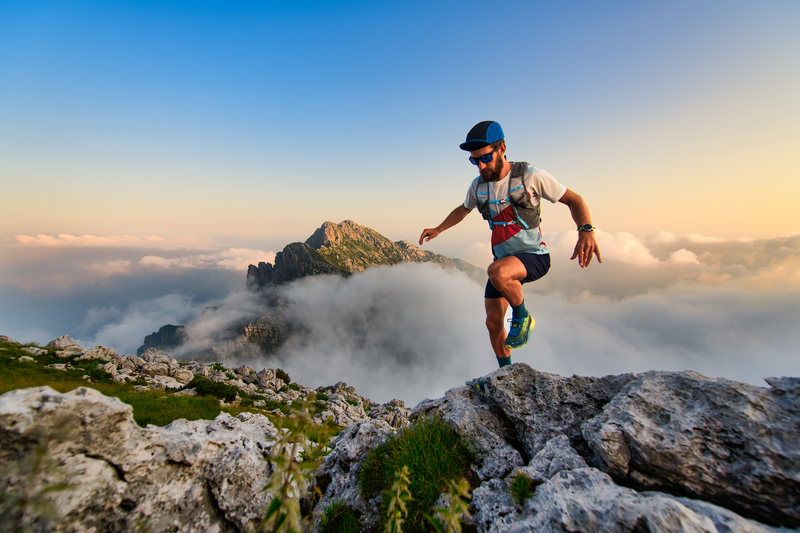
95% of researchers rate our articles as excellent or good
Learn more about the work of our research integrity team to safeguard the quality of each article we publish.
Find out more
METHODS article
Front. Genet. , 20 November 2024
Sec. Statistical Genetics and Methodology
Volume 15 - 2024 | https://doi.org/10.3389/fgene.2024.1489694
This article is part of the Research Topic Statistical Approaches, Applications, and Software for Longitudinal Microbiome Data Analysis and Microbiome Multi-Omics Data Integration View all 8 articles
Sparse canonical correlation analysis (sCCA) has been a useful approach for integrating different high-dimensional datasets by finding a subset of correlated features that explain the most correlation in the data. In the context of microbiome studies, investigators are always interested in knowing how the microbiome interacts with the host at different molecular levels such as genome, methylol, transcriptome, metabolome and proteome. sCCA provides a simple approach for exploiting the correlation structure among multiple omics data and finding a set of correlated omics features, which could contribute to understanding the host-microbiome interaction. However, existing sCCA methods do not address compositionality, and its application to microbiome data is thus not optimal. This paper proposes a new sCCA framework for integrating microbiome data with other high-dimensional omics data, accounting for the compositional nature of microbiome sequencing data. It also allows integrating prior structure information such as the grouping structure among bacterial taxa by imposing a “soft” constraint on the coefficients through varying penalization strength. As a result, the method provides significant improvement when the structure is informative while maintaining robustness against a misspecified structure. Through extensive simulation studies and real data analysis, we demonstrate the superiority of the proposed framework over the state-of-the-art approaches.
The human microbiome is the collection of microorganisms and their genetic makeup associated with the human body. It plays a critical role in human health and disease ranging from gastrointestinal diseases to various cancers (Sepich-Poore et al., 2021). To gain more mechanistic insights, multi-omics approaches have been increasingly employed in microbiome studies to elucidate the intricate interplay between the environment, the human microbiome and the host at different molecular levels (Hasin et al., 2017; Lloyd-Price et al., 2019). Although many multi-omics datasets have been generated in the past few years, it is unclear how to integrate them efficiently. One useful tool for multi-omics data integration is to perform canonical correlation analysis (CCA). CCA, due to Hotelling (1936), connects two sets of variables by finding a linear combination of variables that maximally correlate. However, the standard CCA fails when the sample size is strictly less than the number of variables as one can find meaningless solutions with correlations equal to one. Also, it does not perform variable selection and hence lacks interpretability. To circumvent these problems, sparse CCA (sCCA) has been proposed, aiming to find pairs of sparse canonical directions by imposing sparsity penalty. The first sCCA algorithm was presented by Parkhomenko et al. (2007), which, however, lacks exact criterion and biconvexity. Witten et al. (2009) applied the penalized matrix decomposition to cross-product matrix and yielded a straightforward formulation for sCCA. Some closely related methods include Parkhomenko et al., 2009; Lê Cao et al., 2009. Hardoon and Shawe-Taylor (2011) expressed the sCCA model as a primal-dual Rayleigh quotient, which takes the primal representation and kernel representation as the first view and second view, respectively. Chu et al. (2013) reformed CCA into a trace maximization problem and computed the sparse solution by the linearized Bregman method. To exploit the potential structural information among features, various forms of structure-adaptive sCCA have been proposed (Lin et al., 2013; Chen et al., 2012; Mohammadi-Nejad et al., 2017). In particular, Chen et al. (2013) proposed the structure-constrained sCCA (ssCCA) to exploit the phylogenetic structure in microbiome data.
Advances in next-generation sequencing technologies have enabled the direct sequencing of microbial DNA to determine microbiome composition, using either targeted or shotgun approaches (Wensel et al., 2022). The resulting microbiome data is typically in the form of a count table that records the frequencies of detected taxa in specific samples. However, due to the complexities inherent in the sequencing process, the total count for a sample reflects the sequencing effort rather than the actual microbial load at the sampling site. Consequently, microbiome data are inherently compositional, meaning that we only have information about the relative abundances of taxa. This compositionality presents significant challenges in the statistical analysis of microbiome data. A change in the (absolute) abundance of one taxon can lead to apparent changes in the relative abundances of all other taxa, complicating the identification of the actual causal taxa (Yang and Chen, 2022). The compositional nature also renders many standard multivariate statistical models inappropriate or inapplicable (Aitchison, 1982). Many efforts have been made to address the compositionality in different contexts of microbiome data analysis. For example, Friedman and Alm (2012) developed an iterative procedure named SparCC that allows inference of correlations for compositional data by assuming that the number of taxa is large and the true correlation network is sparse. Lin et al. (2014) dealt with the variable selection in regression with compositional covariates. Jiang et al. (2019) addressed zero inflation and detected pairs of associated compositional and non-compositional covariates using a Bayesian zero-inflated negative binomial regression model. However, existing CCA methods including ssCCA could not address the compositional effects, potentially reducing its precision in recovering relevant taxa.
We propose a new sCCA framework for integrating microbiome data with other high-dimensional omics data. The framework specifically addresses the compositional nature of the microbiome data. It also allows integrating prior structure information by imposing a “soft” constraint on the coefficients through varying penalization strength. As a result, the method provides significant improvement when the structure is informative while maintaining robustness against a misspecified structure. The developed tool aims to be an important resource for investigators to understand the interplay between the microbiome and host, decipher the molecular mechanisms underlying microbiome-disease association, and identify potential microbial targets for intervention.
This paper is organized as follows. Section 2 introduces the new sCCA framework for integrating microbiome compositional data with (non-)compositional high-dimensional data. Section 3 extends the new framework to incorporate additional prior structural information. In Section 4, we conduct numerical simulations to demonstrate the effectiveness of our proposed methods. Section 5 applies the proposed methods in a real microbiome study to investigate the association between gut bacteria and its metabolic output. We conclude with a discussion in Section 6.
Let us consider two random vectors
Therefore, the compositional CCA aims to find
When the dimensions
Here
It has been shown that in high dimensions, treating the covariance matrix as diagonal can yield good results. Following the same strategy adopted by many of the existing high-dimensional CCA algorithms (e.g., Witten et al., 2009), we substitute in the identity matrix for
which can be solved by iteratively optimizing the objective function with respect to one parameter while fixing the other parameter. Specifically, we have the following two updating steps.
1. Update
2. Update
In this section, we discuss the updates in Equations 2, 3. Define the operator
By exploring the Karush-Kuhn-Tuchker conditions, we obtain the following result.
Proposition 2.1. Set
Remark 2.1. We employ the augmented Lagrangian method (ALM) to solve the optimization problem in
where
where
Using similar arguments, we can show that the solution to (3) is given by
where
As our goal is to find two directions
Algorithm 1.Compositional sCCA: compositional data versus non-compositional data.
1. Initialize
2. Update
where
3. Update
4. Iterate Steps 2 and 3 until convergence.
To select the regularization parameters
where
We briefly describe an extension to the case, where both
where
Algorithm 2.Compositional sCCA: compositional data versus compositional data.
1. Initialize
2. Update
3. Update
4. Iterate Steps 2 and 3 until convergence.
In this section, following the strategy proposed in Pramanik and Zhang (2020), we aim to incorporate the prior structure information robustly in the compositional sCCA procedure. The prior structure information could be the grouping structure or the phylogenetic tree structure among the taxa. The idea is to define a set of constraints that encode the prior structure information and use the constraints together with the data to estimate the weights
Based on the setups described in Section 2.1, our procedure is to translate the auxiliary information into different penalization strengths through the weights
where
Following Pramanik and Zhang (2020), we impose a penalty term on the weights and propose an algorithm to jointly estimate weights and parameters. Specifically, we define
We estimate
The design of the function
Next we introduce the algorithm to solve the above problem. We focus on the update for
When
If we do not have any prior structural information on
Algorithm 3 summarizes the implementation details of the structure adaptive Compositional sCCA. The selection of tuning parameter pair
Algorithm 3.Structure-Adaptive Compositional sCCA: compositional data versus non-compositional data.
1. Initialize
2. Update
where
3. Update
4. Update
5. Iterate Steps 2-4 until convergence.
In this section, we evaluate the finite sample performance of the proposed methods through numerical simulations.
We first consider the CCA problem between compositional data (i.e., microbiome data) and non-compositional data (e.g., metabolomics data) following a similar setting considered in Chen et al. (2013). To capture the dependence between the two sets of high-dimensional data, we use a latent variable model to generate the compositional variables
where
S1
S2
where
The first two groups contain both zero and nonzero entries reflecting the fact that the external structure information is imperfect and noisy. We set
where TP, FP, TN, and FN represent the true positives, false positives, true negatives, and false negatives, respectively. The TPR, FPR, MCC, and Precision are computed by averaging over 100 simulation replicates. Denote the estimated canonical coefficients by
We compare the performance of the following four methods.
1. sCCA: sCCA without considering the compositional effect;
2. C-sCCA: compositional sCCA;
3. AC-sCCA: adaptive compositional sCCA, i.e.,
4. SAC-sCCA: structure adaptive compositional sCCA, i.e.,
For AC-sCCA and SAC-sCCA, we also apply adaptive weights on
Table 1 summarizes the results for the above four methods when fixing
Table 1. Performance of sCCA for the association between compositional data and non-compositional data
Figure 1. TPR, FPR, MCC, and Precision of sCCA for the association between compositional data and non-compositional data across association strength. Here, the range of
Figure 2. RMSE of sCCA for the association between compositional data and non-compositional data across association strength. Here, the range of
Finally, we examine the scenario where
Table 2. Performance of sCCA for the association between compositional data and non-compositional data (
In this section, we study the performance of the proposed compositional sCCA for the association between two compositional datasets, for example, bacterial taxa abundance vs fungi taxa abundance. We modify the setting in Section 4.1 by considering the following models
where
S3
S4
where
Table 3. Performance of sCCA for the association between two compositional datasets
Figure 3. TPR, FPR, MCC, and Precision of sCCA for the association between two compositional datasets across signal strength. Here, the range of
Figure 4. RMSE of sCCA for the association between two compositional datasets across association strength. Here, the range of
We applied sCCA, C-sCCA, AC-sCCA and SAC-sCCA to examine the association between gut bacterial composition and gut metabolism in a colorectal adenoma study conducted at the Mayo Clinic. The study utilized both gut microbiome and gut metabolomics data from 241 fecal samples selected from a frozen stool archive. The fecal samples were collected following a standard protocol and metabolomics profiling was conducted by Metabolon, Inc. (Durham, NC, United States) using a UPLCMS/MS platform, as detailed in Kim et al. (2020). Metabolic sub-pathway abundances were calculated by averaging the scaled abundances of metabolites within each sub-pathway, which are grouped into super-pathways. Bacterial DNA extraction and 16S rRNA gene sequencing were described in Hale et al. (2017). Specifically, the sequencing library was prepared at the University of Minnesota Genomics Center, and sequencing was performed using the Illumina MiSeq system at the Mayo Clinic Medical Genome Facility. These sequences were processed through the IM-TORNADO bioinformatics pipeline, clustering them into OTUs based on a 97% identity threshold.
We focused the analysis on the overall association between the bacterial genera and metabolic sub-pathways. We followed Chen et al. (2013) to pre-process data. We excluded genera that were present in less than 1/4 samples and kept 63 relatively common genera, each belonging to a specific phylum. This approach ensures a balance between retaining sufficient taxa for meaningful analysis while filtering out rare genera that could introduce noise. Zeros were replaced with 0.5 in microbiome data to facilitate working on the log scale. Our final dataset is summarized as a metabolic sub-pathway abundance matrix
We performed a two-stage five-fold CV described in Section 2.3 to identify the optimal tuning parameters across a range of models, from the most dense to the most sparse. To mitigate randomness, we conducted 100 replications of sample partitions. We selected the tuning parameter pair for each replication and recorded the corresponding CV values of four methods. As shown in Figure 5, sCCA has the lowest CV correlations, followed by C-sCCA and AC-sCCA, both of which yield comparable CV correlations, while SAC-sCCA achieves slightly higher CV correlations by incorporating grouping information. Therefore, by accounting for the compositional structure, we achieved a stronger association between the two datasets. The final parameters were determined by maximizing the CV values averaged across the 100 replications, with CV values of 0.6076 for sCCA, 0.6578 for C-sCCA, 0.6581 for AC-sCCA, and 0.6584 for SAC-sCCA.
Figure 5. Boxplot of 5-fold cross-validated correlations for sCCA, C-sCCA, AC-sCCA, and SAC-sCCA across 100 replications, with tuning parameters determined by sample partition in each replication.
Figure 6 shows the heatmap of pairwise spearman correlations between metabolic sub-pathways and genera selected by any of the four methods. The signs of the estimated coefficients align with the pairwise correlations. The selected metabolic sub-pathways belong to four super-pathways: Carbohydrate, Lipid, Cofactors and Vitamins, and Amino Acid. Hierarchical clustering analysis, using the complete linkage and Euclidean distance, was applied to cluster the bacterial genera. The coefficients estimated by C-sCCA, AC-sCCA, and SAC-sCCA for bacteria within the third cluster were mostly positive while the other two clusters showed an opposite trend. Interestingly, Fatty Acid, Diacarboxylate (FA-DC), identified by C-sCCA, AC-sCCA, and SAC-sCCA but not by sCCA, was overall negatively correlated with bacterial genera in the third cluster, and positively correlated with those in the first/second clusters. Dicarboxylic acids can be produced by various bacteria through different metabolic pathways (Yu et al., 2018). For example, species in the genus Clostridium, which showed a strong correlation with FA-DC in our data, can produce succinic acid and other dicarboxylic acids as fermentation products (Koendjbiharie et al., 2018). As a comparison, other detected metabolic sub-pathways exhibited both negative and positive correlations with bacterial genera in the third cluster, and overall negative correlations with those in the first/second clusters.
Figure 6. Heatmap of Spearman correlations between the bacterial genera and metabolic sub-pathways selected by either sCCA, C-sCCA, AC-sCCA, or SAC-sCCA. The color indicates the association direction, with red for positive correlations and blue for negative, varying in shade by strength. The bars at the top represent the average relative abundances of these genera on a log 10 scale, with orange indicating higher values and green indicating lower values. Abbreviations: AGE (Advanced Glycation End-product), EC (Endocannabinoid), FA-DC (Fatty Acid, Dicarboxylate), HPM (Hemoglobin and Porphyrin Metabolism), HM (Histidine Metabolism), MCST (Methionine, Cysteine, SAM and Taurine Metabolism), MM (Mevalonate Metabolism), PLM (Phospholipid Metabolism), PAM (Polyamine Metabolism), PUFA-n3&n6 (Polyunsaturated Fatty Acid, n3 and n6), PBAM (Primary Bile Acid Metabolism), SBAM (Secondary Bile Acid Metabolism), Steroid (Steroid), UCAPM (Urea cycle; Arginine and Proline Metabolism).
Despite achieving higher cross-validated correlations, the three methods that accounted for compositional structure failed to induce a sparse structure for the bacterial genera. Although this may be the biological truth, as gut metabolic capabilities are contributed by a large number of bacteria collectively (Cox et al., 2022), to gain more insights into the benefits of using the compositional constraint, we reconsidered C-sCCA by fixing the final parameter for metabolic sub-pathways and varying the parameter for bacterial genera to achieve the same cross-validated correlation as sCCA. Figure 7 shows the average cross-validated correlation of C-sCCA for each parameter pair. We selected the tuning parameter of bacterial genera as 0.4 so that the averaged CV value of C-sCCA is almost the same as that of sCCA.
Figure 7. Averaged cross-validated correlations across 100 replications. The blue horizontal line represents the CV value of sCCA with its optimal threshold. The red points denotes the CV values of C-sCCA with varying tuning parameters for gut bacterial genera.
Figure 8 presents the heatmap of pairwise Spearman correlations between the metabolic sub-pathways and genera selected by any of sCCA and C-sCCA with newly determined tuning parameter pair. C-sCCA identifies 8 metabolism sub-pathways and 17 bacterial genera, while sCCA selects a broader set of 13 metabolic sub-pathways and 35 bacterial genera. By incorporating the compositional structure, C-sCCA achieves the comparable averaged CV value with a more focused selection of metabolic sub-pathways and bacterial genera.
Figure 8. Heatmap of Spearman correlations between the bacterial genera and metabolic sub-pathways selected by either sCCA or C-sCCA. The CV values averaged across 100 replications are approximately 0.61 for both methods with the chosen tuning parameters. Other details are the same as in Figure 6.
Among the eight metabolic sub-pathways identified by C-sCCA, five belong to the Lipid super-pathway, two are part of the Amino Acid super-pathway, and only one, the Advanced Glycation End-product, is associated with the Carbohydrate super-pathway. For the bacterial genera, a comparison between C-sCCA results and the association analysis of bacterial genera and metabolic sub-pathways by Kim et al. (2020) reveals interesting patterns. The bacterial genera identified by C-sCCA are grouped into three clusters. The first two clusters predominantly show a negative association with the selected sub-pathways. In the first cluster, Oscillospira, Clostridium, Ruminococcus, Adlercreutzia, and Dehalobacterium are consistently selected by C-sCCA and were also reported in the findings of Kim et al. (2020). In the second cluster, Odoribacter is detected by C-sCCA but was not identified by Kim et al. (2020). In the third cluster, Bacteroides, Eggerthella, and Butyrivibrio stand out with the largest C-sCCA coefficients, showing strong positive correlations with the selected metabolic sub-pathways. In Kim et al. (2020), the genera were classified into two clusters, with the second cluster consisting of Bacteroides, Epulopiscium, and Butyrivibrio. Our hierarchical tree further reveals that Epulopiscium and Eggerthella exhibit very similar patterns, as they are grouped together.
In this study, we developed a compositional sparse canonical correlation analysis (C-sCCA) framework for association analysis between microbiome data and other high-dimensional datasets, accounting for the compositional nature of microbiome sequencing data. We introduced two variants of the C-sCCA method: one for compositional vs non-compositional data, and another for compositional vs compositional data. Our results show that by incorporating the compositional constraint, we achieved improved selection of relevant taxa, enhancing both power and precision. Additionally, we extended our framework to incorporate prior structural information, such as the grouping of bacterial taxa, among the compositional components. Application of C-sCCA to real microbiome data demonstrated that it produced results that were biologically more interpretable.
There are several potential extensions to our work. While we primarily focused on the grouping structure of bacterial taxa, we could also exploit the hierarchical grouping structure (phylum to genus) and the phylognetic relationship by devising appropriate constraints on the weights
where
for some decreasing function
We can also extend our approach to learn sub-spaces from multiple views, i.e., when we have multiple groups of measurements,
There are several limitations to our framework. First, we did not simultaneously address the zero inflation commonly observed in microbiome data. We used a simple zero replacement strategy before running the C-sCCA. Although this strategy has been commonly used in microbiome data analysis at log scale (Zhou et al., 2022; Lin and Peddada, 2020), better methods can be developed such as replacing the log scale transformation by modified centered log-ratio transform designed for addressing zero inflation (Yoon et al., 2019), imposing another multinomial layer to account for sampling variability associated with the sequencing process (Chen and Li, 2013), or using more informative imputation methods such as mbDenoise (Zeng et al., 2022) and mbImpute (Jiang et al., 2021). Second, although our framework can select subsets of features that explain the largest correlation between the datasets, their detailed relationships can not be learned simultaneously. Developing methods that combine feature-level selection with the construction of feature-feature correlation networks is a promising area for future research. Third, we can enhance robustness to outliers through several strategies, including outlier detection and removal during data preprocessing, replacing empirical covariance estimators with robust estimators (Luo et al., 2024), and investigating the optimal choice of penalty functions (Chalise and Fridley, 2012), such as Huber loss, Tukey loss, or
Publicly available datasets were analyzed in this study. This data can be found here: Genotypes and Phenotypes (https://www.ncbi.nlm.nih.gov/gap) with the study accession number phs001204. v1. p1.
The studies involving humans were approved by Mayo Clinic’s Institutional Review Board. The studies were conducted in accordance with the local legislation and institutional requirements. Written informed consent for participation was not required from the participants or the participants and apos; legal guardians/next of kin in accordance with the national legislation and institutional requirements.
LD: Data curation, Formal Analysis, Writing–original draft. YT: Formal Analysis, Methodology, Writing–original draft. XZ: Conceptualization, Supervision, Writing–review and editing. JC: Conceptualization, Supervision, Writing–review and editing.
The author(s) declare that financial support was received for the research, authorship, and/or publication of this article. The authors gratefully acknowledge National Institute of Health R01GM144351 (JC & XZ), National Science Foundation DMS1830392, DMS2113359, DMS1811747 (XZ) and National Science Foundation DMS2113360 and Mayo Clinic Center for Individualized Medicine (JC).
The author(s) declared that they were an editorial board member of Frontiers, at the time of submission. This had no impact on the peer review process and the final decision.
All claims expressed in this article are solely those of the authors and do not necessarily represent those of their affiliated organizations, or those of the publisher, the editors and the reviewers. Any product that may be evaluated in this article, or claim that may be made by its manufacturer, is not guaranteed or endorsed by the publisher.
The Supplementary Material for this article can be found online at: https://www.frontiersin.org/articles/10.3389/fgene.2024.1489694/full#supplementary-material
Aitchison, J. (1982). The statistical analysis of compositional data. J. R. Stat. Soc. Ser. B Methodol. 44, 139–160. doi:10.1111/j.2517-6161.1982.tb01195.x
Aitchison, J., and Bacon-Shone, J. (1984). Log contrast models for experiments with mixtures. Biometrika 71, 323–330. doi:10.1093/biomet/71.2.323
Akaho, S. (2001). “A kernel method for canonical correlation analysis,” in International meeting of psychometric society, 1.
Andrew, G., Arora, R., Bilmes, J., and Livescu, K. (2013). “Deep canonical correlation analysis,” in International conference on machine learning (Atlanta, GA: PMLR), 1247–1255.
Chalise, P., and Fridley, B. L. (2012). Comparison of penalty functions for sparse canonical correlation analysis. Comput. Statistics and Data Analysis 56, 245–254. doi:10.1016/j.csda.2011.07.012
Chen, J., Bushman, F. D., Lewis, J. D., Wu, G. D., and Li, H. (2013). Structure-constrained sparse canonical correlation analysis with an application to microbiome data analysis. Biostatistics 14, 244–258. doi:10.1093/biostatistics/kxs038
Chen, J., and Li, H. (2013). Variable selection for sparse Dirichlet-multinomial regression with an application to microbiome data analysis. Ann. Appl. Statistics 7. doi:10.1214/12-AOAS592
Chen, X., Han, L., and Carbonell, J. (2012). Structured sparse canonical correlation analysis. Proc. Fifteenth Int. Conf. Artif. Intell. Statistics 22, 199–207.
Chu, D., Liao, L.-Z., Ng, M. K., and Zhang, X. (2013). Sparse canonical correlation analysis: new formulation and algorithm. IEEE Trans. Pattern Analysis Mach. Intell. 35, 3050–3065. doi:10.1109/TPAMI.2013.104
Cox, T. O., Lundgren, P., Nath, K., and Thaiss, C. A. (2022). Metabolic control by the microbiome. Genome Med. 14, 80. doi:10.1186/s13073-022-01092-0
Friedman, J., and Alm, E. (2012). Inferring correlation networks from genomic survey data. PLoS Comput. Biol. 8, e1002687. doi:10.1371/journal.pcbi.1002687
Fukumizu, K., Bach, F. R., and Gretton, A. (2007). Statistical consistency of kernel canonical correlation analysis. J. Mach. Learn. Res. 8.
Hale, V. L., Chen, J., Johnson, S., Harrington, S. C., Yab, T. C., Smyrk, T. C., et al. (2017). Shifts in the fecal microbiota associated with adenomatous polyps. Cancer Epidemiol. Prev. Biomarkers 26, 85–94. doi:10.1158/1055-9965.EPI-16-0337EPI-16-0337
Hardoon, D. R., and Shawe-Taylor, J. (2011). Sparse canonical correlation analysis. Mach. Learn. 83, 331–353. doi:10.1007/s10994-010-5222-7
Hasin, Y., Seldin, M., and Lusis, A. (2017). Multi-omics approaches to disease. Genome Biol. 18, 83. doi:10.1186/s13059-017-1215-1s13059-017-1215-1
Hotelling, H. (1936). Relations between two sets of variates. Biometrika 28, 321–377. doi:10.1093/biomet/28.3-4.321
Jiang, R., Li, W. V., and Li, J. J. (2021). mbImpute: an accurate and robust imputation method for microbiome data. Genome Biol. 22, 192. doi:10.1186/s13059-021-02400-4
Jiang, S., Xiao, G., Koh, A. Y., Kim, J., Li, Q., and Zhan, X. (2019). A bayesian zero-inflated negative binomial regression model for the integrative analysis of microbiome data. Biostatistics 22, 522–540. doi:10.1093/biostatistics/kxz050
Kettenring, J. R. (1971). Canonical analysis of several sets of variables. Biometrika 58, 433–451. doi:10.1093/biomet/58.3.433
Kim, M., Vogtmann, E., Ahlquist, D. A., Devens, M. E., Kisiel, J. B., Taylor, W. R., et al. (2020). Fecal metabolomic signatures in colorectal adenoma patients are associated with gut microbiota and early events of colorectal cancer pathogenesis. Mbio 11, e03186. doi:10.1128/mbio.03186-19
Koendjbiharie, J. G., Wiersma, K., and van Kranenburg, R. (2018). Investigating the central metabolism of Clostridium thermosuccinogenes. Appl. Environ. Microbiol. 84, e00363-18. doi:10.1128/AEM.00363-18
Lancaster, H. O. (1958). The structure of bivariate distributions. Ann. Math. Statistics 29, 719–736. doi:10.1214/aoms/1177706532
Lê Cao, K.-A., Martin, P. G., Robert-Granié, C., and Besse, P. (2009). Sparse canonical methods for biological data integration: application to a cross-platform study. BMC Bioinforma. 10, 34–17. doi:10.1186/1471-2105-10-34
Lin, D., Zhang, J., Li, J., Calhoun, V. D., Deng, H.-W., and Wang, Y.-P. (2013). Group sparse canonical correlation analysis for genomic data integration. BMC Bioinforma. 14, 245. doi:10.1186/1471-2105-14-245
Lin, H., and Peddada, S. D. (2020). Analysis of compositions of microbiomes with bias correction. Nat. Commun. 11, 3514. doi:10.1038/s41467-020-17041-7
Lin, W., Shi, P., Feng, R., and Li, H. (2014). Variable selection in regression with compositional covariates. Biometrika 101, 785–797. doi:10.1093/biomet/asu031
Lindenbaum, O., Salhov, M., Averbuch, A., and Kluger, Y. (2022). “l0-sparse canonical correlation analysis,” in International conference on learning representations.
Lloyd-Price, J., Arze, C., Ananthakrishnan, A. N., Schirmer, M., Avila-Pacheco, J., Poon, T. W., et al. (2019). Multi-omics of the gut microbial ecosystem in inflammatory bowel diseases. Nature 569, 655–662. doi:10.1038/s41586-019-1237-9
Luo, L., Wang, W., Bao, S., Peng, X., and Peng, Y. (2024). Robust and sparse canonical correlation analysis for fault detection and diagnosis using training data with outliers. Expert Syst. Appl. 236, 121434. doi:10.1016/j.eswa.2023.121434
Michaeli, T., Wang, W., and Livescu, K. (2016). “Nonparametric canonical correlation analysis,” in International conference on machine learning (New York, NY: PMLR), 48, 1967–1976.
Mohammadi-Nejad, A.-R., Hossein-Zadeh, G.-A., and Soltanian-Zadeh, H. (2017). Structured and sparse canonical correlation analysis as a brain-wide multi-modal data fusion approach. IEEE Trans. Med. Imaging 36, 1438–1448. doi:10.1109/TMI.2017.2681966
Parkhomenko, E., Tritchler, D., and Beyene, J. (2007). Genome-wide sparse canonical correlation of gene expression with genotypes. BMC Proc. 1, S119. doi:10.1186/1753-6561-1-S1-S119
Parkhomenko, E., Tritchler, D., and Beyene, J. (2009). Sparse canonical correlation analysis with application to genomic data integration. Stat. Appl. Genet. Mol. Biol. 8, Article 1. doi:10.2202/1544-6115.1406
Sepich-Poore, G. D., Zitvogel, L., Straussman, R., Hasty, J., Wargo, J. A., and Knight, R. (2021). The microbiome and human cancer. Science 371, eabc4552. doi:10.1126/science.abc4552
Wensel, C. R., Pluznick, J. L., Salzberg, S. L., and Sears, C. L. (2022). Next-generation sequencing: insights to advance clinical investigations of the microbiome. J. Clin. Investigation 132, e154944. doi:10.1172/JCI154944
Witten, D. M., Tibshirani, R., and Hastie, T. (2009). A penalized matrix decomposition, with applications to sparse principal components and canonical correlation analysis. Biostatistics 10, 515–534. doi:10.1093/biostatistics/kxp008
Yang, L., and Chen, J. (2022). A comprehensive evaluation of microbial differential abundance analysis methods: current status and potential solutions. Microbiome 10, 130. doi:10.1186/s40168-022-01320-0
Yoon, G., Gaynanova, I., and Müller, C. L. (2019). Microbial networks in spring-semi-parametric rank-based correlation and partial correlation estimation for quantitative microbiome data. Front. Genet. 10, 516. doi:10.3389/fgene.2019.00516
Yu, J.-L., Qian, Z.-G., and Zhong, J.-J. (2018). Advances in bio-based production of dicarboxylic acids longer than C4. Eng. Life Sci. 18, 668–681. doi:10.1002/elsc.201800023
Zeng, Y., Li, J., Wei, C., Zhao, H., and Wang, T. (2022). mbDenoise: microbiome data denoising using zero-inflated probabilistic principal components analysis. Genome Biol. 23, 94. doi:10.1186/s13059-022-02657-3
Keywords: canonical correlation analysis, compositional effect, phylogenetic tree, structural information, dimension reduction, variable selection
Citation: Deng L, Tang Y, Zhang X and Chen J (2024) Structure-adaptive canonical correlation analysis for microbiome multi-omics data . Front. Genet. 15:1489694. doi: 10.3389/fgene.2024.1489694
Received: 01 September 2024; Accepted: 31 October 2024;
Published: 20 November 2024.
Edited by:
Weihua Guan, University of Minnesota Twin Cities, United StatesReviewed by:
Qiwei Li, The University of Texas at Dallas, United StatesCopyright © 2024 Deng, Tang, Zhang and Chen. This is an open-access article distributed under the terms of the Creative Commons Attribution License (CC BY). The use, distribution or reproduction in other forums is permitted, provided the original author(s) and the copyright owner(s) are credited and that the original publication in this journal is cited, in accordance with accepted academic practice. No use, distribution or reproduction is permitted which does not comply with these terms.
*Correspondence: Xianyang Zhang, emhhbmd4aWFueUBzdGF0LnRhbXUuZWR1; Jun Chen, Y2hlbi5qdW4yQG1heW8uZWR1
†These authors have contributed equally to this work
Disclaimer: All claims expressed in this article are solely those of the authors and do not necessarily represent those of their affiliated organizations, or those of the publisher, the editors and the reviewers. Any product that may be evaluated in this article or claim that may be made by its manufacturer is not guaranteed or endorsed by the publisher.
Research integrity at Frontiers
Learn more about the work of our research integrity team to safeguard the quality of each article we publish.