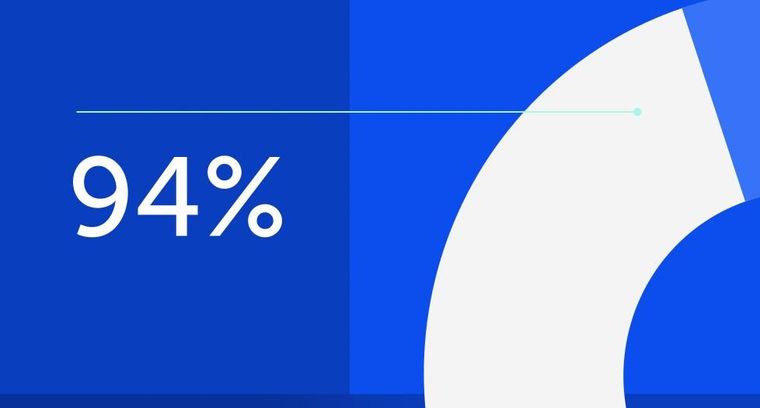
94% of researchers rate our articles as excellent or good
Learn more about the work of our research integrity team to safeguard the quality of each article we publish.
Find out more
ORIGINAL RESEARCH article
Front. Genet., 25 March 2025
Sec. Applied Genetic Epidemiology
Volume 16 - 2025 | https://doi.org/10.3389/fgene.2025.1450259
Background: Epidemiological studies have observed an association between atrial fibrillation (AF) and breast cancer (BC). However, the underlying mechanisms linking these two conditions remain unclear. This study aims to systematically explore the genetic association between AF and BC.
Methods: We utilized the largest available genome-wide association study (GWAS) datasets for European individuals, including summary data for AF (N = 1,030,836) and BC (N = 247,173). Multiple approaches were employed to systematically investigate the genetic relationship between AF and BC from the perspectives of pleiotropy and causality.
Results: Global genetic analysis using LDSC and HDL revealed a genetic correlation between AF and BC (rg = 0.0435, P = 0.039). Mixer predicted genetic overlap between non-MHC regions of the two conditions (n = 125, rg = 0.05). Local genetic analyses using LAVA and GWAS-PW identified 22 regions with potential genetic sharing. Cross-trait meta-analysis by CPASSOC identified one novel pleiotropic SNP and 14 pleiotropic SNPs, which were subsequently annotated. Eight of these SNPs passed Bayesian colocalization tests, including one novel pleiotropic SNP. Further fine-mapping analysis identified a set of causal SNPs for each significant SNP. TWAS analyses using JTI and FOCUS models jointly identified 10 pleiotropic genes. Phenome-wide association study (PheWAS) of novel pleiotropic SNPs identified two eQTLs (PELO, ITGA1). Gene-based PheWAS results showed strong associations with BMI, height, and educational attainment. PCGA methods combining GTEx V8 tissue data and single-cell RNA data identified 16 co-enriched tissue types (including cardiovascular, reproductive, and digestive systems) and 5 cell types (including macrophages and smooth muscle cells). Finally, univariable and multivariable bidirectional Mendelian randomization analyses excluded a causal relationship between AF and BC.
Conclusion: This study systematically investigated the shared genetic overlap between AF and BC. Several pleiotropic SNPs and genes were identified, and co-enriched tissue and cell types were revealed. The findings highlight common mechanisms from a genetic perspective rather than a causal relationship. This study provides new insights into the AF-BC association and suggests potential experimental targets and directions for future research. Additionally, the results underscore the importance of monitoring the potential risk of one disease in patients diagnosed with the other.
Cardiovascular diseases and cancer are the two most common diseases that endanger human health (Siegel et al., 2024; Chen et al., 2022; Schnabel et al., 2015). Among them, breast cancer (BC) is one of the main factors endangering women’s health (Siegel et al., 2024), and atrial fibrillation (AF) is one of the most common cardiovascular diseases, also a significant cause of cardiovascular mortality (Chen et al., 2022; Schnabel et al., 2015). Studies have shown that patients with AF are at an increased risk of developing BC (Boutas et al., 2023; Conen et al., 2016; Guzzetti et al., 2008; Yao et al., 2023). Conversely, BC patients are also at an increased risk of cardiovascular diseases (Guha et al., 2022; Matthews et al., 2021; Gulati and Mulvagh, 2018), with AF being the most common among them (D'Souza et al., 2019). Although common factors such as smoking, alcohol consumption, and inflammation partially explain the association between the two diseases (Giza et al., 2017; Koene et al., 2016), the results of studies are contradictory (Guha et al., 2022; Saliba et al., 2018; Wassertheil-Smoller et al., 2017), and the association between BC and AF remains unclear.
Studies have found that the pharmacological treatment of AF is associated with the occurrence of BC (Su et al., 2013). Conversely, the treatment of BC can also increase the incidence of cardiovascular diseases (Gulati and Mulvagh, 2018). However, this still does not explain the potential biological mechanisms between them. Studies have shown that both AF and BC are influenced by genetic factors (Miyazawa et al., 2023; Moller et al., 2016). By using statistical genetics methods to cross-analyze the two diseases, genetic sharing between the two can be discovered (Zhu et al., 2021). This could provide new insights into epidemiological causal inference and potential biological mechanisms.
In this study, we used large-scale Genome-wide association study (GWAS) summary data to delve into the genetic correlations, common risk loci, and potential functions of genes between AF and BC, which may pave the way for precise treatment strategies for patients with AF and BC.
We obtained GWAS data containing 60,620 cases of AF and 970,216 controls from a meta-analysis that included six studies (Nielsen et al., 2018). We filtered out single nucleotide polymorphisms (SNPs) with a minor allele frequency of less than 1%. After removing SNPs with duplicate rsids and missing data, we acquired a summary dataset consisting of 9,358,555 autosomal SNPs.
BC GWAS data were sourced from the Breast Cancer Association Consortium (BCAC), which included 133,384 cases and 113,789 controls (Zhang et al., 2020). Similarly, we filtered out SNPs with a minor allele frequency of less than 1% and removed SNPs with duplicate rsids and missing data, ultimately obtaining 9,435,343 autosomal summary data.
There was no sample overlap between the two GWAS datasets. Both were aligned with the human reference consortium build 37 and originated from European populations (details in Supplementary Table S1). All GWAS datasets were approved by their respective ethical review boards. The overall study process is shown in the figure (Figure 1).
Figure 1. Flowchart of this study. (A) Heritability analysis using LDSC for two phenotypes: AF and BC. (B) Genetic correlation analysis using LDSC, comparing the genetic correlation between the two diseases. (C) Genetic overlap estimated by MiXeR for AF and BC. (D) PPI network analysis showing key pleiotropic genes, including DNMT3A, related to both diseases. (E) Colocalization analysis of GWAS for both diseases, showing shared genetic variants. (F) Basic assumptions in MR analysis, including assumptions related to IVs, exposure, and outcome. (G) Flowchart summarizing the genetic and causal analysis steps.
We performed global genetic correlation analysis using linkage disequilibrium score regression (LDSC) and high-definition likelihood (HDL) methods (Bulik-Sullivan et al., 2015; Ning et al., 2020). The results of genome-wide genetic correlation (rg) ranged from −1 to 1, indicating negative and positive correlations, respectively. LDSC utilized pre-calculated linkage disequilibrium (LD) scores from the 1000 Genomes Project to analyze SNPs in HapMap 3. HDL used reference data from the HapMap3 SNPs imputed version from the United Kingdom Biobank, utilizing full GWAS data for analysis. Compared to LDSC, HDL provides a smaller error estimate for correlation, enhancing result accuracy (Ning et al., 2020).
We further estimated the local genetic correlation between AF and BC using LAVA and pairwise-GWAS (GWAS-PW) (Pickrell et al., 2016; Werme et al., 2022). GWAS-PW, employing a Bayesian framework, calculated the posterior probabilities of association (PPA) for genomic regions (Pickrell et al., 2016). It used 1,703 pre-partitioned 1000 Genomes Project data as reference. LAVA divided the phase 3 LD panel of the 1,000 Genomes into approximately 1 Mb semi-independent genetic regions, estimating local genetic correlations using significant regions for each phenotype (0.05/2495) (Werme et al., 2022). Segments with PPA>0.5 were also considered locally associated areas. We converted these segments into cytogenetic location using the R package “biomaRt” (version 2.56.1) (Durinck et al., 2009).
We quantified the genetic overlap between AF and BC using the bivariate MiXeR approach. This method assumes that only a portion of the variation affects the trait (Frei et al., 2019). Following official recommendations, we excluded SNPs from the MHC region in both phenotypes due to LD structure (Frei et al., 2019). The results of the bivariate MiXeR were represented using a Venn diagram.
We utilized the cross-phenotype association analysis (CPASSOC) to identify common risk SNPs between AF and BC (Li and Zhu, 2017). CPASSOC, particularly Shet, offers robust statistical power in the presence of heterogeneity. Therefore, this study chose Shet for analysis.
A threshold of 5e-8 represents the widely accepted genome-wide significance level (Sangurdekar et al., 2019; Li et al., 2022). Therefore, in CPASSOC, a P-value < 5e-8 was considered significant for SNPs associated with both phenotypes. PLINK is a widely used open-source toolset for genome-wide association analysis, known for its high computational efficiency and comprehensive genetic analysis capabilities (Purcell et al., 2007). We referenced the 1000 Genomes Project phase 3 data, using Shet in PLINK to identify the most related independent SNPs within a 1.0 Mb area for both trait (Purcell et al., 2007; Byrska-Bishop et al., 2022). The specific parameters and their significance are as follows: SNPs with a P-value ≤5 × 10−8 (–clump-p1) were selected as index SNPs, and SNPs within a ±1 Mb range (–clump-kb 1,000) were searched for with a P-value ≤1 × 10−5 (–clump-p2) and LD r2 ≥ 0.01 (–clump-r2) with the index SNP. These SNPs were grouped into the index SNP’s clump and excluded. Finally, only independent representative SNPs were retained. SNPs with a CPASSOC P < 5e-8 and a single-trait P < 1e-3 were considered pleiotropic. Additionally, SNPs with a P single-trait > 5e-8 and not previously associated with BC or AF were considered new, unreported pleiotropic SNPs (Wu et al., 2023a).
Lastly, we performed functional annotation of identified pleiotropic SNPs using the Ensembl Variant Effect Predictor (VEP) and 3DSNP (Zerbino et al., 2018; Lu et al., 2017). VEP selects candidate genes based on simple physical proximity (Zerbino et al., 2018). 3DSNP annotates the regulatory functions of SNPs by exploring their 3D interactions with genes mediated by chromatin (Lu et al., 2017).
To explore whether the same variants cause the two traits, we employed Coloc for colocalization analysis of pleiotropic SNPs (Giambartolomei et al., 2014). This method provides posterior probabilities for five mutually exclusive hypotheses regarding causal variant sharing in a genomic region. We extracted summary statistics for variants within a 500 kb range of each shared pleiotropic SNP and calculated the posterior probability of PPH4 (the probability of both traits being associated through a shared single causal variant). If PPH4 > 0.75, the locus was considered colocalized. In fine-mapping analysis, PLINK and the 1000 Genomes Project reference panel are required for LD estimation. Additionally, we calculated R2 values for SNPs within a 500 Kb range of each pleiotropic SNP (Purcell et al., 2007; Byrska-Bishop et al., 2022). Using the Bayesian fine-mapping algorithm FM-summary (https://github.com/hailianghuang/FM-summary), we determined a 99% credible set of causal SNPs for each pleiotropic SNP within this range, aiming to provide reliable targets for downstream experiments (Farh et al., 2015).
To identify tissues and cell types closely related to the shared genes, we used the online tool PCGA (https://pmglab.top/pcga) to analyze enrichment in 54 human tissues and cell types from tissues closely associated with AF and BC such as Artery, Blood, Breast, Endothelial cells, Heart, Immune cell, and Immune system (Xue et al., 2022). PCGA employs an iterative estimation framework based on the method of driver tissue estimation by selective expression. It has collected data from 54 types of human tissues via gtxv8 and single-cell RNA data from 2,214 cell types. By integrating GWAS summary statistics and transcriptome data, it effectively estimates related tissue/cell types and genes. Cell enrichment P-values were corrected using False Discovery Rate Correction (FDR).
Considering that genetic variations can influence traits by affecting gene expression, we conducted TWAS to identify overlapping genes potentially having causal relationships. We used Joint-tissue imputation (JTI) models based on multi-tissue transcriptomic data from GTEx v8 comprising 49 tissues (Zhou et al., 2020; Consortium, 2013). This analysis utilized pre-trained expression quantitative trait locus (eQTL) models and GWAS summary statistics. This method accounts for shared genetic effects across different tissues and unique genetic regulation in target tissues. Compared to models like PrediXcan and UTMOST, JTI significantly enhances predictive capability (Zhou et al., 2020). We conducted TWAS using the JTI models for 49 tissues, adjusting p-values within each tissue using FDR.
We employed Fine-mapping of causal gene sets (FOCUS) to further evaluate the significance of genes identified in the TWAS (Mancuso et al., 2019). FOCUS utilizes predicted eQTL weights, LD, and GWAS summary data to estimate potential pathogenic genes from TWAS. It uses a Bayesian algorithm to assess the posterior inclusion probability (PIP) of each feature in the association region. We utilized GTEx v8 eQTL weights from FUSION across 49 tissues (Gusev et al., 2016). Genes with significant p-values in the JTI results and a PIP >0.5 in FOCUS analysis were considered potential pathogenic genes.
We conducted a Phewas of the newly identified pleiotropic SNPs using an online tool (https://gwas.mrcieu.ac.uk/phewas/) with a threshold of P < 5e-8 (Elsworth et al., 2020). Additionally, we performed a Phewas of the genes identified by TWAS using publicly available data from the GWAS Atlas (https://atlas.ctglab.nl) for 4756 phenotypes with a significance threshold of P < 0.05/4756.
To determine if there are interrelationships among the identified pleiotropic genes, we analyzed relevant genes annotated by VEP and 3DSNP, and pleiotropic genes identified through TWAS using STING (https://string-db.org/) (von Mering et al., 2003).
Genetic correlation may be due to pleiotropy or causal relationships (Zhu et al., 2015). Pleiotropy represents the same genetic variation affecting two traits simultaneously. In contrast, causal relationships imply that genetic variation can influence one trait and thereby affect another.
To explore the genetic causal relationship between AF and BC, we designed a Mendelian randomization (MR) analysis based on the STROBE-MR guidelines (Supplementary Materials). We used software packages such as “TwoSampleMR,” “RadialMR,” “CAUSE,” “GSMR,” and “MendelianRandomization” to perform MR analysis on GWAS data. MR analysis relies on three core assumptions (Zheng et al., 2017): (1) the instrumental variables (IVs) is closely associated with the exposure; (2) the IVs is unrelated to confounders; (3) the IVs affects the outcome only through the exposure (Figure 1F).
Initially, we performed univariate MR analysis using seven methods at a threshold of P < 5e-8, including inverse variance weighting (IVW), weighted median, MR-Egger, weighted mode, simple mode, GSMR, CAUSE. Before MR analysis, LD was removed using 1,000 Genomes reference data. Subsequently, we used the GWAS catalog to eliminate SNPs related to known common confounders such as obesity, smoking, and alcohol consumption (Sollis et al., 2023). Sensitivity analyses were then conducted to remove outliers to stabilize results. MR-Egger intercept testing and Cochran’s Q statistic assessed pleiotropy and heterogeneity. Outliers were removed using MR-Presso, radial IVW, and radial Egger. In univariate MR analysis, IVW served as the primary outcome. GSMR analysis considered potential LD among SNPs (Zhu et al., 2018). CAUSE controls for potential pleiotropy, significantly reducing the false-positive rate (Morrison et al., 2020). We included these methods to aid in interpreting results. Finally, a leave-one-out analysis was performed to detect influential SNPs, and statistical power was calculated using mRnd (Brion et al., 2013).
To better manage potential confounders, we performed an additional multivariable MR (MVMR) analysis. This analysis incorporated phenotypes related to obesity from the FinnGen research project (R10) (Kurki et al., 2023). It also included phenotypes for smoking initiation and alcohol consumption from the GWAS and Sequencing Consortium of Alcohol and Nicotine use [GSCAN] study, excluding samples from the United Kingdom Biobank (Liu et al., 2019). MVMR-IVW was the primary outcome, capable of detecting potential outliers and pleiotropy. Additional methods like MVMR-Lasso, MVMR-Egger, and MVMR-Median were used to consolidate results.
In the unrestricted LDSC analysis, the results indicated a potential genetic correlation between AF and BC with rg = 0.0435 and P = 0.0388 (Figure 1B). Although the HDL analysis did not reach a significant threshold, it also showed a positive correlation between AF and BC (rg = 0.0319, P = 0.101, Table 1).
In reference to the results of global genetic analysis, we analyzed the local genetic characteristics between AF and BC. After FDR correction, LAVA identified 11 significant regions (PFDR < 0.05, Supplementary Table S2). GWAS-PW identified 12 significant regions with PPA3 > 0.05 between AF and BC (Supplementary Table S3). Notably, the region near 11p15.5 (chromosome 11: 1,857,846-2,477,449) was consistently validated by both methods (Figure 2).
Figure 2. (A) represents the results of the LAVA analysis, with the X-axis representing genetic correlation and the Y-axis representing the -log10 (PFDR) values. The dashed line represents -log10 (0.05). (B, C) respectively represent the results for AF and BC from the GWAS-PW analysis, with the X-axis showing the maximum absolute value of the Z-score, and the Y-axis displaying data for PPA 3.
In the bivariate MiXer analysis excluding the MHC region, the rg between the two was 0.05. MiXer predicted 348 variants affecting AF and approximately 568 for BC, with about 125 shared variants between them (Figure 1C). The bivariate stratified QQ plot for AF and BC showed a significant left shift in the groups of SNPs with higher significance (Figure 3), strengthening the notion of shared genetic overlap between AF and BC.
Figure 3. (A) A Venn diagram represents the data on the potential pleiotropic genetic overlap between AF and BC from the MiXer results. (B) QQ plots represent the results stratified by P-values for BC and AF. (C) The negative log-likelihood plot shows a minimum model score of about 15, a maximum score of about 110, and the best model score close to 0, indicating a good fit of the model.
Given the observed genetic overlap between AF and BC, we used CPASSOC to explore individual genetic variations. At a genome-wide threshold (P < 5e-8), CPASSOC identified 323 independent SNPs (Supplementary Table S4), including 14 pleiotropic SNPs and 1 new pleiotropic SNP (Table 2). Of the total 15 pleiotropic SNPs, 8 SNPs representing loci were supported by colocalization (PP.H4 > 0.75, N = 8; PP.H4 > 0.9, N = 7), suggesting these loci as key in influencing both traits, reinforcing the causal association between the loci and traits (Supplementary Figures S1–S8). This includes a new pleiotropic SNP, rs114414434, which colocalization results also suggest as a key site affecting both traits (PP.H4 = 0.794, Table 2). At a genome-wide threshold (P < 5e-8), the Phewas results for the newly identified pleiotropic SNP rs114414434 show significant associations with high expression of ENSG00000152684 (P = 2.76e-15) and ENSG00000213949 (P = 2.20e-08) (Supplementary Table S5).
For each pleiotropic SNP, we identified a 99% credible set of causal SNPs within a 500 kb range, totaling 2,035 SNPs related to AF and BC (Supplementary Table S6).
Our findings indicate that AF and BC are jointly enriched in 16 tissues including “Breast - Mammary Tissue,” “Vagina,” “Artery - Tibial,” “Artery - Aorta,” “Artery - Coronary,” “Uterus,” “Heart - Atrial Appendage,” “Colon” (Figure 4A; Supplementary Tables S7–S9). Moreover, cell type enrichment analysis in disease-related tissues such as Artery, Blood, Breast, Endothelial cells, Heart, Immune cell, Immune system showed joint enrichment in Fibroblast, Smooth muscle cell, Endothelial cell, Myofibroblast, Macrophage (Figure 4B; Supplementary Tables S10, S11).
Figure 4. Co-enriched tissues and cell types between atrial fibrillation and breast cancer. Red font indicates co-enrichment. (A): Represents the tissue types. (B): Represents the cell types.
Using JTI models across 49 tissues, we identified 1,276 genes (PFDR < 0.05), reducing to 205 unique genes after accounting for tissue origin (Supplementary Table S12). The FOCUS method identified 61 genes across 49 tissues (PIP >0.5), reducing to 30 unique genes after considering tissue origin (Supplementary Table S13). Of these, 23 genes confirmed by both methods, were reduced to 10 unique genes after removing duplicates (Supplementary Table S14). These included “GMCL1,” “DNMT3A,” “SNRNP27,” “NRBF2,” “FBXO32,” “JMJD1C,” “GTF2IRD2,” “ANXA4,” “RUSC1-AS1,” “GTF2I.”
Phewas associated nine genes (ANXA4, DNMT3A, FBXO32, GMCL1, GTF2I, GTF2IRD2, JMJD1C, NRBF2, SNRNP27) with 459 phenotypes under a threshold of P < 0.05/4756, with the most frequently associated traits being body mass index (N = 23), Height (N = 9), and educational attainment (N = 8) (Supplementary Table S15).
Among the total of 30 relevant genes identified by VEP, 3DSNP annotations, and TWAS, PPI analysis at a confidence score threshold of 0.15 showed that DNMT3A had the most associations with other genes (Figure 1D).
Finally, we predicted the causal relationship between AF and BC from a genetic perspective using two-sample Mendelian randomization. We used 79 A F-related SNPs as IVs to test for potential causal effects of AF on BC (F-statistic from 29.7–510.6, Supplementary Table S16) and 121 BC-related SNPs as IVs for potential causal effects of BC on AF (F-statistic from 29.8-958.4, Supplementary Table S17). The F-statistic >10, avoiding bias from weak IVs (Burgess et al., 2011). Univariate MR results indicated no positive or negative causal relationship between AF and BC (Figure 5A). Results from CAUSE, GSMR, and LOO analyses reinforced this view (Supplementary Figures S10–S15; Supplementary Tables S18, S19). Statistical power is detailed in Supplementary Table S20.
Figure 5. (A) Results of univariate and multivariate Mendelian randomization analyses between AF and BC. This includes 7 methods of univariate MR analysis and 4 methods of multivariate MR analysis. (B) Scatter plot of univariate MR analysis from AF to BC. (C) Funnel plot of univariate MR analysis from AF to BC. (D) Leave-one-out (LOO) plot of univariate MR analysis from AF to BC. (E) Scatter plot of univariate MR analysis from BC to AF. (F) Funnel plot of univariate MR analysis from BC to AF. (G) LOO plot of univariate MR analysis from BC to AF.
In MVMR analysis incorporating major confounders such as obesity, alcohol use, and smoking, we used random effects IVW, which allows for heterogeneity in sensitivity tests (Papadimitriou et al., 2020). By incorporating SNPs associated with relevant confounding factors into the MVMR, the final MVMR results, using 136 SNPs as IVs for AF and 164 SNPs for BC, still showed no positive or negative causal relationship between AF and BC (Figure 5).
In previous epidemiological studies, while a link between AF and BC was observed, the mechanisms underlying this association were unclear. Therefore, we utilized the largest GWAS summary data available for AF and BC to systematically investigate their potential shared genetic structures from both SNP and gene levels. To our knowledge, this is the first comprehensive study to examine the shared genetic architecture between AF and BC. Our results demonstrate significant shared genetics between AF and BC in both global and local genetic analyses. We further analyzed the pleiotropy and causality of these genetic overlaps, ultimately identifying multiple pleiotropic SNPs and genes. Moreover, our findings indicate no causal relationship between AF and BC from a genetic standpoint, which partially explains the epidemiological link observed between these conditions. Our approach from the SNP and gene perspective offers new insights into the association and causality between these conditions.
Previous meta-analysis has elucidated the bidirectional association between AF and BC (Yao et al., 2023). Potential mechanisms include systemic inflammation, with elevated inflammatory markers (such as CRP, TNF-α, and IL-6) in BC patients, and AF sharing pathways with the NLRP-3 inflammasome (Yao et al., 2018; Scott et al., 2019; Ershaid et al., 2019). Furthermore, cancer treatments, such as anthracyclines and trastuzumab, can lead to AF (Leong et al., 2016; Khouri et al., 2012). Conversely, AF medications, such as amiodarone and digoxin, may increase the risk of BC (Fares, 2013; Biggar et al., 2011). However, there is currently a lack of relevant studies from a genetic perspective. Therefore, this study is the first to explore the association between AF and BC from a genetic perspective, providing new insights into the shared pathogenesis of these two diseases, which has important implications for future clinical prevention and treatment strategies.
Our initial analysis using unconstrained intercept LDSC identified a significant overall genetic correlation between AF and BC. To solidify these results and considering no sample overlap, we employed constrained intercept LDSC and HDL methods. Constrained intercept LDSC also indicated significant genetic correlation, and HDL suggested a positive genetic correlation between AF and BC. These findings were corroborated by MiXer (rg = 0.05), which revealed potential genetic overlap outside the MHC region. In local genetic correlation analysis, both LAVA and GWAS-PW highlighted significant regions, notably around 11p15.5 (chr11: 1,857,846-2,477,449). Using the R package “biomaRt,” we identified 27 genes within this segment, including MIR483, LSP1, IGF2, which are associated with the onset and progression of BC (Luo et al., 2021; Hemminki et al., 2010; Cui et al., 2019), and H19, KCNQ1, linked to AF (Chen et al., 2003; Guo et al., 2022).
Considering the pleiotropic genetic variations and genes that can simultaneously affect AF and BC, we initially identified 15 pleiotropic SNPs at the SNP level. Among these 15 pleiotropic SNPs, rs2936870 located near 10q26.13 represented the strongest shared signal (PShet < 5e-324, PP.H4 = 0.932). Multiple studies have reported its close association with BC (Lei and Deng, 2017; Xu et al., 2011). Research has also found that in mouse models, it plays an important role in the recovery of cardiac function and vascular remodeling after myocardial ischemia-reperfusion injury (House et al., 2016). Among other pleiotropic SNPs, rs146518726, rs11205303, rs6440006, rs1973765, rs2936870, and rs35005436 are all located at loci with positive genetic correlations. In the Phewas of the new pleiotropic SNPs, they are shown to be related to the expression of two genes. ENSG00000152684 (PELO) is involved in regulating the ATPase activity of the NOD-like receptor family, controlling its oligomerization assembly and activation, thus participating in the regulation of various immune inflammatory responses mediated by the NLR family (Wu et al., 2023b). Studies related to ENSG00000213949 (ITGA1) have found that elevated levels of ITGA1 are closely associated with cardiac dysfunction in type 2 diabetes (Su et al., 2024) and also linked to the development of various cancers (Boudjadi et al., 2016). However, more detailed functional studies are needed for these pleiotropic sites to help us discover their relationship with AF and BC.
Then, we explored possible pleiotropic genes at the gene level using JTI and FOCUS methods. Among the 10 pleiotropic genes identified by TWAS, ANXA4 is reported to be a key immune-related gene in AF (Yan et al., 2021). Moreover, ANXA4 can activate the JAK-STAT3 signaling pathway by increasing the expression of JAK1 and phosphorylation of STAT3, ultimately affecting the progression of BC (Li et al., 2020). FBXO32 has been reported to be associated with BC risk (Wang et al., 2021). In diabetic mouse models, FBXO32 was found to regulate the expression of small conductance calcium-activated potassium channel 2 (SK2) in the atria, thereby increasing the risk of AF (Ling et al., 2019). Studies have found that silencing of the NRBF2 promoter may be related to the development of breast cancer (Darabi et al., 2015). Additionally, variations within the NRBF2 region have been found to have a significant statistical correlation with the risk of AF in European and East Asian populations (Hong et al., 2021). Changes in the expression of the SNRNP27 gene may play a role in the susceptibility to AF (Hsu et al., 2018). SNRNP27, as one of the potential targets of miR-146b-5p, may play a role in liver inflammation and liver cancer (Kirchmeyer et al., 2018). Mutations in DNMT3A are more common in patients with AF than in individuals without it (Ahn et al., 2024). DNMT3A may promote cardiac fibrosis by silencing RASSF1A, thereby activating the ERK1/2 signaling pathway, which may lead to AF (Tao et al., 2014). In young women receiving BC treatment, DNMT3A is one of the most common mutated genes (Gibson et al., 2023). The PPI results also indicate that DNMT3A could be a key gene between BC and AF. In other genes, such as GTF2I, JMJD1C, RUSC1-AS1, while reported to be associated with the development of BC, they have not been reported in relation to AF (Watanabe et al., 2013; Li et al., 2019; Niinaka et al., 2010). Furthermore, we identified two potential new pleiotropic genes, including GMCL1 and GTF2IRD2. GTF2IRD2 is thought to be derived from the GTF2I sequence (Makeyev et al., 2004), and PPI results reinforce this view. GTF2IRD2 has been reported to play a crucial role in the pathogenesis of Williams-Beuren syndrome (Makeyev et al., 2004). GMCL1 is considered a candidate tumor suppressor gene, and its encoded protein is involved in the control of the MDM2-P53 axis (Masuhara et al., 2003). Abnormal expression of the GMCL1 gene has been found to affect the prognosis of diffuse large B-cell lymphoma (Fournier et al., 2010). In the Phewas of these pleiotropic genes, we found that these genes were most strongly associated with BMI, height, and educational attainment. Furthermore, obesity and height are currently considered independent risk factors for AF and BC (Koene et al., 2016; Aune et al., 2017; Kofler et al., 2017; Gremke et al., 2022), suggesting that the epidemiological observation of a link between AF and BC may be due to risk factors such as obesity. Furthermore, TWAS results and tissue and cell enrichment results show that the shared pathways between AF and BC may extend to multiple systems such as the digestive, reproductive, and circulatory systems. For example, it has been found that gut microbiota can increase the risk of AF or BC (Li et al., 2023; Zhang et al., 2021). In addition, among the 5 cell types enriched in common between AF and BC, macrophages have been reported to participate in tissue regeneration and myocardial injury (Leanca et al., 2022). Resident cardiac macrophages are a subtype of self-renewing cells that can reproduce to protect the myocardium (Sansonetti et al., 2020). In breast development, there is also a resident macrophage subtype (Boutas et al., 2023). Resident macrophages have been found to facilitate the metastatic cascade and growth of tumors (Nalio Ramos et al., 2022). These study results indicate that the relationship between AF and BC may be very complex. The diseases share many common risk genes, which can genetically explain the link between AF and BC. According to the enrichment results, the link between the two can even be traced back to the origins of related tissues and cell types. However, more research is needed to discover the pathophysiological mechanisms between the two.
In the use of genetic tools to predict causal relationships, there is no potential causal link between AF and BC. This result suggests that the potential link between AF and BC may be caused by common risk factors, related treatments for AF or BC, or common pleiotropic genetic outcomes, such as the use of amiodarone, which has been found to be related to an increased risk of cancer (Wassertheil-Smoller et al., 2017; Su et al., 2013). A recent study has shown that young BC patients undergoing breast-conserving surgery have a higher risk of AF, and the use of anthracycline drugs may also be associated with an increased risk of AF (Park et al., 2024). It is noteworthy that these results may mask the potential inherent association between AF and BC, causing us to overlook the inherent link between AF and BC. Our study results indicate that there is some genetic overlap shared between AF and BC. Our study utilizes the largest available AF and BC GWAS summary data to systematically investigate their concurrent relationship, revealing shared genetic pleiotropy rather than a direct causal link. By identifying pleiotropic genes and pathways, our findings suggest potential biological mechanisms that may contribute to the observed epidemiological association. These results provide valuable targets for future research, guiding efforts toward understanding the molecular basis of AF and BC co-occurrence and informing potential risk stratification or therapeutic interventions. Further studies incorporating functional validation and multi-ancestry analyses are warranted to refine these findings and explore their clinical implications.
We acknowledge some limitations in this study. First, our study only uses data from European populations, which does not have universal applicability to other races. Secondly, because the BC population includes only female samples, and due to the limited availability of summary data, we were unable to conduct gender-stratified analysis. Therefore, the distribution of information on the X chromosome is not balanced between the two populations, and most tools used in this study do not support analysis of the X chromosome. Thus, our study did not conduct a more in-depth study of the X chromosome. Third, as this study focuses on the relationship between overall BC and AF throughout the day, it did not further explore the associations between BC subtypes and AF. Finally, more in-depth experimental work and more complete cohort studies are needed to help us better understand the relationship between AF and BC and the pathophysiological mechanisms between them.
Our study provides a genetic correlation analysis between AF and BC, revealing genetic overlaps and identifying 15 pleiotropic SNPs and 10 pleiotropic genes. We further analyzed cross-enriched tissues and cell types and provided evidence from a genetic perspective using MR and MVMR for a non-causal relationship between AF and BC. Our findings enhance understanding of the potential biological mechanisms between AF and BC, which could influence future research aimed at reducing the risks of both conditions.
The original contributions presented in the study are included in the article/Supplementary Material, further inquiries can be directed to the corresponding authors.
YY: Methodology, Visualization, Writing–original draft, Data curation, Software. JC: Investigation, Methodology, Validation, Writing–original draft. XZ: Methodology, Visualization, Writing–original draft. FG: Data curation, Investigation, Resources, Writing–review and editing. RL: Methodology, Resources, Software, Supervision, Writing–review and editing. JM: Investigation, Resources, Software, Supervision, Validation, Writing–review and editing. ML: Resources, Software, Supervision, Writing–review and editing. FG: Conceptualization, Funding acquisition, Investigation, Resources, Writing–review and editing. WC: Funding acquisition, Writing–review and editing.
The author(s) declare that financial support was received for the research, authorship, and/or publication of this article. This study was funded by the National Natural Science Foundation of China (No. 82060538; No. 82060543), the Middle-aged and Young Academic and Technical Leader Reserve Talent Program of Yunnan Province Science and Technology Department (No. 202205AC160008), the 535 Talent Project of the First Affiliated Hospital of Kunming Medical University (No. 2023535D10). The key Project of Yunnan Applied Basic Research (202301AS070061).
We thank BCAC, GWAS CATALOG, and all other institutions and studies that provide open data.
The authors declare that the research was conducted in the absence of any commercial or financial relationships that could be construed as a potential conflict of interest.
All claims expressed in this article are solely those of the authors and do not necessarily represent those of their affiliated organizations, or those of the publisher, the editors and the reviewers. Any product that may be evaluated in this article, or claim that may be made by its manufacturer, is not guaranteed or endorsed by the publisher.
The Supplementary Material for this article can be found online at: https://www.frontiersin.org/articles/10.3389/fgene.2025.1450259/full#supplementary-material
Ahn, H. J., An, H. Y., Ryu, G., Lim, J., Sun, C., Song, H., et al. (2024). Clonal haematopoiesis of indeterminate potential and atrial fibrillation: an east Asian cohort study. Eur. Heart J. 45, 778–790. doi:10.1093/eurheartj/ehad869
Aune, D., Sen, A., Schlesinger, S., Norat, T., Janszky, I., Romundstad, P., et al. (2017). Body mass index, abdominal fatness, fat mass and the risk of atrial fibrillation: a systematic review and dose-response meta-analysis of prospective studies. Eur. J. Epidemiol. 32, 181–192. doi:10.1007/s10654-017-0232-4
Biggar, R. J., Wohlfahrt, J., Oudin, A., Hjuler, T., and Melbye, M. (2011). Digoxin use and the risk of breast cancer in women. J. Clin. Oncol. 29, 2165–2170. doi:10.1200/JCO.2010.32.8146
Boudjadi, S., Carrier, J. C., Groulx, J. F., and Beaulieu, J. F. (2016). Integrin α1β1 expression is controlled by c-MYC in colorectal cancer cells. Oncogene 35, 1671–1678. doi:10.1038/onc.2015.231
Boutas, I., Kontogeorgi, A., Kalantaridou, S. N., Dimitrakakis, C., Patsios, P., Kalantzi, M., et al. (2023). Reverse onco-cardiology: what is the evidence for breast cancer? A systematic review of the literature. Int. J. Mol. Sci. 24, 16500. doi:10.3390/ijms242216500
Brion, M. J., Shakhbazov, K., and Visscher, P. M. (2013). Calculating statistical power in Mendelian randomization studies. Int. J. Epidemiol. 42, 1497–1501. doi:10.1093/ije/dyt179
Bulik-Sullivan, B., Finucane, H. K., Anttila, V., Gusev, A., Day, F. R., Loh, P. R., et al. (2015). An atlas of genetic correlations across human diseases and traits. Nat. Genet. 47, 1236–1241. doi:10.1038/ng.3406
Burgess, S., and Thompson, S. G.CRP CHD Genetics Collaboration (2011). Collaboration CCG: avoiding bias from weak instruments in Mendelian randomization studies. Int. J. Epidemiol. 40, 755–764. doi:10.1093/ije/dyr036
Byrska-Bishop, M., Evani, U. S., Zhao, X., Basile, A. O., Abel, H. J., Regier, A. A., et al. (2022). High-coverage whole-genome sequencing of the expanded 1000 Genomes Project cohort including 602 trios. Cell 185, 3426–3440.e19. doi:10.1016/j.cell.2022.08.004
Chen, M., Li, C., Liao, P., Cui, X., Tian, W., Wang, Q., et al. (2022). Epidemiology, management, and outcomes of atrial fibrillation among 30 million citizens in Shanghai, China from 2015 to 2020: a medical insurance database study. Lancet Reg. Health West Pac 23, 100470. doi:10.1016/j.lanwpc.2022.100470
Chen, Y. H., Xu, S. J., Bendahhou, S., Wang, X. L., Wang, Y., Xu, W. Y., et al. (2003). KCNQ1 gain-of-function mutation in familial atrial fibrillation. Science 299, 251–254. doi:10.1126/science.1077771
Conen, D., Wong, J. A., Sandhu, R. K., Cook, N. R., Lee, I. M., Buring, J. E., et al. (2016). Albert CM: risk of malignant cancer among women with new-onset atrial fibrillation. JAMA Cardiol. 1, 389–396. doi:10.1001/jamacardio.2016.0280
Consortium, G. T. (2013). The genotype-tissue expression (GTEx) project. Nat. Genet. 45, 580–585. doi:10.1038/ng.2653
Cui, K., Zhang, H., and Wang, G. Z. (2019). MiR-483 suppresses cell proliferation and promotes cell apoptosis by targeting SOX3 in breast cancer. Eur. Rev. Med. Pharmacol. Sci. 23, 2069–2074. doi:10.26355/eurrev_201903_17248
Darabi, H., McCue, K., Beesley, J., Michailidou, K., Nord, S., Kar, S., et al. (2015). Polymorphisms in a putative enhancer at the 10q21.2 breast cancer risk locus regulate NRBF2 expression. Am. J. Hum. Genet. 97, 22–34. doi:10.1016/j.ajhg.2015.05.002
D'Souza, M., Smedegaard, L., Madelaire, C., Nielsen, D., Torp-Pedersen, C., Gislason, G., et al. (2019). Incidence of atrial fibrillation in conjunction with breast cancer. Heart rhythm. 16, 343–348. doi:10.1016/j.hrthm.2018.10.017
Durinck, S., Spellman, P. T., Birney, E., and Huber, W. (2009). Mapping identifiers for the integration of genomic datasets with the R/Bioconductor package biomaRt. Nat. Protoc. 4, 1184–1191. doi:10.1038/nprot.2009.97
Elsworth, B., Lyon, M., Alexander, T., Liu, Y., Matthews, P., Hallett, J., et al. (2020). The MRC IEU OpenGWAS data infrastructure. BioRxiv 2020:2020.2008. 2010.244293. doi:10.1101/2020.08.10.244293
Ershaid, N., Sharon, Y., Doron, H., Raz, Y., Shani, O., Cohen, N., et al. (2019). NLRP3 inflammasome in fibroblasts links tissue damage with inflammation in breast cancer progression and metastasis. Nat. Commun. 10, 4375. doi:10.1038/s41467-019-12370-8
Fares, W. H. (2013). Amiodarone and the risk of cancer: a nationwide population-based study. Cancer 119, 4051. doi:10.1002/cncr.28266
Farh, K. K., Marson, A., Zhu, J., Kleinewietfeld, M., Housley, W. J., Beik, S., et al. (2015). Genetic and epigenetic fine mapping of causal autoimmune disease variants. Nature 518, 337–343. doi:10.1038/nature13835
Fournier, A., McLeer-Florin, A., Lefebvre, C., Duley, S., Barki, L., Ribeyron, J., et al. (2010). 1q12 chromosome translocations form aberrant heterochromatic foci associated with changes in nuclear architecture and gene expression in B cell lymphoma. EMBO Mol. Med. 2, 159–171. doi:10.1002/emmm.201000067
Frei, O., Holland, D., Smeland, O. B., Shadrin, A. A., Fan, C. C., Maeland, S., et al. (2019). Bivariate causal mixture model quantifies polygenic overlap between complex traits beyond genetic correlation. Nat. Commun. 10, 2417. doi:10.1038/s41467-019-10310-0
Giambartolomei, C., Vukcevic, D., Schadt, E. E., Franke, L., Hingorani, A. D., Wallace, C., et al. (2014). Bayesian test for colocalisation between pairs of genetic association studies using summary statistics. PLoS Genet. 10, e1004383. doi:10.1371/journal.pgen.1004383
Gibson, C. J., Fell, G., Sella, T., Sperling, A. S., Snow, C., Rosenberg, S. M., et al. (2023). Clonal hematopoiesis in young women treated for breast cancer. Clin. Cancer Res. 29, 2551–2558. doi:10.1158/1078-0432.CCR-23-0050
Giza, D. E., Iliescu, G., Hassan, S., Marmagkiolis, K., and Iliescu, C. (2017). Cancer as a risk factor for cardiovascular disease. Curr. Oncol. Rep. 19, 39. doi:10.1007/s11912-017-0601-x
Gremke, N., Griewing, S., Kalder, M., and Kostev, K. (2022). Positive association between body height and breast cancer prevalence: a retrospective study with 135,741 women in Germany. Breast Cancer Res. Treat. 196, 349–354. doi:10.1007/s10549-022-06730-0
Guha, A., Fradley, M. G., Dent, S. F., Weintraub, N. L., Lustberg, M. B., Alonso, A., et al. (2022). Incidence, risk factors, and mortality of atrial fibrillation in breast cancer: a SEER-Medicare analysis. Eur. Heart J. 43, 300–312. doi:10.1093/eurheartj/ehab745
Gulati, M., and Mulvagh, S. L. (2018). The connection between the breast and heart in a woman: breast cancer and cardiovascular disease. Clin. Cardiol. 41, 253–257. doi:10.1002/clc.22886
Guo, F., Tang, C., Huang, B., Gu, L., Zhou, J., Mo, Z., et al. (2022). LncRNA H19 drives proliferation of cardiac fibroblasts and collagen production via suppression of the miR-29a-3p/miR-29b-3p-VEGFA/TGF-beta Axis. Mol. Cells 45, 122–133. doi:10.14348/molcells.2021.0066
Gusev, A., Ko, A., Shi, H., Bhatia, G., Chung, W., Penninx, B. W., et al. (2016). Integrative approaches for large-scale transcriptome-wide association studies. Nat. Genet. 48, 245–252. doi:10.1038/ng.3506
Guzzetti, S., Costantino, G., Vernocchi, A., Sada, S., and Fundaro, C. (2008). First diagnosis of colorectal or breast cancer and prevalence of atrial fibrillation. Intern Emerg. Med. 3, 227–231. doi:10.1007/s11739-008-0124-4
Hemminki, K., Muller-Myhsok, B., Lichtner, P., Engel, C., Chen, B., Burwinkel, B., et al. (2010). Low-risk variants FGFR2, TNRC9 and LSP1 in German familial breast cancer patients. Int. J. Cancer 126, 2858–2862. doi:10.1002/ijc.24986
Hong, M., Ebana, Y., Shim, J., Choi, E. K., Lim, H. E., Hwang, I., et al. (2021). Ethnic similarities in genetic polymorphisms associated with atrial fibrillation: far East Asian vs European populations. Eur. J. Clin. Invest. 51, e13584. doi:10.1111/eci.13584
House, S. L., Castro, A. M., Lupu, T. S., Weinheimer, C., Smith, C., Kovacs, A., et al. (2016). Endothelial fibroblast growth factor receptor signaling is required for vascular remodeling following cardiac ischemia-reperfusion injury. Am. J. Physiol. Heart Circ. Physiol. 310, H559–H571. doi:10.1152/ajpheart.00758.2015
Hsu, J., Gore-Panter, S., Tchou, G., Castel, L., Lovano, B., Moravec, C. S., et al. (2018). Genetic control of left atrial gene expression yields insights into the genetic susceptibility for atrial fibrillation. Circ. Genom Precis. Med. 11, e002107. doi:10.1161/CIRCGEN.118.002107
Khouri, M. G., Douglas, P. S., Mackey, J. R., Martin, M., Scott, J. M., Scherrer-Crosbie, M., et al. (2012). Cancer therapy-induced cardiac toxicity in early breast cancer: addressing the unresolved issues. Circulation 126, 2749–2763. doi:10.1161/CIRCULATIONAHA.112.100560
Kirchmeyer, M., Servais, F. A., Hamdorf, M., Nazarov, P. V., Ginolhac, A., Halder, R., et al. (2018). Cytokine-mediated modulation of the hepatic miRNome: miR-146b-5p is an IL-6-inducible miRNA with multiple targets. J. Leukoc. Biol. 104, 987–1002. doi:10.1002/JLB.MA1217-499RR
Koene, R. J., Prizment, A. E., Blaes, A., and Konety, S. H. (2016). Shared risk factors in cardiovascular disease and cancer. Circulation 133, 1104–1114. doi:10.1161/CIRCULATIONAHA.115.020406
Kofler, T., Theriault, S., Bossard, M., Aeschbacher, S., Bernet, S., Krisai, P., et al. (2017). Relationships of measured and genetically determined height with the cardiac conduction system in healthy adults. Circ. Arrhythm. Electrophysiol. 10, e004735. doi:10.1161/CIRCEP.116.004735
Kurki, M. I., Karjalainen, J., Palta, P., Sipila, T. P., Kristiansson, K., Donner, K. M., et al. (2023). FinnGen provides genetic insights from a well-phenotyped isolated population. Nature 613, 508–518. doi:10.1038/s41586-022-05473-8
Leanca, S. A., Crisu, D., Petris, A. O., Afrasanie, I., Genes, A., Costache, A. D., et al. (2022). Left ventricular remodeling after myocardial infarction: from physiopathology to treatment. Life (Basel) 12, 1111. doi:10.3390/life12081111
Lei, H., and Deng, C. X. (2017). Fibroblast growth factor receptor 2 signaling in breast cancer. Int. J. Biol. Sci. 13, 1163–1171. doi:10.7150/ijbs.20792
Leong, D. P., Caron, F., Hillis, C., Duan, A., Healey, J. S., Fraser, G., et al. (2016). The risk of atrial fibrillation with ibrutinib use: a systematic review and meta-analysis. Blood 128, 138–140. doi:10.1182/blood-2016-05-712828
Li, K., Xu, C., Du, Y., Junaid, M., Kaushik, A. C., and Wei, D. Q. (2019). Comprehensive epigenetic analyses reveal master regulators driving lung metastasis of breast cancer. J. Cell Mol. Med. 23, 5415–5431. doi:10.1111/jcmm.14424
Li, L., Zhang, R., Liu, Y., and Zhang, G. (2020). ANXA4 activates JAK-STAT3 signaling by interacting with ANXA1 in basal-like breast cancer. DNA Cell Biol. 39, 1649–1656. doi:10.1089/dna.2020.5570
Li, N., Wang, L., Li, L., Yang, M. Z., Wang, Q. X., Bai, X. W., et al. (2023). The correlation between gut microbiome and atrial fibrillation: pathophysiology and therapeutic perspectives. Mil. Med. Res. 10, 51. doi:10.1186/s40779-023-00489-1
Li, P., Shi, D., Shen, W., Shi, S., Guo, X., Li, J., et al. (2022). Pilot genome-wide association study of antibody response to inactivated SARS-CoV-2 vaccines. Front. Immunol. 13, 1054147. doi:10.3389/fimmu.2022.1054147
Li, X., and Zhu, X. (2017). Cross-phenotype association analysis using summary statistics from GWAS. Methods Mol. Biol. 1666, 455–467. doi:10.1007/978-1-4939-7274-6_22
Ling, T. Y., Yi, F., Lu, T., Wang, X. L., Sun, X., Willis, M. S., et al. (2019). F-box protein-32 down-regulates small-conductance calcium-activated potassium channel 2 in diabetic mouse atria. J. Biol. Chem. 294, 4160–4168. doi:10.1074/jbc.RA118.003837
Liu, M., Jiang, Y., Wedow, R., Li, Y., Brazel, D. M., Chen, F., et al. (2019). Association studies of up to 1.2 million individuals yield new insights into the genetic etiology of tobacco and alcohol use. Nat. Genet. 51, 237–244. doi:10.1038/s41588-018-0307-5
Lu, Y., Quan, C., Chen, H., Bo, X., and Zhang, C. (2017). 3DSNP: a database for linking human noncoding SNPs to their three-dimensional interacting genes. Nucleic Acids Res. 45, D643–D649. doi:10.1093/nar/gkw1022
Luo, L., Zhang, Z., Qiu, N., Ling, L., Jia, X., Song, Y., et al. (2021). Disruption of FOXO3a-miRNA feedback inhibition of IGF2/IGF-1R/IRS1 signaling confers Herceptin resistance in HER2-positive breast cancer. Nat. Commun. 12, 2699. doi:10.1038/s41467-021-23052-9
Makeyev, A. V., Erdenechimeg, L., Mungunsukh, O., Roth, J. J., Enkhmandakh, B., Ruddle, F. H., et al. (2004). GTF2IRD2 is located in the Williams-Beuren syndrome critical region 7q11.23 and encodes a protein with two TFII-I-like helix-loop-helix repeats. Proc. Natl. Acad. Sci. U. S. A. 101, 11052–11057. doi:10.1073/pnas.0404150101
Mancuso, N., Freund, M. K., Johnson, R., Shi, H., Kichaev, G., Gusev, A., et al. (2019). Probabilistic fine-mapping of transcriptome-wide association studies. Nat. Genet. 51, 675–682. doi:10.1038/s41588-019-0367-1
Masuhara, M., Nagao, K., Nishikawa, M., Kimura, T., and Nakano, T. (2003). Enhanced degradation of MDM2 by a nuclear envelope component, mouse germ cell-less. Biochem. Biophys. Res. Commun. 308, 927–932. doi:10.1016/s0006-291x(03)01497-9
Matthews, A. A., Peacock, H. S., Stanway, S., Lyon, A. R., Smeeth, L., Bhaskaran, K., et al. (2021). Risk of cardiovascular diseases among older breast cancer survivors in the United States: a matched cohort study. J. Natl. Compr. Canc Netw. 19, 275–284. doi:10.6004/jnccn.2020.7629
Miyazawa, K., Ito, K., Ito, M., Zou, Z., Kubota, M., Nomura, S., et al. (2023). Cross-ancestry genome-wide analysis of atrial fibrillation unveils disease biology and enables cardioembolic risk prediction. Nat. Genet. 55, 187–197. doi:10.1038/s41588-022-01284-9
Moller, S., Mucci, L. A., Harris, J. R., Scheike, T., Holst, K., Halekoh, U., et al. (2016). The heritability of breast cancer among women in the nordic twin study of cancer. Cancer Epidemiol. Biomarkers Prev. 25, 145–150. doi:10.1158/1055-9965.EPI-15-0913
Morrison, J., Knoblauch, N., Marcus, J. H., Stephens, M., and He, X. (2020). Mendelian randomization accounting for correlated and uncorrelated pleiotropic effects using genome-wide summary statistics. Nat. Genet. 52, 740–747. doi:10.1038/s41588-020-0631-4
Nalio Ramos, R., Missolo-Koussou, Y., Gerber-Ferder, Y., Bromley, C. P., Bugatti, M., Nunez, N. G., et al. (2022). Tissue-resident FOLR2(+) macrophages associate with CD8(+) T cell infiltration in human breast cancer. Cell 185, 1189–1207.e25. doi:10.1016/j.cell.2022.02.021
Nielsen, J. B., Thorolfsdottir, R. B., Fritsche, L. G., Zhou, W., Skov, M. W., Graham, S. E., et al. (2018). Biobank-driven genomic discovery yields new insight into atrial fibrillation biology. Nat. Genet. 50, 1234–1239. doi:10.1038/s41588-018-0171-3
Niinaka, Y., Harada, K., Fujimuro, M., Oda, M., Haga, A., Hosoki, M., et al. (2010). Silencing of autocrine motility factor induces mesenchymal-to-epithelial transition and suppression of osteosarcoma pulmonary metastasis. Cancer Res. 70, 9483–9493. doi:10.1158/0008-5472.CAN-09-3880
Ning, Z., Pawitan, Y., and Shen, X. (2020). High-definition likelihood inference of genetic correlations across human complex traits. Nat. Genet. 52, 859–864. doi:10.1038/s41588-020-0653-y
Papadimitriou, N., Dimou, N., Tsilidis, K. K., Banbury, B., Martin, R. M., Lewis, S. J., et al. (2020). Physical activity and risks of breast and colorectal cancer: a Mendelian randomisation analysis. Nat. Commun. 11, 597. doi:10.1038/s41467-020-14389-8
Park, Y. M., Jung, W., Yeo, Y., Park, S. H., Fradley, M. G., Malapati, S. J., et al. (2024). Mid- and long-term risk of atrial fibrillation among breast cancer surgery survivors. BMC Med. 22, 88. doi:10.1186/s12916-024-03308-z
Pickrell, J. K., Berisa, T., Liu, J. Z., Segurel, L., Tung, J. Y., and Hinds, D. A. (2016). Detection and interpretation of shared genetic influences on 42 human traits. Nat. Genet. 48, 709–717. doi:10.1038/ng.3570
Purcell, S., Neale, B., Todd-Brown, K., Thomas, L., Ferreira, M. A., Bender, D., et al. (2007). PLINK: a tool set for whole-genome association and population-based linkage analyses. Am. J. Hum. Genet. 81, 559–575. doi:10.1086/519795
Saliba, W., Rennert, H. S., Gronich, N., Gruber, S. B., and Rennert, G. (2018). Association of atrial fibrillation and cancer: analysis from two large population-based case-control studies. PLoS One 13, e0190324. doi:10.1371/journal.pone.0190324
Sangurdekar, D., Sun, C., McLaughlin, H., Ayling-Rouse, K., Allaire, N. E., Penny, M. A., et al. (2019). Genetic study of severe prolonged lymphopenia in multiple sclerosis patients treated with dimethyl fumarate. Front. Genet. 10, 1039. doi:10.3389/fgene.2019.01039
Sansonetti, M., Waleczek, F. J. G., Jung, M., Thum, T., and Perbellini, F. (2020). Resident cardiac macrophages: crucial modulators of cardiac (patho)physiology. Basic Res. Cardiol. 115, 77. doi:10.1007/s00395-020-00836-6
Schnabel, R. B., Yin, X., Gona, P., Larson, M. G., Beiser, A. S., McManus, D. D., et al. (2015). 50 year trends in atrial fibrillation prevalence, incidence, risk factors, and mortality in the Framingham Heart Study: a cohort study. Lancet 386, 154–162. doi:10.1016/S0140-6736(14)61774-8
Scott, L., Li, N., and Dobrev, D. (2019). Role of inflammatory signaling in atrial fibrillation. Int. J. Cardiol. 287, 195–200. doi:10.1016/j.ijcard.2018.10.020
Siegel, R. L., Giaquinto, A. N., and Jemal, A. (2024). Cancer statistics, 2024. CA Cancer J. Clin. 74, 12–49. doi:10.3322/caac.21820
Sollis, E., Mosaku, A., Abid, A., Buniello, A., Cerezo, M., Gil, L., et al. (2023). The NHGRI-EBI GWAS Catalog: knowledgebase and deposition resource. Nucleic Acids Res. 51, D977–D985. doi:10.1093/nar/gkac1010
Su, M., Hou, Y., Cai, S., Li, W., Wei, Y., Wang, R., et al. (2024). Elevated ITGA1 levels in type 2 diabetes: implications for cardiac function impairment. Diabetologia 67, 850–863. doi:10.1007/s00125-024-06109-4
Su, V. Y., Hu, Y. W., Chou, K. T., Ou, S. M., Lee, Y. C., Lin, E. Y., et al. (2013). Amiodarone and the risk of cancer: a nationwide population-based study. Cancer 119, 1699–1705. doi:10.1002/cncr.27881
Tao, H., Yang, J. J., Chen, Z. W., Xu, S. S., Zhou, X., Zhan, H. Y., et al. (2014). DNMT3A silencing RASSF1A promotes cardiac fibrosis through upregulation of ERK1/2. Toxicology 323, 42–50. doi:10.1016/j.tox.2014.06.006
von Mering, C., Huynen, M., Jaeggi, D., Schmidt, S., Bork, P., and Snel, B. (2003). STRING: a database of predicted functional associations between proteins. Nucleic Acids Res. 31, 258–261. doi:10.1093/nar/gkg034
Wang, H., Liu, H., Zhao, L., Luo, S., Akinyemiju, T., Hwang, S., et al. (2021). Association of genetic variants of FBXO32 and FOXO6 in the FOXO pathway with breast cancer risk. Mol. Carcinog. 60, 661–670. doi:10.1002/mc.23331
Wassertheil-Smoller, S., McGinn, A. P., Martin, L., Rodriguez, B. L., Stefanick, M. L., and Perez, M. (2017). The associations of atrial fibrillation with the risks of incident invasive breast and colorectal cancer. Am. J. Epidemiol. 185, 372–384. doi:10.1093/aje/kww185
Watanabe, S., Watanabe, K., Akimov, V., Bartkova, J., Blagoev, B., Lukas, J., et al. (2013). JMJD1C demethylates MDC1 to regulate the RNF8 and BRCA1-mediated chromatin response to DNA breaks. Nat. Struct. Mol. Biol. 20, 1425–1433. doi:10.1038/nsmb.2702
Werme, J., van der Sluis, S., Posthuma, D., and de Leeuw, C. A. (2022). An integrated framework for local genetic correlation analysis. Nat. Genet. 54, 274–282. doi:10.1038/s41588-022-01017-y
Wu, X., Yang, Z. H., Wu, J., and Han, J. (2023b). Ribosome-rescuer PELO catalyzes the oligomeric assembly of NOD-like receptor family proteins via activating their ATPase enzymatic activity. Immunity 56, 926–943.e7. doi:10.1016/j.immuni.2023.02.014
Wu, X., Zhang, W., Zhao, X., Zhang, L., Xu, M., Hao, Y., et al. (2023a). Investigating the relationship between depression and breast cancer: observational and genetic analyses. BMC Med. 21, 170. doi:10.1186/s12916-023-02876-w
Xu, W. H., Shu, X. O., Long, J., Lu, W., Cai, Q., Zheng, Y., et al. (2011). Relation of FGFR2 genetic polymorphisms to the association between oral contraceptive use and the risk of breast cancer in Chinese women. Am. J. Epidemiol. 173, 923–931. doi:10.1093/aje/kwq460
Xue, C., Jiang, L., Zhou, M., Long, Q., Chen, Y., Li, X., et al. (2022). PCGA: a comprehensive web server for phenotype-cell-gene association analysis. Nucleic Acids Res. 50, W568–W576. doi:10.1093/nar/gkac425
Yan, T., Zhu, S., Shi, Y., Xie, C., Zhu, M., Zhang, Y., et al. (2021). Pan-cancer analysis of atrial-fibrillation-related innate immunity gene ANXA4. Front. Cardiovasc Med. 8, 713983. doi:10.3389/fcvm.2021.713983
Yao, C., Veleva, T., Scott, L., Cao, S., Li, L., Chen, G., et al. (2018). Enhanced cardiomyocyte NLRP3 inflammasome signaling promotes atrial fibrillation. Circulation 138, 2227–2242. doi:10.1161/CIRCULATIONAHA.118.035202
Yao, X., Hu, Q., Liu, X., Ling, Q., Leng, Y., Zhao, H., et al. (2023). Atrial fibrillation and breast cancer-Vicious twins? A systematic review and meta-analysis. Front. Cardiovasc Med. 10, 1113231. doi:10.3389/fcvm.2023.1113231
Zerbino, D. R., Achuthan, P., Akanni, W., Amode, M. R., Barrell, D., Bhai, J., et al. (2018). Ensembl 2018. Nucleic Acids Res. 46, D754–D761. doi:10.1093/nar/gkx1098
Zhang, H., Ahearn, T. U., Lecarpentier, J., Barnes, D., Beesley, J., Qi, G., et al. (2020). Genome-wide association study identifies 32 novel breast cancer susceptibility loci from overall and subtype-specific analyses. Nat. Genet. 52, 572–581. doi:10.1038/s41588-020-0609-2
Zhang, J., Xia, Y., and Sun, J. (2021). Breast and gut microbiome in health and cancer. Genes Dis. 8, 581–589. doi:10.1016/j.gendis.2020.08.002
Zheng, J., Baird, D., Borges, M. C., Bowden, J., Hemani, G., Haycock, P., et al. (2017). Recent developments in mendelian randomization studies. Curr. Epidemiol. Rep. 4, 330–345. doi:10.1007/s40471-017-0128-6
Zhou, D., Jiang, Y., Zhong, X., Cox, N. J., Liu, C., and Gamazon, E. R. (2020). A unified framework for joint-tissue transcriptome-wide association and Mendelian randomization analysis. Nat. Genet. 52, 1239–1246. doi:10.1038/s41588-020-0706-2
Zhu, X., Feng, T., Tayo, B. O., Liang, J., Young, J. H., Franceschini, N., et al. (2015). Meta-analysis of correlated traits via summary statistics from GWASs with an application in hypertension. Am. J. Hum. Genet. 96, 21–36. doi:10.1016/j.ajhg.2014.11.011
Zhu, Z., Hasegawa, K., Camargo, C. A., and Liang, L. (2021). Investigating asthma heterogeneity through shared and distinct genetics: insights from genome-wide cross-trait analysis. J. Allergy Clin. Immunol. 147, 796–807. doi:10.1016/j.jaci.2020.07.004
Keywords: atrial fibrillation, breast cancer, genetic correlation, shared genetic etiology, pleiotropic gene, causal inference
Citation: Yang Y, Chen J, Zhao X, Gong F, Liu R, Miao J, Lin M, Ge F and Chen W (2025) Genetic analysis reveals the shared genetic architecture between breast cancer and atrial fibrillation. Front. Genet. 16:1450259. doi: 10.3389/fgene.2025.1450259
Received: 17 June 2024; Accepted: 28 February 2025;
Published: 25 March 2025.
Edited by:
José M. Álvarez-Castro, University of Santiago de Compostela, SpainReviewed by:
Lazaros Lataniotis, Sana Biotechnology, Inc., United StatesCopyright © 2025 Yang, Chen, Zhao, Gong, Liu, Miao, Lin, Ge and Chen. This is an open-access article distributed under the terms of the Creative Commons Attribution License (CC BY). The use, distribution or reproduction in other forums is permitted, provided the original author(s) and the copyright owner(s) are credited and that the original publication in this journal is cited, in accordance with accepted academic practice. No use, distribution or reproduction is permitted which does not comply with these terms.
*Correspondence: Fei Ge, YWpxbmFkamRAaG90bWFpbC5jb20=; Wenlin Chen, Y2hlbndlbmxpbjFAaG90bWFpbC5jb20=
†These authors have contributed equally to this work
Disclaimer: All claims expressed in this article are solely those of the authors and do not necessarily represent those of their affiliated organizations, or those of the publisher, the editors and the reviewers. Any product that may be evaluated in this article or claim that may be made by its manufacturer is not guaranteed or endorsed by the publisher.
Research integrity at Frontiers
Learn more about the work of our research integrity team to safeguard the quality of each article we publish.