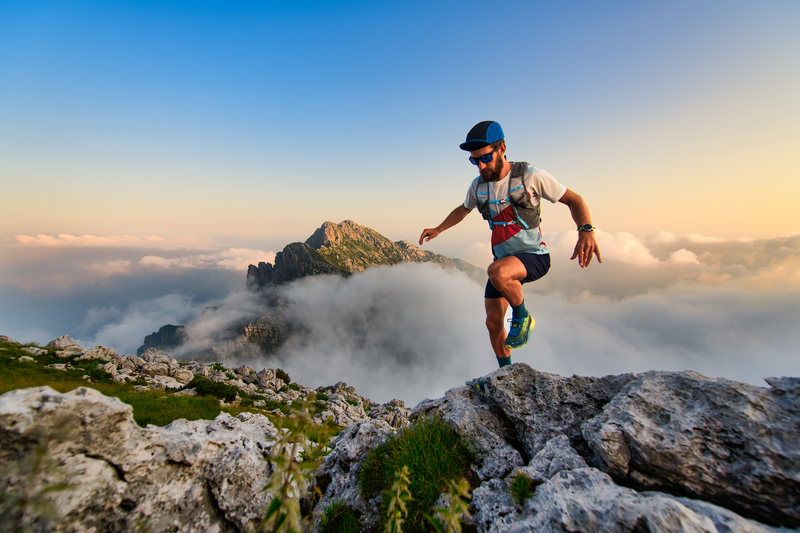
95% of researchers rate our articles as excellent or good
Learn more about the work of our research integrity team to safeguard the quality of each article we publish.
Find out more
ORIGINAL RESEARCH article
Front. Genet. , 24 February 2023
Sec. Human and Medical Genomics
Volume 14 - 2023 | https://doi.org/10.3389/fgene.2023.1130411
This article is part of the Research Topic Advances in the Genetic and Epigenetic of Bone and Joint Diseases View all 10 articles
Background: Existing studies have indicated that mitochondrial dysfunction may contribute to osteoarthritis (OA) development. However, the causal association between mitochondrial DNA (mtDNA) characterization and OA has not been extensively explored.
Methods: Two-sample Mendelian randomization was performed to calculate the impact of mitochondrial genomic variations on overall OA as well as site-specific OA, with multiple analytical methods inverse variance weighted (IVW), weighted median (WM), MR-Egger and MR-robust adjusted profile score (MR-RAPS).
Results: Genetically determined mitochondrial heteroplasmy (MtHz) and mtDNA abundance were not causally associated with overall OA. In site-specific OA analyses, the causal effect of mtDNA abundance on other OA sites, including hip, knee, thumb, hand, and finger, had not been discovered. There was a suggestively protective effect of MtHz on knee OA IVW OR = 0.632, 95% CI: 0.425–0.939, p-value = 0.023. No causal association between MtHz and other different OA phenotypes was found.
Conclusion: MtHz shows potential to be a novel therapeutic target and biomarker on knee OA development. However, the variation of mtDNA abundance was measured from leukocyte in blood and the levels of MtHz were from saliva samples rather than cartilage or synovial tissues. Genotyping samples from synovial and cartilage can be a focus to further exploration.
Osteoarthritis (OA) is a common joint disease with degenerative changes in articular cartilage and remains major impact on public health worldwide (Nelson, 2018). According to the estimation of the Global Burden of Disease Study (GBD), nearly 300 million individuals throughout the world are suffering from OA, and the number is still increasing. OA is one of the leading causes of pain, disability, and great socioeconomic burden in developed countries (Glyn-Jones et al., 2015). However, the mechanism of OA remains unclear. Identifying risk factors such as a disorder-related biomarker is essential to understand OA pathogenesis, decrease the incidence of the disease and develop efficiency prevention strategies.
Mitochondria are complex multifunctional organelles involved in various cell functions, e.g., heat regulation, calcium homeostasis, reactive oxygen species (ROS) production, and proinflammatory cytokines production, the damage of which may affect chondrocyte health at an extent (Blanco et al., 2011; Fernandez-Moreno et al., 2017; Blanco et al., 2018). They have their own genome (mitochondrial DNA, mtDNA) which contain 37 genes and encode 13 proteins, 22 transfer RNAs (tRNAs) and two ribosomal RNAs (rRNAs) (Anderson et al., 1981). As multicopy genome, sequence mutation and copy number variation of mtDNA are prevalent in population (McArdle et al., 2013; McGuire, 2019). As one of the mtDNA quantity characteristics, mtDNA abundance is recognized as a rough estimation for the number of mitochondria (Castellani et al., 2020). Previous studies provided supportive evidence for extensive pathogenicity of mtDNA abundance (Frahm et al., 2005; Hu et al., 2016). As one of the mtDNA quality characteristics, mitochondrial herteroplasmy (MtHz), where mtDNA with distinct sequences coexist, has been found in a large spectrum of human disease, including classical mitochondrial diseases and complex disorders (Ye et al., 2014). MtDNA abundance and MtHz can integrate many aspects of mitochondrial function and serve as promising biomarkers in probing interactions between mitochondria and disorders (Castellani et al., 2020; Tian et al., 2021).
In a recent review, OA was recognized as a potential mitochondrial disease considering the impact of mitochondrial dysfunction on cartilage degradation (Fernandez-Moreno et al., 2022). However, the causality between mitochondria and OA has rarely been investigated. Therefore, the goal of our study was to probe causal effects of characterization of mtDNA quantity and quality on OA development which is crucial to understand the role of mitochondria in OA etiology. A two-sample Mendelian randomization (MR) analysis was performed to investigate potential causal associations between mtDNA abundance and MtHz with OA using summary statistics from large-scale genome-wide association study (GWAS). MR-Steiger test was applied to ascertain whether variation of mtDNA characterization is a cause or a consequence of OA development.
The design of our research is displayed in Figure 1. We adopted a two-sample MR design to compute the causal effect of the characterization of mtDNA quantity and quality on overall OA and site-specific OA separately (Boer et al., 2021). Genetic association estimates for mtDNA abundance were derived from the United Kingdom biobank study (N = 291,950) (Hagg et al., 2021). Single Nucleotide Polymorphisms (SNPs) associated with MtHz were obtained from the 23andME research program (N = 982,072), a personal genomics and biotechnology company (Nandakumar et al., 2021). The participants included in both GWASs were of European ancestry.
SNPs serving as instrumental variables (IVs) for MtHz and mtDNA abundance, all reach genome-wide significance (p < 5
Summary statistics data on overall OA and its specific sites, comprising knee, hip, spine, thumb, and hand OA, were obtained from the latest publicly available GWAS of OA. This GWAS covered nine populations with up to 826,690 participants (177,517 OA patients) (Boer et al., 2021). The definition of OA satisfied the criteria of Genetics of Osteoarthritis (GO) including self-reported status, hospital diagnosed, ICD10 codes or radiographic as defined by the TREAT-OA consortium (Boer et al., 2021). All studies contributing data to our analyses were approved by the relevant ethics committees, and all study participants in these studies provided written, informed consent.
Two-sample MR analysis was executed in R software (R version 4.3.0) with the TwoSampleMR (version 0.5.6), MR-PRESSO (version 1.0) and mr. raps (version 0.2) R packages (Hemani et al., 2018; Verbanck et al., 2018). The methods applied in our research are presented in Figure 2. MR-steiger test was applied to infer causal direction between traits under investigation (Hemani et al., 2017). Four methodologies including inverse variance weighted (IVW), weighted median (WM), MR-Egger and MR-robust adjusted profile score (MR-RAPS) were employed to estimate causality between mtDNA abundance and MtHz and OA. IVW was taken as a primary MR analysis method in our research, which is based on the hypothesis that all selected genetic instruments are valid and give an overall causal estimate strengthening causal inference (Lawlor et al., 2008; Burgess et al., 2013). MR-Egger regression takes presence of directional pleiotropy into consideration and measures horizontal pleiotropy with regression intercept, whereas the results of this method are susceptible to outlying genetic variants (Bowden et al., 2015). Weighted Median based on hypothesis that at least 50% of the variants are valid, improves power of causal effect detection but reduces precision (Bowden et al., 2016). MR-RAPS applies robust adjusted profile scores to correct for pleiotropy and makes our results more reliable (Hartwig et al., 2017).
Several sensitivity analyses were applied to detect and correct for heterogeneity and pleiotropy. MR-PRESSO was conducted to detect the existence of horizontal pleiotropy and correct the causal estimate affected by possible pleiotropic outliers (Verbanck et al., 2018). Corresponding p-values were derived based on 1,000 simulations (Verbanck et al., 2018). And the estimation of MR Egger regression intercept was also employed to reflect presence of pleiotropy (p < 0.05) (Burgess and Thompson, 2017). IVW method was utilized to investigate heterogeneity. The level of heterogeneity was quantified by Cochran Q statistics (Carnegie et al., 2020). Leave-one-out sensitivity analysis was performed to identify possibly influential SNPs, which repeated MR analysis with each SNP excluded in turn (Carnegie et al., 2020). As five separate outcomes were tested in our study, main results had statistical significance at p-value<0.01 (0.05/5) after Bonferroni correction.
Fifteen LD-independent genetic variants were taken for repeated MR analysis (Supplementary Table S1). We extracted data of above-mentioned SNPs from summary GWAS of outcome traits (overall OA and specific-site OA) and one SNP (rs2286639) was removed in all outcomes except finger OA due to the effect of non-concordant alleles (e.g., A/G vs A/C). In finger OA, two SNP (rs2286639, rs3702096) were excluded for absence of proxy SNPs on the online platform SNiPA (https://snipa.helmholtzmuenchen.de/snipa3/). The mean F-statistic was 36.357 which was above the threshold F value of 10. In total, there were 14 IVs for overall, knee, hip, thumb, and hand OA and 13 IVs for finger OA.
Results of the casual association between MtHz and OA are summarized in Table 1. The primary IVW analysis provided no evidence for the casual association between MtHz and overall OA [odds ratio (OR) = 0.852, 95% confidence interval (CI): 0.664–1.093, p= 0.208]. The results of WM method, MR-RAPS and MR-Egger were consistent with the result of IVW. Both MR-Egger and MR-PRESSO reported no existence of horizontal pleiotropy (Egger-intercept: p > 0.05; MR-PRESSO global heterogeneity: p > 0.05). Cochran’s Q test did not detect heterogeneity in overall OA (p= 0.495 > 0.05).
In site-specific OA analyses, the nominally significant results of IVW analysis suggested that MtHz was a potentially protective factor for knee OA (OR = 0.632, 95% CI: 0.425–0.939, p= 0.023, Table 1). MR-RAPS showed similar results with IVW analysis (OR = 0.629, 95% CI: 0.422–0.939, p= 0.023, Table 1). However, no relationship between MtHz and other OA sites, including hip OA (IVW OR = 0.738, 95% CI: 0.447–1.221, p= 0.237), hand OA (IVW OR = 1.357, 95% CI: 0.687–2.681, p= 0.379), thumb OA (IVW OR = 1.270, 95% CI: 0.506–3.188, p= 0.611) or finger OA (IVW OR = 0.483, 95% CI: 0.090–2.588, p= 0.395) was observed in IVW model (Table 1). MR-PRESSO was performed to test for horizontal pleiotropy, and no outliers was identified. The results of MR-Egger intercept were consistent with the MR-PRESSO results (intercept p > 0.05). The heterogeneity was tested by Cochran’s Q test and MR-PRESSO global heterogeneity test, providing no evidence about the existence of heterogeneity. The MR-Steiger results supported that these SNPs were more predictive of the exposure than of the outcome (p < 0.05, Supplementary Table S3). The results of leave-one-out sensitivity analysis and forest plots demonstrated that our study in genetically prediction was robust (Supplementary Figure S1–S3).
After LD test, 47 independent genetic variants were chosen for two-sample MR analysis. Several genetic variants could not be matched in summary statistic GWASs of knee (rs35734242), finger (rs1065853), and hand OA (rs12451555). We searched their proxy SNPs on SNiPA and those whose proxy SNPs were absent on SNiPA were excluded. The mean F-statistic for IVs of mtDNA abundance was 93.380, which was above the threshold 10 (Supplementary Table S2). Finally, the SNPS were selected as IVs for thumb, hip, overall OA was 47, and 46 SNPS for hand, finger, knee. Regarding overall OA, MR-PRESSO identified two outliers s (rs59488041, rs12924138) and therefore removed them for repeated MR analysis. In the presence of heterogeneity (Cochran’s Q: p= 0.033) and absence of pleiotropy (Egger-intercept: p= 0.687 > 0.05), the results of WM analysis on overall OA were nominally significant (OR = 1.108, 95% CI: 1.001–1,226, p= 0.048, Table 2). IVW method in random effects model was utilized to correct results potentially impacted by heterogeneity, and the results suggested that genetically elevated mtDNA abundance was not casually associated with overall OA (OR = 1.040, 95% CI: 0.963–1.125, p= 0.318). MR-RAPS analysis agreed with the results of IVW analysis (OR = 1.041, 95% CI: 0.974–1.112, p= 0.228, Table 2).
With respect to site-specific OA, we observed that genetically determined MtHz was not causally associated with knee OA (OR = 1.034, 95% CI: 0.915–1.168, p= 0.592), thumb OA (OR = 1.148, 95% CI: 0.880–1.499, p= 0.309), hand OA (OR = 1.152, 95% CI: 0.969–1.369, p = 0.108) and finger OA (OR = 1.004, 95% CI: 0.647–1.556, p= 0.987) in IVW model. The results are presented in Table 2. Both MR-Egger and MR-PRESSO reported no existence of horizontal pleiotropy (Egger-intercept: p > 0.05; MR-PRESSO global heterogeneity: p > 0.05). Cochran’s Q test did not detect heterogeneity in above-mentioned outcome traits (p > 0.05). For the analysis of hip OA, rs16978036 were excluded from MR-PRESSO analysis. In presence of horizontal pleiotropy (p= 0.027) and absence of heterogeneity (p= 0.062), the nominally significant result of MR-Egger method was found (OR = 0.616, 95% CI: 0.399–0.950, p= 0.034). However, no evidence about causal relationship between the exposure and hip OA was observed with IVW method and MR-RAPS method (IVW: OR = 0.992, 95% CI: 0.857–1.152, p= 0.931; MR-RAPS: OR = 0.993, 95% CI: 0.873–1.130, p= 0.919) (Table 2). The results of MR-Stegier test were in Supplementary Table S3.
Scatter plots, forest plots and leave-on-out sensitivity analysis plots were displayed in Supplementary Figure S4–6. The results of leave-one-out analysis implicated that the selected genetic variants potentially impacted the pooled results, which suggested that careful interpretations for the results was crucial.
The causal roles of MtHz and mtDNA abundance in OA pathogenesis were poorly studied in previous works. To our best knowledge, this is the first MR study to evaluate the causal association between mitochondrial genome traits and OA. Four MR methods were employed to estimate the causal association between mtDNA characterization and OA. The results of MR steiger test verified the causal direction of our research (MtHz and mtDNA abundance were exposure and OA were outcome). No effect of mtDNA abundance and MtHz on overall OA was detected. In subgroup analysis, a suggestively protective role of MtHz on knee OA was observed, but not on other sites. And we did not find that mtDNA abundance was causally associated with any site-specific OA.
Although mitochondria is widely recognized as an important factor of OA development (Blanco et al., 2011), the role of MtHz has not been thoroughly investigated (Suliman and Piantadosi, 2016). Indeed, heteroplasmic mutations in mtDNA are often pathogenetic (Ye et al., 2014). An animal study has indicated that the state of heteroplasmy itself was deleterious when the two mtDNA sequences contain no pathogenic variants (Sharpley et al., 2012). However, there is potentially a particular level threshold for MtHz (McCormick et al., 2020). For instance, when the A3243G mutation in mitochondrial DNA is present in more than 10%, patients can manifest Type 2 diabetes (Wallace, 2005). And low-frequency mtDNA variants (0.2%–2% heteroplasmy) are extensively presented in healthy subjects (Payne et al., 2013). Furthermore, MtHz can be beneficial in health promotion as an intermediate state in emergence of novel mtDNA haplogroups (Wallace, 2016). In previous study, MtHz was found to be significantly relevant to several haplogroups (haplogroup H, J, K, T, U and X) with different characterizations among haplogroups (Nandakumar et al., 2021). Mitochondrial haplogroup J has been extensively found to mediate the development of OA (5). A Spanish cohort-study (
However, the reason that MtHz showed diverse effects on different sites of OA remains unclear. So far, we did not find relevant research that investigated the relationship between MtHz and hand, finger, and thumb OA. A case-control study suggested that mitochondrial haplogroups was associated with hip OA (Rego et al., 2010) (OR = 0.661, 95% CI: 0.440–0.993, p= 0.045), however, with no evidence on the causality. In addition, the data sources and sample size used in MR analysis varied in site-specific OA, which mainly included more knee OA cases and less other skeletal joints cases. More MR studies and additional GWAS covering more other site-specific OAs are needed to determine a role of MtHz in the risk of different OA sites.
MtDNA abundance has been regarded as a potential biomarker of mitochondrial function and plays a role in several human diseases (Castellani et al., 2020; Clyde, 2022). Existing literature has suggested that mtDNA abundance may involve in production of inflammatory mediators and regulation of immune function that can influence OA development (Blanco et al., 2018; Zhan et al., 2020). In addition, mtDNA abundance is also associated with sex, advanced age, and elevated BMI, which are also risk factors for OA (16). However, there are few studies to estimate the effects of mtDNA abundance on OA. Only two case-control studies in Asian population were found that reported an association between mtDNA copy number and OA, but their findings were inconsistent (Fang et al., 2014; Zhan et al., 2020). One in Thailand (
Our research applies MR methods to investigate causal relationships between mitochondrial genome characterization and OA. However, there are some limitations in our analysis. Firstly, we did not estimate causal effects of mitochondrial genome traits on OA stratified by gender. Mitochondrial genome are maternally inherited, and females may have lower MtHz than males from the perspective of mitochondrial inheritance (Nandakumar et al., 2021). Therefore, the effects of MtHz and mtDNA abundance on OA may differ in gender. Secondly, only participants of European descent are included in the study, but the impact of specific mtDNA variants on diseases could vary in different ethnic groups (Ruiz-Pesini et al., 2004). Additional MR studies on other ethnic groups are needed to probe a causal association between mtDNA characterization and OA. Thirdly, the genetic instruments for mtDNA abundance explain a relatively small amount of phenotypic variance (8.3%), IVs that can account for more variance of mtDNA abundance are warranted to draw robust conclusions. Besides, considering the Bonferroni correction of multiple independent tests, our findings about the association between MtHz and knee OA are deemed suggestive evidence of possible associations (0.01 < p< 0.05). Furthermore, the variation of mtDNA abundance and the levels of MtHz varied in different tissues and cell types. And in original GWASs, mtDNA abundance was measured from leukocyte in blood and MtHz from saliva samples rather than cartilage or synovial tissues. Considering that OA is mainly characterized by progressive loss of cartilage and synovial hyperplasia, the application of data from blood samples and saliva samples would limit the explanation ability of our study to a certain extent (Sellam and Berenbaum, 2010). Observational epidemiology studies exploring an association between concrete levels of MtHz and a risk of OA are needed to improve causal inference.
In conclusion, our MR analyses elucidated that MtHz is a suggestively protective factor of knee OA, implying that MtHz could be a genetically prediction factor and a therapeutic target in the development of knee OA. No causal association was found between mtDNA abundance and OA. Additional MR analyses are warranted to probe causal relationships between mitochondrial genome traits and OA stratified by gender. Moreover, GWAS covering more than one ethnic population are needed to detect the effect of characterization of mtDNA quantity and quality in different ethnic groups.
The original contributions presented in the study are included in the article/Supplementary Material, further inquiries can be directed to the corresponding authors.
HL mainly designed analysis and wrote the manuscript; BC, RG, DZ performed experiments and analysis; YY verified data and analysis results, JW, MY, YL supervised the entire project. All authors have read, provided critical feedback on intellectual content, and approved the final manuscript.
The authors thank all the participants and researchers for their participation in this MR study. All authors interpreted the data, critically revised the manuscript for important intellectual content, approved the final version of the manuscript, and agreed to be responsible for all aspects of the work. The corresponding author attests that all listed co-authors meet authorship criteria and that no others meeting the criteria have been omitted.
The authors declare that the research was conducted in the absence of any commercial or financial relationships that could be construed as a potential conflict of interest.
All claims expressed in this article are solely those of the authors and do not necessarily represent those of their affiliated organizations, or those of the publisher, the editors and the reviewers. Any product that may be evaluated in this article, or claim that may be made by its manufacturer, is not guaranteed or endorsed by the publisher.
The Supplementary Material for this article can be found online at: https://www.frontiersin.org/articles/10.3389/fgene.2023.1130411/full#supplementary-material
Anderson, S., Bankier, A. T., Barrell, B. G., de Bruijn, M. H., Coulson, A. R., Drouin, J., et al. (1981). Sequence and organization of the human mitochondrial genome. Nature 290 (5806), 457–465. doi:10.1038/290457a0
Blanco, F. J., Rego, I., and Ruiz-Romero, C. (2011). The role of mitochondria in osteoarthritis. Nat. Rev. Rheumatol. 7 (3), 161–169. doi:10.1038/nrrheum.2010.213
Blanco, F. J., Valdes, A. M., and Rego-Perez, I. (2018). Mitochondrial DNA variation and the pathogenesis of osteoarthritis phenotypes. Nat. Rev. Rheumatol. 14 (6), 327–340. doi:10.1038/s41584-018-0001-0
Boer, C. G., Hatzikotoulas, K., Southam, L., Stefansdottir, L., Zhang, Y., Coutinho de Almeida, R., et al. (2021). Deciphering osteoarthritis genetics across 826,690 individuals from 9 populations. Cell 184 (18), 6003–6005. doi:10.1016/j.cell.2021.11.003
Bowden, J., Davey Smith, G., and Burgess, S. (2015). Mendelian randomization with invalid instruments: Effect estimation and bias detection through egger regression. Int. J. Epidemiol. 44 (2), 512–525. doi:10.1093/ije/dyv080
Bowden, J., Davey Smith, G., Haycock, P. C., and Burgess, S. (2016). Consistent estimation in mendelian randomization with some invalid instruments using a weighted median estimator. Genet. Epidemiol. 40 (4), 304–314. doi:10.1002/gepi.21965
Burgess, S., Butterworth, A., and Thompson, S. G. (2013). Mendelian randomization analysis with multiple genetic variants using summarized data. Genet. Epidemiol. 37 (7), 658–665. doi:10.1002/gepi.21758
Burgess, S., Small, D. S., and Thompson, S. G. (2017). A review of instrumental variable estimators for Mendelian randomization. Stat. Methods Med. Res. 26 (5), 2333–2355. doi:10.1177/0962280215597579
Burgess, S., Thompson, S. G., and Collaboration, C. C. G. (2011). Avoiding bias from weak instruments in Mendelian randomization studies. Int. J. Epidemiol. 40 (3), 755–764. doi:10.1093/ije/dyr036
Burgess, S., and Thompson, S. G. (2017). Interpreting findings from Mendelian randomization using the MR-Egger method. Eur. J. Epidemiol. 32 (5), 377–389. doi:10.1007/s10654-017-0255-x
Carnegie, R., Zheng, J., Sallis, H. M., Jones, H. J., Wade, K. H., Evans, J., et al. (2020). Mendelian randomisation for nutritional psychiatry. Lancet Psychiatry 7 (2), 208–216. doi:10.1016/S2215-0366(19)30293-7
Castellani, C. A., Longchamps, R. J., Sun, J., Guallar, E., and Arking, D. E. (2020). Thinking outside the nucleus: Mitochondrial DNA copy number in health and disease. Mitochondrion 53, 214–223. doi:10.1016/j.mito.2020.06.004
Chen, L. Y., Lotz, M., Terkeltaub, R., and Liu-Bryan, R. (2018). Modulation of matrix metabolism by ATP-citrate lyase in articular chondrocytes. J. Biol. Chem. 293 (31), 12259–12270. doi:10.1074/jbc.RA118.002261
Clyde, D. (2022). Genomic insights into conifer evolution. Nat. Rev. Genet. 23 (3), 136. doi:10.1038/s41576-022-00452-1
Fang, H., Liu, X., Shen, L., Li, F., Liu, Y., Chi, H., et al. (2014). Role of mtDNA haplogroups in the prevalence of knee osteoarthritis in a southern Chinese population. Int. J. Mol. Sci. 15 (2), 2646–2659. doi:10.3390/ijms15022646
Fernandez-Moreno, M., Rego-Perez, I., and Blanco, F. J. (2022). Is osteoarthritis a mitochondrial disease? What is the evidence. Curr. Opin. Rheumatol. 34 (1), 46–53. doi:10.1097/BOR.0000000000000855
Fernandez-Moreno, M., Soto-Hermida, A., Vazquez-Mosquera, M. E., Cortes-Pereira, E., Relano, S., Hermida-Gomez, T., et al. (2017). Mitochondrial DNA haplogroups influence the risk of incident knee osteoarthritis in OAI and CHECK cohorts. A meta-analysis and functional study. Ann. Rheum. Dis. 76 (6), 1114–1122. doi:10.1136/annrheumdis-2016-210131
Frahm, T., Mohamed, S. A., Bruse, P., Gemund, C., Oehmichen, M., and Meissner, C. (2005). Lack of age-related increase of mitochondrial DNA amount in brain, skeletal muscle and human heart. Mech. Ageing Dev. 126 (11), 1192–1200. doi:10.1016/j.mad.2005.06.008
Glyn-Jones, S., Palmer, A. J., Agricola, R., Price, A. J., Vincent, T. L., Weinans, H., et al. (2015). Osteoarthr. Lancet. 386 (9991), 376–387. doi:10.1016/S0140-6736(14)60802-3
Hagg, S., Jylhava, J., Wang, Y., Czene, K., and Grassmann, F. (2021). Deciphering the genetic and epidemiological landscape of mitochondrial DNA abundance. Hum. Genet. 140 (6), 849–861. doi:10.1007/s00439-020-02249-w
Hartwig, F. P., Davey Smith, G., and Bowden, J. (2017). Robust inference in summary data Mendelian randomization via the zero modal pleiotropy assumption. Int. J. Epidemiol. 46 (6), 1985–1998. doi:10.1093/ije/dyx102
Hemani, G., Tilling, K., and Davey Smith, G. (2017). Orienting the causal relationship between imprecisely measured traits using GWAS summary data. PLoS Genet. 13 (11), e1007081. doi:10.1371/journal.pgen.1007081
Hemani, G., Zheng, J., Elsworth, B., Wade, K. H., Haberland, V., Baird, D., et al. (2018). The MR-Base platform supports systematic causal inference across the human phenome. Elife 7, e34408. doi:10.7554/eLife.34408
Hu, L., Yao, X., and Shen, Y. (2016). Altered mitochondrial DNA copy number contributes to human cancer risk: Evidence from an updated meta-analysis. Sci. Rep. 6, 35859. doi:10.1038/srep35859
Hudson, G., Panoutsopoulou, K., Wilson, I., Southam, L., Rayner, N. W., Arden, N., et al. (2013). No evidence of an association between mitochondrial DNA variants and osteoarthritis in 7393 cases and 5122 controls. Ann. Rheum. Dis. 72 (1), 136–139. doi:10.1136/annrheumdis-2012-201932
Kopinski, P. K., Janssen, K. A., Schaefer, P. M., Trefely, S., Perry, C. E., Potluri, P., et al. (2019). Regulation of nuclear epigenome by mitochondrial DNA heteroplasmy. Proc. Natl. Acad. Sci. U. S. A. 116 (32), 16028–16035. doi:10.1073/pnas.1906896116
Lawlor, D. A., Harbord, R. M., Sterne, J. A., Timpson, N., and Davey Smith, G. (2008). Mendelian randomization: Using genes as instruments for making causal inferences in epidemiology. Stat. Med. 27 (8), 1133–1163. doi:10.1002/sim.3034
McArdle, M. A., Finucane, O. M., Connaughton, R. M., McMorrow, A. M., and Roche, H. M. (2013). Mechanisms of obesity-induced inflammation and insulin resistance: Insights into the emerging role of nutritional strategies. Front. Endocrinol. (Lausanne) 4, 52. doi:10.3389/fendo.2013.00052
McCormick, E. M., Lott, M. T., Dulik, M. C., Shen, L., Attimonelli, M., Vitale, O., et al. (2020). Specifications of the ACMG/AMP standards and guidelines for mitochondrial DNA variant interpretation. Hum. Mutat. 41 (12), 2028–2057. doi:10.1002/humu.24107
McGuire, P. J. (2019). Mitochondrial dysfunction and the aging immune system. Biol. (Basel) 8 (2), 26. doi:10.3390/biology8020026
Nandakumar, P., Tian, C., O'Connell, J., andMe Research, T., Hinds, D., Paterson, A. D., et al. (2021). Nuclear genome-wide associations with mitochondrial heteroplasmy. Sci. Adv. 7 (12), eabe7520. doi:10.1126/sciadv.abe7520
Nelson, A. E. (2018). Osteoarthritis year in review 2017: Clinical. Osteoarthr. Cartil. 26 (3), 319–325. doi:10.1016/j.joca.2017.11.014
Payne, B. A., Wilson, I. J., Yu-Wai-Man, P., Coxhead, J., Deehan, D., Horvath, R., et al. (2013). Universal heteroplasmy of human mitochondrial DNA. Hum. Mol. Genet. 22 (2), 384–390. doi:10.1093/hmg/dds435
Rego, I., Fernandez-Moreno, M., Fernandez-Lopez, C., Gomez-Reino, J. J., Gonzalez, A., Arenas, J., et al. (2010). Role of European mitochondrial DNA haplogroups in the prevalence of hip osteoarthritis in Galicia, Northern Spain. Ann. Rheum. Dis. 69 (1), 210–213. doi:10.1136/ard.2008.105254
Rego-Perez, I., Fernandez-Moreno, M., Fernandez-Lopez, C., Arenas, J., and Blanco, F. J. (2008). Mitochondrial DNA haplogroups: Role in the prevalence and severity of knee osteoarthritis. Arthritis Rheum. 58 (8), 2387–2396. doi:10.1002/art.23659
Ruiz-Pesini, E., Mishmar, D., Brandon, M., Procaccio, V., and Wallace, D. C. (2004). Effects of purifying and adaptive selection on regional variation in human mtDNA. Science 303 (5655), 223–226. doi:10.1126/science.1088434
Sellam, J., and Berenbaum, F. (2010). The role of synovitis in pathophysiology and clinical symptoms of osteoarthritis. Nat. Rev. Rheumatol. 6 (11), 625–635. doi:10.1038/nrrheum.2010.159
Sharpley, M. S., Marciniak, C., Eckel-Mahan, K., McManus, M., Crimi, M., Waymire, K., et al. (2012). Heteroplasmy of mouse mtDNA is genetically unstable and results in altered behavior and cognition. Cell 151 (2), 333–343. doi:10.1016/j.cell.2012.09.004
Suliman, H. B., and Piantadosi, C. A. (2016). Mitochondrial quality control as a therapeutic target. Pharmacol. Rev. 68 (1), 20–48. doi:10.1124/pr.115.011502
Tian, Q., Moore, A. Z., Oppong, R., Ding, J., Zampino, M., Fishbein, K. W., et al. (2021). Mitochondrial DNA copy number and heteroplasmy load correlate with skeletal muscle oxidative capacity by P31 MR spectroscopy. Aging Cell 20 (11), e13487. doi:10.1111/acel.13487
Verbanck, M., Chen, C. Y., Neale, B., and Do, R. (2018). Detection of widespread horizontal pleiotropy in causal relationships inferred from Mendelian randomization between complex traits and diseases. Nat. Genet. 50 (5), 693–698. doi:10.1038/s41588-018-0099-7
Wallace, D. C. (2005). A mitochondrial paradigm of metabolic and degenerative diseases, aging, and cancer: A dawn for evolutionary medicine. Annu. Rev. Genet. 39, 359–407. doi:10.1146/annurev.genet.39.110304.095751
Wallace, D. C. (2016). Genetics: Mitochondrial DNA in evolution and disease. Nature 535 (7613), 498–500. doi:10.1038/nature18902
Ye, K., Lu, J., Ma, F., Keinan, A., and Gu, Z. (2014). Extensive pathogenicity of mitochondrial heteroplasmy in healthy human individuals. Proc. Natl. Acad. Sci. U. S. A. 111 (29), 10654–10659. doi:10.1073/pnas.1403521111
Keywords: mitochondrial heteroplasmy, mitochondrial abundance, osteoarthritis, mendelian randomization, knee OA (KOA)
Citation: Liu H, Cai B, Gong R, Yang Y, Wang J, Zhou D, Yu M and Li Y (2023) Impact of genetically predicted characterization of mitochondrial DNA quantity and quality on osteoarthritis. Front. Genet. 14:1130411. doi: 10.3389/fgene.2023.1130411
Received: 23 December 2022; Accepted: 13 February 2023;
Published: 24 February 2023.
Edited by:
Qiling Yuan, Xi’an Jiaotong University, ChinaReviewed by:
Francisco J. Blanco Garcia, University of A Coruña, SpainCopyright © 2023 Liu, Cai, Gong, Yang, Wang, Zhou, Yu and Li. This is an open-access article distributed under the terms of the Creative Commons Attribution License (CC BY). The use, distribution or reproduction in other forums is permitted, provided the original author(s) and the copyright owner(s) are credited and that the original publication in this journal is cited, in accordance with accepted academic practice. No use, distribution or reproduction is permitted which does not comply with these terms.
*Correspondence: Min Yu, bXljZGMxMjM0QDE2My5jb20=; Yingjun Li, MjAxNjAzNDAzNkBobWMuZWR1LmNu
Disclaimer: All claims expressed in this article are solely those of the authors and do not necessarily represent those of their affiliated organizations, or those of the publisher, the editors and the reviewers. Any product that may be evaluated in this article or claim that may be made by its manufacturer is not guaranteed or endorsed by the publisher.
Research integrity at Frontiers
Learn more about the work of our research integrity team to safeguard the quality of each article we publish.