- 1Crops Research Institute, Council for Scientific and industrial Research (CSIR), Kumasi, Ghana
- 2College of Science and Technology, Council for Scientific and industrial Research (CSIR), Kumasi, Ghana
- 3Plant Genetic Resources Research Institute, Council for Scientific and industrial Research (CSIR), Bunso, Ghana
Maize is a major cereal crop for humans and animals. The production and consumption of maize are adversely affected by aflatoxin contamination resulting in reduced grain quality with high health risk to consumers. Unsafe aflatoxin levels above 20 ppb have been reported in maize from farmers’ fields in Ghana. A study was undertaken to evaluate the yield and aflatoxin contamination resistance stabilities of selected hybrids being developed in Ghana. Eighteen maize genotypes (14 hybrids with the following code names: GH01, GH02, GH03, GH04, GH05, GH06, GH07, GH08, GH09, GH10, GH11, GH12, GH13, and GH14 and four local checks: Etubi, Obotantim, Tintim, and Mamaba) were evaluated across six environments in two seasons. Artificial inoculation was done randomly using the side needle injection method 14 days after midsilk at a concentration of 9 × 107 conidia/ml. After the harvest levels of aflatoxin in the harvested grains were determined using high-performance liquid chromatography (HPLC). Data analysis showed that genotypic effects and environmental effects on some traits were consistently significant across environments, which mean that there was useful variation among the genotypes that could be utilized for the development of hybrids. Non-significant genotype by environment interaction effects for grain yield suggests that a promising genotype selected in one of these locations will also be suitable for production in the other locations in the same agroecological zone. GGE biplot analyses provided clear bases for determining stability and performance of the hybrids. Two hybrids have the potential for production in Ejura, Fumesua, Ohawu, Akumadan, and Kpeve and similar agroecological zones. GH04, GH10, and GH01 were lowest yielding, but GH10 was most stable. GH05 was identified as the most promising for production in Ejura, Ohawu, and Akumadan. Again, GH08 was the Vertex genotype for Wenchi environment. Ejura, located in the transition zone, was identified as the ideal testing environment for the hybrids. Most of the hybrids showed low levels of aflatoxin accumulation below 20 ppb despite artificial inoculation. The study revealed GH01, GH05, and GH08 as the most stable among the top yielding hybrids with aflatoxin levels of 11.9, 14.3, and 3.8 ppb, respectively, after artificial inoculation, which can be considered for cultivation as varieties to help mitigate aflatoxin contamination in maize in Ghana and beyond.
Introduction
Maize (Zea mays L) is a major cereal crop for both human and animal nutrition worldwide (Atmaca et al., 2015). In Ghana, maize is the most important cereal crop accounting for 58% of local cereal that is produced and consumed (Scheiterle and Birner, 2016). Maize is cultivated in all the agroecological zones (Agyare et al., 2015) on an area of about 1,189,000 ha with a national average yield of 2.48 mt/ha in farmers’ fields (MoFA, 2017).
Maize production is constrained by both biotic and abiotic stressors. Biotic stressors such as diseases and pests attacks as well as aflatoxin contamination caused by Aspergillus flavus affect productivity, quality of grains, and income of farmers. Abiotic factors such as poor soil conditions and climatic factors also expose maize crop to yield losses, which can result in food insecurity.
The production and consumption of maize in Ghana are particularly affected by mycotoxins (aflatoxin) contamination, which results in reduced grain quality and its wholesomeness to be used as food or feed. Aflatoxins are secondary fungal toxic metabolites that are produced by Aspergillus species such as Aspergillus parasiticus, Aspergillus nomius, and Aspergillus flavus mainly on grains and nuts. There are more than 10 compounds classified as aflatoxin with the most important ones being B1, B2, B2a, G1, G2, M1, M2, Q1, R0, and P1 (Moreno and Kang, 1999). Aflatoxins B1, B2, G1, and G2 are all considered harmful in this order of toxicity B1>G1>B2>G2 LD50 0.36, 0.78, 1.70–3.44 mg/kg, respectively, to humans.
Aflatoxin production on the field is influenced by high temperature and humidity, which is favorable to the Aspergillus growth and contamination of grains on the field (Namjoo et al., 2016). Its presence is also enhanced by factors such as stress or injury to the crop due to drought before harvest, insect activity, soil type, and poor storage conditions (Alcaide-Molina et al., 2009).
The presence of aflatoxins in food and feed makes them unsafe for consumption as there are reports of serious health hazards it poses (Moreno and Kang, 1999). When ingested, inhaled, or adsorbed into the body, it can lead to primary hepatocellular carcinoma (Namjoo et al., 2016), hepatotoxic (capable of causing liver cancer), teratogenic (capable of effecting deformities in embryo), and mutagenic effects in human and animals at very low concentrations (Espinosa-Calderón et al., 2009). Kenya recorded an incidence of deaths as a result of consumption of aflatoxin contaminated commodity in 2004 (Probst et al., 2007). According to EU-European Commision (2018), food commodities from Ghana were rejected at the European border due to high level of detectable aflatoxins. Aflatoxin contamination has negative impact on economic growth and trade (Bandyopadhyay et al., 2017), because food and feed commodities with high levels of aflatoxin cannot enter the premium markets of developed countries with strict testing procedures.
The European Union (EU) has a rigorous regulation that set the limit for aflatoxins at 5 and 10 ppb for aflatoxin B1 and total aflatoxin, respectively (Espinosa-Calderón et al., 2009). According to FAO (2011), maize for human consumption should not exceed 5 μg/kg for aflatoxin contamination. In Ghana, aflatoxin limit is set at 8 ppb for maize produced in the country by Ghana Standard Authority (GSA, 2018).
There are many interventional technologies for the control of aflatoxin contamination. These include good agricultural practices, monitoring and crop destruction, postharvest interventions (Bandyopadhyay et al., 2017), and the use of genotypes resistant to aflatoxin contamination (Warburton and Williams, 2014). In Ghana, the commodities market is less developed; hence, there is very little enforcement of standards and grades. This situation means health and safety of consumers cannot be assured (Acheampong et al., 2017). Therefore, the safe measure to aflatoxin contamination in this part of the world and for most developing countries where food quality standard checks and monitoring are less developed will be the use of resistant maize varieties.
Currently, there are no released maize varieties with resistance to aflatoxin contamination in the country; hence, any approach that contributes to the release of aflatoxin resistant varieties is a great step in the area of aflatoxin research for food safety for both consumers and maize farmers. This study was therefore conducted with the main objective to evaluate the performance of CSIR-CRI maize genotypes with promising resistance to aflatoxin contamination across six (6) different locations in two seasons to ascertain their yield stability as well as resistance to aflatoxin contamination in Ghana.
The specific objectives of the study were as follows:
● To determine the yield stability of aflatoxin accumulation resistant hybrids across six (6) locations in two (2) seasons.
● To identify stable hybrids with low level of aflatoxin contamination.
Materials and methods
Experimental material
The experiment comprised 18 entries of maize genotypes consisting of four local checks and 14 single cross hybrids. These materials were obtained from Corn Host Plant Resistance Research Unit (CHPRRU) of the USDA ARS, Station at Starkville, MS and some adapted local inbred lines received from IITA, Ibadan, Nigeria. The characteristics of the varieties used are summarized in Table 1.
The research was carried out in six locations. The locations were Ejura (7° 23’ 0” North, 1° 22’ 0” transition ecology, fine coarse sandy loam Oxisol), Fumesua (6°41 North, 1°28 deciduous forest, ferric acrisols, Akumadan (7° 24’ 0” North, 1° 57’ 0” West), Wenchi (80 45’N, 20 6’W,33), Kpeve (30 20’N, 00 17’E–coastal savannah, achrosols) and Ohawu both in the Volta region.The average yearly minimum and maximum temperatures of the agroecological zones were Fumesua 21°C minimum and 33°C maximum, Wenchi 21.2°C minimum and 31°C maximum, and Akomadan 20°C minimum and 35°C maximum, whereas Ejura was 24°C minimum and 33°C maximum.3.1
The fields were disc plowed and harrowed before planting to ensure good tillage. Glyphosate at 1.5 l/ha was applied soon after planting to control weed growth. The seeds of the 18 hybrids for the two seasons (major and minor) were generated by planting the male and female inbred lines in a crossing block. Artificial pollination was done by introducing the pollen of the male inbred lines to their respective female inbred line. At maturity, the seeds were harvested, dried, treated, and packed for planting.
Planting for the major season was carried out between April and May 2019 in all six locations when there was appreciable rainfall. However, planting was carried out sequentially with intermittent planting dates between the various locations to ensure sequential harvesting at the various locations. Planting in the minor season was carried out in a similar manner beginning from 3 September to second week of September 2019. In all plantings, the randomized complete block design (RCBD) was used with three replications for each treatment in each location. Each of the 18 genotypes were sown at three seeds per hill and thinned to two plants per hill after 2 weeks. Each plot consisted of two rows on a 5-m long plot, 0.75-m inter-rows, and 0.4-m intra-row spacing. Post-emergence herbicide, Atrazine™, was used to control weeds immediately after sowing.
The application of NPK 15:15:15 was applied at the rate of 250 and 60 kg/ha of P2O5 as basal fertilizer at 2 weeks after sowing and top dressed with additional N 60 kg/ha at 4 weeks after planting. Hoe and hand weeding was also done when necessary to control weeds during the growing period. Fall armyworm was controlled by the application of Emamectin benzoate at manufacturer’s recommendation in every 2 weeks to control fall armyworm (Spodoptera frugiperda) infestation and other management practices were carried out as required for each area.
Inoculum preparation
The identified toxigenic isolate of Aspergillus sp was used to prepare the inoculum as described by Windham and Williams (2002). The procedure involved multiplication of the isolate on sterile corn cob grit in 500-ml flasks each containing 50 g of grits and 100 ml of sterile distilled water and incubated at 28°C for 3 weeks. Conidia in each flask was washed from the grits using 500 ml of sterile distilled water containing 20 drops of Tween 20 per liter and then filtered through four layers of sterile cheesecloth. The concentration of conidia was determined with a hemacytometer and adjusted with sterile distilled water to 9 × 107 conidia per ml. Excess inoculum not used immediately was refrigerated at 4°C.
Inoculation
The side needle method was used for inoculating ears of five plants at random per plot 14 days after midsilk. A preparation of 3.4 ml of a spore suspension of 9 × 107 conidia/ml was injected over the kernels as reported by Scott and Zummo (1994).
Harvesting and processing
Each plot was harvested when matured at a moisture content of 18–20%. Cobs from each plot were harvested into separate bags labeled with the plot number and weight taken. At processing, the maize was shelled plot by plot and the grain weight data were taken together with their respective moisture contents. Samples of the grains were taken to the laboratory to determine the aflatoxin contents.
Aflatoxin analysis
Aflatoxin was extracted using methods described by Sirhan et al. (2013), with modifications. Samples were milled using a Preethi Mixer Grinder into homogenized flour. A weight of 2 g of slurry was weighed into a 15-ml centrifuge tube and topped-up with a 4 ml of 60:40 (v/v) methanol:acetronitrile solution. The resultant mixture was vortexed using Genie Vortex machine for 3 min. 1.32 g of anhydrous MgSO4 and 0.2 g of NaCl were added to the mixture and then vortexed for 1 min. The tube was centrifuged for 5 min at 4000 rpm and the upper organic layer filtered through a 0.45 μm nylon syringe prior to injection. A volume of 100 μl of the filtered extract was injected into the HPLC.
A Cecil-Adept Binary Pump HPLC coupled with Shimadzu 10 AxL fluorescence detector (Ex: 360 nm, Em: 440 nm) with Phenomenex HyperClone BDS C18 Column (150 × 4.60 mm, 5 μm). The mobile phase used was methanol: water (40:60, v/v) at a flow rate of 1 ml/min with column temperature maintained at 40°C. To 1 L of mobile phase were added 119 mg of potassium bromide and 350 μl of 4 M nitric acid (required for postcolumn electrochemical derivatization with Kobra Cell, R-Biopharm Rhone). Aflatoxin Mix (G1, G2, B1, and B2) standards (ng/g) were prepared from Supelco® aflatoxin standard of 2.6 ng/μl in methanol. The concentrations of B1 and G1 were 0.5, 1, 2, 8, and 16 ng/g per 100 μl injection.
The concentrations of B2 and G2 were 0.15, 0.3, 0.6, 2.4, and 4.8 ng/g per 100 μl injection. The limit of detection and the limit of quantification of total aflatoxin were established at 0.5 and 1 ng/g, respectively. Aflatoxin concentration was estimated as ng/g = A × (T/I) × (1/W), where A = ng of aflatoxin as eluate injected, T = final test solution eluate volume (μl), I = volume eluate injected into LC (μl), and W = mass (g) of commodity represented by final extract.
Data collection
The following data were recorded on all plants across locations:
1. Days to anthesis: The number of days from the date of planting to when 50% of the maize plants tassel and sheds pollen.
2. Days to silking: The number of days from the date of planting to when 50% of the silk emerges.
3. Plant height: Measured with a graduated stick on five randomly selected plants and measured from the ground level to the node bearing the flag leaf.
4. Ear height: Measured by using the same plants from which the heights were taken; the height of the ear from ground level to the node bearing the uppermost ear were recorded.
5. Ear aspect: Based on a scale of 1–5 where 1 = clean, uniform, large, and well-filled ear and 5 = ears with undesirable features, plants were scored.
6. Husk cover: Data on husk cover were taken when the ears were fully developed and about 3 weeks to harvest using a scale of 1–5 rating: 1 = husk tightly arranges and extends beyond ear tip and 5= husk poorly arrange and ear tips exposed.
7. Diseases
8. Diseases were scored based on 1–5 rating. Where 1 = absence of disease and 5 = severe infection.
The following data were computed before the data analysis was done:
8. Anthesis-Silking interval
Anthesis-Silking interval (ASI) was obtained by the difference between the days to anthesis and days to silking.
9. Grain yield
The grain yield in kilograms were calculated for each plot at 15% grain moisture content (Rahman et al., 2007) using the formulas below:
where FW = field weight; MC = percentage moisture content; and 0.83 = shelling coefficient.
Other data taken during harvesting include field weight (the weight of cobs per row measured in kilograms), ears harvested (total number of ears harvested per row), and plants harvested (total number of plants harvested per row).
Data analysis
The data were subjected to the analysis of variance (ANOVA) per location (Table 2) and across location for agronomic traits using the Genstat statistical package (Genstat, 2007).
The grain yield of the individual hybrids was analyzed and used to determine the stability of the genotype using the GGE biplot model (Yan et al., 2001).
Results
Performance of genotypes for aflatoxin accumulation resistance and other agronomic traits across locations and seasons
The genotypes were analyzed across locations for each season and then combined across locations and all seasons. The results on performances of the genotypes across the six locations in all the seasons are presented here. The ANOVA across the six environments in both seasons shows significant (P< 0.05) differences among genotypes with respect to some of the agronomic traits (Table 3). Environment was significant (P< 0.05) for all the traits except days to 50% pollen and days to 50% silking. Genotype was significant (P< 0.05) for most of the traits except yield per hectare, days to 50% pollen, days to 50% silking, ear aspect, plants aspect, ear rot, and MSV (Table 3).
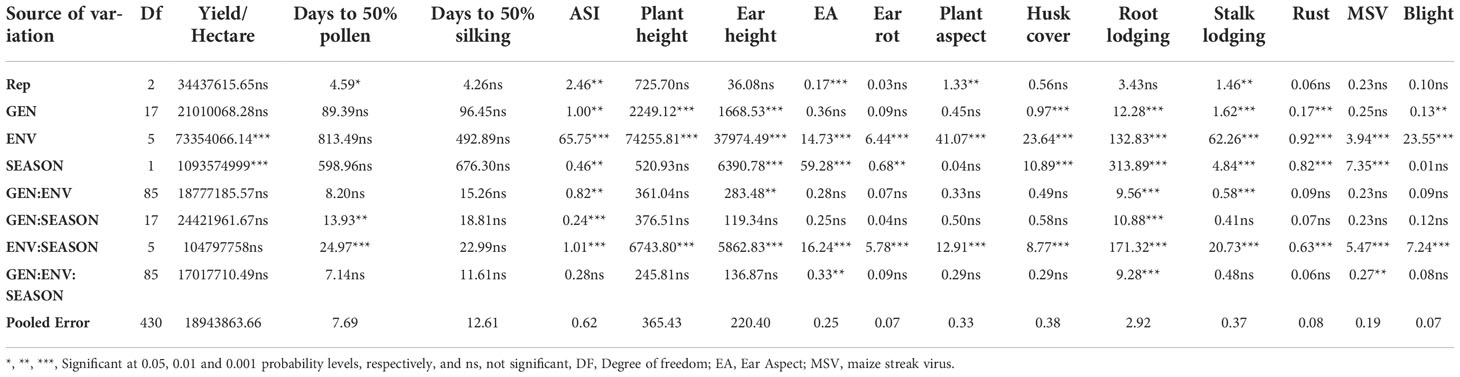
Table 3 Mean squares of 18 maize genotypes evaluated across six environments during both minor and major seasons.
However, genotype by environment interactions was not significant (P< 0.05) for most of the trait except anthesis silking interval, plant height, ear height, root, and stalk lodging (Table 3). Mean squares of the other traits across the environments and seasons can be found in Table 3.
The mean performance of the genotypes had significant differences (P< 0.05) in grain yield and other traits as a result of environmental effect (Table 4). Grain yield varied from 3311.75 kg/ha for GH18 (Etubi) to 4848.20 kg/ha GH11 ENT-5 x T21-8. Genotypic effect for the 18 genotypes showed significant difference (P< 0.05) for most of the traits. Days to 50% pollen ranged from 46 to 52 days, whereas days to 50% silking ranged between 49 and about 55 days (Table 4). The performance of the other traits has been presented in Table 4.
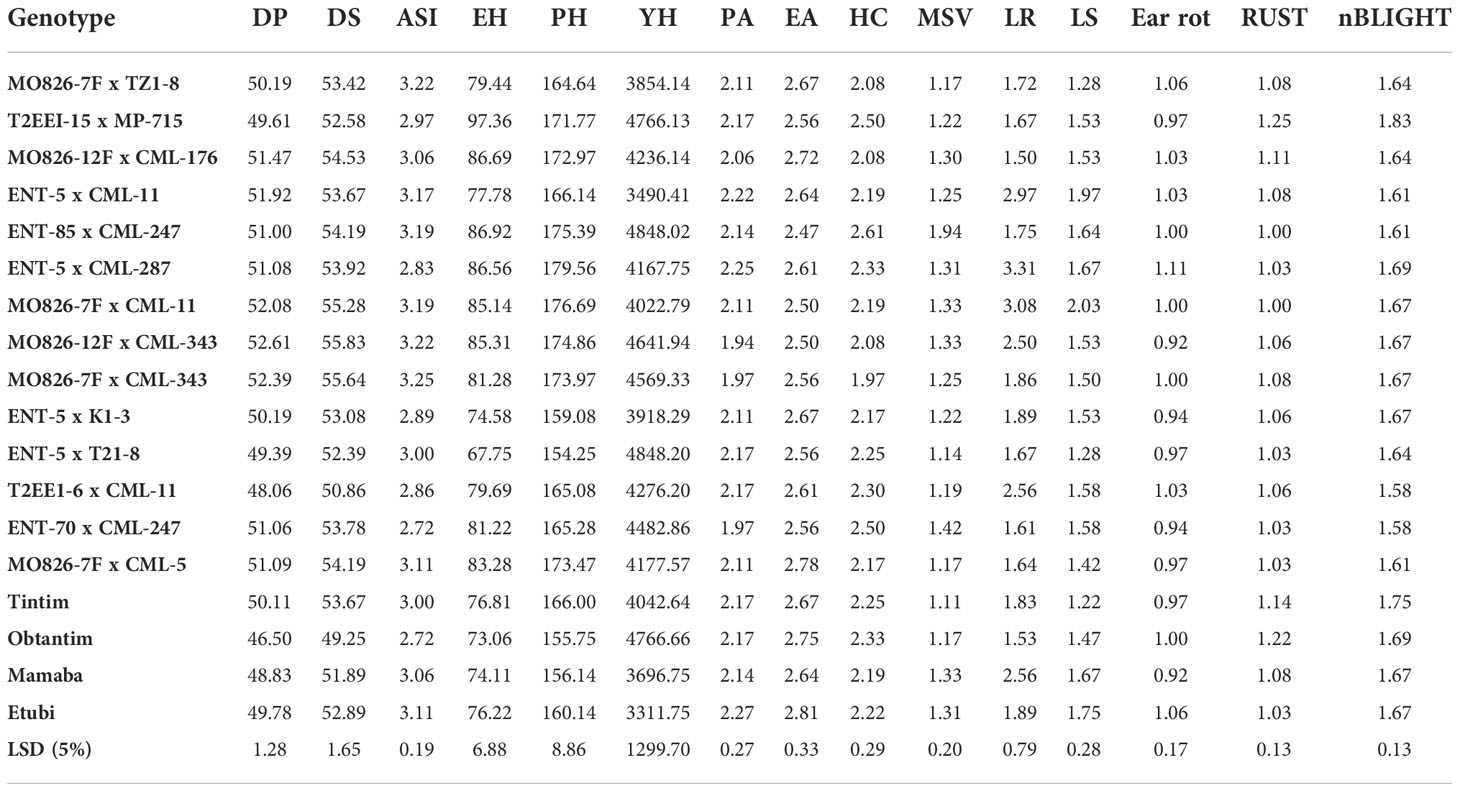
Table 4 Mean performances of 18 genotypes for grain yield, and other agronomic traits across six environments during the major and minor season.
A combined ranking of the top 6 maize genotypes and four checks evaluated in the major and minor seasons across the different locations is a presented in the table below (Table 5). It could be observed that GH11 (ENT-5 x TZ1-8) performed appreciably better, ranking highest in yield (4848.20 kg/ha), followed by GH05 (ENT-85 x CML-247) with average yield of 4848.02 kg/ha. Aflatoxin levels were generally low for the top 6 new maize genotypes ranging from 0 ppb for GH11 (ENT-5 x TZ1-8), GH08 (MO826-12F x CML-343), and GH06 (ENT-5 x CML-287) to 9.6 ppb for genotype GH09 (MO826-7F x CML-343) for the uninoculated, whereas it ranged from 3.6 to 36.6 ppb for the inoculated (Table 5). Aflatoxin accumulation levels for the controls can also be seen in Table 5.
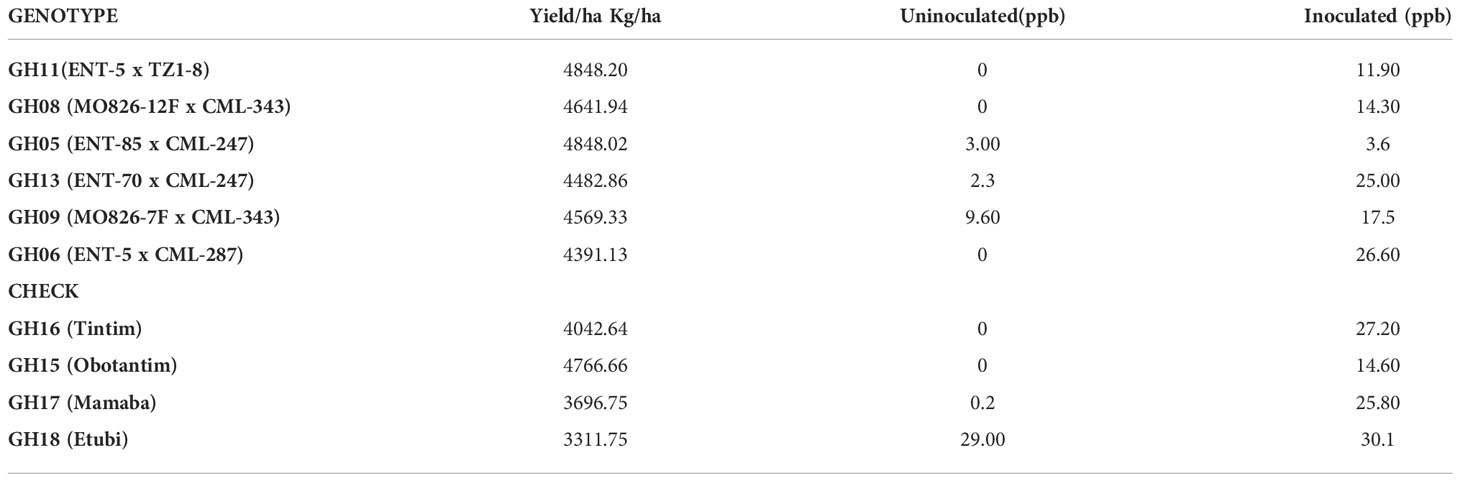
Table 5 Top six yielding and four local genotypes and Aflatoxin levels across 6 environments for both major and minor Seasons.
Stability analysis of the genotypes
The GGE biplot analysis of grain yield for the hybrids (Table 6) together with checks across six environments for the two seasons showed that axis 1 (principal component axis 1) explained 30.32% of the total variation, whereas axis 2 explained 18.78% of the total variation in grain yield with both axis explaining 49.1% of the total variation. The double-arrow line that separates genotypes into two, those with below average means and those with above average means are presented in (Figure 1). The yield of a genotype was estimated by the projections of their representative markers on the average-tester axis, whereas the stability of the genotypes was determined by the projection length of their markers onto the average-tester coordinate y-axis single-arrow line. The longer the absolute length of the projection for a genotype, the less stable it was. The presentation by the GGE biplot analysis revealed that the best top 5 yielding maize genotypes were GH 05 (ENT-85 x CML-247), GH 08 (MO826-12F x CML-343), GH09 (MO826-7F x CML-343), GH13 (ENT-70 x CML-247), and GH 11 (ENT-5 x T21-8). However, the worst performing genotypes were revealed as follows: GH18 (Etubi) followed by GH04 (ENT-5 x CML-11), GH17 (Mamaba), GH10 (ENT-5 x K1-3), and GH01 (MO826-7F X TZ1-8). Even though GH05 (ENT-85 x CML-247) was ranked highest yielding genotype, it was unstable as portrayed by the length of its projection. GH13 (ENT-70 x CML-247) and GH 08 (MO826-12F x CML-343) were most stable among the top 5 yielding genotypes. GH 10 (ENT-5 x K1-3) was identified as poor yielding among the worst of the five genotypes, but it was very stable (Figure 1).
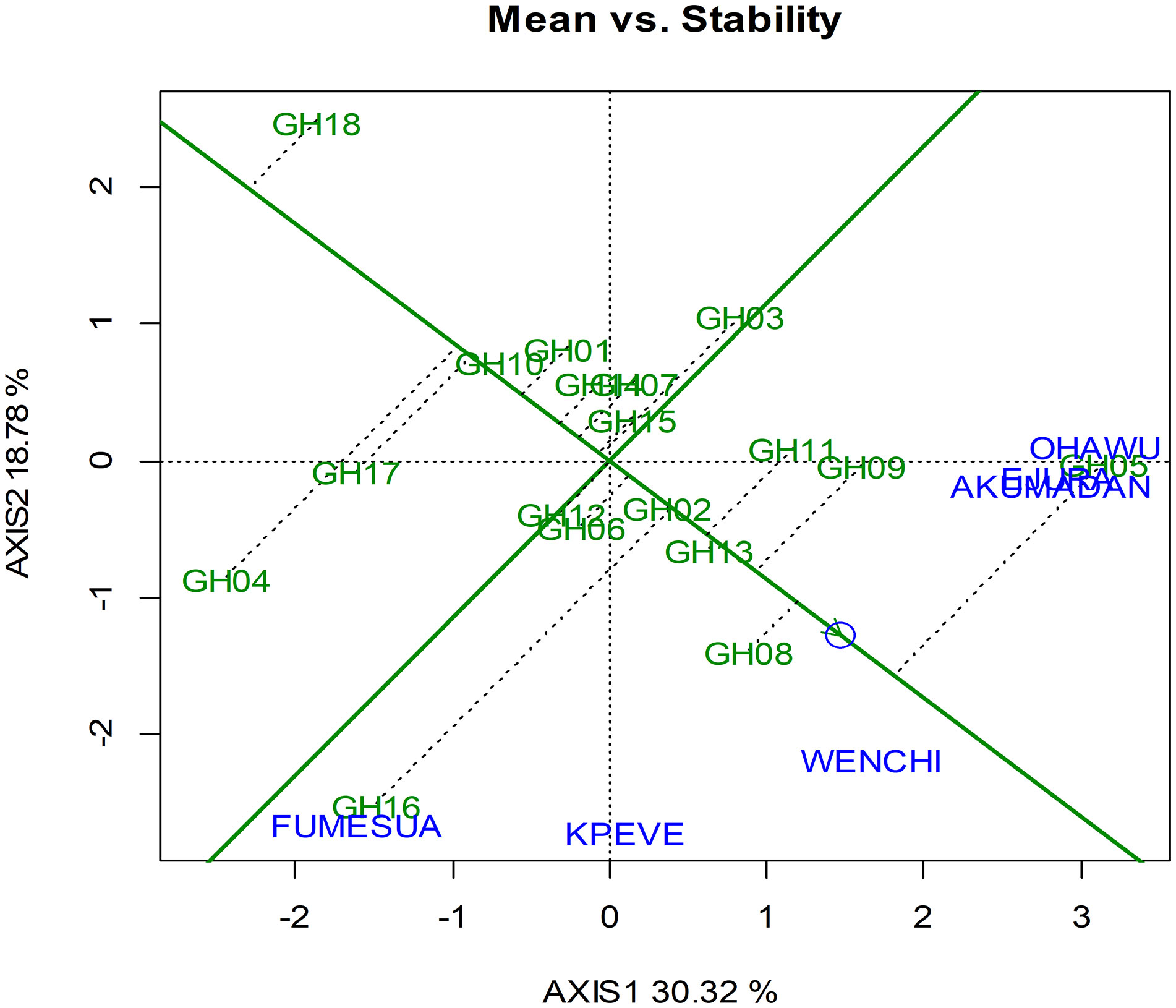
Figure 1 Mean yield and stability biplot of grain yield of 18 hybrids across environments. Another feature of the GGE biplot is its ability to showcase the best performing genotypes in their respective environments and the low yielding once across the environments.
The five sectors observed in the biplot were created by the perpendicular line that starts from the origin of the biplot and runs perpendicular to the side of the polygon. A total of five sectors were recognized out of which three had environments within them and two sectors had no environment within them. Genotypes and environments that fell within the same sectors inferred a relationship between the genotypes with that environment(s). The genotypes at the different vertices of the polygon are likely to be responsive as they are at the extreme from the origin. Although, the responsive vertex genotypes can be either the top performing or the worst at one or other environments (Mohammadi et al., 2010).
The vertex genotype identified for environments Ohawu, Ejura, and Akumadan was GH 05 (ENT-85 x CML-247), whereas GH 16 (Obotantim) was the vertex genotype for Fumesua and Kpeve. Again GH 08 (MO826-12F x CML-343) was the Vertex genotype for Wenchi environment. All other genotypes which were present in the other vertex but did not fall in any test environment were considered to be low-yielding hybrids in those particular environments. They comprised GH 04 (ENT-5 x CML-11) and GH 18 (Etubi). The other genotypes, which did not occupy any environment nor occupy any vertex, were assumed as low yielding; thus, GH 04 (ENT-5 x CML-11), GH 03 (M0826-12F x CML-176), GH 11 (ENT-5 x TZI-8), and GH 13 (ENT-70 x CML-247) were identified as less responsive to the environments (Figure 2).
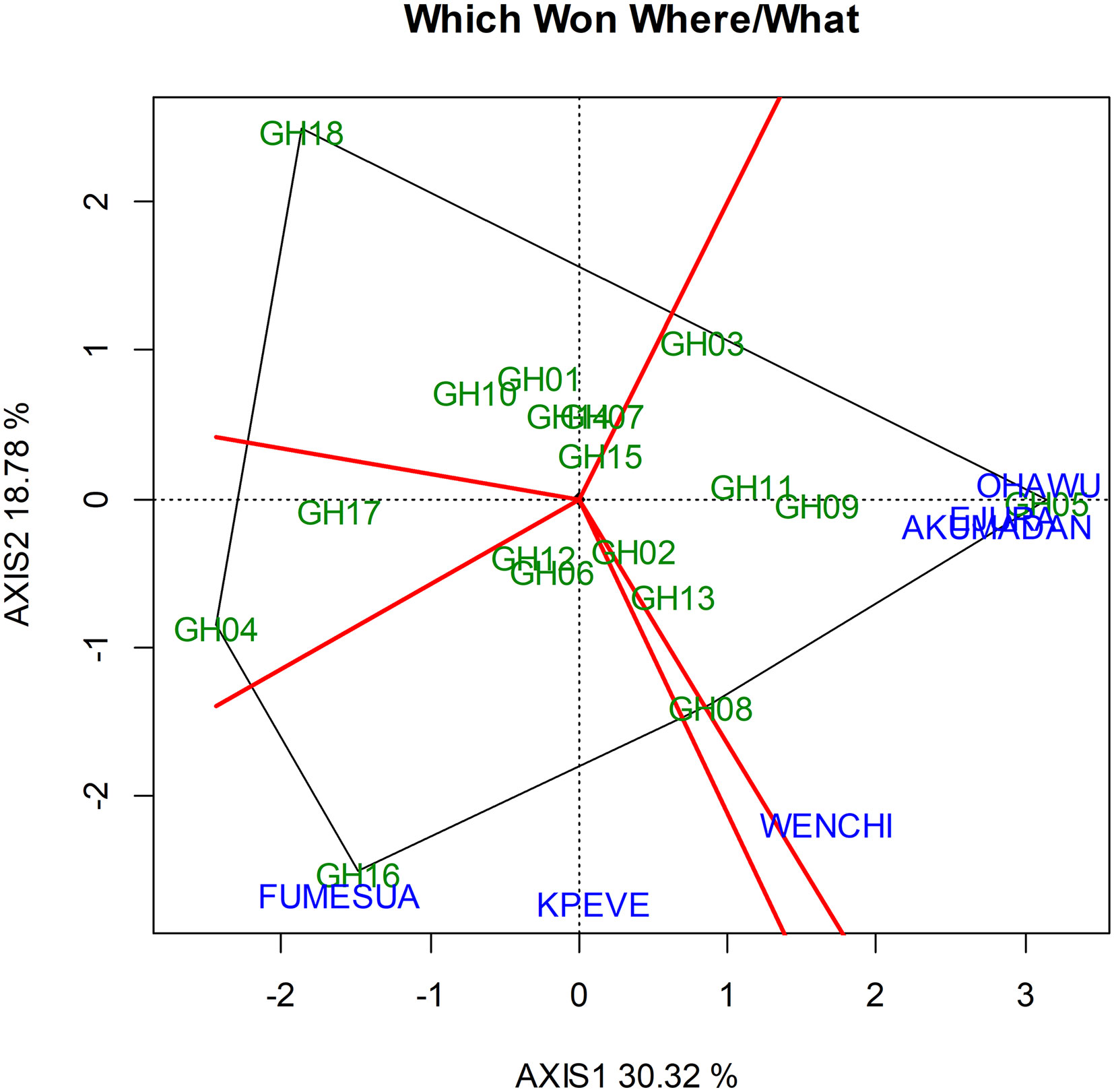
Figure 2 Polygon view of “which won where” GGE biplot of grain yield of the hybrids across environments.
Furthermore, the GGE Biplot identifies the representativeness and discriminating ability of the environments. The lines proceeding from the origin to the coordinates where an environment fall is the research environment vector, whereas a bold straight line which passes through the origin and the average environment represents the average environment axis.
The vector length signifies the discriminating ability of the environment in evaluating genotypes in the test environments. Longer length implies more discriminatory environment. The angle between an environment and the average environment axis determines its representativeness; hence, the shorter projection from the marker of an environment, the more representative the environment. Shorter environmental vectors implies that the specific environments were not strongly interrelated with environments having longer vectors and were possibly not strongly interrelated with each other (Mohammadi et al., 2010). As a result, Fumesua, Kpeve, Wenchi, and Ohawu were identified to be more discriminatory among genotypes as a result of their vector length, whereas Akumadan and Ejura were the least discriminating environments and the most representative (Figure 3). According to Yan and Rajcan (2002), an ideal test environment should be able to discriminate among genotypes and represent their mega-environment. The biplot identified Ejura as the ideal test environment. It can also be deduced that Ohawu and Fumesua, which have long vectors and large angles, can be used in removing unstable genotypes effectively but cannot be used in selecting superior genotypes (Figure 3).
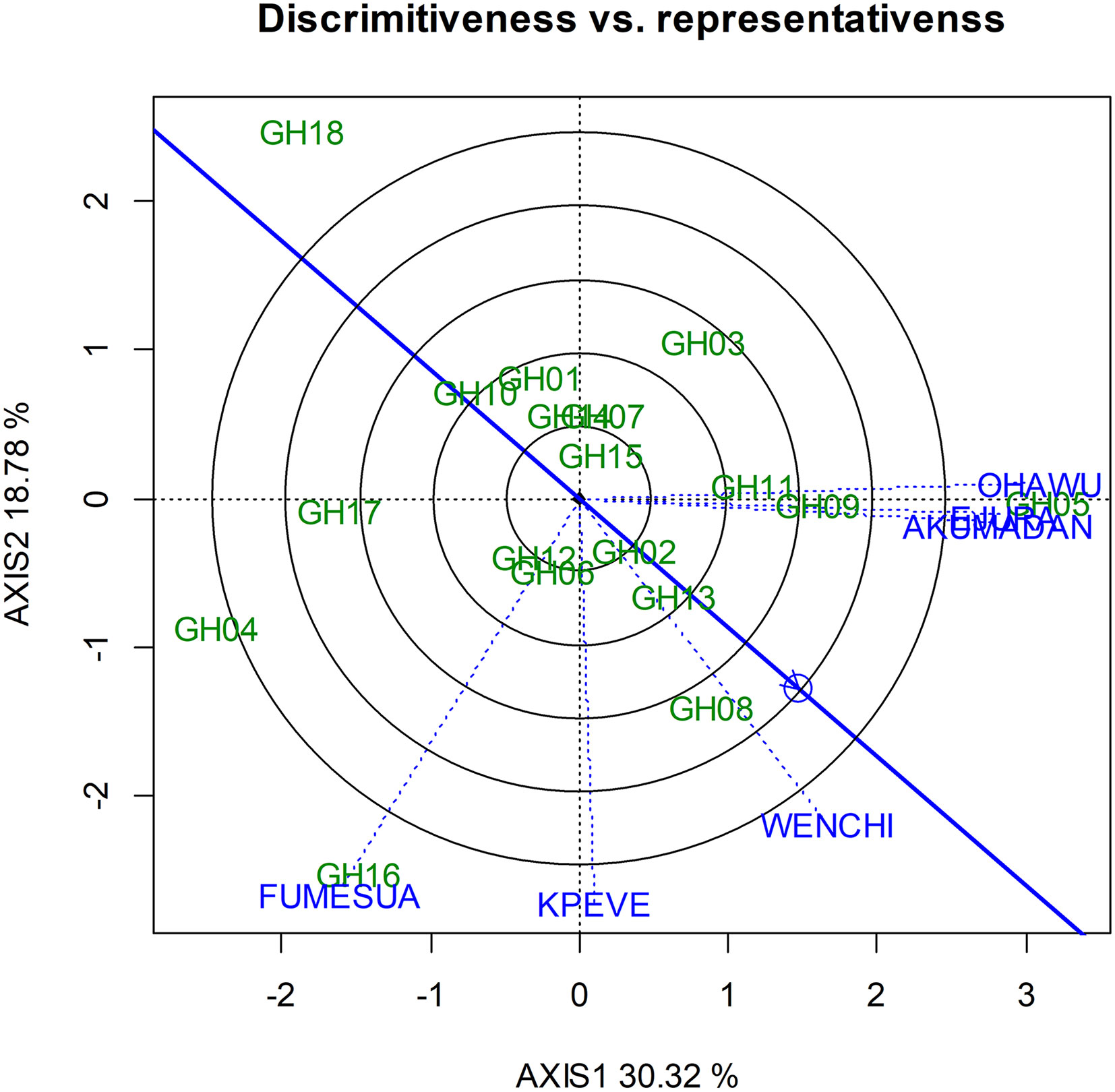
Figure 3 “Discriminating power and representativeness” view of GGE biplot on the hybrids across environments.
GGE Biplot has a unique feature that helps to determine the relationship among tester environments. The cosine of the angle between the lines that join the environments (vectors) to the biplot origin of two environments estimates the correlation between the two environments (Mohammadi et al., 2010). Thus, the smaller the angle between two environments, the more highly correlated the environments are to each other. On this premise, environmental groupings, which showed groupings of environments within the target region where tested plant materials responded similarly, were determined based on the biplot analysis and correlations (Figure 4).
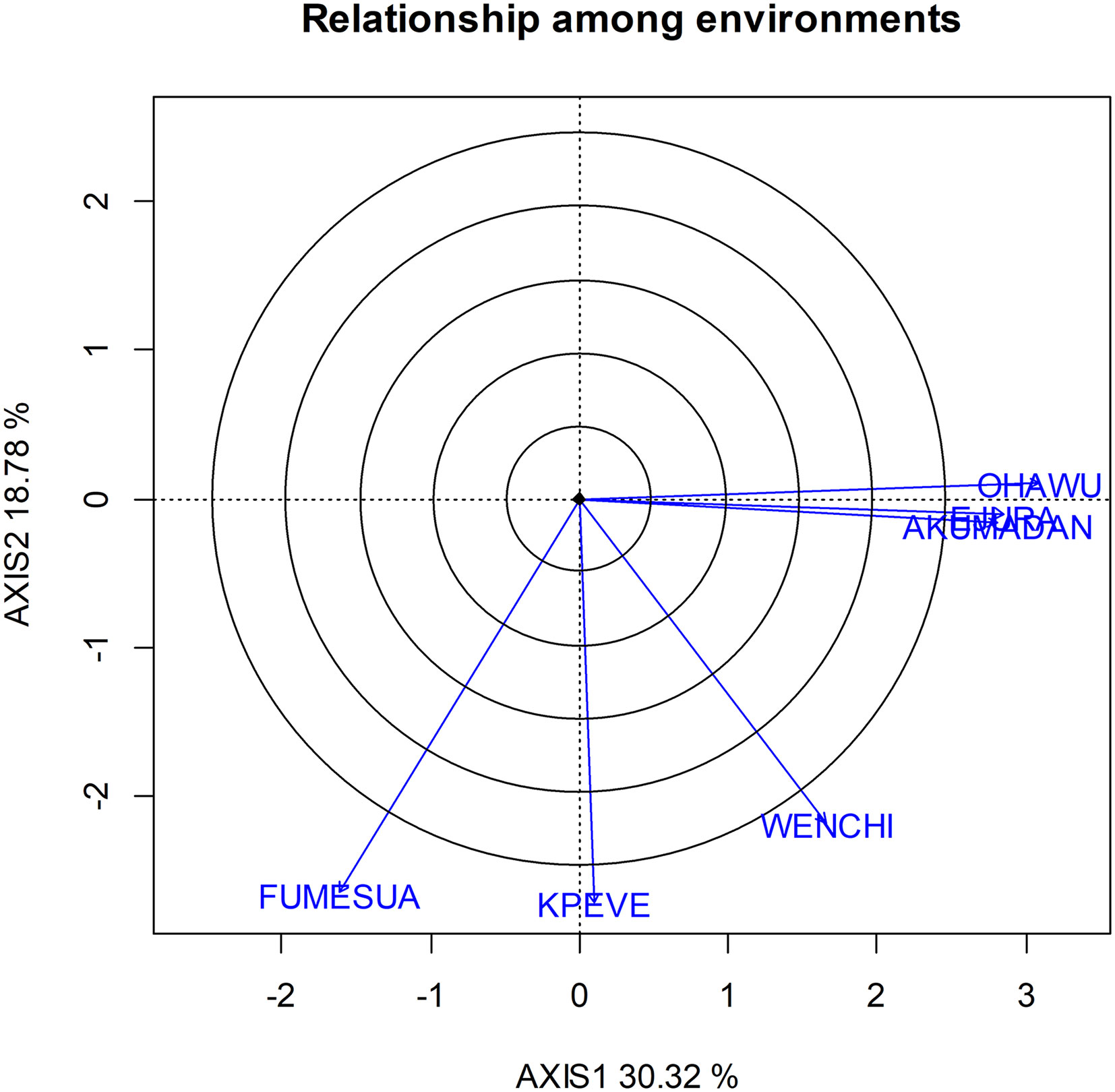
Figure 4 GGE biplot showing relationship among the six testing environments based on the cosine angle between them.
Ejura, Ohawu, and Akumadan environments tend to show high correlation among them and are nearly identical, which suggests that they are similar in their ability to discriminate among genotypes for yield performance. However, the maximum angle formed between the vectors corresponding to Fumesua, Kpeve, and Wenchi is below 90°, which likewise suggests that these three environments also discriminate genotype in a similar manner. Again, between Ohawu, Wenchi and Fumesua formed an obtuse angle suggesting that the three environments tend to be distinctly independent.
Discussion
The objective of this study was to identify genotypes that are high yielding and stable across environments, with good agronomic characteristics, and, most importantly, resistant to aflatoxin accumulation. Warburton and Williams (2014) reported that variability among genotypes could provide novel or favorable alleles for population improvement and the identification of parents for the development of superior hybrids that combine resistance to aflatoxin accumulation and high yields. The observed significant phenotypic variation across seasons and locations among the genotypes for aflatoxin accumulation reduction and other agronomic traits suggest that potential progress could be made in developing well-adapted hybrids with aflatoxin accumulation resistance.
Large environmental effects detected for most of the agronomic traits indicated variability among the genotypes under different environments. The observed no significant genotype by environment interactions across the seasons and environments indicates that the performance of each of the genotypes for the various traits is consistent in the same manner across the various environments. According to Baye et al. (2011), a no significant genotype by environment interaction implies that the performance of the genotypes will not vary much (Sumalini et al., 2020) and that there will be no need for replication at another environment; thus, testing at only one location can help to identify the best hybrids, which should perform equally well in other locations as well. Adu et al. (2013) observed a no significant genotype by environmental interaction for grain yield and other agronomic traits among extra early hybrids in Ghana, which is similar to findings observed in this study. However, Dadzie et al. (2018) reported of significant genotype by environment interaction when he evaluated a larger number of hybrids including these same hybrids across three locations in two seasons in Ghana, which is in contrast with the findings of this work. Because this study involved only the top-performing hybrids identified by Dadzie et al. (2018), it suggests that no significant differences existed among those top-performing hybrids identified by Dadzie et al. (2018), even when evaluated in diverse ecologies as was done in this study.
The assessment of the agronomic traits showed a range of genotypic influence on several parameters studied. Badu-Apraku et al. (2010) classified maize genotypes into extra early, early, intermediate, and late maturity types. However, in this study, the hybrids could clearly be classified as intermediate based on the days to 50% pollen and silking based on the combined analysis. There was no significant difference in the corresponding (ASI), which was all approximately 3 days. This observation is consistent with a report by Ngugi and Ndiema (2013), where ASI for intermediate maize varieties ranges from 3 to 7 days. This finding suggests that the genotypes could be suitable for draught prone ecologies, because Ngugi and Ndiema (2013) reported of ASI to be associated for selection of draught resistant genotypes.
Same assessments of the yield performance of the hybrids revealed that GH 11 (ENT-5 x TZ1-8) and GH06 (ENT-5 x CML-287) were among the top-performing genotypes that were also found in a previous study by Dadzie et al. (2018).
There are scarce reports on well-defined aflatoxin hotspots in Ghana generally. Stable hybrids with potential for aflatoxin accumulation resistance need to be evaluated across contrasting growing areas and across seasons in the country to identify the most stable ones. Stability analysis revealed that GH08 (M0826-12F x CML-343) and GH13 (ENT-70 x CML-247) as the most stable hybrid among the top 5 yielding hybrids, whereas GH10 (ENT-5 x K1-3) was identified as low yielding yet very stable (Choudhary et al., 2019).
Due to the cost involved in aflatoxin analysis, only six hybrids with high grain yields and four local checks were selected for this analysis. Aflatoxin accumulation levels were determined during the minor season, because Henry et al. (2013) reported that aflatoxin accumulation levels increase with increase in draught and heat; this observation agrees with Dadzie et al. (2018) that aflatoxin levels during the major seasons in Ghana were relatively lower across environments than what was observed during the minor season. Hence, testing samples during minor seasons provide an informed decision on the actual potential aflatoxin accumulation. Genotypes such as GH 11 (ENT-5 x TZ1-8), GH08 (MO826-12F x CML-343), and GH06 (ENT-5 x CML-287) were observed to have 0 ppb aflatoxin accumulation in the un-inoculated samples. However, GH11 (ENT-5 x TZ1-8), GH08 (MO826-12F x CML-343), GH05 (ENT-85 x CML-247), and GH09 (MO826-7F x CML-343) all recorded aflatoxin levels, which were below the 20 ppb threshold although under artificial inoculation. This observation compares with the findings from Dadzie et al. (2018) who reported aflatoxin levels of 17.20 ppb, 21.74 ppb for ENT-5 x TZ1-8 and ENT-5 x CML-287, respectively, after inoculation.
Conclusions
The study has identified GH11, GH05, GH08, GH09, GH13, and GH06 as the highest yielding with GH08, GH13 being most stable hybrids and, therefore, can be considered as the most suitable hybrids for cultivation in Ejura, Fumesua, Ohawu, Akumadan, and Kpeve and other locations within the same agroecological zones. Ejura, located in the transition zone, was identified as the ideal testing environment for this set of genotypes. Most of the hybrids showed low levels of aflatoxin accumulation below 20 ppb despite artificial inoculation. The study revealed GH11, GH5, and GH08 as the most stable among the top yielding hybrids with aflatoxin levels of 11.9, 14.3, and 3.8 ppb, respectively, after artificial inoculation. The study has identified some high yielding and stable hybrids as well as diverse hybrid sources that can be considered for cultivation as varieties to help mitigate aflatoxin contamination in maize in Ghana and beyond.
Data availability statement
The original contributions presented in the study are included in the article/Supplementary Material. Further inquiries can be directed to the corresponding author.
Author contributions
AO: did literature search, conceptualized the concept, and wrote manuscript. KAJ: literature research, data collection and analysis. ZA-K: lab work, data collection and analysis. HA-D: contributed to the writing of manuscript, provided guideline and edited manuscript. RA-A: data analysis. All authors contributed to the article and approved the submitted version.
Acknowledgments
The authors are grateful to the USAID through National Academies of Sciences, Engineering Medicine through the programme, Partnership for enhanced engagement in research (PEER) grant no. P 4-040, 2015–2018 for providing the initial grant to start this work. We also acknowledge the contribution of Dr. Marilyn L. Warburton for her immense support and contribution to the success of this work.
Conflict of interest
The authors declare that the research was conducted in the absence of any commercial or financial relationships that could be construed as a potential conflict of interest.
Publisher’s note
All claims expressed in this article are solely those of the authors and do not necessarily represent those of their affiliated organizations, or those of the publisher, the editors and the reviewers. Any product that may be evaluated in this article, or claim that may be made by its manufacturer, is not guaranteed or endorsed by the publisher.
References
Acheampong P. P., Nimo-Wiredu A., Amengor N. E., Nsiah-Frimpong B., Haleegoah J., Adu-Appiah A., et al. (2017). Root and tuber crops technologies adoption and impact study in Ghana: The case of improved cassava technologies. Final Report to WAAPP-GHANA. Accra, Ghana.
Adu G. B., Akromah R., Abdulai M. S., Obeng-Antwi K., Kena A. W., Tengan K. M. L., et al (2013). Assessment of genotype by environment interactions and grain yield performance of extra-early maize (Zea mays L.) hybrids.
Agyare W. A., Asare I. K., Sogbedji J., Clottey V. A. (2015). Challenges to maize fertilization in the forest and transition zones of Ghana. Afr. J. Agric. Res. 9, 6, 593–602. doi: 10.5897/AJAR12.1698
Alcaide-Molina M., Ruiz-Jiménez J., Mata-Granados J. M., de Castro M. L. (2009). High through-put aflatoxin determination in plant material by automated solid-phase extraction on-line coupled to laser-induced fluorescence screening and determination by liquid chromatography–triple quadrupole mass spectrometry. J. Chromatogr. A 1216 (7), 1115–1125. doi: 10.1016/j.chroma.2008.12.049
Atmaca E., Güvenç D., Aksoy A. (2015). Determination of aflatoxin levels in maize grain by high performance liquid chromatography using an immunoaffinity column cleanup. Kafkas Universitesi Veteriner Fakultesi Dergisi 21 (4), 619–622. doi: 10.9775/kvfd.2015.13081
Bandyopadhyay R., Agbetiameh D., Awuah R. T., Atehnkeng J., Ortega-Beltran A., Cotty P. J. (2017). Prevalence of aflatoxin contamination in maize and groundnut in Ghana: Population structure, distribution, and toxigenicity of the causal agents. Plant Dis. 102 (4), 764–772. doi: 10.1094/pdis-05-17-0749-re
Baye T. M., Abebe T., Wilke R. A. (2011). Genotype-environment interactions and their translational implications. Personalized Med. 8 (1), 59–70. doi: 10.2217/pme.10.75
Choudhary M., Kumar B., Kumar P., Guleria S. K., Singh N. K., Khulbe R., et al. (2019). GGE biplot analysis of genotype× environment interaction and identification of mega-environment for baby corn hybrids evaluation in India. Indian J. Genet. Plant Breed. 79(04)658–669. doi: 10.31742/IJGPB.79.4.3
Dadzie A. M., Adu-Gyamfi P. K. K. A., Akpertey A., Ofori S. Y., Opoku J., Yeboah E. G., et al (2018). Assessment of juvenile growth and yield relationship among dwarf cashew types in Ghana. J. Agri. Sci. 12 (10), 116.
EU-European Commission (2018). The rapid alert system for food and feed: 2017 annual report (Geneva: Health and Food Safety, RASFF).
Espinosa-Calderón A., Tadeo-Robledo M., Sierra-Macías M., Turrent-Fernández A., Valdivia-Bernal R., Zamudio-González B. (2009). "Rendimiento de híbridos de maíz bajo diferentes combinaciones de semilla androestéril y fértil en méxico.". Agron. Mesoamerican, 211–216.
FAO (2011). “Situation analysis: improving food safetyin the maize value chain in kenya,” (FAO by Prof. Erastus Kang'e the College of Agriculture and Veterinary Science, University of Nairobi).
Genstat (2007). Genstat for Windows, Discovery. 3rd Ed. (UK: Lawes Agricultural Trust, Rothamsted Experimental Station).
Henry W., Windham G., Rowe D., Blanco M., Murray S., Williams W. (2013). Diallel analysis of diverse maize germplasm lines for resistance to aflatoxin accumulation. Crop Science. 53, 394. doi: 10.2135/cropsci2012.04.0240
Moreno O. J., Kang M. S. (1999). Aflatoxins in maize : The problem and genetic solutions. Plant breeding 188(1):1–16. doi: 10.1046/j.1439-0523.1999.118001001.x
Ministry Of Food and Agriculture (MoFA) (2017). AGRICULTURE IN GHANA. (MoFA) Statistics, Research and Information Directorate (SRID) 20.
Mohammadi R., Haghparast R., Amri A., Ceccarelli S. (2010). Yield stability of rainfed durum wheat and GGE biplot analysis of multi-environment trials. Crop Pasture Sci. 61 (1), 92–101. doi: 10.1071/CP09151
Namjoo M., Salamat F., Rajabli N., Hajihoseeini R., Niknejad F., Kohsar F., et al. (2016). Quantitative determination of aflatoxin by high performance liquid chromatography in wheat silos in golestan province, north of Iran. Iranian J. Public Health 45 (7), 905–10.
Ngugi K., Ndiema G. (2013). Anthesis to silking interval usefulness in developing drought tolerant maize anthesis to silking interval usefulness in developing drought tolerant maize. J. Renewable Agric. 1(5), 84–90. doi: 10.12966/jra.08
Probst C., Njapau H., Cotty P. J. (2007). Outbreak of an acute aflatoxicosis in Kenya in 2004: Identification of the causal agent. Appl. Environ. Microbiol. 73 (8), 2762–2764. doi: 10.1128/AEM.02370-06
Rahman H., Khalil I. H., Islam N., Rafi A. (2007). Comparison of original and selected maize populations for grain yield traits. Sarhad J. Agric. (Pakistan) 23(3):641–644.
Scheiterle L., Birner R. (2016). Comparative advantage and factors affecting maize production in northern Ghana : A policy analysis matrix study No. 310-2016-5480. 2016.
Scott G. E., Zummo N. (1994). Kernel infection and aflatoxin production in maize by aspergillus flavus relative to inoculation and harvest dates. Plant Dis. 78 (2), 123–1125. doi: 10.1094/PD-78-0123
Sirhan A. Y., Tan G. H., Wong R. C. S. (2013). Determination of aflatoxins in food using liquid chromatography coupled with electrospray ionization quadrupole time of flight mass spectrometry (LC-ESI-QTOF-MS/MS). Food Control 31, 35–34. doi: 10.1016/j.foodcont.2012.09.016
Sumalini K., Pradeep T., Sravani D.. G× E interaction studies in relation to heterosis and stability of grain yield in maize (Zea mays L.). Indian Journal Of Genetics And Plant Breeding 80(03):250–60. doi: 10.31742/IJGPB.80.3.3
Warburton M. L., Williams W. P. (2014). Aflatoxin resistance in Maize : What have we learned Lately? Adv. Bot 2014. doi: 10.1155/2014/352831
Windham G.l., Williams P. (2002). Evaluation of corn inbred lines and advanced breeding lines for resistance to aflatoxin contamination in the field. Plant Dis. 86, 232–234. doi: 10.1094/PDIS.2002.86.3.232
Yan W., Cornelius P. L., Crossa J., Hunt L. A. (2001). Two types of GGE biplots for analyzing multi-environment trial data. Crop Sci. 41 (3), 656–663. doi: 10.2135/cropsci2001.413656x
Keywords: maize, yield stability, environment, seasons, hybrids, aflatoxin contamination
Citation: Oppong A, Appiah Jnr K, Appiah-Kubi Z, Adu-Amoah R and Adu-Dapaah H (2022) Mitigating aflatoxin contamination in maize: Breeding for stable resistant cultivars. Front. Hortic. 1:1029804. doi: 10.3389/fhort.2022.1029804
Received: 27 August 2022; Accepted: 17 October 2022;
Published: 25 November 2022.
Edited by:
Emidio Albertini, University of Perugia, ItalyReviewed by:
Akbar Hossain, Bangladesh Wheat and Maize Research Institute, BangladeshMukesh Choudhary, ICAR-Indian Institute of Maize Research, India
Copyright © 2022 Oppong, Appiah Jnr, Appiah-Kubi, Adu-Amoah and Adu-Dapaah. This is an open-access article distributed under the terms of the Creative Commons Attribution License (CC BY). The use, distribution or reproduction in other forums is permitted, provided the original author(s) and the copyright owner(s) are credited and that the original publication in this journal is cited, in accordance with accepted academic practice. No use, distribution or reproduction is permitted which does not comply with these terms.
*Correspondence: Allen Oppong, YWxub3BwQHlhaG9vLmNvLnVr; Hans Adu-Dapaah, aGFkYXBhYWhAeWFob28uY29t