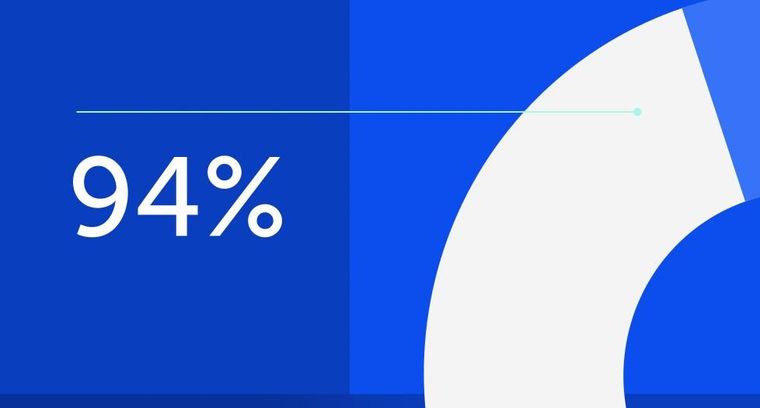
94% of researchers rate our articles as excellent or good
Learn more about the work of our research integrity team to safeguard the quality of each article we publish.
Find out more
ORIGINAL RESEARCH article
Front. Genet., 12 March 2025
Sec. Computational Genomics
Volume 16 - 2025 | https://doi.org/10.3389/fgene.2025.1535279
Accurate prediction of microbe-drug associations is essential for drug development and disease diagnosis. However, existing methods often struggle to capture complex nonlinear relationships, effectively model long-range dependencies, and distinguish subtle similarities between microbes and drugs. To address these challenges, this paper introduces a new model for microbe-drug association prediction, CLMT. The proposed model differs from previous approaches in three key ways. Firstly, unlike conventional GCN-based models, CLMT leverages a Graph Transformer network with an attention mechanism to model high-order dependencies in the microbe-drug interaction graph, enhancing its ability to capture long-range associations. Then, we introduce graph contrastive learning, generating multiple augmented views through node perturbation and edge dropout. By optimizing a contrastive loss, CLMT distinguishes subtle structural variations, making the learned embeddings more robust and generalizable. By integrating multi-view contrastive learning and Transformer-based encoding, CLMT effectively mitigates data sparsity issues, significantly outperforming existing methods. Experimental results on three publicly available datasets demonstrate that CLMT achieves state-of-the-art performance, particularly in handling sparse data and nonlinear microbe-drug interactions, confirming its effectiveness for real-world biomedical applications. On the MDAD, aBiofilm, and Drug Virus datasets, CLMT outperforms the previously best model in terms of Accuracy by 4.3%, 3.5%, and 2.8%, respectively.
The human body hosts trillions of microorganisms, including bacteria, archaea, fungi, protozoa, and viruses, collectively forming the human microbiota, which interacts closely with its host (Gevers et al., 2012; Sommer and Bäckhed, 2013). These microorganisms inhabit various regions such as the skin, oral and nasal cavities, gastrointestinal tract, and genitourinary system, exerting profound effects on health. For instance, they regulate gastrointestinal function, support internal balance, and facilitate metabolic activities (Gill et al., 2006; Ventura et al., 2009). Additionally, the microbiota collaborates with mucosal barriers to prevent pathogen invasion (Macpherson and Harris, 2004). Microbes also contribute to processes like sugar metabolism and vitamin synthesis, both critical for T-cell response (Kau et al., 2011). However, an imbalance in microbial populations, or dysbiosis, can lead to conditions such as diabetes (Wen et al., 2008), inflammatory bowel disease (Durack and Lynch, 2019), and even cancer (Schwabe and Jobin, 2013). Furthermore, pathogens like certain bacteria and viruses are linked to numerous infectious diseases, including pneumococcal pneumonia, with evidence suggesting involvement in up to 27 conditions (Wang D. et al., 2020). The overuse and misuse of medications in recent years have accelerated microbial resistance, creating significant obstacles for clinical treatments and drug development. Microbial metabolism also influences drug efficacy, absorption, and toxicity, highlighting its critical role in pharmacology (Zimmermann and Curtis, 2019; McCoubrey et al., 2023). For example, interactions between intestinal flora and anticancer drugs can alter therapeutic outcomes and side effects. Strategies such as probiotics, prebiotics, synbiotics, biologics, and antibiotics have been proposed to manage microbial populations and enhance treatment effectiveness (Panebianco et al., 2018). Consequently, identifying microbe-drug relationships is a vital challenge in precision medicine, underscoring the urgent need for advanced computational models to explore these interactions.
In recent years, the rise of microbial resistance has paralleled the increasing diversity of drug candidates explored by the medical community (Jiang et al., 2024). Traditional pharmaceutical research often relied on cultivating specific microbial populations under controlled conditions before integrating them into drugs, a process that is both time-intensive and laborious. This challenge underscores the pressing need for advanced computational methods to identify potential microbe-drug relationships, which could revolutionize drug discovery and disease diagnosis (Jiang et al., 2023; Jiang et al., 2025). The advent of bioinformatics has facilitated the establishment of several databases documenting experimentally validated microbe-drug associations, including MDAD (Sun et al., 2018), aBiofilm (Rajput et al., 2018), and DrugVirus (Andersen et al., 2020).
To complement these resources, numerous computational approaches have emerged. For instance, HMDAKATZ, developed by (Zhu et al., 2019), utilizes KATZ metrics within a heterogeneous network to predict microbial-drug correlations. However, its applicability is limited for novel drugs without known microbial associations or isolated microbes lacking disease links. Similarly (Long et al., 2020a), introduced EGATMDA, a graph attention network-based framework with hierarchical attention mechanisms for analyzing microbial-drug interactions. Despite its innovation, this model’s accuracy is constrained by its reliance on pre-existing association data for similarity computation.
Another approach, WHGMF, proposed by Ma and Liu (2022), employs weighted hypergraph learning with generalized matrix decomposition to estimate potential microbe-drug interactions. Yet, it overlooks critical biological details, such as microbial sequences and drug side effect-based similarities, which diminishes prediction accuracy. GCNMDA, introduced by Long et al. (2020a), combines graph convolutional networks and conditional random fields with an attention mechanism to predict microbial-drug associations. Nevertheless, its performance is hindered by noise within extracted similarity features.
Deng et al. (2022) presented Graph2MDA, which uses multimodal attribute graphs and a variogram self-encoder to analyze node-level information and infer potential interactions. In contrast (Tan et al., 2022), proposed GSAMDA, a model integrating graph attention networks with sparse self-encoders to compute microbe-drug correlations. However, GSAMDA struggles with sparse data matrices, limiting its effectiveness. Although these computational models exhibit strengths in certain areas, each faces distinct challenges, emphasizing the need for continued innovation in this field.
In binary relation prediction, selecting appropriate negative samples is critical for effective model training. However, identifying informative negative samples from a pool of candidate negatives remains a significant challenge (Li et al., 2022). This issue is particularly evident in link prediction tasks, where generating meaningful negative samples has long been a persistent problem. Conventional machine learning methods typically classify known associations between entities (labeled samples) as positive samples, while unrecognized or unlabeled associations are treated as candidate negatives (Yang et al., 2012). Yet, due to the scarcity of known microbe-drug associations in publicly available datasets, the imbalance between positive and negative samples becomes a critical issue. To mitigate this imbalance and preserve model performance, advanced negative sampling strategies are essential.
The most widely used approach, random sampling, involves selecting a subset of negative samples equal in number to the positive samples (Lou et al., 2022). While straightforward, this method often fails to prioritize informative negatives and may include irrelevant or noisy examples (López et al., 2013). Efforts to enhance negative sampling strategies (Zeng et al., 2020; Wei et al., 2021; Dai et al., 2022) have achieved limited success, as they do not sufficiently focus on identifying the most valuable negatives critical for effective classifier training. This oversight can result in undertraining and reduced predictive performance.
To address these limitations, we developed a novel microbe-drug association prediction model, CLMT. This model leverages a Graph Transformer network to identify potential associations between graph nodes. It incorporates contrastive learning and employs a four-phase approach with diverse augmented views as positive samples, significantly enhancing prediction accuracy. The key contributions of our work are as follows:
(1) We develop a novel heterogeneous graph-based model that employs a Graph Transformer network to effectively capture complex interactions between microbes and drugs. This allows the model to leverage long-range dependencies within the network structure, surpassing traditional GCN-based methods.
(2) We introduce contrastive learning into microbe-drug association prediction, a technique previously underexplored in this domain. The model generates multiple augmented graph views through node perturbation, treating them as positive samples, while negative samples are selected from different graphs. This contrastive loss mechanism significantly enhances the model’s ability to learn discriminative and generalizable embeddings.
(3) We conduct extensive experiments on three widely used public datasets (MDAD, aBiofilm and Drug Virus), demonstrating that CLMT significantly outperforms state-of-the-art prediction methods. We further validate CLMT’s ability to uncover novel microbe-drug associations through case studies on two common drugs, reinforcing the model’s practical value in biomedical research.
In this study, we used three publicly available datasets for model training and validation: the Microbe-Drug Association Database (MDAD), the aBiofilm database, and the Drug Virus database.
MDAD is a comprehensive resource specializing in known associations between microbes and drugs, integrating data from authoritative sources such as DrugBank, the Human Microbiome Project (HMP), KEGG, and PubChem. Specifically, the MDAD database includes 2,470 clinically or experimentally validated associations between 1,373 drugs and 173 microorganisms. Each association is backed by high-quality data and confirmed through rigorous experimental validation or clinical trials.
The aBiofilm database contains 2,884 associations between 1,720 drugs and 140 microorganisms, focusing on biofilm-associated microbial-drug interactions. It collects a substantial amount of experimental data, particularly on drug-microbe associations related to biofilm formation and inhibition.
The Drug Virus database provides an extensive collection of drug-virus interactions, which are critical for understanding the potential antiviral effects of drugs. This dataset integrates data from multiple biomedical resources, including DrugBank, CTD, and literature-reported associations, and contains over 3,000 drug-virus interactions covering a wide range of viral pathogens. The inclusion of this dataset allows us to assess the model’s ability to handle a broader spectrum of drug-target interactions, particularly in the context of antiviral drug discovery and drug repurposing.
To ensure the reliability of the analyzed results and the biological significance of the associations, we further incorporated drug-disease and microbe/virus-disease association data. The results of the analyses of the MDAD, aBiofilm, and Drug Virus datasets are presented in Table 1.
Consistent with the methodology described by Tan et al. (2022), we implemented the following data screening strategy. First, we selected diseases that were associated with at least one drug and one microorganism in the MDAD dataset. This screening step yielded 109 diseases linked to both drugs and microorganisms. From these, we further extracted 1,121 drug-disease associations and 402 microbe-disease associations.
Similarly, we screened the aBiofilm dataset for diseases associated with at least one drug and one microorganism. This process identified 72 diseases, from which we extracted 435 drug-disease associations and 254 microbe-disease associations.
For the Drug Virus dataset, we applied the same screening criteria, selecting diseases associated with at least one drug and one virus. This step identified 85 diseases, from which we extracted 720 drug-disease associations and 580 virus-disease associations. The inclusion of the Drug Virus dataset allows us to evaluate the model’s performance on a larger and more diverse dataset, particularly in the context of antiviral drug discovery and cross-domain generalization.
By integrating the Drug Virus dataset into our study, we aim to assess the model’s scalability and robustness when applied to a broader range of biomedical problems. Additionally, given the growing need for antiviral drug repurposing—particularly in response to emerging viral diseases—this dataset provides an important benchmark for evaluating the model’s ability to predict drug-virus associations with potential clinical relevance.
Figure 1 shows the detailed architecture of the Graph Contrastive Learning Model with Transformer proposed in this study for Microbe-Drug Associations Prediction (abbreviated as CLMT). The model aims to capture underlying structural relationships in microbe-drug graphs and enhance the robustness and discriminative power of the representation through contrastive learning. The CLMT model consists of four main modules: the Input Microbe-Drug Graph, the Graph Transformer Module, the Graph Contrastive Learning Module, and the Association Prediction Network.
Figure 1. Structural diagram of the model proposed in this study for the microbe-drug association prediction task.
First, the model constructs a heterogeneous graph structure composed of microbes and drugs as input. This graph is then processed by the Graph Transformer Network to capture potential association relationships between the nodes (Yun et al., 2019). Next, the Graph Transformer encoder further refines these association relationships within the microbe-drug graph structure. The model also incorporates contrastive learning (You et al., 2020), generating multiple augmented views of the graph as positive samples, while negative samples are derived from different graphs. By calculating the contrastive loss between the original graph and its augmented views, the model learns a more robust representation. Finally, the Association Prediction Network formalizes this task as a binary classification problem to compute the potential association information between microbes and drugs.
The Microbe-Drug Graph Representation Layer is the base module of the CLMT model. The layer receives raw microbe and drug data, transforms them into heterogeneous graph structures and computes the similarity matrices of drugs and microbes, and finally generates microbe-drug embedding representations. These embeddings representations are used as input vectors for the subsequent graph Transformer learning module.
The initial inputs to this layer are raw microbial data and drug data. First, based on known microbe-drug associations, we construct a heterogeneous network structure by combining drug similarity and microbe similarity networks. We define the microbe-drug neighbor matrix
To calculate the similarity of microbial nodes as well as drug nodes, we introduce exponential similarity. Exponential similarity is a method commonly used to calculate similarity between nodes (Goodall, 1966). Setting
Where
Next, we use Jaccard Similarity (Bag S et al., 2019) to measure the similarity between nodes. Jaccard similarity measures similarity based on the ratio of intersection to concatenation. The Jaccard similarity between drug node pairs is defined as follows:
where
Further, we combine the index similarity of drugs
Similarly, the integrated microbial similarity matrix
Ultimately, we construct graph networks based on these integrated similarity and adjacency matrices:
The graph network constructed in this way
In this study, the Graph Transformer module is the core component, which is designed to capture potential microbe-drug association features by learning the deep representation of nodes in the microbe-drug graph structure.
The graph attention mechanism is the key mechanism of the Graph Transformer module, which allows nodes to dynamically adjust their own representations in microbial-drug networks by taking into account the information of neighboring nodes (Wang X et al., 2019). Specifically, in the first
where
where
where
where
After iterative updating by the multi-layer graph attention mechanism, the feature representation of each node in the final layer of the microbe-drug network
The Graph Contrastive Learning module is designed to enhance the model’s ability to extract informative and discriminative representations of nodes in the heterogeneous microbe-drug interaction network. By leveraging contrastive learning, our model learns to maximize the agreement between positive samples (different augmented views of the same node) while minimizing the similarity with negative samples (nodes from different distributions).
In order to enhance the model’s understanding of the structure of the microbe-drug graph and to improve the generalization ability of the overall model, we employed graph data augmentation techniques to generate multiple augmented views of the original graph, thereby enriching the data sample space for model training. Specifically, we used the node perturbation method to generate augmented graphs (Hiratani N et al., 2022). For each node in the microbe-drug graph structure, we randomly perturbed its feature vector to simulate the variation and uncertainty of node features. Let the node in the original graph
where
The purpose of the node perturbation operation is to introduce enough randomness to increase the diversity of the data and thus help the model learn a representation that is robust to noise and variation in the input data. In the graph contrast learning framework, these augmented views are used as the basis for the generation of positive sample pairs for optimizing the contrast learning process of the model. For the multiple augmented views generated, further inputs are provided to learn the deep feature representation of the nodes in Graph Transformer. For the output of Graph Transformer, the high-dimensional node representations are mapped to a low-dimensional space suitable for comparative learning through Feature Transformation. The goal of Feature Transformation is to reduce the dimensionality of the representations and to enhance their expressive power, typically using a fully connected layer, a process that can be formalized as:
where
After generating augmented views, we apply a contrastive loss function to maximize agreement between the original and augmented representations while ensuring separation from negative samples. Specifically, for each node in the microbe-drug graph structure
where
The loss function optimizes the embeddings such that positive pairs (nodes representing the same entity in different augmentations) are pulled closer together, while negative pairs (nodes from different distributions) are pushed apart.
The graph contrast learning module combines graph data enhancement and unsupervised contrast learning ideas to effectively optimize the representation learning process of microbial-drug graphs, and experimental results show that the module can improve the model’s prediction accuracy and generalization ability of microbial-drug associations.
In the association prediction layer, we will utilize the microbial and drug graph structure representations obtained from the prelude steps for association prediction. Since the output of the model is still the node representations learned by Graph Transformer and Graph Comparison Learning Module, we first map these high-dimensional node representations to the final association prediction results. Specifically, we reduce the set of nodes by a linear transformation
where
Where.
At this point, we complete the inference process of the CLMT model, and the pseudo-code corresponding to this process is shown in Figure 2. In order to measure the difference between the predicted and true values of the model, we use the cross-entropy loss function to evaluate the effect of microbe-drug association prediction (Mao A et al., 2023). The cross-entropy loss function is a commonly used loss function in classification problems, and in microbe-drug association prediction, we modeled the problem as a binary classification task, i.e., predicting whether a certain pair of microbes and drugs are associated. The cross-entropy loss function is defined as follows:
where
To prevent model overfitting, we add a regularization term to the loss function. The regularization term improves the generalization ability of the model by adding a penalty to the model complexity in the loss function, encouraging the model to choose simpler parameter configurations (Kukačka J et al., 2017). In the CLMT model, we use L2 regularization, i.e., weight decay. It is defined as follows:
Where
Ultimately, the combined loss function of the CLMT model consists of an unsupervised graph-contrast learning loss, a cross-entropy loss, and a regularization term of the following form:
This comprehensive loss function optimizes the node representation in the microbe-drug graph structure on the one hand, and takes into account the accuracy of the model prediction and the complexity of the model to ensure that the model not only can accurately fit the training data during the training process, but also has good generalization ability.
This section provides a comprehensive description of the experimental setup, evaluation metrics, and baseline methods used to assess the performance of the CLMT model. We also present the results of the experiments along with a detailed analysis. The effectiveness and superiority of the CLMT model in predicting microbe-disease associations are demonstrated through comparisons with several baseline methods. Additionally, Figure 2 presents the corresponding pseudo-code of the CLMT model.
In this study, we extracted drug features, microbial characteristics, and microbe-drug association matrices from the MDAD and aBiofilm databases. These feature matrices were subsequently used to construct heterogeneous networks that represent the interactions between drugs and microbes.
For the CLMT model, we set the number of epochs to 1,000 and the learning rate for the optimization algorithm to 0.001. The Graph Transformer model was configured with 3 layers, while the Multihead Self-Attention module contained 6 heads. Specifically, we tested different configurations of the Graph Transformer layer (ranging from 1 to 5 layers) and found that using 3 layers achieved the best balance between model complexity and performance. A smaller number of layers (e.g., 1 or 2) led to insufficient representation learning, while a larger snumber of layers (e.g., 4 or 5) caused overfitting and increased computational costs without significant performance improvements. For the multi-head attention mechanism, we experimented with different head numbers (ranging from 2 to 8). We found that 6 heads provided the most effective feature aggregation, allowing the model to capture diverse interaction patterns between microbes and drugs. Using fewer heads (e.g., 2 or 4) limited the model’s ability to focus on multiple aspects of the relationships, while using more heads (e.g., 8) led to increased computational overhead without notable gains in predictive accuracy. In the contrastive learning module, we set the temperature parameter
To improve model generalization, we incorporated a stochastic deactivation strategy in the association prediction module of the linear layer, with a dropout rate of 50%.
The model was trained using the Adam optimizer with a weight decay prevent overfitting. We applied an early stopping criterion with a patience of 20 epochs, monitoring the validation loss to avoid unnecessary training cycles. During both training and evaluation, we performed multiple rounds of cross-validation. Specifically, 5-fold cross-validation was applied, where the dataset was randomly split into five subsets. In each fold, one subset was used as the test set, and the remaining four were used for training. To ensure the reliability and robustness of the results, the entire experiment was repeated five times, and the average performance metrics were reported. All experiments were conducted on a NVIDIA 2080Ti GPU (11GB VRAM). The GPU acceleration significantly improved the efficiency of graph-based operations, particularly in the Graph Transformer module and contrastive learning calculations.
In order to evaluate the methodology proposed in this paper, we employ a series of evaluation metrics to comprehensively measure the performance of the model, including AUC, AUPR and Accuracy. The following are the formal definitions and calculations of each evaluation metric:
AUC (Area Under the ROC Curve) represents the area under the receiver operating characteristic curve (ROC Curve), which is used to measure the classification performance of the model. The ROC Curve plots the True Positive Rate (TPR) and False Positive Rate (FPR) through different thresholds. TPR and FPR are defined as follows:
where
AUPR (Area Under the Precision-Recall Curve) denotes the area under the Precision-Recall Curve, which is used to measure the classification performance of the model on unbalanced datasets. The Precision-Recall Curve plots Precision and Recall through different thresholds. Precision and Recall are defined as follows:
where
In addition to AUC and AUPR, we also report Accuracy as a standard evaluation metric to measure the overall correctness of the model’s predictions. Accuracy is defined as:
Accuracy provides a simple and intuitive measure of the model’s classification ability. It is useful when the dataset is relatively balanced, as it evaluates both positive and negative class predictions equally.
To evaluate the performance of our proposed method, we compared it with five existing microbe-drug association prediction approaches. A brief overview of each method and their limitations is provided below:
HMDAKATZ (Zhu et al., 2019): This method predicts microbe-drug associations using the KATZ metric. However, it primarily relies on traditional graph metrics, which are unable to capture complex long-range dependencies. It also fails to account for important biological features of microbes and drugs, such as drug side effects, which limits its applicability to novel drugs or microbes with unknown associations.
GCNMDA (Long et al., 2020b): This method is based on Graph Convolutional Networks (GCNs) and conditional random fields to predict associations between microbes and drugs. While GCNs can capture local interactions, they struggle to model complex heterogeneous network structures and long-range dependencies, which affects their performance in handling noisy data and unknown associations.
GSAMDA (Tan et al., 2022): GSAMDA uses graph attention networks and sparse autoencoders to model both topological and attribute features within a microbe-drug heterogeneous network. However, its performance is limited by data sparsity, especially when there is insufficient labeled data, and it does not adequately model the intricate biological interactions between microbes and drugs.
LAGCN (Yu et al., 2021): LAGCN applies graph convolution to learn drug and disease embeddings, using an attentional mechanism to integrate embeddings from multiple layers for drug-disease association prediction. However, it is optimized for drug-disease predictions and does not specifically target microbe-drug associations, limiting its effectiveness for the task at hand.
NTSHMDA (Luo and Long, 2018): This method uses an improved randomized roaming algorithm to infer microbe-disease associations by integrating topological similarities within a microbe-drug network. However, it overlooks important biological features such as microbial genome information and drug side effects, which reduces its predictive power, especially for microbe-drug interactions.
These methods were evaluated on the MDAD, aBiofilm and Drug Virus datasets, using their default configurations and tuning their hyperparameters. All methods underwent 5-fold cross-validation, with known microbe-drug associations serving as positive samples and randomly generated negative samples for the training and test sets. To minimize sampling bias, each comparison was repeated five times, and the final AUC score was reported as the average of these iterations.
In contrast to these methods, our CLMT model introduces several innovations:
1. Graph Transformer Network: CLMT uses a Graph Transformer network to capture complex, long-range dependencies within the microbe-drug interaction network, surpassing the limitations of GCN-based approaches.
2. Contrastive Learning: By leveraging contrastive learning and generating multiple augmented views of the graph, CLMT significantly improves the model’s ability to learn discriminative and generalizable embeddings, even with sparse data.
3. Prediction of Novel Interactions: CLMT excels at predicting not only known associations but also novel microbe-drug interactions, making it more versatile and applicable in real-world scenarios where data may be limited or incomplete.
Our extensive experiments on the MDAD, aBiofilm and Drug Virus datasets demonstrate that CLMT outperforms these existing methods, offering superior predictive accuracy and uncovering novel microbe-drug associations with greater reliability.
Tables 2, 3 present the AUC, AUPR, and Accuracy scores of the CLMT model proposed in this paper, along with those of the compared methods on the MDAD and aBiofilm datasets. As shown in the tables, the CLMT method achieved the highest AUC (0.9735 and 0.9742), AUPR (0.9720 and 0.9714), and Accuracy (0.9045 and 0.9121) scores on both datasets, significantly outperforming the other five methods.
First, several comparative methods have demonstrated effectiveness in microbe-disease association tasks. For instance, the GSAMDA model, which utilizes graph attention networks and sparse autoencoders, achieved AUC scores of 0.9460 and 0.8955, and AUPR scores of 0.9223 and 0.9073 on the MDAD and aBiofilm datasets, respectively. These results indicate that GSAMDA effectively captures the topological and attribute features of nodes in the newly constructed microbial-drug heterogeneous network. Specifically, when dealing with graph data involving complex relationships, the graph attention network (GAT) can effectively focus on important node features through the attention mechanism, while the sparse autoencoder (SAE) can capture the data’s sparse structure. These characteristics enable GSAMDA to perform well in this task, demonstrating the feasibility of using graph neural networks and autoencoders for microbe-drug association prediction.
However, despite the satisfactory performance of many methods on this task, their AUC, AUPR, and Accuracy metrics still have room for improvement. Taking the GSAMDA model as an example, its AUC on the aBiofilm dataset is 0.8955, and its AUPR is 0.9073, which represents a gap of 5.05% and 1.5%, respectively, compared to its performance on the MDAD dataset. This gap suggests that GSAMDA has limitations, particularly when handling different datasets, indicating its potential shortcomings in capturing features and modeling relationships. Therefore, the microbe-drug association prediction task requires further exploration, and more powerful and robust methods are necessary to enhance prediction performance.
In comparison, the CLMT method proposed in this paper significantly outperforms all other methods on both datasets. The three evaluation metrics on the MDAD dataset are 0.9735, 0.9720, and 0.9045, respectively, while on the aBiofilm dataset, the corresponding metrics are 0.9742, 0.9714, and 0.9121. We attribute this superior performance to the unique model structure and design principles of CLMT.
To further verify whether the experimental results of CLMT are statistically significant, we conducted a statistical analysis on the AUC scores from 5-fold cross-validation and compared them with a baseline method. Since some existing methods do not have publicly available implementations, we reproduced GSAMDA, one of the best-performing models on the MDAD dataset, as a comparison model and computed the p-value to assess whether CLMT provides a statistically significant improvement. On the MDAD test set, the AUC scores from 5-fold cross-validation for GSAMDA were [0.9497, 0.9277, 0.9389, 0.9539, 0.9581], while our proposed CLMT achieved [0.9735, 0.9730, 0.9737, 0.9748, 0.9729] under the same conditions. To quantify whether the performance gain of CLMT over GSAMDA is statistically significant, we applied a paired t-test, obtaining a p-value of 0.0010 (p < 0.05). This result confirms that the improvement of CLMT over GSAMDA is not due to random variations but represents a statistically significant performance enhancement driven by the methodological improvements introduced in CLMT.
CLMT employs data enhancement techniques such as node perturbation, which enriches the training data by generating a multi-view graph structure. This technique helps the model better learn the diversity of nodes and edges within the graph, thereby improving its generalization ability. More importantly, in the graph contrastive learning module, CLMT utilizes a projection head to map the node representations output by the graph encoder to a space suitable for contrastive learning. By calculating the contrastive loss, this mechanism maximizes the consistency between different views of the same graph structure and minimizes the similarity between different graph structures. This contrastive learning mechanism effectively enhances the model’s ability to capture graph structure features, enabling it to make more accurate association predictions when faced with different graph structures.
Additionally, CLMT incorporates a Transformer model based on a multi-head self-attention mechanism within the graph encoder. This approach enhances the model’s representational capacity by capturing various relationships and feature interactions between nodes through multiple attention heads. The multi-head self-attention mechanism not only focuses on globally important features but also mitigates the overfitting problem that can arise from relying on a single attention head.
Table 4 presents the AUC, AUPR, and Accuracy scores of the CLMT model proposed in this paper, along with those of the compared methods on the Drug Virus dataset. As shown in the table, the CLMT method achieved the highest AUC (0.9727), AUPR (0.9699), and Accuracy (0.9235), significantly outperforming the other five methods.
Despite the reasonable performance of many existing methods, their AUC, AUPR, and Accuracy metrics still have room for improvement. For example, the GSAMDA model, which utilizes graph attention networks and sparse autoencoders, achieved an AUC of 0.8754 and an AUPR of 0.8868 on the Drug Virus dataset. While GSAMDA successfully captures node attributes and sparse structures, its performance lags behind that of CLMT, highlighting potential limitations in generalizing to diverse datasets. Similarly, the HMDAKATZ model, based on heterogeneous graph diffusion, showed the lowest performance, with an AUC of 0.8523 and an Accuracy of 0.7245, indicating its struggles in capturing complex relationships in Virus-drug interactions.
In comparison, the CLMT method proposed in this paper significantly outperforms all other methods across all evaluation metrics. The three evaluation metrics on the Drug Virus dataset are 0.9727, 0.9699, and 0.9235, respectively. We attribute this superior performance to the unique model structure and design principles of CLMT.
Overall, the results on the Drug Virus dataset further validate the effectiveness of CLMT in microbial-drug association prediction. By leveraging contrastive learning, self-attention mechanisms, and data augmentation techniques, CLMT demonstrates superior adaptability and generalization capabilities, setting a new benchmark for future research in this domain.
To further validate the effectiveness of the individual modules in our proposed CLMT method, we conducted ablation experiments on the MDAD, aBiofilm, and DrugVirus datasets. The results are shown in Table 5 and Figure 3.
Figure 3. Performance comparison of CLMT, CLMT-CL, CLMT-Transformer, CLMT-node perturbation on MDAD, aBiofilm, and DrugVirus datasets.
When the Graph Contrastive Learning Module was removed, the model exhibited consistent performance degradation across all datasets. Specifically, the AUC decreased from 0.9735 to 0.9629 on MDAD, 0.9742 to 0.9576 on aBiofilm, and 0.9727 to 0.9514 on DrugVirus. These results highlight the critical role of contrastive learning in enhancing the model’s discriminative ability by maximizing consistency between augmented graph views. The significant performance drop (average 1.9% across datasets) underscores its contribution to generalization. Additionally, to qualitatively analyze the effectiveness of the contrastive learning module, we present the embedding distribution of the MDAD dataset’s test data. Specifically, we obtained the high-dimensional embeddings of the test data both “before” and “after” contrastive learning, performed clustering, and visualized the results using t-SNE, as shown in Figure 4. The left image shows the embeddings before contrastive learning, where clusters are present but may overlap due to large variance. The right image shows the embeddings after contrastive learning, where the clusters are more compact and distinctly separated, indicating improved feature discrimination.
Removing the Graph Transformer Module led to the most pronounced performance decline, with AUC values dropping to 0.9481 (MDAD), 0.9482 (aBiofilm), and 0.9493 (DrugVirus). This demonstrates the Transformer’s irreplaceable capability in modeling complex global dependencies and feature interactions within the graph structure. The multi-head self-attention mechanism effectively captures long-range relationships, which is particularly crucial for sparse biological networks like DrugVirus.
Replacing node perturbation with random edge deletion caused minor but consistent performance degradation across all datasets: AUC decreased to 0.9727 (MDAD), 0.9719 (aBiofilm), and 0.9701 (DrugVirus). While edge deletion remains a viable augmentation strategy, node perturbation’s superior performance (average 0.3% improvement) suggests its advantage in preserving critical structural information during view generation. This effect is especially notable on DrugVirus, where biological interaction sparsity demands more nuanced augmentation.
The ablation experiments confirm that each module uniquely enhances CLMT’s performance:Contrastive learning mitigates overfitting through view invariance. Graph Transformer enables global relational reasoning. Node perturbation optimizes augmentation for biological graph characteristics. Their combined effect achieves state-of-the-art AUC values (>0.97 on all datasets), validating CLMT’s robustness in diverse microbe-drug-virus association prediction scenarios.
In this case study, we aimed to validate the practical effectiveness of the CLMT model in identifying new microbe-drug associations by selecting three commonly used drugs-Cloxacillin, Carvacrol, and Ciprofloxacin-and the microorganism Mycobacterium tuberculosis from the MDAD dataset. For each drug, we cross-checked the top 20 predicted microorganisms by searching for their synonyms in the MeSH and DrugBank databases. Additionally, we verified whether the predicted microbe-drug associations had been reported in the scientific literature through PubMed searches.
Cloxacillin, a semi-synthetic penicillin antibiotic, is widely used to treat infections caused by beta-hemolytic streptococci, pneumococci, and staphylococci (Grillo et al., 2023). It is particularly effective against penicillinase-producing strains of Staphylococcus aureus and Staphylococcus epidermidis, which are resistant to other antibiotics (Aldman et al., 2022). Research has demonstrated that cloxacillin inhibits up to 50% of the activity of S. aureus, S. haematobium, and Salmonella typhi (Orogade and Akuse, 2004). In our study, 15 of the top 20 microorganisms predicted to be associated with cloxacillin (75%) were confirmed in the literature, as shown in Table 6.
Carvacrol, a naturally occurring phenolic monoterpene found in aromatic plants, has demonstrated a wide range of bioactivities in both in vivo and in vitro studies. These include antioxidant (Churklam et al., 2020), diabetes prevention (Arkali et al., 2021), hepatoprotective (Elbe et al., 2020), reproductive (Saghrouchni et al., 2023), antimicrobial, and immunomodulatory properties (Chraibi et al., 2020). Additionally, carvacrol is used as a food preservative due to its flavoring properties (Patel, 2015). Previous research has highlighted its association with various microorganisms. For instance (Abdelhamid and Yousef, 2021), described how carvacrol counteracts desiccation-resistant Salmonella nacionalis, suggesting its potential as an additive against desiccation-adapted Enterococcus faecalis in low-moisture foods (Javed et al., 2021). demonstrated that carvacrol and its metabolites have beneficial effects on immune dysfunction and infection related to COVID-19. Moreover (Wang Y. et al., 2020), found that carvacrol reduced biofilm formation and extracellular polysaccharide secretion by Pseudomonas fluorescens and S. aureus, without affecting cell viability. Of the top 20 microorganisms predicted to be associated with carvacrol, 17 were confirmed by the literature, as shown in Table 7.
Ciprofloxacin, a fluoroquinolone antibiotic, is widely used for treating a variety of infections, including pneumonia, typhoid fever, and skin and soft tissue infections (McCurdy et al., 2017). Numerous studies have confirmed its effectiveness against various human microorganisms. For example (Rehaman et al., 2019), demonstrated ciprofloxacin’s efficacy against Pseudomonas aeruginosa, an opportunistic pathogen (Liu et al., 2021). reported reduced lung inflammation in pneumonia patients treated with ciprofloxacin, while (Trinh et al., 2017) found that combining ciprofloxacin with ceftriaxone provided the most effective treatment for foodborne Vibrio traumaticus. In our study, all 20 of the top microorganisms predicted to be associated with ciprofloxacin were validated by the literature, as shown in Table 8.
In addition, M. tuberculosis was selected for our case study. This Gram-positive, aerobic bacterium is the causative agent of tuberculosis, one of the deadliest diseases worldwide. According to the 2019 Global Tuberculosis Report (WHO Global, 2019), tuberculosis resulted in 1.5 million deaths in 2018. As shown in Table 9, 17 of the top 20 predicted drugs for M. tuberculosis have been supported by prior studies. This underscores the CLMT model’s strong predictive ability in case studies involving drugs and microorganisms.
Table 9. The top 20 Mycobacterium tuberculosis-associated drugs predicted by CLMT and the related publications.
The microbe-drug association prediction task seeks to identify potential associations between microbes and drugs, which can support drug development and disease treatment. In this study, we propose the CLMT model for this task. The CLMT model improves learning capabilities by integrating a Graph Transformer network with contrastive learning techniques. Specifically, we utilize a multilayer Graph Convolutional Network (GCN) to capture the complex relationships between microbes and drugs. The contrastive learning module further enhances the model’s discriminative ability, thereby improving prediction accuracy.
By effectively modeling complex interactions and overcoming data sparsity, CLMT can serve as a valuable tool in early-stage drug screening, ultimately reducing experimental costs and speeding up the development pipeline. Its robust performance on public datasets suggests that CLMT has the potential to be integrated into clinical decision-making frameworks, offering insights that could lead to more personalized and effective treatment strategies. The findings of this study have notable biological implications. By elucidating previously unknown associations between microbes and drugs, CLMT can contribute to a deeper understanding of the molecular mechanisms underlying drug efficacy and resistance. These insights are particularly relevant in the context of rising antimicrobial resistance and the need for precision medicine. Furthermore, the ability of CLMT to highlight subtle, yet biologically meaningful patterns in microbe-drug interactions may inform future research on microbial metabolism, host-microbe interactions, and the role of the microbiome in disease progression. In this way, the model not only advances computational methodology but also holds promise for driving novel biological discoveries.
While our experimental results on two publicly available datasets demonstrate the effectiveness of CLMT, it is important to acknowledge several limitations and failure cases. In certain instances, the model’s performance was less robust. For example, in cases where the microbe-drug association data is extremely sparse, CLMT sometimes struggled to capture weaker or less obvious associations. This may be due to insufficient signal in the available data or limitations in the current data augmentation strategy. When the relationships between certain microbes and drugs are subtle or not well-characterized by the provided features, the model occasionally misclassified these associations. This suggests that additional biological information (e.g., gene expression profiles or metabolic pathways) might be needed to fully capture the underlying mechanisms. Although CLMT performs well on the MDAD and aBiofilm datasets, its scalability and effectiveness on larger or more heterogeneous datasets remain to be thoroughly evaluated. Future work is needed to optimize the model structure for such scenarios.
The original contributions presented in the study are publicly available. This data can be found here: GitHub repository, https://github.com/qimou-515/CLMT.
LX: Conceptualization, Data curation, Formal Analysis, Writing–original draft. JW: Conceptualization, Data curation, Formal Analysis, Writing–original draft. LF: Conceptualization, Data curation, Formal Analysis, Writing–original draft. LW: Methodology, Project administration, Resources, Writing–review and editing. XZ: Methodology, Project administration, Resources, Writing–review and editing.
The author(s) declare that financial support was received for the research, authorship, and/or publication of this article. This work was partly sponsored by the National Natural Science Foundation of China (No. 62272064), the Scientific Research Program of Education Department of Hunan Province (23A0514), the Natural Science Foundation of Hunan Province (No. 2023JJ60185), the Natural Science Foundation of Hunan Province Program (2022JJ50138),the Application-oriented Special Disciplines, Double First-Class University Project of Hunan Province (Xiangjiaotong [2018] 469) and the Hunan Provincial Education Department Scientific Research Project (No. 20B080).
The authors declare that the research was conducted in the absence of any commercial or financial relationships that could be construed as a potential conflict of interest.
The author(s) declare that no Generative AI was used in the creation of this manuscript.
All claims expressed in this article are solely those of the authors and do not necessarily represent those of their affiliated organizations, or those of the publisher, the editors and the reviewers. Any product that may be evaluated in this article, or claim that may be made by its manufacturer, is not guaranteed or endorsed by the publisher.
Abdelhamid, A. G., and Yousef, A. E. (2021). Carvacrol and thymol combat desiccation resistance mechanisms in Salmonella enterica serovar Tennessee. Microorganisms 10 (1), 44. doi:10.3390/microorganisms10010044
Aldman, M. H., Kavyani, R., Kahn, F., and Påhlman, L. I. (2022). Treatment outcome with penicillin G or cloxacillin in penicillin-susceptible Staphylococcus aureus bacteraemia: a retrospective cohort study. Int. J. Antimicrob. Agents 59 (4), 106567. doi:10.1016/j.ijantimicag.2022.106567
Andersen, P. I., Ianevski, A., Lysvand, H., Vitkauskiene, A., Oksenych, V., Bjørås, M., et al. (2020). Discovery and development of safe-in-man broad-spectrum antiviral agents. Int. J. Infect. Dis. 93, 268–276. doi:10.1016/j.ijid.2020.02.018
Arkali, G., Aksakal, M., and Kaya, Ş. Ö. (2021). Protective effects of carvacrol against diabetes-induced reproductive damage in male rats: modulation of Nrf2/HO-1 signalling pathway and inhibition of Nf-kB-mediated testicular apoptosis and inflammation. Andrologia 53 (2), e13899. doi:10.1111/and.13899
Bag, S., Kumar, S. K., and Tiwari, M. K. (2019). An efficient recommendation generation using relevant Jaccard similarity. Inf. Sci. 483, 53–64. doi:10.1016/j.ins.2019.01.023
Chraibi, M., Farah, A., Elamin, O., Iraqui, H. M., and Fikri-Benbrahim, K. (2020). Characterization, antioxidant, antimycobacterial, antimicrobial effcts of Moroccan rosemary essential oil, and its synergistic antimicrobial potential with carvacrol. J. Adv. Pharm. Technol. and Res. 11 (1), 25–29. doi:10.4103/japtr.JAPTR_74_19
Churklam, W., Chaturongakul, S., Ngamwongsatit, B., and Aunpad, R. (2020). The mechanisms of action of carvacrol and its synergism with nisin against Listeria monocytogenes on sliced bologna sausage. Food control. 108, 106864. doi:10.1016/j.foodcont.2019.106864
Dai, Q., Wang, Z., Liu, Z., Duan, X., Song, J., and Guo, M. (2022). Predicting miRNA-disease associations using an ensemble learning framework with resampling method. Briefings Bioinforma. 23 (1), bbab543. doi:10.1093/bib/bbab543
Deng, L., Huang, Y., Liu, X., and Liu, H. (2022). Graph2MDA: a multi-modal variational graph embedding model for predicting microbe-drug associations. Bioinformatics 38 (4), 1118–1125. doi:10.1093/bioinformatics/btab792
Durack, J., and Lynch, S. V. (2019). The gut microbiome: relationships with disease and opportunities for therapy. J. Exp. Med. 216 (1), 20–40. doi:10.1084/jem.20180448
Elbe, H., Yigitturk, G., Cavusoglu, T., Baygar, T., Ozgul Onal, M., and Ozturk, F. (2020). Comparison of ultrastructural changes and the anticarcinogenic effects of thymol and carvacrol on ovarian cancer cells: which is more effective? Ultrastruct. Pathol. 44 (2), 193–202. doi:10.1080/01913123.2020.1740366
Gevers, D., Knight, R., Petrosino, J. F., Huang, K., McGuire, A. L., Birren, B. W., et al. (2012). The Human Microbiome Project: a community resource for the healthy human microbiome. PLoS Biol. 10, e1001377. doi:10.1371/journal.pbio.1001377
Gill, S. R., Pop, M., DeBoy, R. T., Eckburg, P. B., Turnbaugh, P. J., Samuel, B. S., et al. (2006). Metagenomic analysis of the human distal gut microbiome. science 312 (5778), 1355–1359. doi:10.1126/science.1124234
Goodall, D. W. (1966). A new similarity index based on probability. Biometrics 22, 882–907. doi:10.2307/2528080
Grillo, S., Pujol, M., Miró, J. M., López-Contreras, J., Euba, G., Gasch, O., et al. (2023). Cloxacillin plus fosfomycin versus cloxacillin alone for methicillin-susceptible Staphylococcus aureus bacteremia: a randomized trial. Nat. Med. 29 (10), 2518–2525. doi:10.1038/s41591-023-02569-0
Hiratani, N., Mehta, Y., Lillicrap, T., and Latham, P. E. (2022). On the stability and scalability of node perturbation learning. Adv. Neural Inf. Process. Syst. 35, 31929–31941.
Javed, H., Meeran, M. F. N., Jha, N. K., and Ojha, S. (2021). Carvacrol, a plant metabolite targeting viral protease (Mpro) and ACE2 in host cells can be a possible candidate for COVID-19. Front. Plant Sci. 11, 2237. doi:10.3389/fpls.2020.601335
Jiang, M., Liu, G., Su, Y., Jin, W., and Zhao, B. (2025). Hierarchical multi-relational graph representation learning for large-scale prediction of drug-drug interactions. IEEE Trans. Big Data, 1–14. doi:10.1109/tbdata.2025.3536924
Jiang, M., Liu, G., Zhao, B., Su, Y., and Jin, W. (2023). Relation-aware subgraph embedding with co-contrastive learning for drug-drug interaction prediction. arXiv Prepr. arXiv:2307.01507.
Jiang, M., Liu, G., Zhao, B., Su, Y., and Jin, W. (2024). Relation-aware graph structure embedding with co-contrastive learning for drug–drug interaction prediction. Neurocomputing 572, 127203. doi:10.1016/j.neucom.2023.127203
Kau, A. L., Ahern, P. P., Griffin, N. W., Goodman, A. L., and Gordon, J. I. (2011). Human nutrition, the gut microbiome and the immune system. Nature 474 (7351), 327–336. doi:10.1038/nature10213
Kukačka, J., Golkov, V., and Cremers, D. (2017). Regularization for deep learning: a taxonomy. arXiv Prepr. arXiv:1710.10686.
Li, F., Dong, S., Leier, A., Han, M., Guo, X., Xu, J., et al. (2022). Positive-unlabeled learning in bioinformatics and computational biology: a brief review. Briefings Bioinforma. 23 (1), bbab461. doi:10.1093/bib/bbab461
Liu, X., Xiang, L., Yin, Y., Li, H., Ma, D., and Qu, Y. (2021). Pneumonia caused by Pseudomonas fluorescens: a case report. BMC Pulm. Med. 21 (1), 212–216. doi:10.1186/s12890-021-01573-9
Long, Y., Wu, M., Kwoh, C. K., Luo, J., and Li, X. (2020a). Predicting human microbe-drug associations via graph convolutional network with conditional random field. Bioinformatics 36 (19), 4918–4927. doi:10.1093/bioinformatics/btaa598
Long, Y., Wu, M., Liu, Y., Kwoh, C. K., Luo, J., and Li, X. (2020b). Ensembling graph attention networks for human microbe-drug association prediction. Bioinformatics 36 (Suppl. ment_2), i779–i786. doi:10.1093/bioinformatics/btaa891
López, V., Fernández, A., García, S., Palade, V., and Herrera, F. (2013). An insight into classification with imbalanced data: empirical results and current trends on using data intrinsic characteristics. Inf. Sci. 250, 113–141. doi:10.1016/j.ins.2013.07.007
Lou, Z., Cheng, Z., Li, H., Teng, Z., Liu, Y., and Tian, Z. (2022). Predicting miRNA-disease associations via learning multimodal networks and fusing mixed neighborhood information. Briefings Bioinforma. 23 (5), bbac159. doi:10.1093/bib/bbac159
Luo, J., and Long, Y. (2018). NTSHMDA: prediction of human microbe-disease association based on random walk by integrating network topological similarity. IEEE/ACM Trans. Comput. Biol. Bioinforma. 17 (4), 1341–1351. doi:10.1109/TCBB.2018.2883041
Ma, Y., and Liu, Q. (2022). Generalized matrix factorization based on weighted hypergraph learning for microbe-drug association prediction. Comput. Biol. Med. 145, 105503. doi:10.1016/j.compbiomed.2022.105503
Macpherson, A. J., and Harris, N. L. (2004). Interactions between commensal intestinal bacteria and the immune system. Nat. Rev. Immunol. 4 (6), 478–485. doi:10.1038/nri1373
Mao, A., Mohri, M., and Zhong, Y. (2023). “Cross-entropy loss functions: theoretical analysis and applications,” in International conference on Machine learning. pmlr, 23803–23828.
McCoubrey, L. E., Favaron, A., Awad, A., Orlu, M., Gaisford, S., and Basit, A. W. (2023). Colonic drug delivery: formulating the next generation of colon-targeted therapeutics. J. Control. Release 353, 1107–1126. doi:10.1016/j.jconrel.2022.12.029
McCurdy, S., Lawrence, L., Quintas, M., Woosley, L., Flamm, R., Tseng, C., et al. (2017). In vitro activity of delafloxacin and microbiological response against fluoroquinolone-susceptible and nonsusceptible Staphylococcus aureus isolates from two phase 3 studies of acute bacterial skin and skin structure infections. Antimicrob. agents Chemother. 61 (9). doi:10.1128/aac.00772-17
Orogade, A. A., and Akuse, R. M. (2004). Changing patterns in sensitivity of causative organisms of septicaemia in children: the need for quinolones. Afr. J. Med. Med. Sci. 33 (1), 69–72.
PubMed Abstract PubMed Abstract PubMed Abstract | Google Scholar
Panebianco, V., Barchetti, G., Simone, G., Del Monte, M., Ciardi, A., Grompone, M. D., et al. (2018). Negative multiparametric magnetic resonance imaging for prostate cancer: what's next? Eur. Eurology 74 (1), 48–54. doi:10.1016/j.eururo.2018.03.007
Patel, S. (2015). Plant essential oils and allied volatile fractions as multifunctional additives in meat and fish-based food products: a review. Food Addit. and Contam. Part A 32 (7), 1049–1064. doi:10.1080/19440049.2015.1040081
Rajput, A., Thakur, A., Sharma, S., and Kumar, M. (2018). aBiofilm: a resource of anti-biofilm agents and their potential implications in targeting antibiotic drug resistance. Nucleic acids Res. 46 (D1), D894-D900–D900. doi:10.1093/nar/gkx1157
Rehman, A., Patrick, W. M., and Lamont, I. L. (2019). Mechanisms of ciprofloxacin resistance in Pseudomonas aeruginosa: new approaches to an old problem. J. Med. Microbiol. 68 (1), 1–10. doi:10.1099/jmm.0.000873
Saghrouchni, H., Barnossi, A. E., Mssillou, I., Lavkor, I., Ay, T., Kara, M., et al. (2023). Potential of carvacrol as plant growth-promotor and green fungicide against fusarium wilt disease of perennial ryegrass. Front. Plant Sci. 14, 973207. doi:10.3389/fpls.2023.973207
Schwabe, R. F., and Jobin, C. (2013). The microbiome and cancer. Nat. Rev. Cancer 13 (11), 800–812. doi:10.1038/nrc3610
Sommer, F., and Bäckhed, F. (2013). The gut microbiota-masters of host development and physiology. Nat. Rev. Microbiol. 11 (4), 227–238. doi:10.1038/nrmicro2974
Sun, Y. Z., Zhang, D. H., Cai, S. B., Ming, Z., Li, J. Q., and Chen, X. (2018). MDAD: a special resource for microbe-drug associations. Front. Cell. Infect. Microbiol. 8, 424. doi:10.3389/fcimb.2018.00424
Tan, Y., Zou, J., Kuang, L., Wang, X., Zeng, B., Zhang, Z., et al. (2022). GSAMDA: a computational model for predicting potential microbe-drug associations based on graph attention network and sparse autoencoder. BMC Bioinforma. 23 (1), 492. doi:10.1186/s12859-022-05053-7
Trinh, S. A., Gavin, H. E., and Satchell, K. J. F. (2017). Efficacy of ceftriaxone, cefepime, doxycycline, ciprofloxacin, and combination therapy for Vibrio vulnificus foodborne septicemia. Antimicrob. agents Chemother. 61 (12). doi:10.1128/aac.01106-17
Ventura, M., O'flaherty, S., Claesson, M. J., Turroni, F., Klaenhammer, T. R., van Sinderen, D., et al. (2009). Genome-scale analyses of health-promoting bacteria: probiogenomics. Nat. Rev. Microbiol. 7 (1), 61–71. doi:10.1038/nrmicro2047
Wang, D., Hu, B., Hu, C., Zhu, F., Liu, X., Zhang, J., et al. (2020). Clinical characteristics of 138 hospitalized patients with 2019 novel coronavirus-infected pneumonia in wuhan, China. jama 323 (11), 1061–1069. doi:10.1001/jama.2020.1585
Wang, X., Ji, H., Shi, C., Wang, B., Ye, Y., Cui, P., et al. (2019). Heterogeneous graph attention network the world wide web conference, 2022–2032.
Wang, Y., Hong, X., Liu, J., Zhu, J., and Chen, J. (2020). Interactions between fish isolates Pseudomonas fluorescens and Staphylococcus aureus in dual-species biofilms and sensitivity to carvacrol. Food Microbiol. 91, 103506. doi:10.1016/j.fm.2020.103506
Wei, H., Xu, Y., and Liu, B. (2021). iPiDi-PUL: identifying Piwi-interacting RNA-disease associations based on positive unlabeled learning. Briefings Bioinforma. 22 (3), bbaa058. doi:10.1093/bib/bbaa058
Wen, L., Ley, R. E., Volchkov, P. Y., Stranges, P. B., Avanesyan, L., Stonebraker, A. C., et al. (2008). Innate immunity and intestinal microbiota in the development of Type 1 diabetes. Nature 455 (7216), 1109–1113. doi:10.1038/nature07336
WHO Global (2019). Tuberculosis report. World Health Organization. Available at: http://www.who.int/tb/publications/global_report/en/.
Yang, P., Li, X. L., Mei, J. P., Kwoh, C. K., and Ng, S. K. (2012). Positive-unlabeled learning for disease gene identification. Bioinformatics 28 (20), 2640–2647. doi:10.1093/bioinformatics/bts504
You, Y., Chen, T., Sui, Y., and Chen, T. (2020). Graph contrastive learning with augmentations. Adv. neural Inf. Process. Syst. 33, 5812–5823.
Yu, Z., Huang, F., Zhao, X., Xiao, W., and Zhang, W. (2021). Predicting drug-disease associations through layer attention graph convolutional network. Briefings Bioinforma. 22 (4), bbaa243. doi:10.1093/bib/bbaa243
Yun, S., Jeong, M., Kim, R., and Kang, J. (2019). Graph transformer networks. Adv. neural Inf. Process. Syst., 32.
Zeng, X., Zhong, Y., Lin, W., and Zou, Q. (2020). Predicting disease-associated circular RNAs using deep forests combined with positive-unlabeled learning methods. Briefings Bioinforma. 21 (4), 1425–1436. doi:10.1093/bib/bbz080
Zhu, L., Duan, G., Yan, C., and Wang, J. (2019). “Prediction of microbe-drug associations based on KATZ measure,” in 2019 IEEE international conference on bioinformatics and biomedicine (BIBM). IEEE, 183–187.
Keywords: microbe-drug association, graph transformer, similarity matrices, contrastive learning, nonlinear relationships, prediction accuracy, graph augmentation
Citation: Xiao L, Wu J, Fan L, Wang L and Zhu X (2025) CLMT: graph contrastive learning model for microbe-drug associations prediction with transformer. Front. Genet. 16:1535279. doi: 10.3389/fgene.2025.1535279
Received: 27 November 2024; Accepted: 21 February 2025;
Published: 12 March 2025.
Edited by:
Andrei Rodin, City of Hope National Medical Center, United StatesReviewed by:
Akanksha Rajput, University of California, San Diego, United StatesCopyright © 2025 Xiao, Wu, Fan, Wang and Zhu. This is an open-access article distributed under the terms of the Creative Commons Attribution License (CC BY). The use, distribution or reproduction in other forums is permitted, provided the original author(s) and the copyright owner(s) are credited and that the original publication in this journal is cited, in accordance with accepted academic practice. No use, distribution or reproduction is permitted which does not comply with these terms.
*Correspondence: Lei Wang, d2FuZ2xlaUB4dHUuZWR1LmNu; Xianyou Zhu, enh5QGh5bnUuZWR1LmNu
Disclaimer: All claims expressed in this article are solely those of the authors and do not necessarily represent those of their affiliated organizations, or those of the publisher, the editors and the reviewers. Any product that may be evaluated in this article or claim that may be made by its manufacturer is not guaranteed or endorsed by the publisher.
Research integrity at Frontiers
Learn more about the work of our research integrity team to safeguard the quality of each article we publish.