Agricultural Research Organization—National Crop Resource Research Institute (NARO-NaCRRI), Makerere Regional Center for Crop Improvement (MaRCCI), Council for Scientific and Industrial Research—Savannah Agricultural Research Institute (CSIR-SARI) and the International Institute of Tropical Agriculture (IITA) for their technical support and experiences shared by all the institutional staff.
Conflict of interest
The authors declare that the research was conducted in the absence of any commercial or financial relationships that could be construed as a potential conflict of interest.
Publisher’s note
All claims expressed in this article are solely those of the authors and do not necessarily represent those of their affiliated organizations, or those of the publisher, the editors and the reviewers. Any product that may be evaluated in this article, or claim that may be made by its manufacturer, is not guaranteed or endorsed by the publisher.
Supplementary material
The Supplementary Material for this article can be found online at: https://www.frontiersin.org/articles/10.3389/fhort.2024.1365567/full#supplementary-material
References
Acquaah G. (2012). Principles of Plant Genetics and Breeding. 2nd ed (UK: John Willey and Sons Ltd).
Adeniji M., Shoyinka S., Ikotun T., Asiedu R., Hughes J., Odu B. (2012). Yield loss in Guinea yam (Dioscorea rotundata Poir.) due to infection by Yam mosaic virus (YMV) genus potyvirus. Ife J. Sci. 14, 237–244.
Adjei E. A., Esuma W., Alicai T., Bhattacharjee R., Dramadri I. O., Agaba R., et al. (2022a). Phenotypic Diversity within Ugandan Yam (Dioscorea species) Germplasm Collection. Int. J. Agronomy. 2022, 1–10. doi: 10.1155/2022/5826012
Adjei E. A., Esuma W., Alicai T., Chamba E. B., Edema R., Dramadri I. O., et al. (2022b). Genotype-by-environment interaction of yam (Dioscorea species) for yam mosaic virus resistance, dry matter content and yield in Uganda. Agronomy. 12, 1984. doi: 10.3390/agronomy12091984
Agre P., Asibe F., Darkwa K., Edemodu A., Bauchet G., Asiedu R., et al. (2019). Phenotypic and molecular assessment of genetic structure and diversity in a panel of winged yam (Dioscorea alata) clones and cultivars. Sci. Rep. 9, 18221. doi: 10.1038/s41598-019-54761-3
Agre P. A., Norman P. E., Asiedu R., Asfaw A. (2021). Identification of quantitative trait nucleotides and candidate genes for tuber yield and mosaic virus tolerance in an elite population of white Guinea yam (Dioscorea rotundata) using genome-wide association scan. BMC Plant Biol. 21, 552. doi: 10.1186/s12870-021-03314-w
Ahn E., Hu Z., Perumal R., Prom L. K., Odvody G., Upadhyaya H. D., et al. (2019). Genome wide association analysis of sorghum mini core lines regarding anthracnose, downy mildew, and head smut. PLoS One 14, e0216671. doi: 10.1371/journal.pone.0216671
Amponsah Adjei E., Esuma W., Alicai T., Bhattacharjee R., Dramadri I. O., Edema R., et al. (2023). Genetic diversity and population structure of Uganda’s yam (Dioscorea spp.) genetic resource based on DArTseq. PLoS One 18. doi: 10.1371/journal.pone.0277537
Asfaw A. (2016). Standard Operating Protocol for Yam Variety Performance Evaluation Trial (IITA). Available online at: https://hdl.handle.net/10568/76212.
Bailer S. M., Balduf C., Hurt E. (2001). The nsp1p carboxy-terminal domain is organized into functionally distinct coiled-coil regions required for assembly of nucleoporin subcomplexes and nucleocytoplasmic transport. Mol. Cell Biol. 21, 7944–7955. doi: 10.1128/MCB.21.23.7944-7955.2001
Barral P., Batanero E., Palomares O., Quiralte J., Villalba M., Rodriíguez R. (2004). A major allergen from pollen defines a novel family of plant proteins and shows intra- and interspecie cross-reactivity. J. Immunol. 172, 3644–3651. doi: 10.4049/jimmunol.172.6.3644
Bhattacharjee R., Agre P., Bauchet G., De Koeyer D., Lopez-Montes A., Kumar P. L., et al. (2020). Genotyping-by-sequencing to unlock genetic diversity and population structure in white yam (Dioscorea rotundata poir.). Agronomy. 10, 1437. doi: 10.3390/agronomy10091437
Bhattacharjee R., Lopez-Montes A., Abberton M., Asiedu R. (2013). “Application of advanced genomic technologies to accelerate yam breeding,” in Yams 2013: First Global Conference on Yam. Available online at: https://biblio.iita.org.
Bradbury P. J., Zhang Z., Kroon D. E., Casstevens T. M., Ramdoss Y., Buckler E. S. (2007). TASSEL: software for association mapping of complex traits in diverse samples. Bioinformatics 23. doi: 10.1093/bioinformatics/btm308
Cuevas H. E., Prom L. K., Cooper E. A., Knoll J. E., Ni X. (2018). Genome-wide association mapping of anthracnose (Colletotrichum sublineolum) resistance in the U.S. Sorghum Assoc. Panel. Plant Genome. 11, 170099. doi: 10.3835/plantgenome2017.11.0099
De Mendiburu F. (2015). agricolae: Statistical Procedures for Agricultural Research (R package version), 2–8. doi: 10.7287/peerj.preprints.1404v1
Dunwell J. M. (1998). Cupins: A new superfamily of functionally diverse proteins that include germins and plant storage proteins. Biotechnol. Genet. Eng. Rev. 15, 1–32. doi: 10.1080/02648725.1998.10647950
Forbes G., Pérez W., Andrade-Piedra J. L. (2014). Field assessment of resistance in potato to Phytophthora infestans. Lima (Peru): International Potato Center (CIP), 35 p. doi: 10.4160/9789290604402
Gatarira C., Agre P., Matsumoto R., Edemodu A., Adetimirin V., Bhattacharjee R., et al. (2020). Genome-Wide association analysis for tuber dry matter and oxidative browning in Water Yam (Dioscorea alata L.). Plants. 9, 969. doi: 10.3390/plants9080969
Gazit K., Moshonov S., Elfakess R., Sharon M., Mengus G., Davidson I., et al. (2009). TAF4/4b·TAF12 displays a unique mode of DNA binding and is required for core promoter function of a subset of genes. J. Biol. Chem. 284, 26286–26296. doi: 10.1074/jbc.M109.011486
Girma G., Nida H., Seyoum A., Mekonen M., Nega A., Lule D., et al. (2019). A large-scale genome-wide association analyses of Ethiopian Sorghum landrace collection reveal loci associated with important traits. Front. Plant Sci. 10. doi: 10.3389/fpls.2019.00691
Haneishi Y., Okello S. E., Asea G., Tsuboi T., Maruyama A., Kikuchi M. (2013). Exploration of rainfed rice farming in Uganda based on a nationwide survey: Evolution, regionality, farmers and land. Afr J. Agric. Res. 8, 3318–3329. Available at: http://www.academicjournals.org/AJAR.
Hanks S. K., Quinn A. M. (1991). Protein kinase catalytic domain sequence database: Identification of conserved features of primary structure and classification of family members. Methods Enzymol. 200, 38–62. doi: 10.1016/0076-6879(91)00126-H
Henrissat B., Davies G. J. (2000). Glycoside hydrolases and glycosyltransferases. Families, modules, and implications for genomics. Plant Physiol. 124, 1515–1519. doi: 10.1104/pp.124.4.1515
Hunter S., Jones P., Mitchell A., Apweiler R., Attwood T. K., Bateman A., et al. (2012). InterPro in 2011: new developments in the family and domain prediction database. Nucleic Acids Res. 40, D306–D312. doi: 10.1093/nar/gks456
Jing Y., Zhao X., Wang J., Teng W., Qiu L., Han Y., et al. (2018). Identification of the Genomic Region Underlying Seed Weight per Plant in Soybean (Glycine max L. Merr.) via High-Throughput Single-Nucleotide Polymorphisms and a Genome-Wide Association Study. Front. Plant Sci. 9. doi: 10.3389/fpls.2018.01392
Kagoda F., Coyne D. L., Asiedu J., Wanyera N., Mudiope J., Dusabe J., et al. (2005). “Yam germplasm conservation and screening in Uganda,” in 7th African Crop Science Conference Proceedings. Eds. Tenywa J. S., Adipala E., Nampala P., Tusiime G., Okori P., Kyamuhangire W. (Entebbe, Uganda), 245–249.
Kayondo S. I., Pino Del Carpio D., Lozano R., Ozimati A., Wolfe M., Baguma Y., et al. (2018). Genome-wide association mapping and genomic prediction for CBSD resistance in Manihot esculenta. Sci. Rep. 8, 1549. doi: 10.1038/s41598-018-19696-1
Kilian A., Sanewski G., Ko L. (2016). The application of DArTseq technology to pineapple. Acta Hortic. 1111, 181–188. doi: 10.17660/ActaHortic.2016.1111.27
Kilimo Trust. (2013). Analysis of Demand and Value chain of Yams in Tanzania, Uganda and the Rest of East African Community Vol. 1 (Tanzania). Available at: www.kilimotrust.org.
Kim J. H., Choi D., Kende H. (2003). The AtGRF family of putative transcription factors is involved in leaf and cotyledon growth in Arabidopsis. Plant J. 36, 94–104. doi: 10.1046/j.1365-313X.2003.01862.x
Li B., Liu Y., Uno T., Gray N. (2004). Creating chemical diversity to target protein kinases. Comb Chem. High Throughput Screen. 7, 453–472. doi: 10.2174/1386207043328580
Li D., Roberts R. (2001). Human Genome and Diseases:¶WD-repeat proteins: structure characteristics, biological function, and their involvement in human diseases. Cell. Mol. Life Sci. 58, 2085–2097. doi: 10.1007/PL00000838
Loko Y., Bhattacharjee R., Agre A., Dossou-Aminon I., Orobiyi A., Djedatin G., et al. (2017). Genetic diversity and relationship of Guinea yam (Dioscorea cayenensis Lam.–D. rotundata Poir. complex) germplasm in Benin (West Africa) using microsatellite markers. Genet. Genet. Resour. Crop Evol. 64, 1205–1219. doi: 10.1007/s10722-016-0430-z
Manning G., Whyte D. B., Martinez R., Hunter T., Sudarsanam S. (2002). The protein kinase complement of the human genome. Science (1979) 298, 1912–1934. doi: 10.1126/science.1075762
Mignouna H. D., Njukeng P., Abang M. M., Asiedu R. (2001). Inheritance of resistance to Yam mosaic virus, genus Potyvirus, in white yam (Dioscorea rotundata). Theor. Appl. Genet. 103, 1196–2000. doi: 10.1007/s001220100728
Mondo J. M., Agre P. A., Asiedu R., Akoroda M. O., Asfaw A. (2021). Genome-wide association studies for sex determination and cross-compatibility in water yam (Dioscorea alata L.). Plants. 10, 1412. doi: 10.3390/plants10071412
Mouyna I., Monod M., Fontaine T., Henrissat B., Léchenne B., Latgé J. P. (2000). Identification of the catalytic residues of the first family of β(1–3)glucanosyltransferases identified in fungi. Biochem. J. 347, 741–747. doi: 10.1042/bj3470741
Nemorin A., Abraham K., David J., Arnau G. (2012). Inheritance pattern of tetraploid Dioscorea alata and evidence of double reduction using microsatellite marker segregation analysis. Mol. Breeding. 30, 1657–1667. doi: 10.1007/s11032-012-9749-0
Norman P. E., Paterne A. A., Danquah A., Tongoona P. B., Danquah E. Y., De Koeyer D., et al. (2020). Paternity assignment in white Guinea yam (Dioscorea rotundata) half-sib progenies from polycross mating design using SNP markers. Plants. 9, 527. doi: 10.3390/plants9040527
Nsubuga F. W., Olwoch J. M., Rautenbach CJ de W. (2011). Climatic trends at namulonge in Uganda: 1947-2009. J. Geogr. Geology 3. doi: 10.5539/jgg.v3n1p119
R Core Team (2022). R: A language and environment for statistical computing (Vienna, Austria: R Foundation for Statistical Computing). Available at: https://www.R-project.org/.
Rowsell S., Pauptit R. A., Tucker A. D., Melton R. G., Blow D. M., Brick P. (1997). Crystal structure of carboxypeptidase G2, a bacterial enzyme with applications in cancer therapy. Structure. 5, 337–347. doi: 10.1016/S0969-2126(97)00191-3
Shin J. H., Blay S., Graham J., McNeney B. (2006). LDheatmap : an R function for graphical display of pairwise linkage disequilibria between single nucleotide polymorphisms. J. Stat. Softw 16. doi: 10.18637/jss.v016.c03
Siadjeu C., Pucker B., Viehöver P., Albach D. C., Weisshaar B. (2020). High Contiguity de novo Genome Sequence Assembly of Trifoliate Yam (Dioscorea dumetorum) Using Long Read Sequencing. Genes (Basel). 11, 274. doi: 10.3390/genes11030274
Sorho Fatogoma Brahima Kone YKA& GOEDJB, Ettien Djecthi Jean Baptiste SFBKYKA& GO (2014). Productivity of new yam assessions as affected by mosaic virus in transition forest-savanna zone of côte D’ivoire. Int. J. Agric. Sci. Res. (Chennai) 4, 137–146. Available at: http://www.tjprc.org/view-archives.php?year=2014&id=50&jtype=2&page=3.
Stout T., Foster P., Matthews D. (2004). High-throughput structural biology in drug discovery: protein kinases. Curr. Pharm. Des. 10, 1069–1082. doi: 10.2174/1381612043452695
Sugihara Y., Darkwa K., Yaegashi H., Natsume S., Shimizu M., Abe A., et al. (2020). Genome analyses reveal the hybrid origin of the staple crop white Guinea yam (Dioscorea rotundata). Proc. Natl. Acad. Sci. 117, 31987–31992. doi: 10.1073/pnas.2015830117
Tamba C., Zhang Y. (2018). A fast mrMLM algorithm for multi-locus genome-wide association studies. BioRxiv. doi: 10.1101/341784
Tamiru M., Natsume S., Takagi H. H., White B., Yaegashi H., Shimizu M., et al. (2017). Genome sequencing of the staple food crop white Guinea yam enables the development of a molecular marker for sex determination. BMC Biol. 15, 1–20. doi: 10.1186/s12915-017-0419-x
Troyanskaya O., Cantor M., Sherlock G., Brown P., Hastie T., Tibshirani R., et al. (2001). Missing value estimation methods for DNA microarrays. Bioinformatics. 17, 520–525. doi: 10.1093/bioinformatics/17.6.520
Wang S. B., Feng J. Y., Ren W. L., Huang B., Zhou L., Wen Y. J., et al. (2016). Improving power and accuracy of genome-wide association studies via a multi-locus mixed linear model methodology. Sci. Rep. 6, 19444. doi: 10.1038/srep19444
Wen Y. J., Zhang H., Ni Y. L., Huang B., Zhang J., Feng J. Y., et al. (2018). Methodological implementation of mixed linear models in multi-locus genome-wide association studies. Brief Bioinform. 19, 700–712. doi: 10.1093/bib/bbw145
Wu Z. G., Jiang W., Mantri N., Bao X. Q., Chen S. L., Tao Z. M. (2015). Transciptome analysis reveals flavonoid biosynthesis regulation and simple sequence repeats in yam (Dioscorea alata L.) tubers. BMC Genomics. doi: 10.1186/s12864-015-1547-8
Zhang J., Feng J. Y., Ni Y. L., Wen Y. J., Niu Y., Tamba C. L., et al. (2017). pLARmEB: integration of least angle regression with empirical Bayes for multilocus genome-wide association studies. Heredity (Edinb). 118, 517–524. doi: 10.1038/hdy.2017.8
Keywords: Dioscorea rotundata, Uganda, DArTseq, marker-trait association, gene annotation, mapping
Citation: Adjei EA, Odong TL, Esuma W, Bhattacharjee R, Agre PA, Adebola PO, Chamba EB, Asfaw A, Dramadri IO, Mbabazi ST, Edema R, Ozimati AA, Ochwo-Ssemakula M and Alicai T (2024) Genome-wide mapping uncovers significant quantitative trait loci associated with yam mosaic virus infection, yield and dry matter content in White Guinea yam (Dioscorea rotundata Poir.). Front. Hortic. 3:1365567. doi: 10.3389/fhort.2024.1365567
Received: 04 January 2024; Accepted: 09 September 2024;
Published: 04 October 2024.
Edited by:
Dionysia A. Fasoula, Agricultural Research Insitute, CyprusReviewed by:
Vijay Bahadur Singh Chauhan, Central Tuber Crops Research Institute (ICAR), IndiaFilippo Biscarini, National Research Council (CNR), Italy
Copyright © 2024 Adjei, Odong, Esuma, Bhattacharjee, Agre, Adebola, Chamba, Asfaw, Dramadri, Mbabazi, Edema, Ozimati, Ochwo-Ssemakula and Alicai. This is an open-access article distributed under the terms of the Creative Commons Attribution License (CC BY). The use, distribution or reproduction in other forums is permitted, provided the original author(s) and the copyright owner(s) are credited and that the original publication in this journal is cited, in accordance with accepted academic practice. No use, distribution or reproduction is permitted which does not comply with these terms.
*Correspondence: Paterne Angelot Agre, p.agre@cgiar.org