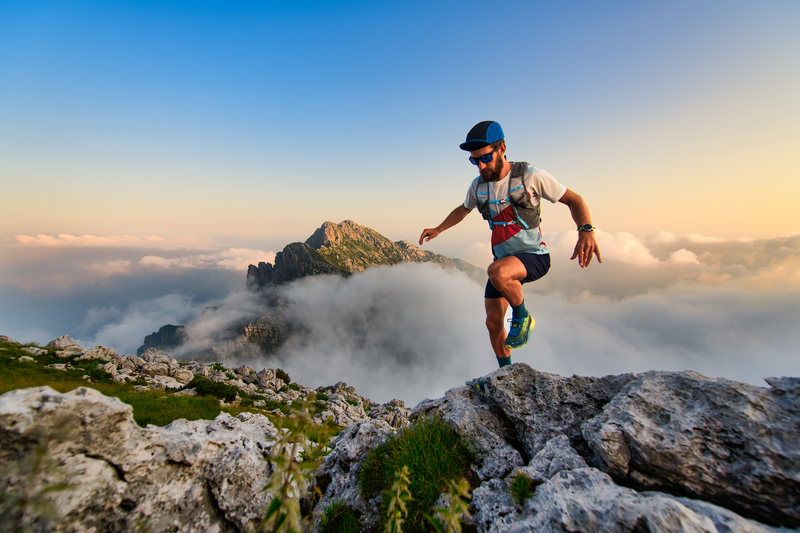
94% of researchers rate our articles as excellent or good
Learn more about the work of our research integrity team to safeguard the quality of each article we publish.
Find out more
ORIGINAL RESEARCH article
Front. Genet. , 08 October 2024
Sec. Behavioral and Psychiatric Genetics
Volume 15 - 2024 | https://doi.org/10.3389/fgene.2024.1480761
This article is part of the Research Topic Methylation in the Human Brain View all 4 articles
Mathematical algorithms known as “epigenetic clocks” use methylation values at a set of CpG sites to estimate the biological age of an individual in a tissue-specific manner. These clocks have demonstrated both acceleration and delays in epigenetic aging in multiple neuropsychiatric conditions, including schizophrenia and neurodevelopmental disorders such as autism spectrum disorder. However, no study to date has examined epigenetic aging in ADHD despite its status as one of the most prevalent neurodevelopmental conditions, with 1 in 9 children having ever received an ADHD diagnosis in the US. Only a handful of studies have examined epigenetic age in brain tissue from neurodevelopmental conditions, with none focused on ADHD, despite the obvious relevance to pathogenesis. Thus, here we asked if post-mortem brain tissue in those with lifetime histories of ADHD would show accelerated or delayed epigenetic age, as has been found for other neurodevelopmental conditions. We applied four different epigenetic clocks to estimate epigenetic age in individuals with ADHD and unaffected controls from cortical (anterior cingulate cortex, N = 55) and striatal (caudate, N = 56) post-mortem brain tissue, as well as peripheral blood (N = 84) and saliva (N = 112). After determining which epigenetic clock performed best in each tissue, we asked if ADHD was associated with altered biological aging in corticostriatal brain and peripheral tissues. We found that a range of epigenetic clocks accurately predicted chronological age in all tissues. We also found that a diagnosis of ADHD was not significantly associated with differential epigenetic aging, neither for the postmortem ACC or caudate, nor for peripheral tissues. These findings held when accounting for comorbid psychiatric diagnoses, substance use, and stimulant medication. Thus, in this study of epigenetic clocks in ADHD, we find no evidence of altered epigenetic aging in corticostriatal brain regions nor in peripheral tissue. We consider reasons for this unexpected finding, including the limited sampling of brain regions, the age range of individuals studied, and the possibility that processes that accelerate epigenetic age may be counteracted by the developmental delay posited in some models of ADHD.
Alterations of the intrinsic biological aging process have been shown to be a feature of neuropsychiatric disorders. A widely used method for estimating biological age is to examine variations in the methylation of CpG sites with age (Horvath and Raj, 2018). Such “epigenetic clocks” are mathematical algorithms that take a linear combination of methylation values at a set of specific CpG methylation sites to estimate age (Field et al., 2018). Given that changes in the methylome are tissue specific, multiple epigenetic clocks have been trained on different tissue types (Bergsma and Rogaeva, 2020; Field et al., 2018; Horvath, 2013; McEwen et al., 2020; Shireby et al., 2020; Zhang et al., 2019), with most studies using peripheral tissues such as whole blood and saliva to estimate epigenetic age (Shenk et al., 2021; Wolf et al., 2019; Han et al., 2018; Protsenko et al., 2021; McEwen et al., 2020). However, studies focusing on changes in epigenetic markers in brain tissues are particularly important to advance our understanding of neuropsychiatric disorders.
Prior studies using post-mortem brain tissue have demonstrated acceleration of brain epigenetic aging in major depressive disorder (Han et al., 2018), bipolar disorder (Fries et al., 2020), and substance use disorders (Rosen et al., 2018; Poisel et al., 2023; Zillich et al., 2024). Studies in schizophrenia found delayed epigenetic aging in the cerebellum and frontal cortex (McKinney et al., 2017; AuthorAnonymous, 2018; Voisey et al., 2017; Liu et al., 2023; Teeuw et al., 2021; Wu et al., 2021). Among neurodevelopmental conditions, one study has demonstrated accelerated aging in ASD restricted to the cerebellum of older adults (Liu et al., 2023). Here we ask if a similar phenomenon applies to another neurodevelopmental condition, attention-deficit hyperactivity disorder (ADHD), which is characterized by impairing, age-inappropriate symptoms of inattention, hyperactivity, and impulsivity (Faraone et al., 2015). A prior study found increased ADHD genetic burden as measured by polygenic score to be associated with advanced epigenetic age in blood (Arpawong et al., 2023), and another study applied BrainAGE, a machine learning algorithm trained on structural neuroimaging data, to demonstrate delayed age in children with ADHD (Kurth et al., 2022). Importantly, no prior study to date has examined epigenetic aging in post-mortem brain tissue in ADHD. We focus on the caudate and anterior cingulate cortex (ACC), as neuroimaging studies have demonstrated subtle changes to their structure and function in ADHD (Hoogman et al., 2019; Hoogman et al., 2017; Hart et al., 2013; Yap, Abdul Manan, and Sharip, 2021). Further, the caudate and ACC are key components of corticostriatal circuitry that support cognitive processes pertinent to ADHD such inhibitory processing (Hart et al., 2013). Finally, the ACC and caudate are representative of cortical and subcortical regions, respectively, that could potentially capture alterations related to ADHD trajectory with age (Shaw and Sudre, 2021).
What alterations to epigenetic aging might be found in the brain in ADHD? We consider three possibilities. First, ADHD may be tied to accelerated biological aging in the brain, analogous to the shifts seen in other neurodevelopmental conditions such as ASD (Liu et al., 2023; Teeuw et al., 2021). Such accelerated epigenetic aging has been previously tied to the cumulative stresses of living, for example, with a neurodevelopmental challenge such as ADHD (Zannas et al., 2015). Secondly, ADHD, which is sometimes held to stem from developmental immaturity or delays, could be tied to a younger biological age–an instance of epigenetic age deceleration. Evidence for developmental immaturity has come from in vivo neuroimaging studies, including neuroanatomic (Muetzel et al., 2018; Shaw et al., 2013), functional connectivity (Francx et al., 2015; Clerkin et al., 2013; Schulz et al., 2017), and electrophysiological indices (Michelini et al., 2016; Cheung et al., 2016; Michelini et al., 2019). Finally, different brain regions may show different epigenetic ages. This proposition rests upon a model that ties the onset of ADHD to changes in the prenatal and infantile deep structures, such as the caudate, whereas the course of the disorder into adolescence and beyond is linked to prefrontal cortical plasticity, such as in the anterior cingulate cortex (Halperin and Kurt, 2006; Shaw and Sudre, 2021; Schulz et al., 2017). This model would suggest that the caudate and ACC might have different epigenetic ages, aligned with the different cognitive and neural changes occurring in these regions in ADHD.
In this study, we first determined which epigenetic clock algorithm(s) most accurately predicted chronological age in individuals with no lifetime psychiatric disorders. Next, we use the best performing clocks to determine if a diagnosis of ADHD is associated with altered epigenetic age in the brain. Finally, we ask if our findings for brain tissue hold in peripheral tissues (blood, saliva) obtained from a different cohort of youth with ADHD.
Postmortem brain tissue was obtained from three brain banks: the NIMH Human Brain Collection Core, the Maryland Brain Collection, and the University of Pittsburgh Brain Tissue Donation Program. Anterior cingulate cortex and/or caudate tissue was obtained from 26 individuals with ADHD and 33 individuals with no psychiatric diagnoses (see Table 1). For the NIMH HBCC and Maryland Brain Collection, postmortem diagnostic interviews were performed with at least one family member of the deceased by a trained psychiatric social worker using the Structured Clinical Interview for the DSM-IV (SCID) and Diagnostic Evaluation After Death (DEAD). Two psychiatrists then reviewed the case write-up and arrived at a consensus for a possible DSM diagnosis, and in the case of the Maryland Brain Collection, medical records as well as records from the medical examiner’s office all contributed to the diagnosis (Donati et al., 2008). The University of Pittsburgh program similarly conducts a postmortem interview with the family of the deceased using a structured interview for the DSM-5, and this information is then reviewed during a consensus conference of psychiatrists and psychologists along with any medical records and publicly available records to arrive at a DSM diagnosis (Department of PsychiatryUniversity of Pittsburgh, 2020). Unaffected controls had no psychiatric disorders during the consensus diagnostic process. Race data was not available for one donor, so ACC and caudate tissue from this individual was excluded from the linear regression analysis where race was a covariate.
Blood and saliva samples were obtained from a separate cohort based at the NIH Intramural Research Program in a study approved by the NIH central IRB. Individuals with no psychiatric diagnoses were contrasted against those with a diagnosis of ADHD, as determined by the clinician-administered Diagnostic Interview for Children and Adolescents-Fourth Edition (DICA-IV) with the parent. For adults aged 18 or over, diagnoses were made using the Connors’ Adult ADHD Diagnostic Interview for DSM-IV (CAADID; Epstein et al., 2001) and Structured Clinical Interview for DSM-IV Axis I Disorders (SCID). The main exclusion criteria were full scale IQ less than 70, birth before 32 weeks gestation, neurological disorder impacting brain structure, and psychoses. All adults and parents provided written informed consent, and written assent was obtained from children under 18. After methylation quality control, the final cohort included saliva specimens from 112 participants and peripheral whole blood specimens from 84 participants (see Table 1). There were 17 individuals who provided both saliva and blood. All tissues were from unrelated individuals.
Anterior cingulate cortex and caudate tissues were dissected from coronal slabs, which were prepared and frozen at −80°C during autopsy. DNA extraction and bisulfite conversion was performed on bulk homogenates of the dissected tissues using the EZ DNA Methylation Kit (Zymo Research, Irvine, CA, United States). In whole blood, DNA extraction and bisulfite conversion was performed using the EZ Methylation-Gold Kit (Zymo Research), and saliva was collected using the Oragene DNA collection Kit (Genotek).
All biospecimens were processed using the Infinium HumanMethylationEPIC BeadChip (Illumina, San Diego, CA), performed by the Genomics Core at the National Human Genome Research Institute (NHGRI). Methylation data was separated based on tissue type and processed using the minfi (Aryee et al., 2014), wateRmelon (Pidsley et al., 2013), and ChAMP (Tian et al., 2017) packages in R. Quality control involved removal of samples with incorrect predicted sex based on methylation values, poor quality based on plots of the log median intensity of methylated and unmethylated signals, mean detection p-value greater than or equal to 0.05, and bisulfite conversion rate less than or equal to 80%. These steps resulted in the removal of 0 brain samples, 0 blood samples, and 24 saliva samples. Probes with a detection p-value greater than or equal to 0.01 in any sample or bead count <3 in at least 5% of samples were removed, and only autosomal probes were considered. Methylation values were normalized using the Noob normalization method and were not batch-corrected, both as suggested by the authors of the Horvath Online Age Calculator (https://dnamage.genetics.ucla.edu) (Horvath, 2013). The final beta matrices were 827,701 CpG probes x 56 caudate specimens, 827,891 CpG probes x 55 ACC specimens, 828,686 CpG probes x 84 blood specimens, and 827,891 CpG probes x 112 saliva specimens.
Four epigenetic clocks were included in this study: the Cortical Clock trained on brain tissue by Shireby et al (Shireby et al., 2020), the DNAm multi-tissue clock by Horvath et al (Horvath, 2013), a blood and saliva-derived clock by Zhang et al that utilizes two different methods for age estimation (Zhang et al., 2019), and the PedBE clock trained on pediatric buccal epithelial cells by McEwen et al (McEwen et al., 2020). These clocks were selected because they were trained on cohorts with age ranges and tissue types (post-mortem brain, blood, or saliva) most similar to our study samples. Beta values were submitted to the Horvath Online Age Calculator, and the “Advanced Analysis” option was selected for blood samples to obtain immune cell proportion estimates based on the Horvath and Houseman methods (Horvath, 2013; Houseman et al., 2012). The remaining epigenetic age estimates were obtained using publicly available code published by the respective clock authors. The most accurate clock was selected for each tissue type by finding the lowest mean absolute difference between epigenetic and chronological age in unaffected (non-ADHD) individuals.
We examined the difference between chronological and epigenetic age in ADHD individuals versus unaffected individuals in the two brain regions separately. In this comparison, positive values indicate advanced epigenetic age, while negative values indicate younger epigenetic age as compared to chronological age. All regression analyses adjusted for the following technical factors: plate on which samples were processed during the EPIC methylation array procedure (“sample group”) and array scanner used to survey methylation sites. Analysis of brain tissue included additional technical covariates (brain bank of origin, post-mortem interval), neuronal proportion estimated using the R package CETYGO (selecting the most accurate estimates based on the CETYGO “score”) (Vellame et al., 2023; Hannon et al., 2024), and whether the manner of death was by suicide versus all other modes of death. The ADHD and unaffected groups did not differ significantly in proportion of sex assigned at birth (see Table 1) but did differ significantly in proportion of those of different race/ethnicity, and we adjusted for both these demographic factors. Mean chronological age did not differ significantly between groups for the post-mortem analyses but was also included as a covariate as suggested by the Horvath tutorial.
We tested the hypothesis that different brain regions would show different epigenetic aging using a mixed model regression, first testing for an interaction between diagnosis and brain region and including a random intercept term for donor identity. Brain tissue analyses were repeated with a subset of individuals with no comorbid psychiatric diagnoses (N = 45 for ACC and N = 47 for caudate), and with a subset of individuals without documented substance use (N = 42 for ACC and N = 44 for caudate, see Table 1).
Analyses of peripheral tissue were conducted in a similar manner, adjusting for relevant technical variables (methylation array plate and scanner) as well as race/ethnicity, sex assigned at birth, and chronological age. In blood analyses, there was further adjustment for immune cells known to be influenced by chronological age. Per the Horvath Online Age Calculator tutorial (Horvath, 2013), the included cell types were naïve CD8+ T cells, CD8+CD28-CD45RA- T cells, plasmablasts, CD4+ T cells, natural killer cells, monocytes, and granulocytes (see Models S1, S2). Cell abundance measures were generated using the Horvath Online Age Calculator’s “Advanced Analysis” option. For robustness, peripheral sample analyses were repeated with stimulant use as a covariate and again with only individuals with no comorbid psychiatric diagnoses (N = 76 for blood and N = 100 for saliva).
We first determined which of the five epigenetic clock methods most accurately predicted chronological age in the brain regions, considering only the individuals with no lifetime history of psychiatric disorders. We found that the Zhang et al. elastic net method produced the lowest mean absolute age difference between predicted and actual age in both the ACC [absolute mean 2.6 years (SD 2.35 years)] and caudate [absolute mean 3.36 years (SD 2.26 years)]—see Table 2, Figure 1. For the caudate, this clock provided estimates of epigenetic age that were a mean of 0.97 years (SD 3.97) less than the chronological age; for the ACC, the epigenetic age was a mean of 1.00 years (SD 3.39 years) more than chronological age. The performance of the other clocks is shown in Table 2.
Figure 1. Epigenetic Clock Comparison: Plot of epigenetic versus chronological age for ACC and Caudate samples from unaffected (non-ADHD) individuals. Predicted ages are color-coded based on the clock used to generate the ages. The black line represents the line of perfect prediction (y = x) for reference.
As is illustrated in Figure 2, there was no diagnostic effect on the difference between epigenetic and chronological age for the caudate (t = −0.13, p = 0.90). Among those with ADHD, epigenetic age slightly underestimated chronological age in the caudate (mean −0.85, SD 2.85 years), similar to the underestimation observed in the unaffected group as noted above (mean −0.97, SD 3.97 years). For the ACC, epigenetic age among those with ADHD was again close to chronological age (mean 0.26, SD 3.22 years), and this did not differ significantly (t = 0.83, p = 0.41), from estimates for the unaffected group (mean 1.00, SD 3.39 years).
Figure 2. Comparison of age difference (epigenetic age–chronological age) in participants with ADHD versus unaffected volunteers in post-mortem ACC and caudate using the Zhang EN clock. Positive age difference indicates advanced epigenetic age.
We next asked if there were differences in epigenetic aging between the two brain regions using mixed model linear regression, adjusting for covariates as described in the methods. We found no significant interaction between brain region and diagnosis in association with the age prediction error (beta = 0.60, t = 0.65, p = 0.52).
All findings held for analyses confined to those with no comorbid psychiatric diagnosis and when we excluded those with known substance use (Supplementary Table S1)
We asked if a similar pattern of results would be found for peripheral tissues. The Horvath multi-tissue clock performed best in predicting epigenetic age in unaffected individuals for both blood (mean absolute age difference 2.02 years [SD 1.52]) and saliva (mean absolute age difference 1.31 years [SD 1.12])—see Figure 3, Table 2.
Figure 3. Epigenetic clock comparison and comparison of age prediction accuracy in peripheral tissues. Top two panels show epigenetic versus chronological age for blood (left) and saliva (right) samples from unaffected (non-ADHD) individuals. The black line represents the line of perfect prediction (y = x) for reference. The bottom two panels show the comparison of age difference (epigenetic age–chronological age) in participants with ADHD versus unaffected volunteers in blood (left) and saliva (right) using the Horvath multi-tissues clock. Positive age difference indicates advanced epigenetic age.
Using the Horvath clock, for those with ADHD, epigenetic age predicted chronological age well in saliva (mean difference −0.05, SD 1.90 years). This did not differ significantly from the unaffected group (mean difference 0.02 years, SD 1.74, t = 0.22, p = 0.82). In blood from ADHD individuals, epigenetic age was a little over a year greater than chronological age (mean difference 1.20, SD 1.90 years), which did not significantly differ from estimates in the unaffected individuals (mean difference 1.51 years, SD 2.03, t = 0.73, p = 0.47), indicating a similar overestimation–see Figure 3. Findings held when stimulant use was included as a covariate, and when analyses were restricted to those without comorbid psychiatric diagnoses—see Supplementary Table S2.
This is the first study to examine epigenetic aging in the brain of those with lifetime histories of ADHD. There were three central findings. First, we find that epigenetic clocks trained on peripheral tissue performed as well as, if not better than, clocks trained on brain tissue in estimating brain epigenetic age. Second, we find no evidence of significantly altered epigenetic aging in either the post-mortem anterior cingulate cortex or caudate of individuals with lifetime histories of ADHD. Finally, there are no ADHD-related differences in epigenetic aging in peripheral blood or saliva obtained from a separate cohort of individuals.
This study reinforces the validity of epigenetic clock algorithms in predicting chronological age across tissue types. It is noteworthy that the Zhang elastic-net clock most closely predicted chronological age in brain tissue from unaffected individuals, as this clock was initially trained on blood and saliva-derived methylation data. This suggests some degree of similarity between peripheral methylation changes and those found in the brain. This finding is consistent with a study by Cabrera-Mendoza et al. (Cabrera-Mendoza et al., 2023) which found significant positive correlations between blood and brain epigenetic age in healthy controls across multiple epigenetic clocks. Another consideration is differences in neuropathological load, as the age range of our post-mortem cohort is younger on average than the training cohort of the Cortical Clock by Shireby et al. Prior work has demonstrated that neurodegenerative pathology including neuritic plaques and amyloid load is associated with advanced epigenetic age (Levine et al., 2015). While the Cortical Clock excluded individuals with a known history of Alzheimer’s disease or other major neurological phenotypes, such histological findings can exist without clinical diagnosis and may skew the model. Finally, the PedBE clock by McEwen et al. performed the poorest in predicting chronological age in unaffected controls. This was also the only clock of those selected in this study to be trained exclusively on buccal epithelial cells from a pediatric cohort, potentially limiting its generalizability.
We found no evidence for altered epigenetic aging in ADHD, contrary to other neurodevelopmental conditions (Liu et al., 2023; Wu et al., 2021). There are several possible explanations. First, we examined only the caudate and ACC, while shifts in epigenetic age may lie in other brain regions. Such regional specificity in brain epigenetic patterns has been reported for other disorders. For example, Liu et al. (2023) demonstrated advanced epigenetic age in ASD in post-mortem cerebellar tissue of adults older than 45 years, but not in the prefrontal cortex or temporal cortex. The same study also found delayed epigenetic age in the post-mortem cerebellar tissue from adults with a diagnosis of schizophrenia aged 50–70 years, but not in the striatum or hippocampus. Secondly, there may be developmental effects. For example, studies suggest that there is delayed epigenetic aging in the frontal cortex among those with schizophrenia aged between 20 and 39 years, but not for those aged 60–90 years, though it is unclear whether neurodegenerative histopathology was fully accounted for in this work (Wu et al., 2021). Thus, it is possible that we might have found altered epigenetic aging if we had examined younger or older donors. Third, we used whole brain tissue homogenate, and methylation changes may be cell-specific. For example, several studies find accelerated epigenetic age to correlate with the proportion of non-neuronal cells, including oligodendrocytes and microglia, in the brain tissue samples used (Shireby et al., 2020; Stevenson et al., 2022; Murthy et al., 2023). However, we found no association between neuronal proportion and epigenetic age prediction accuracy in the present study. A final consideration is that ADHD may combine components of accelerated epigenetic aging, through the stress of living with a mental health challenge, with components of delayed epigenetic aging due to developmental immaturity—with these antagonistic processes “cancelling” one another out. Related to this possibility is the “last in, first out” hypothesis, developed based on P3 event-related potential data in ADHD, which proposes that the same brain regions with delayed maturation will also develop accelerated degeneration over time (Kakuszi et al., 2020).
There are several limitations to consider. First, the study’s biospecimens were obtained from different cohorts with no overlap between postmortem brain donors (ACC, caudate) and peripheral sample donors (blood, saliva). Our study samples were derived from a majority of white, non-Hispanic males, limiting the generalizability of our results. Additionally, the ADHD cohort included individuals with other comorbid psychiatric conditions as well as patients taking psychotropic medication. However, previous findings held when post-mortem brain analysis was repeated with a subset of individuals with no comorbid psychiatric illness, and again with the subset of individuals with no known substance use. Similarly, peripheral findings remained consistent when accounting for stimulant use or restricted to individuals with no comorbid psychiatric diagnoses.
In summary, we find no evidence of altered epigenetic aging tied to a diagnosis of ADHD in post-mortem ACC or caudate tissues, nor in peripheral blood or saliva. Future work might look at cell specific change, other brain regions, and a wider age range of individuals.
The data presented in the study are deposited in the NIMH Data Archive, Collection 3151, Experiment 2443: https://nda.nih.gov/edit_collection.html?id=3151.
All study sites that acquired post-mortem tissue used in the study conducted all related procedures under protocols approved by their local IRB. Procedures at the NIMH HBCC are approved by an oversight committee and the NIH Department of Bioethics. Blood and saliva samples were obtained under a IRB protocol at NIH in which all participants provided written informed consent and/or assent that was obtained using institutional review board–approved procedures.
GaS: Conceptualization, Formal Analysis, Investigation, Methodology, Visualization, Writing–original draft, Writing–review and editing, Data curation. GuS: Conceptualization, Data curation, Writing–review and editing. KA: Data curation, Writing–review and editing. BJ: Data curation, Writing–review and editing. BK: Data curation, Supervision, Writing–review and editing. PA: Writing–review and editing. LE: Supervision, Writing–review and editing. PS: Writing–original draft, Writing–review and editing, Conceptualization, Funding acquisition, Resources, Supervision.
The author(s) declare that financial support was received for the research, authorship, and/or publication of this article. Open access funding was provided by the National Institutes of Health. The study was funded by the intramural programs of the NIMH and NHGRI (ZIC MH002903-15 and ZIA HG200378-10).
We acknowledge sites within the NIH funded Neurobiobank for the provision of tissues, specifically the University of Pittsburgh and Maryland Brain Collection. We acknowledge the Human Brain Collection Core for preparing DNA for epigenotyping, and Chandrasekharappa Settara and Frank Donovan at the Genomics Core for genotyping and running the methylation arrays.
The authors declare that the research was conducted in the absence of any commercial or financial relationships that could be construed as a potential conflict of interest.
All claims expressed in this article are solely those of the authors and do not necessarily represent those of their affiliated organizations, or those of the publisher, the editors and the reviewers. Any product that may be evaluated in this article, or claim that may be made by its manufacturer, is not guaranteed or endorsed by the publisher.
The Supplementary Material for this article can be found online at: https://www.frontiersin.org/articles/10.3389/fgene.2024.1480761/full#supplementary-material
Arpawong, T. E., Klopack, E. T., Kim, J. K., and Crimmins, E. M. (2023). ADHD genetic burden associates with older epigenetic age: mediating roles of education, behavioral and sociodemographic factors among older adults. Clin. Epigenetics 15 (1), 67. doi:10.1186/s13148-023-01484-yAvailable at: https://www.ncbi.nlm.nih.gov/pubmed/37101297.
Aryee, M. J., Jaffe, A. E., Corrada-Bravo, H., Ladd-Acosta, C., Feinberg, A. P., Hansen, K. D., et al. (2014). Minfi: a flexible and comprehensive Bioconductor package for the analysis of Infinium DNA methylation microarrays. Bioinformatics 30 (10), 1363–1369. doi:10.1093/bioinformatics/btu049Available at: https://www.ncbi.nlm.nih.gov/pubmed/24478339.
AuthorAnonymous, , Lin, H., Ding, Y., Lewis, D. A., and Sweet, R. A. (2018). DNA methylation age is not accelerated in brain or blood of subjects with schizophrenia. Schizophr. Res. 196, 39–44. doi:10.1016/j.schres.2017.09.025Available at: https://www.ncbi.nlm.nih.gov/pubmed/28988914.
Bergsma, T., and Rogaeva, E. (2020). DNA methylation clocks and their predictive capacity for aging phenotypes and healthspan. Neurosci. Insights 15, 2633105520942221. doi:10.1177/2633105520942221Available at: https://www.ncbi.nlm.nih.gov/pubmed/32743556.
Cabrera-Mendoza, B., Stertz, L., Najera, K., Selvaraj, S., Teixeira, A. L., Meyer, T. D., et al. (2023). Within subject cross-tissue analyzes of epigenetic clocks in substance use disorder postmortem brain and blood. Am. J. Med. Genet. B Neuropsychiatr. Genet. 192 (1-2), 13–27. doi:10.1002/ajmg.b.32920Available at: https://www.ncbi.nlm.nih.gov/pubmed/36056652.
Cheung, C. H., Rijsdijk, F., McLoughlin, G., Brandeis, D., Banaschewski, T., Asherson, P., et al. (2016). Cognitive and neurophysiological markers of ADHD persistence and remission. Br. J. Psychiatry 208 (6), 548–555. doi:10.1192/bjp.bp.114.145185Available at: https://www.ncbi.nlm.nih.gov/pubmed/26250744.
Clerkin, S. M., Schulz, K. P., Berwid, O. G., Fan, J., Newcorn, J. H., Tang, C. Y., et al. (2013). Thalamo-cortical activation and connectivity during response preparation in adults with persistent and remitted ADHD. Am. J. Psychiatry 170 (9), 1011–1019. doi:10.1176/appi.ajp.2013.12070880Available at: https://www.ncbi.nlm.nih.gov/pubmed/24030612.
Department of Psychiatry, University of Pittsburgh (2020). A unique approach to lifespan diagnosis: the psychological autopsy. Available at: https://psychiatry.pitt.edu/unique-approach-lifespan-diagnosis-psychological-autopsy.
Donati, R. J., Dwivedi, Y., Roberts, R. C., Conley, R. R., Pandey, G. N., and Rasenick, M. M. (2008). Postmortem brain tissue of depressed suicides reveals increased Gs alpha localization in lipid raft domains where it is less likely to activate adenylyl cyclase. J. Neurosci. 28 (12), 3042–3050. doi:10.1523/JNEUROSCI.5713-07.2008Available at: https://www.ncbi.nlm.nih.gov/pubmed/18354007.
Faraone, S. V., Asherson, P., Banaschewski, T., Biederman, J., Buitelaar, J. K., Ramos-Quiroga, J. A., et al. (2015). Attention-deficit/hyperactivity disorder. Nat. Rev. Dis. Prim. 1, 15020. doi:10.1038/nrdp.2015.20Available at: https://www.ncbi.nlm.nih.gov/pubmed/27189265.
Field, A. E., Robertson, N. A., Wang, T., Havas, A., Ideker, T., and Adams, P. D. (2018). DNA methylation clocks in aging: categories, causes, and consequences. Mol. Cell 71 (6), 882–895. doi:10.1016/j.molcel.2018.08.008Available at: https://www.ncbi.nlm.nih.gov/pubmed/30241605.
Francx, W., Oldehinkel, M., Oosterlaan, J., Heslenfeld, D., Hartman, C. A., Hoekstra, P. J., et al. (2015). The executive control network and symptomatic improvement in attention-deficit/hyperactivity disorder. Cortex 73, 62–72. doi:10.1016/j.cortex.2015.08.012Available at: https://www.ncbi.nlm.nih.gov/pubmed/26363140.
Fries, G. R., Bauer, I. E., Scaini, G., Valvassori, S. S., Walss-Bass, C., Soares, J. C., et al. (2020). Accelerated hippocampal biological aging in bipolar disorder. Bipolar Disord. 22 (5), 498–507. doi:10.1111/bdi.12876Available at: https://www.ncbi.nlm.nih.gov/pubmed/31746071.
Halperin, J. M., and Kurt, P. S. (2006). Revisiting the role of the prefrontal cortex in the pathophysiology of attention-deficit/hyperactivity disorder. Psychol. Bull. 132 (4), 560–581. doi:10.1037/0033-2909.132.4.560
Han, L. K. M., Aghajani, M., Clark, S. L., Chan, R. F., Hattab, M. W., Shabalin, A. A., et al. (2018). Epigenetic aging in major depressive disorder. Am. J. Psychiatry 175 (8), 774–782. doi:10.1176/appi.ajp.2018.17060595Available at: https://www.ncbi.nlm.nih.gov/pubmed/29656664.
Hannon, E., Dempster, E. L., Davies, J. P., Chioza, B., Blake, G. E. T., Burrage, J., et al. (2024). Quantifying the proportion of different cell types in the human cortex using DNA methylation profiles. BMC Biol. 22 (1), 17. doi:10.1186/s12915-024-01827-yAvailable at: https://www.ncbi.nlm.nih.gov/pubmed/38273288.
Hart, H., Radua, J., Nakao, T., Mataix-Cols, D., and Rubia, K. (2013). Meta-analysis of functional magnetic resonance imaging studies of inhibition and attention in attention-deficit/hyperactivity disorder: exploring task-specific, stimulant medication, and age effects. JAMA Psychiatry 70 (2), 185–198. doi:10.1001/jamapsychiatry.2013.277
Hoogman, M., Buitelaar, J. K., Faraone, S. V., Shaw, P., and Franke, B.ENIGMA-ADHD working group (2017). Subcortical brain volume differences in participants with attention deficit hyperactivity disorder in children and adults–Authors' reply. Lancet Psychiatry 4 (6), 440–441. doi:10.1016/S2215-0366(17)30200-6
Hoogman, M., Muetzel, R., Guimaraes, J. P., Shumskaya, E., Mennes, M., Zwiers, M. P., et al. (2019). Brain imaging of the cortex in ADHD: a coordinated analysis of large-scale clinical and population-based samples. Am. J. Psychiatry 176 (7), 531–542. doi:10.1176/appi.ajp.2019.18091033
Horvath, S. (2013). DNA methylation age of human tissues and cell types. Genome Biol. 14 (10), R115. doi:10.1186/gb-2013-14-10-r115Available at: https://www.ncbi.nlm.nih.gov/pubmed/24138928.
Horvath, S., and Raj, K. (2018). DNA methylation-based biomarkers and the epigenetic clock theory of ageing. Nat. Rev. Genet. 19 (6), 371–384. doi:10.1038/s41576-018-0004-3Available at: https://www.ncbi.nlm.nih.gov/pubmed/29643443.
Houseman, E. A., Accomando, W. P., Koestler, D. C., Christensen, B. C., Marsit, C. J., Nelson, H. H., et al. (2012). DNA methylation arrays as surrogate measures of cell mixture distribution. BMC Bioinforma. 13, 86. doi:10.1186/1471-2105-13-86Available at: https://www.ncbi.nlm.nih.gov/pubmed/22568884.
Kakuszi, B., Szuromi, B., Bitter, I., and Czobor, P. (2020). Attention deficit hyperactivity disorder: last in, first out - delayed brain maturation with an accelerated decline? Eur. Neuropsychopharmacol. 34, 65–75. doi:10.1016/j.euroneuro.2020.03.011Available at: https://www.ncbi.nlm.nih.gov/pubmed/32279924.
Kurth, F., Levitt, J. G., Gaser, C., Alger, J., Loo, S. K., Narr, K. L., et al. (2022). Preliminary evidence for a lower brain age in children with attention-deficit/hyperactivity disorder. Front. Psychiatry 13, 1019546. doi:10.3389/fpsyt.2022.1019546Available at: https://www.ncbi.nlm.nih.gov/pubmed/36532197.
Levine, M. E., Lu, A. T., Bennett, D. A., and Horvath, S. (2015). Epigenetic age of the pre-frontal cortex is associated with neuritic plaques, amyloid load, and Alzheimer's disease related cognitive functioning. Aging (Albany NY) 7 (12), 1198–1211. doi:10.18632/aging.100864Available at: https://www.ncbi.nlm.nih.gov/pubmed/26684672.
Liu, L., Qi, X., Cheng, S., Meng, P., Yang, X., Pan, C., et al. (2023). Epigenetic analysis suggests aberrant cerebellum brain aging in old-aged adults with autism spectrum disorder and schizophrenia. Mol. Psychiatry 28 (11), 4867–4876. doi:10.1038/s41380-023-02233-6Available at: https://www.ncbi.nlm.nih.gov/pubmed/37612365.
McEwen, L. M., O'Donnell, K. J., McGill, M. G., Edgar, R. D., Jones, M. J., MacIsaac, J. L., et al. (2020). The PedBE clock accurately estimates DNA methylation age in pediatric buccal cells. Proc. Natl. Acad. Sci. U. S. A. 117 (38), 23329–23335. doi:10.1073/pnas.1820843116Available at: https://www.ncbi.nlm.nih.gov/pubmed/31611402.
McKinney, B. C., Lin, H., Ding, Y., Lewis, D. A., and Sweet, R. A. (2017). DNA methylation evidence against the accelerated aging hypothesis of schizophrenia. NPJ Schizophr. 3, 13. doi:10.1038/s41537-017-0017-5Available at: https://www.ncbi.nlm.nih.gov/pubmed/28560259.
Michelini, G., Jurgiel, J., Bakolis, I., Cheung, C. H. M., Asherson, P., Loo, S. K., et al. (2019). Atypical functional connectivity in adolescents and adults with persistent and remitted ADHD during a cognitive control task. Transl. Psychiatry 9 (1), 137. Available at: https://www.ncbi.nlm.nih.gov/pubmed/30979865. doi:10.1038/s41398-019-0469-7
Michelini, G., Kitsune, G. L., Cheung, C. H., Brandeis, D., Banaschewski, T., Asherson, P., et al. (2016). Attention-deficit/hyperactivity disorder remission is linked to better neurophysiological error detection and attention-vigilance processes. Biol. Psychiatry 80 (12), 923–932. doi:10.1016/j.biopsych.2016.06.021Available at: https://www.ncbi.nlm.nih.gov/pubmed/27591125.
Muetzel, R. L., Blanken, L. M. E., van der Ende, J., El Marroun, H., Shaw, P., Sudre, G., et al. (2018). Tracking brain development and dimensional psychiatric symptoms in children: a longitudinal population-based neuroimaging study. Am. J. Psychiatry 175 (1), 54–62. doi:10.1176/appi.ajp.2017.16070813Available at: https://www.ncbi.nlm.nih.gov/pubmed/28817944.
Murthy, M., Shireby, G., Miki, Y., Viré, E., Lashley, T., Warner, T. T., et al. (2023). Epigenetic age acceleration is associated with oligodendrocyte proportions in MSA and control brain tissue. Neuropathol. Appl. Neurobiol. 49 (1), e12872. doi:10.1111/nan.12872Available at: https://www.ncbi.nlm.nih.gov/pubmed/36542090.
Pidsley, R., Wong Cc, Y., Volta, M., Lunnon, K., Mill, J., and Schalkwyk, L. C. (2013). A data-driven approach to preprocessing Illumina 450K methylation array data. BMC Genomics 14, 293. doi:10.1186/1471-2164-14-293Available at: https://www.ncbi.nlm.nih.gov/pubmed/23631413.
Poisel, E., Zillich, L., Streit, F., Frank, J., Friske, M. M., Foo, J. C., et al. (2023). DNA methylation in cocaine use disorder-An epigenome-wide approach in the human prefrontal cortex. Front. Psychiatry 14, 1075250. doi:10.3389/fpsyt.2023.1075250Available at: https://www.ncbi.nlm.nih.gov/pubmed/36865068.
Protsenko, E., Yang, R., Nier, B., Reus, V., Hammamieh, R., Rampersaud, R., et al. (2021). GrimAge, an epigenetic predictor of mortality, is accelerated in major depressive disorder. Transl. Psychiatry 11 (1), 193. doi:10.1038/s41398-021-01302-0Available at: https://www.ncbi.nlm.nih.gov/pubmed/33820909.
Rosen, A. D., Robertson, K. D., Hlady, R. A., Muench, C., Lee, J., Philibert, R., et al. (2018). DNA methylation age is accelerated in alcohol dependence. Transl. Psychiatry 8 (1), 182. doi:10.1038/s41398-018-0233-4Available at: https://www.ncbi.nlm.nih.gov/pubmed/30185790.
Schulz, K. P., Li, X., Clerkin, S. M., Fan, J., Berwid, O. G., Newcorn, J. H., et al. (2017). Prefrontal and parietal correlates of cognitive control related to the adult outcome of attention-deficit/hyperactivity disorder diagnosed in childhood. Cortex 90, 1–11. doi:10.1016/j.cortex.2017.01.019Available at: https://www.ncbi.nlm.nih.gov/pubmed/28292705.
Shaw, P., Malek, M., Watson, B., Greenstein, D., de Rossi, P., and Sharp, W. (2013). Trajectories of cerebral cortical development in childhood and adolescence and adult attention-deficit/hyperactivity disorder. Biol. Psychiatry 74 (8), 599–606. doi:10.1016/j.biopsych.2013.04.007Available at: https://www.ncbi.nlm.nih.gov/pubmed/23726514.
Shaw, P., and Sudre, G. (2021). Adolescent attention-deficit/hyperactivity disorder: understanding teenage symptom trajectories. Biol. psychiatry 89 (2), 152–161. doi:10.1016/j.biopsych.2020.06.004
Shenk, C. E., O'Donnell, K. J., Pokhvisneva, I., Kobor, M. S., Meaney, M. J., Bensman, H. E., et al. (2021). Epigenetic age acceleration and risk for posttraumatic stress disorder following exposure to substantiated child maltreatment. J. Clin. Child. Adolesc. Psychol., 1–11. doi:10.1080/15374416.2020.1864738Available at: https://www.ncbi.nlm.nih.gov/pubmed/33471576.
Shireby, G. L., Davies, J. P., Francis, P. T., Burrage, J., Walker, E. M., Neilson, G. W. A., et al. (2020). Recalibrating the epigenetic clock: implications for assessing biological age in the human cortex. Brain 143 (12), 3763–3775. doi:10.1093/brain/awaa334Available at: https://www.ncbi.nlm.nih.gov/pubmed/33300551.
Stevenson, A. J., McCartney, D. L., Gadd, D. A., Shireby, G., Hillary, R. F., King, D., et al. (2022). A comparison of blood and brain-derived ageing and inflammation-related DNA methylation signatures and their association with microglial burdens. Eur. J. Neurosci. 56 (9), 5637–5649. doi:10.1111/ejn.15661Available at: https://www.ncbi.nlm.nih.gov/pubmed/35362642.
Teeuw, J., Ori, A. P. S., Brouwer, R. M., de Zwarte, S. M. C., Schnack, H. G., Hulshoff Pol, H. E., et al. (2021). Accelerated aging in the brain, epigenetic aging in blood, and polygenic risk for schizophrenia. Schizophr. Res. 231, 189–197. doi:10.1016/j.schres.2021.04.005Available at: https://www.ncbi.nlm.nih.gov/pubmed/33882370.
Tian, Y., Morris, T. J., Webster, A. P., Yang, Z., Beck, S., Feber, A., et al. (2017). ChAMP: updated methylation analysis pipeline for Illumina BeadChips. Bioinformatics 33 (24), 3982–3984. doi:10.1093/bioinformatics/btx513Available at: https://www.ncbi.nlm.nih.gov/pubmed/28961746.
Vellame, D. S., Shireby, G., MacCalman, A., Dempster, E. L., Burrage, J., Gorrie-Stone, T., et al. (2023). Uncertainty quantification of reference-based cellular deconvolution algorithms. Epigenetics 18 (1), 2137659. doi:10.1080/15592294.2022.2137659Available at: https://www.ncbi.nlm.nih.gov/pubmed/36539387.
Voisey, J., Lawford, B. R., Morris, C. P., Wockner, L. F., Noble, E. P., Young, R. M., et al. (2017). Epigenetic analysis confirms no accelerated brain aging in schizophrenia. NPJ Schizophr. 3 (1), 26. doi:10.1038/s41537-017-0026-4Available at: https://www.ncbi.nlm.nih.gov/pubmed/28871179.
Wolf, E. J., Logue, M. W., Morrison, F. G., Wilcox, E. S., Stone, A., Schichman, S. A., et al. (2019). Posttraumatic psychopathology and the pace of the epigenetic clock: a longitudinal investigation. Psychol. Med. 49 (5), 791–800. doi:10.1017/S0033291718001411Available at: https://www.ncbi.nlm.nih.gov/pubmed/29897034.
Wu, X., Ye, J., Wang, Z., and Zhao, C. (2021). Epigenetic age acceleration was delayed in schizophrenia. Schizophr. Bull. 47 (3), 803–811. doi:10.1093/schbul/sbaa164Available at: https://www.ncbi.nlm.nih.gov/pubmed/33269797.
Yap, K. H., Abdul Manan, H., and Sharip, S. (2021). Heterogeneity in brain functional changes of cognitive processing in ADHD across age: a systematic review of task-based fMRI studies. Behav. Brain Res. 397, 112888. doi:10.1016/j.bbr.2020.112888Available at: https://www.ncbi.nlm.nih.gov/pubmed/32882284.
Zannas, A. S., Arloth, J., Carrillo-Roa, T., Iurato, S., Röh, S., Ressler, K. J., et al. (2015). Lifetime stress accelerates epigenetic aging in an urban, African American cohort: relevance of glucocorticoid signaling. Genome Biol. 16, 266. doi:10.1186/s13059-015-0828-5Available at: https://www.ncbi.nlm.nih.gov/pubmed/26673150.
Zhang, Q., Vallerga, C. L., Walker, R. M., Lin, T., Henders, A. K., Montgomery, G. W., et al. (2019). Improved precision of epigenetic clock estimates across tissues and its implication for biological ageing. Genome Med. 11 (1), 54. doi:10.1186/s13073-019-0667-1Available at: https://www.ncbi.nlm.nih.gov/pubmed/31443728.
Zillich, L., Cetin, M., Hummel, E. M., Poisel, E., Fries, G. R., Frank, J., et al. (2024). Biological aging markers in blood and brain tissue indicate age acceleration in alcohol use disorder. Alcohol Clin. Exp. Res. Hob. 48 (2), 250–259. doi:10.1111/acer.15241Available at: https://www.ncbi.nlm.nih.gov/pubmed/38276909.
Keywords: epigenetic age, ADHD (attention deficit and hyperactivity disorder), epigenetic clock, DNA methylation, biological age, postmortem brain
Citation: Shastri GG, Sudre G, Ahn K, Jung B, Kolachana B, Auluck PK, Elnitski L and Shaw P (2024) Examining epigenetic aging in the post-mortem brain in attention deficit hyperactivity disorder. Front. Genet. 15:1480761. doi: 10.3389/fgene.2024.1480761
Received: 14 August 2024; Accepted: 24 September 2024;
Published: 08 October 2024.
Edited by:
Francisco Navarrete Rueda, Miguel Hernández University of Elche, SpainReviewed by:
Cibele Edom Bandeira, Federal University of Rio Grande do Sul, BrazilCopyright © 2024 Shastri, Sudre, Ahn, Jung, Kolachana, Auluck, Elnitski and Shaw. This is an open-access article distributed under the terms of the Creative Commons Attribution License (CC BY). The use, distribution or reproduction in other forums is permitted, provided the original author(s) and the copyright owner(s) are credited and that the original publication in this journal is cited, in accordance with accepted academic practice. No use, distribution or reproduction is permitted which does not comply with these terms.
*Correspondence: Philip Shaw, d2FzaC5zaGF3QGdtYWlsLmNvbQ==
Disclaimer: All claims expressed in this article are solely those of the authors and do not necessarily represent those of their affiliated organizations, or those of the publisher, the editors and the reviewers. Any product that may be evaluated in this article or claim that may be made by its manufacturer is not guaranteed or endorsed by the publisher.
Research integrity at Frontiers
Learn more about the work of our research integrity team to safeguard the quality of each article we publish.