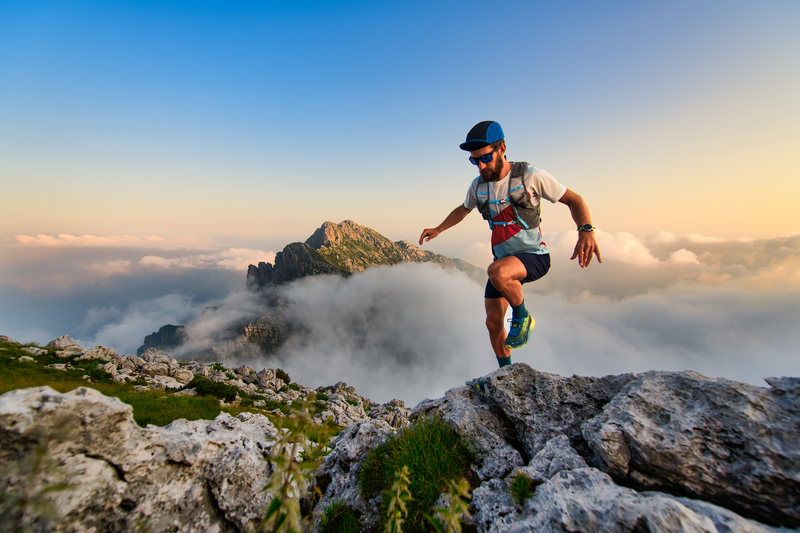
94% of researchers rate our articles as excellent or good
Learn more about the work of our research integrity team to safeguard the quality of each article we publish.
Find out more
ORIGINAL RESEARCH article
Front. Genet.
Sec. Livestock Genomics
Volume 16 - 2025 | doi: 10.3389/fgene.2025.1551967
This article is part of the Research Topic Advances in Livestock Genetics: Enhancing Breeding Practices and Improving Animal Health View all 7 articles
The final, formatted version of the article will be published soon.
You have multiple emails registered with Frontiers:
Please enter your email address:
If you already have an account, please login
You don't have a Frontiers account ? You can register here
Despite high-throughput and large-scale phenotyping becoming easier, interpretation of such data in cattle production remains challenging due to the complex and highly correlated nature of many traits. Underlying biological traits (UBT) of economic importance are defined by a subset of easy-tomeasure traits, leading to challenges in making appropriate selection decisions on them. Research on UBT in beef cattle is limited. In this study, the phenotypic data of admixed beef heifers (n = 336) for reproductive, body conformation, and carcass-related traits (traits, t = 35) were used to identify latent variables from factor analysis (FA) that can be characterized as UBT. Given sample size constraints for carcass (n = 161) and other body size-related traits (n = 336), two models were explored. In Model 1, all individual traits were considered (n = 161), while in Model 2, the dataset was split into body size (n = 336) and carcass (n = 161) traits to maximize available heifers per dataset. A combination of FA and Bayesian network (BN) learning was adopted to develop UBT and infer BN structure for subsequent analyses. All heifers (n = 336) were genotyped using GeneSeek Genomic Profiler 150K for Beef Cattle. Following quality checks, 117,373 autosomal SNP markers were retained and used for genomic estimated breeding values (gEBV) in BN learning steps. Using exploratory and confirmatory FA, Body Size (BS) and Body Composition (BC) were identified as UBT for Model 1, explaining 14 phenotypic traits (t = 14). For Model 2, BS, Ovary Size, and Yield Grade (YG) were identified as UBT, explaining 12 phenotypic traits (t = 12). When using gEBV, the causal network structure inferred showed BS contributed to BC in Model 1 and to Ovary Size in Model 2. Therefore, a structure equation-based approach should be used in subsequent modeling for these traits. From Model 2, YG should be modeled univariately. This study is the first to identify UBT in growing admixed heifers using body size, conformation, and carcass traits. We also identified that BC and YG did not explain intra-muscular fat and body density, indicating these two traits should also be modeled univariately.
Keywords: Bayesian network, Latent phenotypes, multi-trait modeling, Factor analytic models, Heifer development, Phenomics
Received: 26 Dec 2024; Accepted: 27 Feb 2025.
Copyright: © 2025 Anas, Zhao, Yu, Dahlen, Swanson, Ringwall and Hulsman Hanna. This is an open-access article distributed under the terms of the Creative Commons Attribution License (CC BY). The use, distribution or reproduction in other forums is permitted, provided the original author(s) or licensor are credited and that the original publication in this journal is cited, in accordance with accepted academic practice. No use, distribution or reproduction is permitted which does not comply with these terms.
* Correspondence:
Lauren L. Hulsman Hanna, Department of Animal Sciences, North Dakota State University, Fargo, United States
Disclaimer: All claims expressed in this article are solely those of the authors and do not necessarily represent those of their affiliated organizations, or those of the publisher, the editors and the reviewers. Any product that may be evaluated in this article or claim that may be made by its manufacturer is not guaranteed or endorsed by the publisher.
Research integrity at Frontiers
Learn more about the work of our research integrity team to safeguard the quality of each article we publish.