- 1Postgraduate School, Medical School of Chinese PLA, Beijing, China
- 2Department of Traditional Chinese Medicine, The Sixth Medical Center, Chinese PLA General Hospital, Beijing, China
- 3School of Pharmacy, Key Laboratory for Modern Research of Traditional Chinese Medicine of Jiangsu, Nanjing University of Chinese Medicine, Nan Jing, Jiangsu, China
- 4Department of Gastroenterology and Hepatology, The First Medical Center, Chinese PLA General Hospital, Beijing, China
Objective: Ferroptosis, a novel form of cell death, is closely associated with excessive iron accumulated within the substantia nigra in Parkinson’s disease (PD). Despite extensive research, the underlying molecular mechanisms driving ferroptosis in PD remain elusive. Here, we employed a bioinformatics and machine learning approach to predict the genes associated with ferroptosis in PD and investigate the interactions between natural products and their active ingredients with these genes.
Methods: We comprehensively analyzed differentially expressed genes (DEGs) for ferroptosis associated with PD (PDFerDEGs) by pairing 3 datasets (GSE7621, GSE20146, and GSE202665) from the NCBI GEO database and the FerrDb V2 database. A machine learning approach was then used to screen PDFerDEGs for signature genes. We mined the interacted natural product components based on screened signature genes. Finally, we mapped a network combined with ingredients and signature genes, then carried out molecular docking validation of core ingredients and targets to uncover potential therapeutic targets and ingredients for PD.
Results: We identified 109 PDFerDEGs that were significantly enriched in biological processes and KEGG pathways associated with ferroptosis (including iron ion homeostasis, iron ion transport and ferroptosis, etc.). We obtained 29 overlapping genes and identified 6 hub genes (TLR4, IL6, ADIPOQ, PTGS2, ATG7, and FADS2) by screening with two machine learning algorithms. Based on this, we screened 263 natural product components and subsequently mapped the “Overlapping Genes-Ingredients” network. According to the network, top 5 core active ingredients (quercetin, 17-beta-estradiol, glycerin, trans-resveratrol, and tocopherol) were molecularly docked to hub genes to reveal their potential role in the treatment of ferroptosis in PD.
Conclusion: Our findings suggested that PDFerDEGs are associated with ferroptosis and play a role in the progression of PD. Taken together, core ingredients (quercetin, 17-beta-estradiol, glycerin, trans-resveratrol, and tocopherol) bind well to hub genes (TLR4, IL6, ADIPOQ, PTGS2, ATG7, and FADS2), highlighting novel biomarkers for PD.
1 Introduction
Parkinson’s disease (PD) is a prevalent and progressive neurodegenerative disorder characterized by resting tremors, stiffness, bradykinesia, and postural instability, its incidence and prevalence rise with age (Hayes et al., 2019; Bloem et al., 2021). Epidemiological studies reveal that young-onset PD (onset age <40 years) is on the rise in China and Europe, posing a serious threat to human health (Jankovic, 2008). The pathological mechanism of PD is multifactorial, involving the death of dopaminergic neurons resulting from complex interactions between abnormal α-synuclein aggregation, mitochondrial and lysosomal dysfunction, and neuroinflammation (Bloem et al., 2021; Wang et al., 2022). Normally, dopaminergic neurons in the substantia nigra transmit dopamine to the striatum via the substantia nigra-striatum pathway, which is antagonistic to acetylcholine and participates in the regulation of motor function in the basal ganglia. In PD, the degeneration and loss of dopaminergic neurons in the substantia nigra lead to a significant reduction in dopamine levels in the striatum, resulting in relative hyperfunction of the acetylcholine system and clinical symptoms such as increased muscle tone and bradykinesia (Belarbi et al., 2020). Currently, there is no cure for PD, and available treatments only alleviate symptoms. Thus, it is crucial to identify new targets to improve the diagnosis and treatment of PD patients.
Ferroptosis is a novel form of iron-dependent and reactive oxygen species (ROS)-dependent cell death, which is distinct from apoptosis, necrosis, and autophagy. Although the exact pathogenesis of Parkinson’s disease (PD) remains elusive, the imbalance of iron homeostasis and lipid peroxidation have long been implicated as potential contributing factors in PD pathology (Yan et al., 2021). Moreover, mounting evidence strongly suggested that ferroptosis plays a significant role in the PD-related neurodegeneration. For instance, several mutations in ferroptosis genes are associated with PD, including the key regulator of ferroptosis DJ-1, autosomal recessive PD gene PARK7 and PLA2G6 (Cao et al., 2020). Additionally, the features of ferroptosis induction are highly consistent with the pathological changes observed in PD patients, including iron overload (Ayton et al., 2015), reduced GSH levels (Li et al., 1997), and reduced CoQ10 (Bersuker et al., 2019).
Transcriptomics, which involves studying the complete sequence information of RNA, is a potent tool for investigating potential gene regulation mechanisms related to complex traits and exploring the pathogenesis of PD. (Borrageiro et al., 2018; Sertbas and Ulgen, 2018). High-throughput sequencing technology, also known as next-generation sequencing (NGS), is an important tool for detecting differentially expressed genes from two or more samples, offering fast monitoring, high accuracy, and wide coverage (Borrageiro et al., 2018; Sertbas and Ulgen, 2018; Bersuker et al., 2019). In clinical settings, NGS technology is used to enhance the detection of pathogenic genes (Schilter et al., 2023; Yigit et al., 2023). For instance, high-throughput technology can be employed to identify rare variants and candidate genes linked to familial and sporadic PD (Kim J. Y. et al., 2023; Qin et al., 2023). NGS has yielded promising results in the diagnosis of neurodegenerative diseases. However, there are still several challenges, including technical limitations, high costs, lack of standardization of methods and data analysis, which require further investigation.
Natural products have been utilized for centuries for the treatment and prevention of human diseases, with a particular emphasis on plants and traditional Chinese medicines in China. Fumarate, peiminine, and aconitine alkaloids are examples of compounds extracted from natural products that have been utilized for the treatment of various ailments (Shen and Hao, 2020). Recent research has demonstrated that natural products have potential anti-Parkinson’s disease (PD) effects, attributable to their antioxidant and anti-inflammatory properties, as well as their ability to inhibit iron accumulation and maintain proteasome degradation and mitochondrial homeostasis (Solayman et al., 2017; Zhang et al., 2017). While a variety of small molecules and natural products with anti-PD activity have been identified, including flavonoid and polyphenol compounds, phenylpropanoid (coumarin) compounds, quinone compounds, saponin compounds, alkaloid compounds, and terpenoid compounds, none of them are capable of completely curing PD (Solayman et al., 2017). Therefore, it is imperative to utilize modern technologies, such as high-throughput sequencing combined with machine learning, to identify additional natural product components with anti-PD effects.
This article presents an integrated approach to analyze transcriptome data from the substantia nigra of the brain using machine learning techniques to identify potential key genes involved in the pathogenesis of PD ferroptosis. Furthermore, we aim to identify natural products that can potentially treat PD. Our study provides a valuable reference for the exploration of novel therapeutic targets and natural product-based interventions for PD.
2 Materials and methods
2.1 Microarray data retrieval
The PD dataset was obtained from the public repository NCBI GEO (http://www.ncbi.nlm.nih.gov/geo) (Barrett et al., 2013) using “Parkinson’s disease” or “Parkinson” as keywords for the search. Further screening was performed based on sequencing type (transcriptomics), species (HOMO Sapiens), and sample size (≥10). Finally, GSE7621, GSE20146, and GSE202665 were obtained. GSE7621 (Lesnick et al., 2007) [(HG-U133_Plus_2) Affymetrix Human Genome U133 Plus 2.0 Array] was generated on the GPL570 platform. This dataset analyzed the brain substantia nigra tissue of 9 normal samples and 16 PD samples after death. GSE20146 (Zheng et al., 2010) [(HG-U133_Plus_2) Affymetrix Human Genome U133 Plus 2.0 Array] was also generated on the GPL570 platform. This dataset consisted of 20 substantia nigra samples, including 10 PD samples and 10 control samples. GSE202665 (Diener et al., 2023) [Agilent-072363 SurePrint G3 Human GE v3 8 × 60 K Microarray 039494 (Feature Number Version)] was generated on the GPL20844 platform and consisted of 5 serum samples from PD stages 1–4, and 5 control samples.
2.2 Acquisition of microarray data and identification of differentially expressed genes (DEGs)
Microarray data for each GEO dataset can be obtained using R package “GEOquery”. Differential gene expression analysis to identify DEGs can be performed with R package “limma” (Ritchie et al., 2015). The identified DEGs must meet the criteria of adj. p < 0.05 and |log2(Fold-change)| > 1. The resulting DEGs were visualized using R packages “ggplot2” (Gustavsson et al., 2022) and “pheatmap” (Diao et al., 2018). Subsequently, we used the R package “sva” to remove batch effects, resulting in 49 control samples and 55 PD samples.
2.3 Identification of DEGs related to ferroptosis in Parkinson’s disease (PDFerDEGs)
FerrDb V2 (http://www.zhounan.org/ferrdb/current/) (Zhou et al., 2023) is a dedicated database for ferroptosis regulators and ferroptosis-disease associations. The database includes two categories of ferroptosis regulators: gene regulators (drivers, suppressors, markers, and unclassified regulators) and substance regulators. We downloaded the driver, suppressor, and marker regulator genes from the database and identified the DEGs and their expression levels related to ferroptosis gene regulators in the three datasets using the R package “limma” (Ritchie et al., 2015), based on sample expression levels after batch effect removal. The differentially expressed genes in PD-Ferroptosis-DEGs were filtered based on a significance level of p < 0.05.
2.4 Fitting generalized linear model and support vector machine recursive feature elimination for screening feature genes of ferroptosis
A generalized linear model was fitted using lasso regression to screen and predict feature genes of ferroptosis. The R package “glmnet” (Friedman et al., 2010), developed by the lasso regressor Trevor Hastie of Stanford University, was used. This package is characterized by fitting a range of different λ values to each previous fit, resulting in significant improvements in operational efficiency. The model loss function was set to a log-likelihood of -2-fold, and 10-fold cross-validation was performed by specifying “nfolds”.
The support vector machine (SVM) is a supervised learning algorithm used for dichotomous variables. The SVM recursive feature elimination (SVM-RFE) algorithm trains samples through a model, ranks the scores for each feature, removes the feature with the smallest score, trains the model again with the remaining features, performs the next iteration, and finally selects the optimal number of features. In this study, we used the R package “e1071” (version 1.6–8; https://cran.r-project.org/web/packages/e1071) to generate two training and testing datasets to screen the DEGs for key genes associated with ferroptosis.
To assess the performance of the selected genes, we plotted a receiver operating characteristic (ROC) curve, which shows the trade-off between specificity and sensitivity, and calculated the area under the curve (AUC) using the R package “pROC” (Robin et al., 2011). We used the intersection genes identified by both the lasso regression and SVM-RFE methods and considered genes with an AUC greater than 0.7 as accurate diagnostic markers for ferroptosis.
2.5 PDFerDEGs logFC functional enrichment analysis
The Gene Ontology (GO) is a widely-used tool for defining and describing gene product functions, including biological processes (BPs), cellular components (CCs), and molecular functions (MFs). The Kyoto Encyclopedia of Genes and Genomes (KEGG) is a comprehensive database that integrates genomic, chemical, and functional information. To perform GO and KEGG enrichment analysis of the intersection genes, we utilized version 3.18.1 of the R package “clusterProfiler” (Yu et al., 2012), with a p-value threshold of less than 0.05. Furthermore, we calculated the Z-score for each item using the R package “Goplot” (Walter et al., 2015).
2.6 Analysis of protein–protein interactions (PPI) and identification of hub genes
We also downloaded the string database (https://string-db.org/), which was used to filter protein-protein interactions (PPIs) between the intersection genes of the machine learning model, with the minimum required interaction score set to 0.4 and hidden connected nodes in the network. After analysis of internode relationships in the string network diagram using Perl imported into Cytoscape v3.7.1, the intersecting genes were calculated with the built-in cytoHubba plugin. After the computational results were exported, the R package “UpSetR” (Conway et al., 2017) was used to score hub genes and finally obtain the core intersection genes. Visualization of core intersecting genes was performed using the R package “pheatmap” (Diao et al., 2018).
2.7 Identification of intersecting genes related to active ingredients and the construction of an “Overlapping Genes-Ingredients” network
The HERB (http://herb.ac.cn/) (Fang et al., 2021) database is a natural medicine database platform that integrates high-throughput experimental data and reference mining data. The database provides functions such as browsing, searching, viewing and downloading of TCM, TCM active ingredients, target genes, diseases, high-throughput experimental and reference mining data. The obtained intersection gene targets were uploaded to this database to deduce the active ingredients by reverse, then the “Overlapping Genes-Ingredients” network was constructed. Using the computational tools integrated into Cytoscape, we calculated the topology values of individual nodes in the network, including degree, closeness, and betweenness.
2.8 Molecular docking
Molecular docking is a widely used computational technique in drug discovery and drug design that enables the study of the interaction and recognition of receptors and ligands. It is a theoretical simulation method for studying intermolecular interactions, predicting their binding modes and affinities (Chen et al., 2020). In this study, we employed molecular docking to investigate whether the top five ingredients, ranked by degree in the “Overlapping Genes-Ingredients” network, are capable of binding to the hub genes. The specific operation flow was as follows.
1) Protein receptor file preparation: The 3D structures of the target proteins were retrieved from the Protein Data Bank (PDB) based on the hub genes identified using the cytoHubba plugin (http://www.rcsb.org/) (Berman et al., 2000). The PDB format files of these proteins were downloaded and prepared using AutoDock Vina 1.2.0 (Eberhardt et al., 2021). This involved removing any water molecules present in the protein structures and replacing them with hydrogens. The altered protein structures were then saved in the PDBQT format.
2) The preparation of drug receptor documents involved retrieving drug molecular structures from TCMSP and PubChem (https://pubchem.ncbi.nlm.nih.gov/) databases (Ru et al., 2014; Kim S. et al., 2023). The resulting structures were processed using AutoDock Vina, which involved the addition of hydrogen atoms and detection of torsion tree root, followed by selection of the detected torsions. The final output consisted of ligand files in PDBQT format.
3) Defining docking parameters: We imported the protein receptors and small molecule receptors separately into AutoDock Vina to determine the scope of molecular docking. We set the protein acceptor as the grid’s center and adjusted the center coordinates (center X/Y/Z) and box size (size X/Y/Z) parameters to ensure that the protein was fully enclosed by the grid’s box. After this step, we exported the docking result file in Vina Config format.
4) Molecular docking and visualization were carried out using the AutoDock Vina functional module. The resulting pdqbt docking result files were imported into PyMOL (https://pymol.org/2/), which was used to visualize the docking results with the best binding energies and export them as PDB files. Detailed docking information was visualized using igplot + v.2.1 (Ye et al., 2021).
3 Results
3.1 Identification of PD DEGs and PDFerDEGs
The overall analysis strategy is shown in the flow chart in Figure 1. After eliminating the batch effect (Figure 2), three PD-related GEO datasets, GSE7621, GSE20146, and GSE202665, were obtained for analysis. There were 317 DEGs in the GSE7621 dataset, including 144 upregulated and 173 downregulated genes. There were 433 DEGs in the GSE20146 dataset, of which 197 were upregulated genes, and 236 were downregulated genes. There were 189 DEGs in the GSE202665 dataset, of which 115 were upregulated genes, and 74 were downregulated genes. The DEGs were visualized by volcano plots and heatmaps (Figures 3A–F). After eliminating batch effects across datasets, the three datasets were merged to obtain a total of 55 PD samples and 49 normal control samples (Table 1).
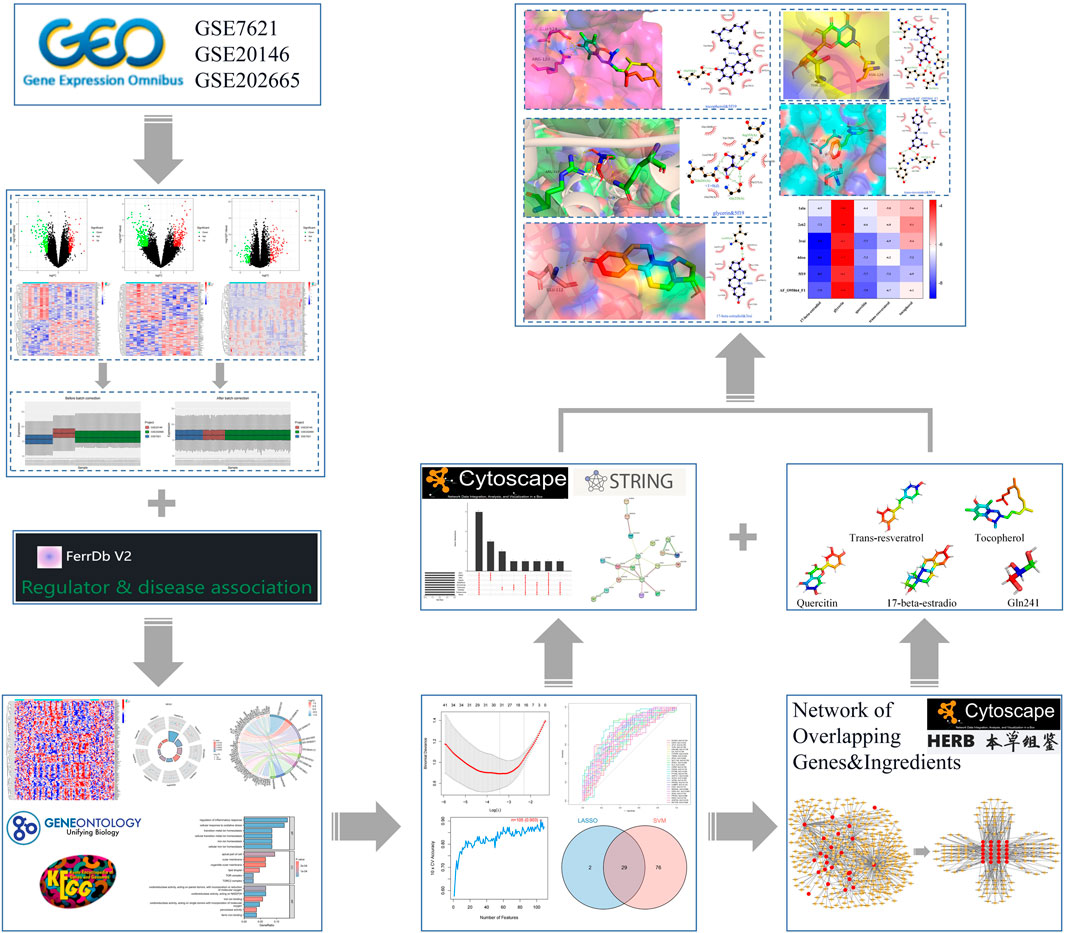
FIGURE 1. Flow chart of machine learning strategy to screen PD ferroptosis signature genes and mining potential therapeutic drugs.
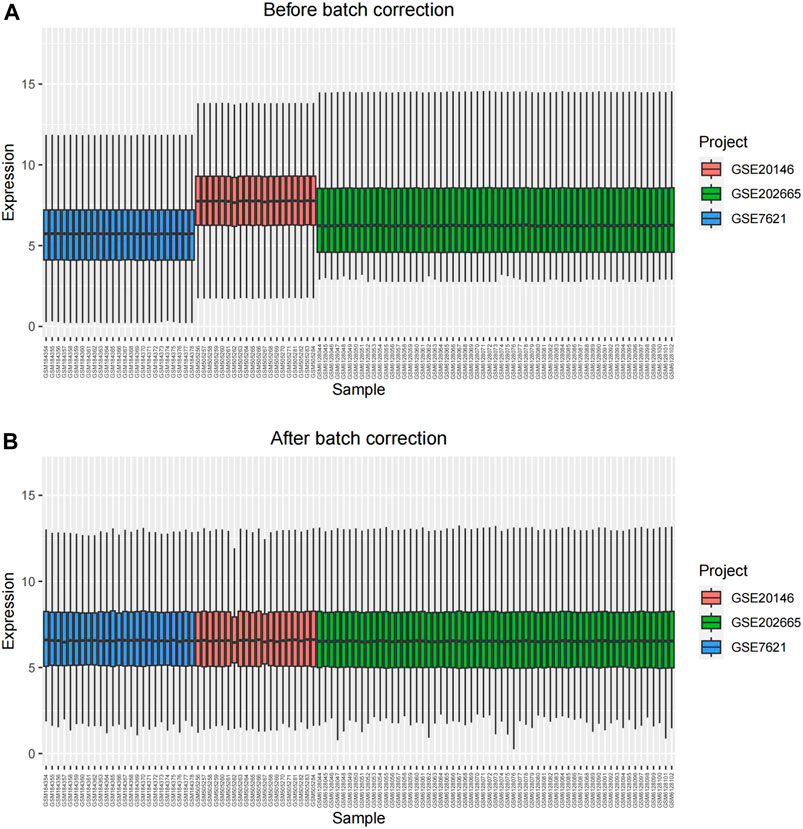
FIGURE 2. The result of eliminating batch effects. (A) before batch correction; (B) after batch correction.
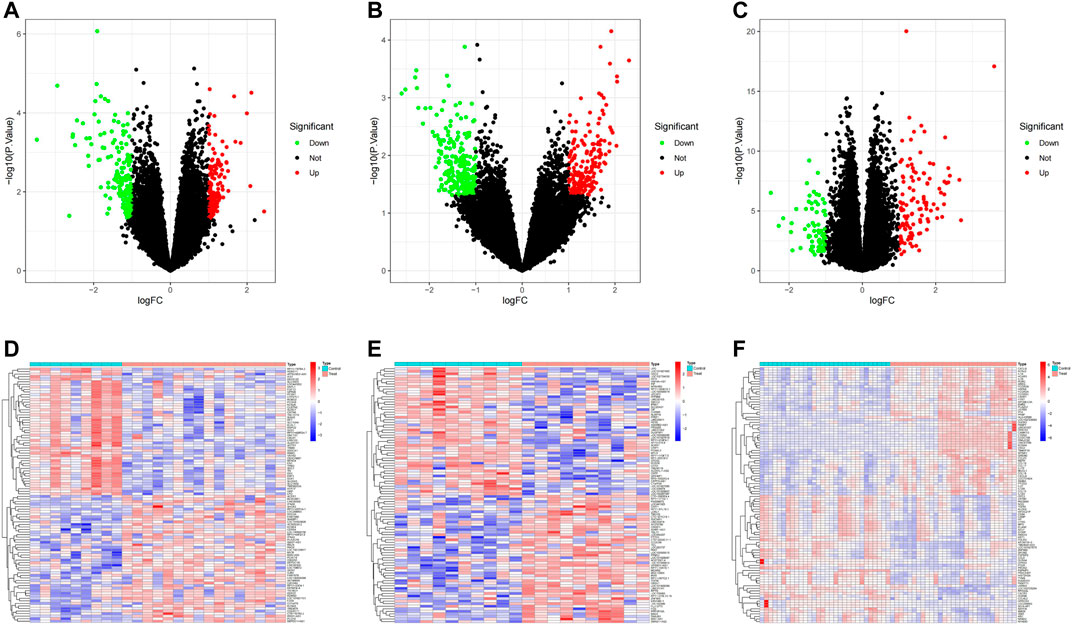
FIGURE 3. PD transcriptome dataset differential analysis. (A–C) volcano plot DEGs in GSE7621, GSE20146, and GSE202665; (D–F) Clustered heatmap of DEGs in GSE7621, GSE20146, and GSE202665.
To investigate the potential link between ferroptosis and PD, we initially retrieved iron-related genes from the ferrdb V2 (http://www.zhounan.org/ferrdb/current/) database, which compiles drivers, suppressors, and markers associated with ferroptosis, resulting in a total of 728 ferroptosis-related genes. Subsequently, we identified 398 ferroptosis-associated genes that were expressed in the three datasets. Finally, as depicted in Figure 4, we obtained 109 differentially expressed ferroptosis genes in PD. Of these, 61 were upregulated genes, and 48 were downregulated genes (Supplementary Table S1).
3.2 Enrichment analysis of PDFerDEGs
Integrated with the logFC values of the 109 DEGs, enrichment analysis of the differentially expressed genes was performed. GO enrichment analysis yielded a total of 891 biological process (BP), 26 cellular component (CC) and 39 molecular function (MF) in the BP for a total of 891 biological process (BP), 26 molecular function (CC) and 39 molecular function (MF) for a total of 21 genes (IREB2, HMOX1, NCOA4, ACO1, SLC39A14, SLC11A2, FTL, CP, TF, ATG5, LGMN, PTGS2, NFE2L2, NQO1, HSPA5, CDH1, NEDD4L, G6PD, PRKAA2, SMPD1, and IREB2) involved in 11 ferroptosis processes or transport of metal ions or transport of metal ions, respectively (Table 2;Figure 5; Supplementary Table S2).In addition, 33 pathways were obtained from KEGG enrichment. Among them, 11 genes (HMOX1, ATG5, NCOA4, SLC39A14, SLC11A2, SLC7A11, ACSL3, FTL, CP, TF, and ATG7) are involved in the ferroptosis (hsa04216) pathway (Supplementary Table S3). Ferroptosis is able to participate in cell growth and death, as well as in the regulation of neurodegenerative diseases (Conrad et al., 2016).
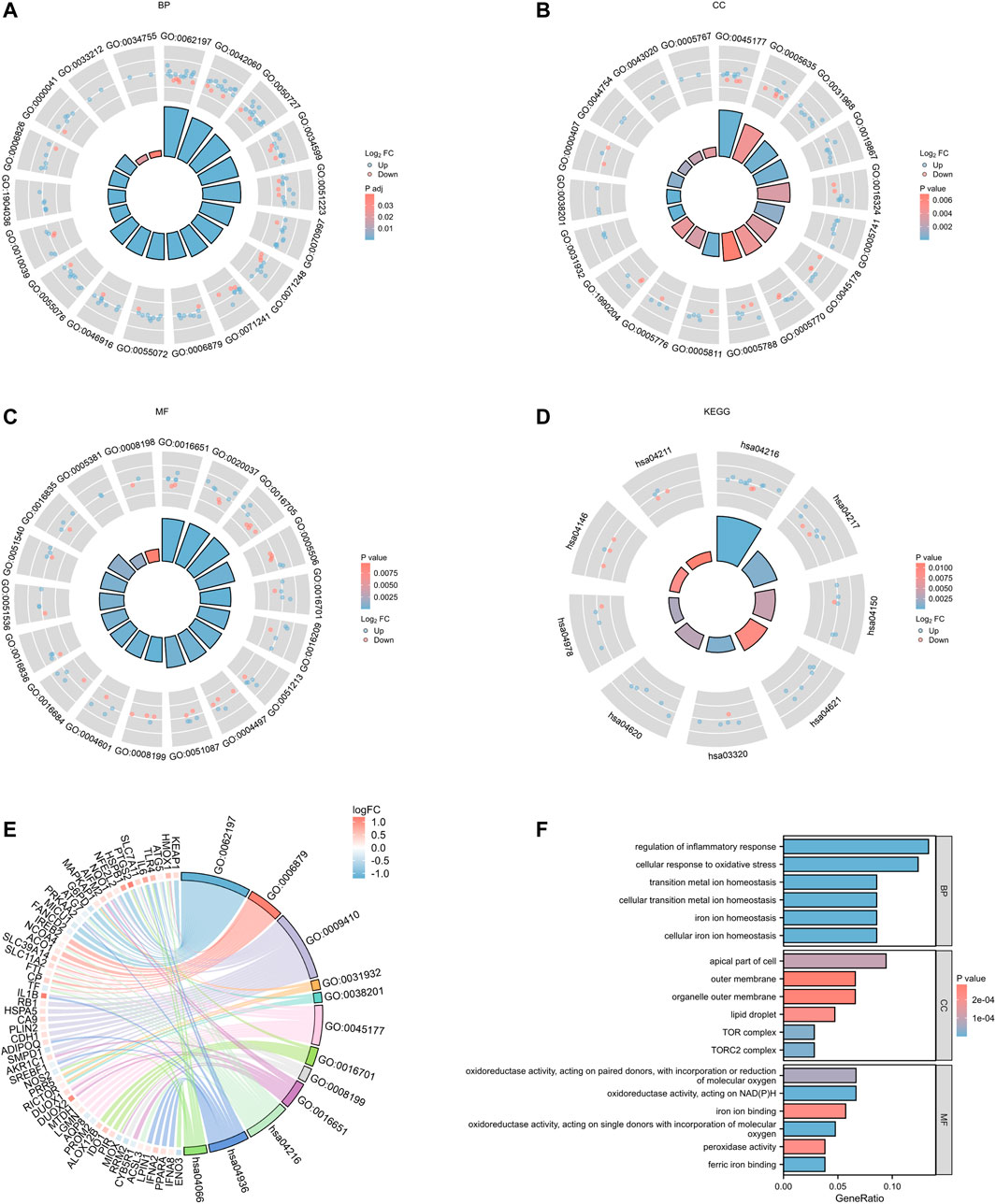
FIGURE 5. PDFerDEGs logFC functional enrichment. (A–C) functional enrichment of GO; (D) result of KEGG; (E) Sankey diagram combined with GO and KEGG; (F) overall result of GO related to ferroptosis.
3.3 Machine learning models to predict ferroptosis feature genes
LASSO regression was used to analyze the resulting 109 binary discrete variables, resulting in 31 feature genes (Supplementary Table S4). Support vector machine recursive feature elimination was performed after scoring the 109 differential genes, and machine learning automatically selected the 105 feature genes with the smallest error (Supplementary Table S5). As shown in Figure 6, we screened the overlapping targets of the two prediction results by constructing a Venn diagram, resulting in a total of 29 intersecting genes. After using receiver operating characteristic (ROC) evaluation, we found that among the above intersecting genes, DUOX2, ATG7, TLR4, DNAJB6, IL6, SLC11A2, CIRBP, FADS2, PTGS2, AIFM2, PROM2, CHMP6, FZD7, SOX2, and ENO3 had an area under the curve >0.7, suggesting that the above 7 target genes have high predictive value in PD induced by the progression of ferroptosis.
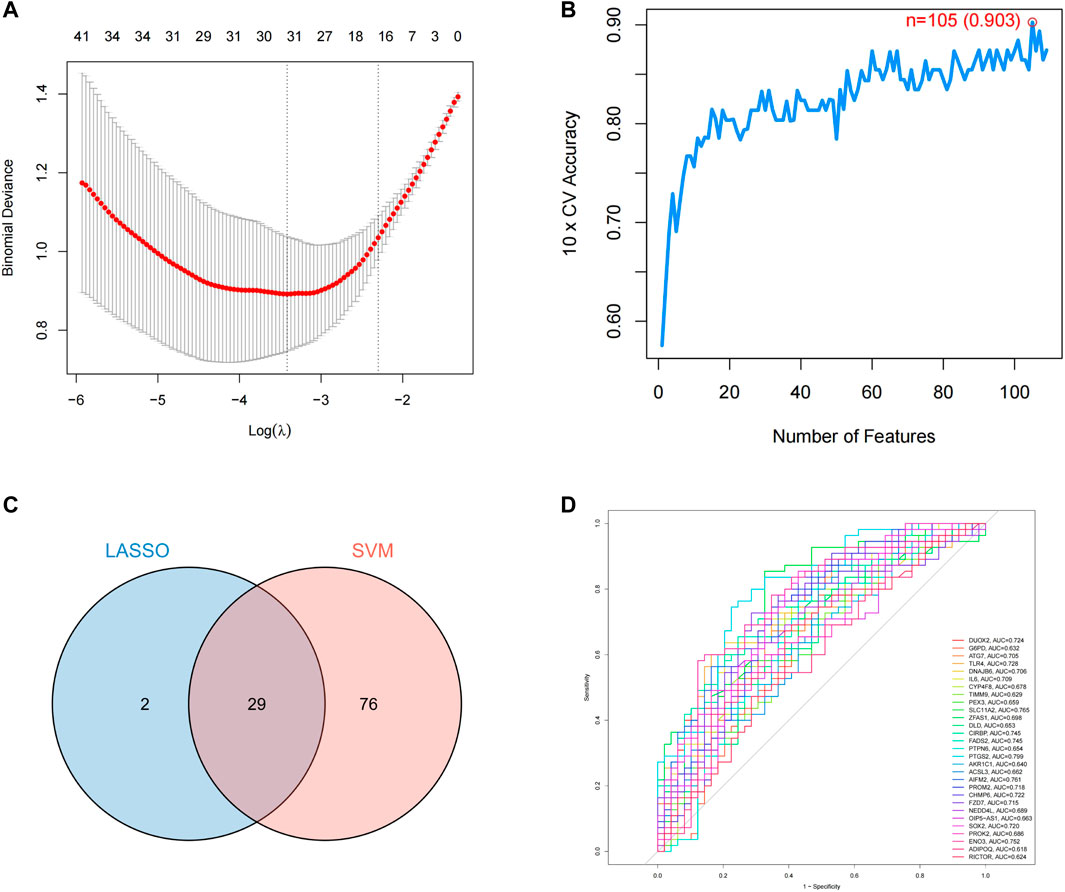
FIGURE 6. Prediction of PD ferroptosis signature genes. (A) lasso regression analysis; (B) number of features predicted by SVM-RFE; (C) Venn diagram of LASSO and SVM-RFE; (D) ROC evaluation of intersecting genes.
3.4 Acquisition results of hub genes for overlapping genes and PPI network construction
In the STRING database, we first set the species as “Homo sapiens” and subsequently imported 29 intersecting genes to obtain the PPI network (Figure 7A), which involved 27 nodes and 24 edges. The obtained TSV files were imported into Cytoscape software (v3.7.1) for further analysis and visualization (Figure 7B). To obtain the hub genes in the intersection genes, based on the PPI network described above, we used the cytoHubba plug-in of Cytoscape, which currently contains 12 topological analysis methods. We ranked them according to MCC, DMNC, MNC, Degree, BottleNeck, EcCentricity, Closeness, Radiality, Betweenness and Stress scoring (Table 3). As shown in Figure 7C, six hub genes (TLR4, IL6, ADIPOQ, PTGS2, ATG7, and FADS2) were finally obtained.
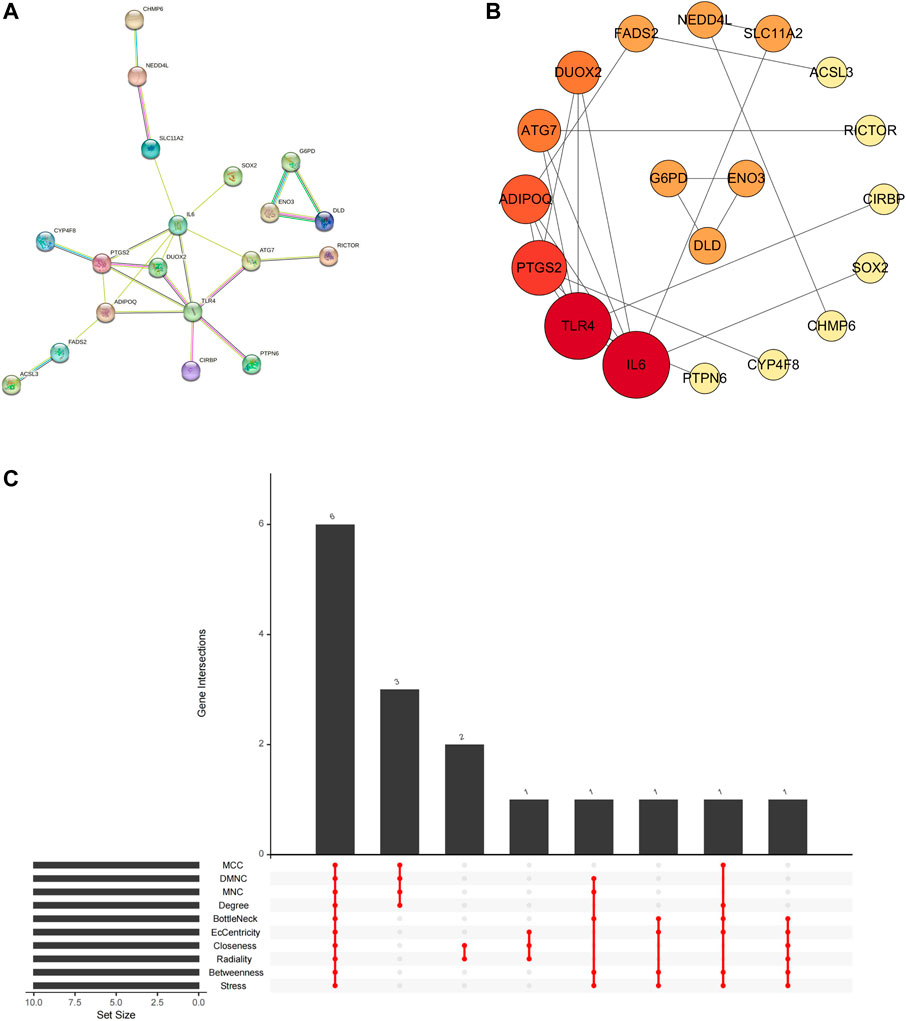
FIGURE 7. Network of PD ferroptosis signature genes. (A) protein-protein interaction network; (B) topology characteristic of each node (arranging from highest to lowest according to node degree value); (C) hub genes calculated by plugin cytoHubba.
3.5 Identification of overlapping genes-related compounds and construction of the “Overlapping Genes-Ingredients” network
After uploading 29 overlapping genes to HERB (http://herb.ac.cn/), we obtained the ingredients associated with Overlapping Gene. After identification, 24 genes had corresponding ingredients (Supplementary Table S6). Finally, a total of 363 ingredients related to 24 genes that were characterized after machine learning model screening were obtained (Supplementary Table S7). Although PTGS2 also had related ingredients, the number was more than three thousand, and we thought that too many ingredients were not beneficial to this study, so we did not include the ingredients related to this gene.
We imported the correspondence between ingredients and overlapping genes into Cytoscape v3.7.1 to build the “Overlapping Genes-Ingredients” network (Figure 8A). The topological values of the nodes in the network were then calculated using its built-in calculation tool (Supplementary Table S8). We filtered ingredient nodes (Figure 8A) based on the median value and took with a median value greater than 1 (Figure 8B). Besides, the top five core ingredients were obtained according to calculation result. They were quercetin (Ingredient ID:HBIN041721), 17-beta-estradiol (Ingredient ID:HBIN001991), glycerin (Ingredient ID:HBIN028102), trans-resveratrol (Ingredient ID:HBIN046831) and tocopherol (Ingredient ID:HBIN046506) (Table 4).
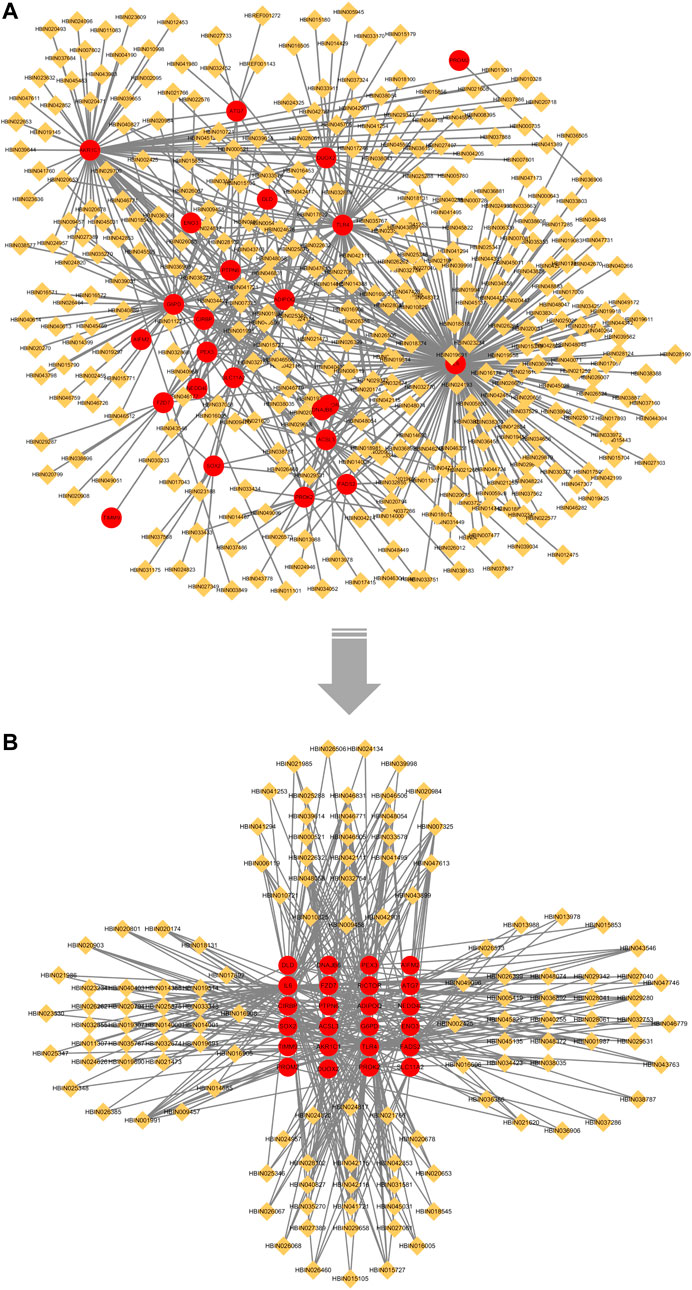
FIGURE 8. “Overlapping Genes-Ingredients” network. Red circles represent overlapping genes between LASSO and SVM-RFE; orange diamond represents active ingredient. (A) no median value node removed; (B) after removing the median value node.
3.6 Molecular docking
We subjected the top five core ingredients of the “Overlapping Genes-Ingredients” network screening to molecular docking with the 6 hub genes TLR4 (PDB ID:2Z62), IL6 (PDB ID:1ALU), ADIPOQ (PDB ID:4DOU), PTGS2 (PDB ID:5F19), ATG7 (PDB ID:3RUI), and FADS2 (PDB ID:AF_AFO95864F1) calculated by cytoHubba. The docking results of compounds with proteins are shown in Figures 9A–E. Figures 9F–J shows the amino acid residue information versus hydrogen bonding distance for the docking of each compound to proteins.
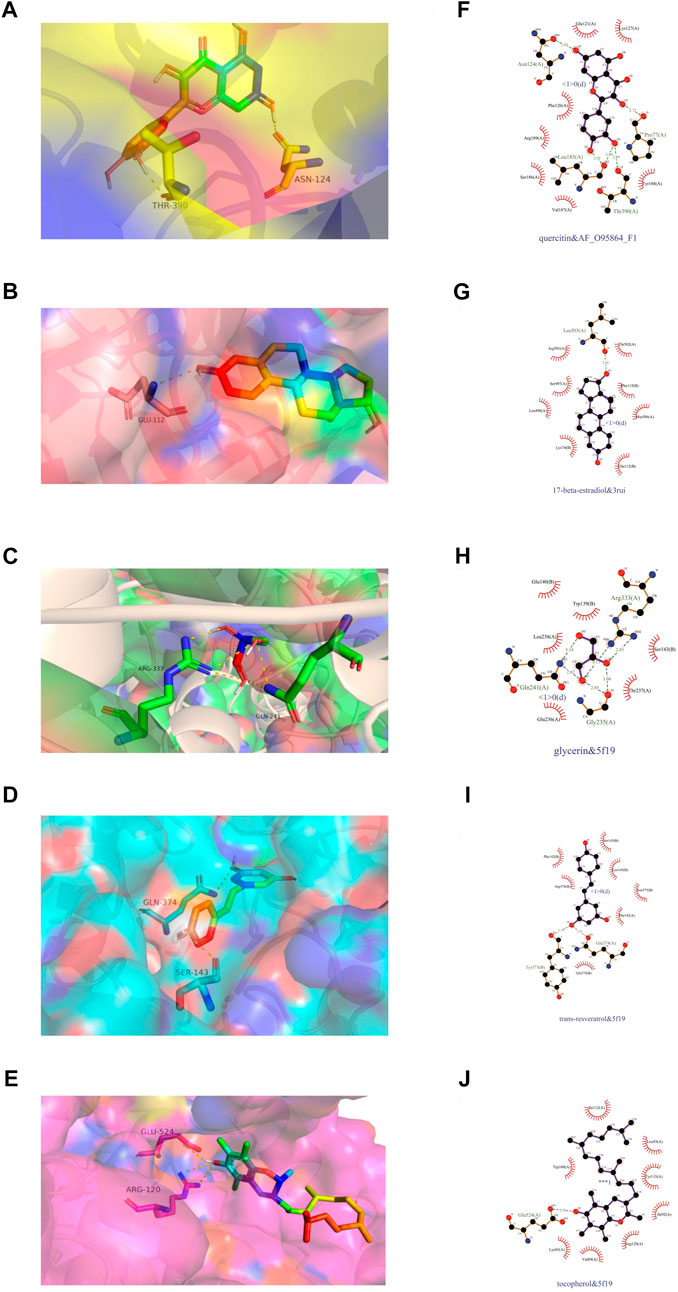
FIGURE 9. Visualization of molecular docking. (A,F) quercetin and FADS2, binding energy = −7.9 kca/mol; (B,G) 17-beta-estradiol and ATG7, binding energy = −8.8 kca/mol; (C,H) glycerin and PTGS2, binding energy = −4.2 kca/mol; (D,I) trans-resveratrol and PTGS2, binding energy = −7.2 kca/mol; (E,J) tocopherol and PTGS2, binding energy = −6.9 kca/mol.
As a result (Table 5), the top five ingredients could all interact with the six hub genes. Quercitin has the smallest binding energy to FADS2. Figure 9F shows that quercetin formed 1 hydrogen bond with Asn124, Pro77 and Thr390, and 2 hydrogen bonds with Leu185 in FADS2. 17 beta estradio has the smallest binding energy to Atg7. Figure 9G shows that 17 beta estradio formed 1 hydrogen bond with Leu503 in ATG7. Glycerin has the smallest binding energy to PTGS2. Figure 9H shows that glycerin formed 2 hydrogen bonds with Gln241, Gly235 and Arg333 in PTGS2. Trans resveratrol has the smallest binding energy to PTGS2. Figure 9I shows that trans resveratrol formed 1 hydrogen bond with Tyr373 and Gln374 in PTGS2. Tocopherol had the smallest binding energy to ADIPOQ but could not exhibit its hydrogen bond, so we selected the docking results of PTGS2 for visualization. Figure 9J shows that tocopherol formed 1 hydrogen bond with Glu524 in PTGS2.
The binding energy results of the docking between each compound and hub genes are shown as a heatmap (Figure 10), and it can be seen that the binding energy ranged from −3.7 kca/mol to −11.5 kca/mol, indicating that these compounds may be potential therapeutic ingredients for hub genes.
4 Discussion
High-throughput can help us better understand the complexity and diversity of biological systems, gives us a clearer understanding of gene expression profiles in living organisms. Machine learning, a branch of artificial intelligence, is a process of learning from experience. It can train a model with a large amount of data, which enables the prediction and classification of unknown data. Currently, although there are individual bioinformatics reports and studies on ferroptosis in PD, the development of compound prediction based on machine learning has not been reported (Moni et al., 2019; Falchetti et al., 2020; Jian et al., 2022). This study is the first to integrate bioinformatics analysis methods, machine learning model prediction classification, and data mining-based inverse derivation of active ingredients, screen core compounds of feature gene action derived from machine learning predictions, and validate using molecular docking.
In this study, we first integrated the transcriptome datasets of three human brain substantia nigra tissues from the GEO database, combined with the targets included in the public ferroptosis database, bioinformatically analyzed the expression of ferroptosis-related genes in the three datasets, and finally obtained 103 genes that were differentially regulated in ferroptosis. Ferroptosis DEGs functional enrichment found that 11 BP items were directly related to ferroptosis or metal ion transport processes such as cellular iron ion homeostasis, iron ion transport, and transport metal ion transport. In addition, KEGG enrichment was supported by the ferroptosis (hsa04216) pathway. This illustrates that ferroptosis may contribute to the onset and progression of PD. Subsequently, to further predict the ferroptosis feature genes for PD, we predicted and classified 109 ferroptosis differential genes using two machine learning models, Lasso regression and SVM-RFE. The intersection of the genes obtained from the two prediction models was used to obtain 29 ferroptosis feature genes after machine learning. A total of 363 related compounds were derived from feature genes. According to the correspondence between compounds and targets, we constructed the “Overlapping Genes-Ingredients” network, and after calculating the values of node topology, the top five core ingredients were obtained, namely, quercetin, 17 beta estradiol, glycerin, trans resveratrol, and tocopherol. In addition, PPI network node topology analysis revealed 6 hub genes among the 29 characterized genes, i.e., TLR4, IL6, ADIPOQ, PTGS2, Atg7, and FADS2.
A total of 109 ferroptosis DEGs were used for functional enrichment analysis, including BP, CC, MP, and KEGG. Cellular iron ion homeostasis, iron ion transport, and cellular response to oxidative stress were significantly enriched in biological processes. Cellular iron homeostasis is tightly regulated to maximize iron supply in times of cellular iron deficiency and to limit iron supply and facilitate storage when cellular iron is adequate. Cellular iron ion homeostasis (Anderson and Frazer, 2017) refers to the process of maintaining the internal steady state of iron ions at the cellular level. Iron ions, as one of the metals whose homeostasis is essential for the physiological function of the brain, perturbed homeostasis, may be responsible for causing specific local cell death. It has been found that substantia nigra pars compacta iron levels are significantly elevated in PD disease progression. Elevated iron concentrations predispose the brain to oxidative stress. Iron ion transport (Anderson and Frazer, 2017) refers to the directed movement of iron ions into and out of the cell either within or between cells via transporters or other transport vehicles. Metal ion transporters are involved in maintaining the required levels of various metal ions in the cell (Bowers and Srai, 2018). Thus, increased iron levels in the substantia nigra pars compacta are associated with the transport of ferric ions. Cellular response to oxidative stress refers to any process that changes cell state or activity due to oxidative stress, usually caused by exposure to high levels of reactive oxygen species, such as superoxide anions, hydrogen peroxide (H2O2), and hydroxyl radicals. Iron in nigral neurons is primarily bound to the biopolymer neuromelanin (NM) (Zecca et al., 2001), and this binding may provide a degree of antioxidant defense (Belaidi and Bush, 2016). However, an imbalance in iron homeostasis, such that free iron exerts toxic effects, catalyzes the Fenton reaction and produces damaging reactive free radicals, causing oxidative stress and, ultimately, dopaminergic neurons (Ganz, 2003). The above three biological processes suggested that the enriched genes were associated with the imbalance of intracellular iron homeostasis in dopaminergic neurons and caused oxidative stress, which ultimately led to neuronal cell damage.
Pathways related to ferroptosis were significantly enriched in KEGG pathway analysis, including ferroptosis, PPAR signaling pathway, peroxisome, necroptosis and Toll-like receptor signaling pathway. Ferroptosis is considered a novel form of regulated cell death resulting from severe lipid peroxidation and depends on reactive oxygen species (ROS) production and iron overload (Dixon et al., 2012; Do Van et al., 2016). As nuclear receptors, PPAR signaling pathways (PPARs) are expressed in neurons and astrocytes of the central nervous system, and studies have confirmed that PPARs can play a neuroprotective role against oxidative damage, apoptosis, and neuroinflammation during the progression of PD (Heneka and Landreth, 2007; Iranpour et al., 2016). The peroxisome is an important organelle in this signaling pathway, and its biogenesis starts from the early peroxidase pex3, which can participate in key processes such as free radical detoxification (Wanders and Waterham, 2006). Increased levels of free iron lead to the formation of reactive hydroxyl radicals, resulting in an oxidative stress response that accelerates dopaminergic neuronal death, and pex3 can antagonize neuronal injury by free radical detoxification (Ganz, 2003). Necroptosis, a form of programmed necrosis, is crucial in nervous system inflammation and can be caused by Toll-like receptors (Zhang et al., 2019). Inflammatory responses mediated by Toll-like receptor signaling pathways can result from engagement of the TLR4 (included among the significantly enriched pathways) with associated ligands, allowing inflammatory factors to be released and causing inflammation (Kagan et al., 2008; Netea et al., 2009). In addition, proinflammatory cytokines released by microglia exacerbate neuronal iron deposition, increase neurologic iron overload, and ultimately exacerbate ferroptosis in dopaminergic neurons (Healy et al., 2016; Dachert et al., 2020). These findings demonstrate that 109 DEGs can be involved in ferroptosis-related processes, demonstrating the potential of these target genes as research targets.
The hub genes obtained from machine learning postscreening provide references for further exploring the core target genes of PD ferroptosis pathogenesis progression. TLR4 is one of the key targets of inflammasomes triggered by the Toll-like receptor signaling pathway in the KEGG pathway, which not only participates in neuroinflammation but also induces necroptosis (Netea et al., 2009). Misfolded α-synuclein activates microglia to release IL6 and promote intracellular iron accumulation in neurons (Sterling et al., 2022). Furthermore, it was found that when organotypic hippocampal cultures were exposed to ferrous ammonium sulfate, ferric ammonium citrate (FAC), or ferrocene, microglial activation became evident, as evidenced by increased ferritin expression in microglia, as well as IL6 proinflammatory factor release (Wang et al., 2013; Healy et al., 2016). IL6 is involved in the inflammatory response and the progression of iron accumulation in neuronal cells. ADIPOQ is an adipokine that acts as a metabolic controller involved in the metabolism of fatty acids (Yamauchi et al., 2002) and has antioxidant and anti-inflammatory effects (Liu et al., 2015). The activity of ADIPOQ involved in metabolism is induced by its three receptors, namely, T-cadherin, ADIPOQR1 and ADIPOQR2, but also by PPAR γ- α of the signaling cascade (Thundyil et al., 2012). ADIPOQR1 and ADIPOQR2 have been reported to be expressed in primary human astrocytes (Hillenbrand et al., 2012), which can mediate proinflammatory signaling in astrocytes by elevating IL6 (Wan et al., 2014). Investigation of this coding gene in PD has not been reported, which deserves our attention. Prostaglandin-endoperoxide synthase (PTGS) is also known as cyclooxygenase (Hla and Neilson, 1992). PTGS2 (COX2), a neuroinflammatory marker (NMS), plays a role in the occurrence of neurodegenerative diseases (Hoozemans et al., 2008). COX2 is induced in cells undergoing ferroptosis (Li et al., 2017). Therefore, COX2 can be considered one of the crucial markers of ferroptosis (Zuo et al., 2021). The ATG7 gene encodes an E1-like activating enzyme implicated in the regulation of organismal mitophagy (Barrientos-Riosalido et al., 2023). Studies have shown that silencing ATG7 expression can slow the proinflammatory response of microglia, thereby slowing the progression of inflammation-mediated neurotoxicity during PD (Burguillos et al., 2011; Friess et al., 2021). FADS2 is a fatty acid desaturase (FADS) gene family member. Its long-chain polyunsaturated fatty acids (PUFAs), which are involved in biosynthesis, are most highly expressed in the brain and play an essential role in inflammatory processes (Park et al., 2011; Fagerberg et al., 2014). Notably, the involvement of FADS2 in the progression of PD pathogenesis has not been reported, and this gene provides a potential target for investigation in our future studies.
Molecular docking results showed that quercetin (QCT) and 17 beta estradiol (E2) had high binding energies to all the hub genes (Table 5). QCT is a flavonoid with anti-inflammatory, antioxidant and anti-ferritin functions (Boots et al., 2008; Tang et al., 2020). In addition, it ameliorates mitochondrial dysfunction, one of the hallmarks of ferroptosis (Dixon et al., 2012; de Oliveira et al., 2016). The mechanism of QCT is to protect neurons by inhibiting microglial activation (Han et al., 2021). E2 has protective effects against many neurodegenerative diseases and mediates its effects through dopamine receptors (Brann et al., 2007; Varmazyar et al., 2019). A study has shown that E2 can activate autophagy by regulating the expression of UKL1, thereby preventing the α-abnormal accumulation of synuclein and exerting a neuronal protective effect (Varmazyar et al., 2019). This suggests that QCT and E2 may serve as potent ingredients to explore the regulatory impact on the pathogenesis of ferroptosis in PD.
Despite the identification of ferroptosis feature genes and hub genes related to PD by machine learning model screening, this study has some limitations that need to be addressed. The lack of in vivo and in vitro experiments to validate the regulatory relationship between the feature genes derived from the screen and natural products against ferroptosis in PD is a noteworthy limitation. Additionally, the potential therapeutic agents identified in this study require further comprehensive and detailed experimental analysis. Nevertheless, our study provides valuable insights into the exploration of PD ferroptosis feature genes and therapeutic agents by combining transcriptome data with the ferroptosis database. The machine learning model prediction was used to identify the feature genes, which were then combined with natural products of traditional Chinese medicine to explore potential therapeutic agents. These findings might pave the way for future research in this area.
5 Conclusion
As neurodegenerative disease, PD has a slowly progressive course and positive correlation between incidence and age. It has attracted increasing attention with the arrival of the global aging society. Ferroptosis, a regulated iron-dependent cell death pathway involving the fatal accumulation of lipid peroxides, shares several features with the pathophysiology of PD. Machine learning is becoming more widely applied to predicting and deciding new data. In the present study, we analyzed 3 integrated transcriptome datasets and found 109 DEGs related to ferroptosis in PD, and the related pathways were identified by GO and KEGG analyses. Subsequently, 29 feature genes were predicted after screening by machine learning. PPI network topology value analysis of feature genes found that TLR4, IL6, ADIPOQ, PTGS2, Atg7, and FADS2 may be hub genes in the progression of PD ferroptosis. Notably, we performed data mining based on reverse derivation for natural products related to the 29 feature genes and clarified the top 5 core active ingredients according to the “Overlapping Genes-Ingredients” network, followed by molecular docking for validation, suggesting that quercetin, 17-beta-estradiol, glycerin, trans-resveratrol, and tocopherol might serve as potential therapeutic agents against the ferroptosis process of PD.
Data availability statement
The datasets presented in this study can be found in online repositories. The names of the repository/repositories and accession number(s) can be found in the article/Supplementary Material.
Author contributions
PW, QC, ZT, SL, and MY designed this study. Data collection and analyzation were performed by PW, QC, BG, LW, and ZT. The manuscript was drafted by PW, QC, LW, and BG. Manuscript review and editing were performed by ML, SL, and MY. All authors contributed to the article and approved the submitted version.
Funding
This research was funded by the National Natural Science Foundation of China, Grant No. 82130117; the Inheritance and Innovation of TCM “Hundreds, Thousands, Tens of Thousands” Talent Project Qihuang Scholars Fund [(2018)12].
Conflict of interest
The authors declare that the research was conducted in the absence of any commercial or financial relationships that could be construed as a potential conflict of interest.
Publisher’s note
All claims expressed in this article are solely those of the authors and do not necessarily represent those of their affiliated organizations, or those of the publisher, the editors and the reviewers. Any product that may be evaluated in this article, or claim that may be made by its manufacturer, is not guaranteed or endorsed by the publisher.
Supplementary material
The Supplementary Material for this article can be found online at: https://www.frontiersin.org/articles/10.3389/fgene.2023.1231707/full#supplementary-material
References
Anderson, G. J., and Frazer, D. M. (2017). Current understanding of iron homeostasis. Am. J. Clin. Nutr. 106, 1559S–1566S. doi:10.3945/ajcn.117.155804
Ayton, S., Lei, P., Hare, D. J., Duce, J. A., George, J. L., Adlard, P. A., et al. (2015). Parkinson's disease iron deposition caused by nitric oxide-induced loss of beta-amyloid precursor protein. J. Neurosci. 35, 3591–3597. doi:10.1523/JNEUROSCI.3439-14.2015
Barrett, T., Wilhite, S. E., Ledoux, P., Evangelista, C., Kim, I. F., Tomashevsky, M., et al. (2013). NCBI GEO: Archive for functional genomics data sets--update. Nucleic Acids Res. 41, D991–D995. doi:10.1093/nar/gks1193
Barrientos-Riosalido, A., Real, M., Bertran, L., Aguilar, C., Martinez, S., Parada, D., et al. (2023). Increased hepatic ATG7 mRNA and ATG7 protein expression in nonalcoholic steatohepatitis associated with obesity. Int. J. Mol. Sci. 24, 1324. doi:10.3390/ijms24021324
Belaidi, A. A., and Bush, A. I. (2016). Iron neurochemistry in alzheimer's disease and Parkinson's disease: Targets for therapeutics. J. Neurochem. 139 (Suppl. 1), 179–197. doi:10.1111/jnc.13425
Belarbi, K., Cuvelier, E., Bonte, M. A., Desplanque, M., Gressier, B., Devos, D., et al. (2020). Glycosphingolipids and neuroinflammation in Parkinson's disease. Mol. Neurodegener. 15, 59. doi:10.1186/s13024-020-00408-1
Berman, H. M., Westbrook, J., Feng, Z., Gilliland, G., Bhat, T. N., Weissig, H., et al. (2000). The protein Data Bank. Nucleic Acids Res. 28, 235–242. doi:10.1093/nar/28.1.235
Bersuker, K., Hendricks, J. M., Li, Z., Magtanong, L., Ford, B., Tang, P. H., et al. (2019). The CoQ oxidoreductase FSP1 acts parallel to GPX4 to inhibit ferroptosis. Nature 575, 688–692. doi:10.1038/s41586-019-1705-2
Bloem, B. R., Okun, M. S., and Klein, C. (2021). Parkinson's disease. Lancet 397, 2284–2303. doi:10.1016/s0140-6736(21)00218-x
Boots, A. W., Haenen, G. R., and Bast, A. (2008). Health effects of quercetin: From antioxidant to nutraceutical. Eur. J. Pharmacol. 585, 325–337. doi:10.1016/j.ejphar.2008.03.008
Borrageiro, G., Haylett, W., Seedat, S., Kuivaniemi, H., and Bardien, S. (2018). A review of genome-wide transcriptomics studies in Parkinson's disease. Eur. J. Neurosci. 47, 1–16. doi:10.1111/ejn.13760
Bowers, K., and Srai, S. K. S. (2018). The trafficking of metal ion transporters of the Zrt- and Irt-like protein family. Traffic 19, 813–822. doi:10.1111/tra.12602
Brann, D. W., Dhandapani, K., Wakade, C., Mahesh, V. B., and Khan, M. M. (2007). Neurotrophic and neuroprotective actions of estrogen: Basic mechanisms and clinical implications. Steroids 72, 381–405. doi:10.1016/j.steroids.2007.02.003
Burguillos, M. A., Deierborg, T., Kavanagh, E., Persson, A., Hajji, N., Garcia-Quintanilla, A., et al. (2011). Caspase signalling controls microglia activation and neurotoxicity. Nature 472, 319–324. doi:10.1038/nature09788
Cao, J., Chen, X., Jiang, L., Lu, B., Yuan, M., Zhu, D., et al. (2020). DJ-1 suppresses ferroptosis through preserving the activity of S-adenosyl homocysteine hydrolase. Nat. Commun. 11, 1251. doi:10.1038/s41467-020-15109-y
Chen, G., Seukep, A. J., and Guo, M. (2020). Recent advances in molecular docking for the research and discovery of potential marine drugs. Mar. Drugs 18, 545. doi:10.3390/md18110545
Conrad, M., Angeli, J. P., Vandenabeele, P., and Stockwell, B. R. (2016). Regulated necrosis: Disease relevance and therapeutic opportunities. Nat. Rev. Drug Discov. 15, 348–366. doi:10.1038/nrd.2015.6
Conway, J. R., Lex, A., and Gehlenborg, N. (2017). UpSetR: an R package for the visualization of intersecting sets and their properties. Bioinformatics 33, 2938–2940. doi:10.1093/bioinformatics/btx364
Dachert, J., Ehrenfeld, V., Habermann, K., Dolgikh, N., and Fulda, S. (2020). Targeting ferroptosis in rhabdomyosarcoma cells. Int. J. Cancer 146, 510–520. doi:10.1002/ijc.32496
de Oliveira, M. R., Nabavi, S. M., Braidy, N., Setzer, W. N., Ahmed, T., and Nabavi, S. F. (2016). Quercetin and the mitochondria: A mechanistic view. Biotechnol. Adv. 34, 532–549. doi:10.1016/j.biotechadv.2015.12.014
Diao, C., Xi, Y., and Xiao, T. (2018). Identification and analysis of key genes in osteosarcoma using bioinformatics. Oncol. Lett. 15, 2789–2794. doi:10.3892/ol.2017.7649
Diener, C., Hart, M., Kehl, T., Becker-Dorison, A., Tanzer, T., Schub, D., et al. (2023). Time-resolved RNA signatures of CD4+ T cells in Parkinson's disease. Cell Death Discov. 9, 18. doi:10.1038/s41420-023-01333-0
Dixon, S. J., Lemberg, K. M., Lamprecht, M. R., Skouta, R., Zaitsev, E. M., Gleason, C. E., et al. (2012). Ferroptosis: An iron-dependent form of nonapoptotic cell death. Cell 149, 1060–1072. doi:10.1016/j.cell.2012.03.042
Do Van, B., Gouel, F., Jonneaux, A., Timmerman, K., Gele, P., Petrault, M., et al. (2016). Ferroptosis, a newly characterized form of cell death in Parkinson's disease that is regulated by PKC. Neurobiol. Dis. 94, 169–178. doi:10.1016/j.nbd.2016.05.011
Eberhardt, J., Santos-Martins, D., Tillack, A. F., and Forli, S. (2021). AutoDock Vina 1.2.0: New docking methods, expanded force field, and Python bindings. J. Chem. Inf. Model 61, 3891–3898. doi:10.1021/acs.jcim.1c00203
Fagerberg, L., Hallstrom, B. M., Oksvold, P., Kampf, C., Djureinovic, D., Odeberg, J., et al. (2014). Analysis of the human tissue-specific expression by genome-wide integration of transcriptomics and antibody-based proteomics. Mol. Cell Proteomics 13, 397–406. doi:10.1074/mcp.M113.035600
Falchetti, M., Prediger, R. D., and Zanotto-Filho, A. (2020). Classification algorithms applied to blood-based transcriptome meta-analysis to predict idiopathic Parkinson's disease. Comput. Biol. Med. 124, 103925. doi:10.1016/j.compbiomed.2020.103925
Fang, S., Dong, L., Liu, L., Guo, J., Zhao, L., Zhang, J., et al. (2021). Herb: A high-throughput experiment- and reference-guided database of traditional Chinese medicine. Nucleic Acids Res. 49, D1197–D1206. doi:10.1093/nar/gkaa1063
Friedman, J., Hastie, T., and Tibshirani, R. (2010). Regularization paths for generalized linear models via coordinate descent. J. Stat. Softw. 33, 1–22. doi:10.18637/jss.v033.i01
Friess, L., Cheray, M., Keane, L., Grabert, K., and Joseph, B. (2021). Atg7 deficiency in microglia drives an altered transcriptomic profile associated with an impaired neuroinflammatory response. Mol. Brain 14, 87. doi:10.1186/s13041-021-00794-7
Ganz, T. (2003). Hepcidin, a key regulator of iron metabolism and mediator of anemia of inflammation. Blood 102, 783–788. doi:10.1182/blood-2003-03-0672
Gustavsson, E. K., Zhang, D., Reynolds, R. H., Garcia-Ruiz, S., and Ryten, M. (2022). ggtranscript: an R package for the visualization and interpretation of transcript isoforms using ggplot2. Bioinformatics 38, 3844–3846. doi:10.1093/bioinformatics/btac409
Han, X., Xu, T., Fang, Q., Zhang, H., Yue, L., Hu, G., et al. (2021). Quercetin hinders microglial activation to alleviate neurotoxicity via the interplay between NLRP3 inflammasome and mitophagy. Redox Biol. 44, 102010. doi:10.1016/j.redox.2021.102010
Hayes, M. T., Brown, V., Tan, E. J., Chevalier, A., D'Souza, M., Rissel, C., et al. (2019). Patterns and costs of health-care utilisation in Australian children: The first 5 years. Am. J. Med. 132, 802–808. doi:10.1111/jpc.14292
Healy, S., McMahon, J., Owens, P., and FitzGerald, U. (2016). Significant glial alterations in response to iron loading in a novel organotypic hippocampal slice culture model. Sci. Rep. 6, 36410. doi:10.1038/srep36410
Heneka, M. T., and Landreth, G. E. (2007). PPARs in the brain. Biochim. Biophys. Acta 1771, 1031–1045. doi:10.1016/j.bbalip.2007.04.016
Hillenbrand, A., Weiss, M., Knippschild, U., Wolf, A. M., and Huber-Lang, M. (2012). Sepsis-induced adipokine change with regard to insulin resistance. Int. J. Inflam. 2012, 972368. doi:10.1155/2012/972368
Hla, T., and Neilson, K. (1992). Human cyclooxygenase-2 cDNA. Proc. Natl. Acad. Sci. U. S. A. 89, 7384–7388. doi:10.1073/pnas.89.16.7384
Hoozemans, J. J., Rozemuller, J. M., van Haastert, E. S., Veerhuis, R., and Eikelenboom, P. (2008). Cyclooxygenase-1 and -2 in the different stages of Alzheimer's disease pathology. Curr. Pharm. Des. 14, 1419–1427. doi:10.2174/138161208784480171
Iranpour, N., Zandifar, A., Farokhnia, M., Goguol, A., Yekehtaz, H., Khodaie-Ardakani, M. R., et al. (2016). The effects of pioglitazone adjuvant therapy on negative symptoms of patients with chronic schizophrenia: A double-blind and placebo-controlled trial. Hum. Psychopharmacol. 31, 103–112. doi:10.1002/hup.2517
Jankovic, J. (2008). Parkinson's disease: Clinical features and diagnosis. J. Neurol. Neurosurg. Psychiatry 79, 368–376. doi:10.1136/jnnp.2007.131045
Jian, X., Zhao, G., Chen, H., Wang, Y., Li, J., Xie, L., et al. (2022). Revealing a novel contributing landscape of ferroptosis-related genes in Parkinson's disease. Comput. Struct. Biotechnol. J. 20, 5218–5225. doi:10.1016/j.csbj.2022.09.018
Kagan, J. C., Su, T., Horng, T., Chow, A., Akira, S., and Medzhitov, R. (2008). TRAM couples endocytosis of Toll-like receptor 4 to the induction of interferon-β. Nat. Immunol. 9, 361–368. doi:10.1038/ni1569
Kim, J. Y., Lee, S. Y., Kim, G. G., Song, H. I., Jang, M. M., Lee, C. S., et al. (2023a). Validation and application of new NGS-based HLA genotyping to clinical diagnostic practice. HLA 101, 496–506. doi:10.1111/tan.15003
Kim, S., Chen, J., Cheng, T., Gindulyte, A., He, J., He, S., et al. (2023b). PubChem 2023 update. Nucleic Acids Res. 51, D1373–D1380. doi:10.1093/nar/gkac956
Lesnick, T. G., Papapetropoulos, S., Mash, D. C., Ffrench-Mullen, J., Shehadeh, L., de Andrade, M., et al. (2007). A genomic pathway approach to a complex disease: Axon guidance and Parkinson disease. PLoS Genet. 3, e98. doi:10.1371/journal.pgen.0030098
Li, Q., Han, X., Lan, X., Gao, Y., Wan, J., Durham, F., et al. (2017). Inhibition of neuronal ferroptosis protects hemorrhagic brain. JCI Insight 2, e90777. doi:10.1172/jci.insight.90777
Li, Y., Maher, P., and Schubert, D. (1997). A role for 12-lipoxygenase in nerve cell death caused by glutathione depletion. Neuron 19, 453–463. doi:10.1016/s0896-6273(00)80953-8
Liu, Y., Palanivel, R., Rai, E., Park, M., Gabor, T. V., Scheid, M. P., et al. (2015). Adiponectin stimulates autophagy and reduces oxidative stress to enhance insulin sensitivity during high-fat diet feeding in mice. Diabetes 64, 36–48. doi:10.2337/db14-0267
Moni, M. A., Rana, H. K., Islam, M. B., Ahmed, M. B., Xu, H., Hasan, M. A. M., et al. (2019). A computational approach to identify blood cell-expressed Parkinson's disease biomarkers that are coordinately expressed in brain tissue. Comput. Biol. Med. 113, 103385. doi:10.1016/j.compbiomed.2019.103385
Netea, M. G., Nold-Petry, C. A., Nold, M. F., Joosten, L. A., Opitz, B., van der Meer, J. H., et al. (2009). Differential requirement for the activation of the inflammasome for processing and release of IL-1beta in monocytes and macrophages. Blood 113, 2324–2335. doi:10.1182/blood-2008-03-146720
Park, W. J., Kothapalli, K. S., Lawrence, P., and Brenna, J. T. (2011). FADS2 function loss at the cancer hotspot 11q13 locus diverts lipid signaling precursor synthesis to unusual eicosanoid fatty acids. PLoS One 6, e28186. doi:10.1371/journal.pone.0028186
Qin, C., Zhang, S., Zhao, Y., Ding, X., Yang, F., and Zhao, Y. (2023). Diagnostic value of metagenomic next-generation sequencing in sepsis and bloodstream infection. Front. Cell Infect. Microbiol. 13, 1117987. doi:10.3389/fcimb.2023.1117987
Ritchie, M. E., Phipson, B., Wu, D., Hu, Y., Law, C. W., Shi, W., et al. (2015). Limma powers differential expression analyses for RNA-sequencing and microarray studies. Nucleic Acids Res. 43, e47. doi:10.1093/nar/gkv007
Robin, X., Turck, N., Hainard, A., Tiberti, N., Lisacek, F., Sanchez, J. C., et al. (2011). pROC: an open-source package for R and S+ to analyze and compare ROC curves. BMC Bioinforma. 12, 77. doi:10.1186/1471-2105-12-77
Ru, J., Li, P., Wang, J., Zhou, W., Li, B., Huang, C., et al. (2014). Tcmsp: A database of systems pharmacology for drug discovery from herbal medicines. J. Cheminform 6, 13. doi:10.1186/1758-2946-6-13
Schilter, K. F., Smith, B. A., Nie, Q., Stoll, K., Felix, J. C., Jarzembowski, J. A., et al. (2023). Analytical validation and implementation of a pan cancer next-generation sequencing panel, CANSeq(TM)Kids for molecular profiling of childhood malignancies. Front. Genet. 14, 1067457. doi:10.3389/fgene.2023.1067457
Sertbas, M., and Ulgen, K. O. (2018). Unlocking human brain metabolism by genome-scale and multiomics metabolic models: Relevance for neurology research, health, and disease. OMICS 22, 455–467. doi:10.1089/omi.2018.0088
Shen, Y., and Hao, X. (2020). Natural product sciences: An integrative approach to the innovations of plant natural products. Sci. China Life Sci. 63, 1634–1650. doi:10.1007/s11427-020-1799-y
Solayman, M., Islam, M. A., Alam, F., Khalil, M. I., Kamal, M. A., and Gan, S. H. (2017). Natural products combating neurodegeneration: Parkinson's disease. Curr. Drug Metab. 18, 50–61. doi:10.2174/1389200217666160709204826
Sterling, J. K., Kam, T. I., Guttha, S., Park, H., Baumann, B., Mehrabani-Tabari, A. A., et al. (2022). Interleukin-6 triggers toxic neuronal iron sequestration in response to pathological α-synuclein. Cell Rep. 38, 110358. doi:10.1016/j.celrep.2022.110358
Tang, S. M., Deng, X. T., Zhou, J., Li, Q. P., Ge, X. X., and Miao, L. (2020). Pharmacological basis and new insights of quercetin action in respect to its anti-cancer effects. Biomed. Pharmacother. 121, 109604. doi:10.1016/j.biopha.2019.109604
Thundyil, J., Pavlovski, D., Sobey, C. G., and Arumugam, T. V. (2012). Adiponectin receptor signalling in the brain. Br. J. Pharmacol. 165, 313–327. doi:10.1111/j.1476-5381.2011.01560.x
Varmazyar, R., Noori-Zadeh, A., Rajaei, F., Darabi, S., and Bakhtiyari, S. (2019). 17 beta-estradiol oxidative stress attenuation and autophagy-induced dopaminergic neuroprotection. Cell J. 21, 1–6. doi:10.22074/cellj.2019.5799
Walter, W., Sanchez-Cabo, F., and Ricote, M. (2015). GOplot: an R package for visually combining expression data with functional analysis. Bioinformatics 31, 2912–2914. doi:10.1093/bioinformatics/btv300
Wan, Z., Mah, D., Simtchouk, S., Klegeris, A., and Little, J. P. (2014). Globular adiponectin induces a pro-inflammatory response in human astrocytic cells. Biochem. Biophys. Res. Commun. 446, 37–42. doi:10.1016/j.bbrc.2014.02.077
Wanders, R. J., and Waterham, H. R. (2006). Peroxisomal disorders: The single peroxisomal enzyme deficiencies. Biochim. Biophys. Acta 1763, 1707–1720. doi:10.1016/j.bbamcr.2006.08.010
Wang, J., Song, N., Jiang, H., Wang, J., and Xie, J. (2013). Pro-inflammatory cytokines modulate iron regulatory protein 1 expression and iron transportation through reactive oxygen/nitrogen species production in ventral mesencephalic neurons. Biochim. Biophys. Acta 1832, 618–625. doi:10.1016/j.bbadis.2013.01.021
Wang, Z. L., Yuan, L., Li, W., and Li, J. Y. (2022). Ferroptosis in Parkinson's disease: Glia-neuron crosstalk. Trends Mol. Med. 28, 258–269. doi:10.1016/j.molmed.2022.02.003
Yamauchi, T., Kamon, J., Minokoshi, Y., Ito, Y., Waki, H., Uchida, S., et al. (2002). Adiponectin stimulates glucose utilization and fatty-acid oxidation by activating AMP-activated protein kinase. Nat. Med. 8, 1288–1295. doi:10.1038/nm788
Yan, H. F., Zou, T., Tuo, Q. Z., Xu, S., Li, H., Belaidi, A. A., et al. (2021). Ferroptosis: Mechanisms and links with diseases. Signal Transduct. Target Ther. 6, 49. doi:10.1038/s41392-020-00428-9
Ye, M., Luo, G., Ye, D., She, M., Sun, N., Lu, Y. J., et al. (2021). Network pharmacology, molecular docking integrated surface plasmon resonance technology reveals the mechanism of Toujie Quwen Granules against coronavirus disease 2019 pneumonia. Phytomedicine 85, 153401. doi:10.1016/j.phymed.2020.153401
Yigit, E. N., Sonmez, E., Yuksel, I., Aksan Kurnaz, I., and Cakir, T. (2023). A transcriptome based approach to predict candidate drug targets and drugs for Parkinson's disease using an in vitro 6-OHDA model. Mol. Omics 19, 218–228. doi:10.1039/d2mo00267a
Yu, G., Wang, L. G., Han, Y., and He, Q. Y. (2012). clusterProfiler: an R package for comparing biological themes among gene clusters. OMICS 16, 284–287. doi:10.1089/omi.2011.0118
Zecca, L., Gallorini, M., Schunemann, V., Trautwein, A. X., Gerlach, M., Riederer, P., et al. (2001). Iron, neuromelanin and ferritin content in the substantia nigra of normal subjects at different ages: Consequences for iron storage and neurodegenerative processes. J. Neurochem. 76, 1766–1773. doi:10.1046/j.1471-4159.2001.00186.x
Zhang, H., Bai, L., He, J., Zhong, L., Duan, X., Ouyang, L., et al. (2017). Recent advances in discovery and development of natural products as source for anti-Parkinson's disease lead compounds. Eur. J. Med. Chem. 141, 257–272. doi:10.1016/j.ejmech.2017.09.068
Zhang, S., Su, Y., Ying, Z., Guo, D., Pan, C., Guo, J., et al. (2019). RIP1 kinase inhibitor halts the progression of an immune-induced demyelination disease at the stage of monocyte elevation. Proc. Natl. Acad. Sci. U. S. A. 116, 5675–5680. doi:10.1073/pnas.1819917116
Zheng, B., Liao, Z., Locascio, J. J., Lesniak, K. A., Roderick, S. S., Watt, M. L., et al. (2010). PGC-1α, a potential therapeutic target for early intervention in Parkinson's disease. Sci. Transl. Med. 2, 52ra73. doi:10.1126/scitranslmed.3001059
Zhou, N., Yuan, X., Du, Q., Zhang, Z., Shi, X., Bao, J., et al. (2023). FerrDb V2: Update of the manually curated database of ferroptosis regulators and ferroptosis-disease associations. Nucleic Acids Res. 51, D571–D582. doi:10.1093/nar/gkac935
Keywords: Parkinson’s disease, ferroptosis, transcriptomics, machine learning, natural product, ingredient
Citation: Wang P, Chen Q, Tang Z, Wang L, Gong B, Li M, Li S and Yang M (2023) Uncovering ferroptosis in Parkinson’s disease via bioinformatics and machine learning, and reversed deducing potential therapeutic natural products. Front. Genet. 14:1231707. doi: 10.3389/fgene.2023.1231707
Received: 30 May 2023; Accepted: 27 June 2023;
Published: 06 July 2023.
Edited by:
Ke Ma, Shandong University of Traditional Chinese Medicine, ChinaReviewed by:
Xinglong Yang, The First Affiliated Hospital of Kunming Medical University, ChinaXu Zhai, China Academy of Chinese Medical Sciences, China
Copyright © 2023 Wang, Chen, Tang, Wang, Gong, Li, Li and Yang. This is an open-access article distributed under the terms of the Creative Commons Attribution License (CC BY). The use, distribution or reproduction in other forums is permitted, provided the original author(s) and the copyright owner(s) are credited and that the original publication in this journal is cited, in accordance with accepted academic practice. No use, distribution or reproduction is permitted which does not comply with these terms.
*Correspondence: Shaodan Li, bHNkMzAxQDEyNi5jb20=; Minghui Yang, eW1oOTY1MUBzaW5hLmNvbQ==
†These authors have contributed equally to this work and share first authorship