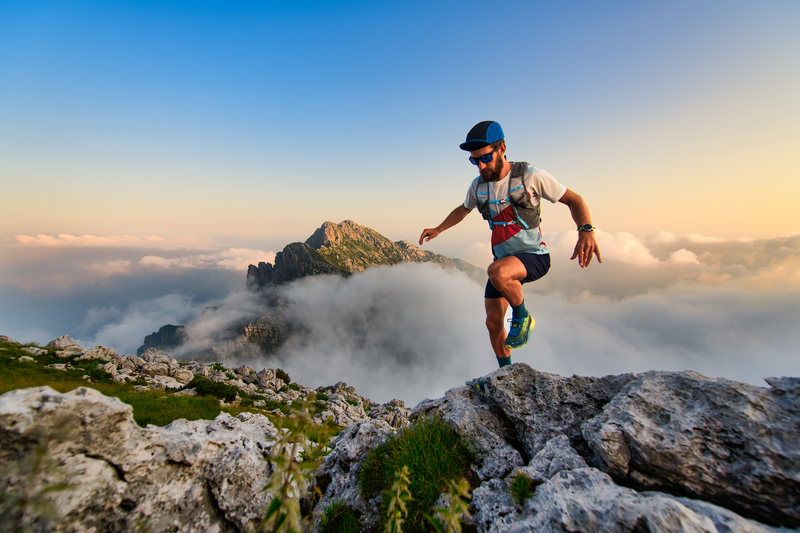
95% of researchers rate our articles as excellent or good
Learn more about the work of our research integrity team to safeguard the quality of each article we publish.
Find out more
ORIGINAL RESEARCH article
Front. Genet. , 20 February 2023
Sec. Statistical Genetics and Methodology
Volume 14 - 2023 | https://doi.org/10.3389/fgene.2023.1113804
Background: The association between immune imbalances and adverse pregnancy outcomes has been extensive investigated by observational studies, but remain unclear. Thus, this study aimed to establish the causality of the circulation levels of cytokines on adverse pregnancy outcomes, such as offspring’s birthweight (BW), preterm birth (PTB), spontaneous miscarriage (SM), and stillbirth (SB).
Methods: Two-sample Mendelian randomization (MR) analysis was employed to investigate potential causal relations between 41 cytokines and pregnancy outcomes on the basis of previously published GWAS datasets. Multivariable MR (MVMR) analysis was implemented to investigate the effect of the composition of cytokine networks on the pregnancy outcomes. Potential risk factors were further estimated to explore the potential mediators.
Results: Genetic correlation analysis based on large GWAS data sources revealed that genetically predicted MIP1b (β = −0.027, S.E. = 0.010, p = 0.009) and MCSF (β = −0.024, S.E. = 0.011, p = 0.029) were associated with reduced offspring’s BW, MCP1 (OR: 0.90, 95% CI: 0.83–0.97, p = 0.007) was associated with reduced SM risk, SCF (β = −0.014, S.E. = 0.005, p = 0.012) associated with decreased number of SB in MVMR. The univariable MR showed that GROa (OR: 0.92, 95% CI: 0.87–0.97, p = 0.004) was associated with decreased PTB risk. Except for the MCSF-BW association, all above associations surpassed the Bonferroni corrected threshold. The MVMR results revealed that MIF, SDF1a, MIP1b, MCSF and IP10 composed cytokine networks, associated with offspring’s BW. Risk factors analysis indicated that the above causal associations might be mediated by smoking behaviors.
Conclusion: These findings suggest the causal associations of several cytokines with adverse pregnancy outcomes, which were potentially mediated by smoking and obesity. Some of the results did not been corrected through multiple tests and larger samples verification is required in further studies.
Although the world population has increased to over eight billion, reproduction continues to be a relatively low-efficiency and low-flexibility process. The high incidence of implantation failure, spontaneous miscarriage (SM), and stillbirth (SB) shows that adverse pregnancy outcomes are prevalent, resulting in 30%–60% failure in achieving the survival of a clinical progeny (Quenby et al., 2021; Whittaker et al., 1983; Wilcox et al., 1988). Approximately 10.6% of pregnant women in their late pregnancy has preterm birth (PTB) (Chawanpaiboon et al., 2019). Many studies have indicated that children born prematurely had a great risk of neurodevelopmental disorders, chronic lung disease, and behavior problems, which pose a high risk of chronic disease in adulthood (Chawanpaiboon et al., 2019). PTB is a major cause of disease worldwide and challenges health systems and economies (Chawanpaiboon et al., 2019).
The pathogenesis of adverse pregnancy outcomes remains unclear, and effective therapeutic strategy for the prevention of these outcomes remained elusive. To better predict and improve pregnancy outcomes, identifying new sensitive, reliable biomarkers and developing new targeted treatments are crucial. Physiological processes during pregnancy and childbirth are tightly and special. In particular, uterine cavity creates a local environment to protect the embryo from rejection and regulate fetal growth and development. Birth labor is a unique physiological process, in which the terine immune environment shifts immune responses away from immune tolerance state to activation states, leading to myometrial activation (Green and Arck, 2020; Menon, 2022; Meuleman et al., 2015). Thus, the fine balance between fetal and maternal immunological tolerance plays an important role in maintaining normal pregnancy. In recent observational studies, adverse pregnancy outcomes may have been caused by an imbalance of maternal immune tolerance or by direct stimulation by premature or excessive inflammation (Green and Arck, 2020). Aberrant cytokines have been linked to risks of adverse perinatal outcomes, such as SM, PTB, and low birthweight (BW) (Bodnar et al., 2020; Brou et al., 2012; Carp, 2004; Makhseed et al., 2000; Polettini et al., 2017; Sharabi-Nov et al., 2020), however, few Mendelian randomization (MR) analysis have conducted on above causality so far. The results could have been affected by confounding factors, such as smoking, alcohol consumption, underlying disease, and complications, and a correlation was found between findings and many cytokines (Bhat et al., 2013; Brou et al., 2012; Polettini et al., 2017). Nevertheless, population-based studies did not provide robust evidence about the association between cytokines and adverse pregnancy outcomes or how cytokines may impact them.
Two-sample MR analysis is the best source of evidence because of the non-feasibility of randomized controlled trials. MR analysis can benefit from the genetic variants used as instrumental variables of modifiable exposures, eliminating the potential confounds of conventional observational studies. Therefore, we used publicly available large-scale GWAS data. We conducted an MR analysis to investigate 41 kinds of cytokines on adverse pregnancy outcomes (i.e., BW, PTB, SM, and SB). We focused on elucidating the potential causes and effects of adverse pregnancy outcomes to help improve perinatal management, enhance population quality, and provide a new reference for the formulation of maternal and infant health prevention strategies.
The genetic instruments of 41 plasma/serum cytokines were obtained from the IEU GWAS database (https://gwas.mrcieu.ac.uk/) (Ben et al., 2020), which was the most comprehensive and largest meta-analysis consisting of 8,293 individuals from two studies in Finland, the Cardiovascular Risk in Young Finns Study, and FINRISK survey (Ahola-Olli et al., 2017). For the pregnancy outcomes, we obtained summary statistics based on participants of European ancestry by using the offspring’s BW (n = 68,258) (Beaumont et al., 2018), the gestational age and PTB (n = 1,04,106), SM (n = 98,453) and SB (n = 78,879) from the IEU GWAS database (https://gwas.mrcieu.ac.uk/) (Ben et al., 2020). PTB and SM from the FinnGen study (https://www.finngen.fi/en). GWAS summary datasets for the number of SBs was obtained from the UK Biobank (http://www.nealelab.is/uk-biobank). Ethical review and informed consent were obtained from the original GWAS. The study design is shown in Figure 1.
FIGURE 1. Study design. Abbreviations: BW, birthweight; PTB, preterm birth; SM, spontaneous miscarriage; SB, stillbirth.
SNPs associated with the circulation levels of cytokines in the genome-wide significance threshold (p < 10−5) and independent (10,000 kb pairs apart, r2 < 0.001) were selected as instrumental variables (IVs). Approximated F-statistics (beta2/se2) were applied to evaluate the strength of each genetic instrument (Pierce et al., 2011) and remove the SNP with F-statistics <10 (Supplementary Tables S1, S2). Instruments were then clumped for linkage disequilibrium to help ensure that the SNPs were independent by selecting the SNP with the lowest p-value.
Inverse variance weighted (IVW) regression was used as the primary method to obtain the MR effect estimates. We also performed weighted median and MR-Egger regression and compared the results. Cytokines showing evidence derived from univariable MR analysis were considered for multivariable MR (MVMR) analysis, and then other potential cytokines were included for MVMR. Bonferroni correction of the evidential threshold (p < 0.05) based on the number of exposures in each analysis. To assess the robustness of the results, we conducted several sensitivity analyses. Cochran’s Q test was used to evaluate heterogeneity among SNPs. MR Egger regression intercept was applied to evaluate the horizontal pleiotropy. MR pleiotropy residual sum and outlier (MR-PRESSO) global test were also performed to assess the presence of pleiotropy and to identify and remove outliers.
To explore the potential mechanisms of association between cytokines and pregnancy outcomes, we further estimated the potential risk factors, such as body mass index (BMI), height, smoking, systolic blood pressure, and type 2 diabetes. Information from the data sources of each risk factor is summarized in the Supplementary Table S3. Cytokines showing evidence derived from univariable MR and MVMR analysis were taken as exposures, and the above potential confounders and risk factors were included as outcomes in the MR analysis. Inverse variance weighted (IVW) regression was used in the primary MR analysis. Then, cytokines derived from MVMR analysis and risk factors were included as exposures, and pregnancy outcome were included as outcomes for MVMR.
All analyses were performed using two-sample MR (Version 0.5.6) and MendelianRandomization (version 0.6.0) in R (version 4.0.5).
Univariable MR analysis showed per standard deviation (SD) increased in genetically predicted levels of MIP1b (β = −0.031, S.E. = 0.011, p = 0.005), SDF1a (β = −0.051, S.E. = 0.020, p = 0.009) and MCSF(β = −0.020, S.E. = 0.010, p = 0.046) were associated with reduced offspring’s BW, but each association did not surpass the Bonferroni-corrected p-value threshold (Figure 2; Supplementary Table S4).
FIGURE 2. Analytical results from the MR study of the associations between the circulation levels of cytokines and offspring’s BW, PTB, SM, SB. Abbreviations: BW, birthweight; PTB, preterm birth; SM, spontaneous miscarriage; SB, stillbirth; OR, odds ratio; 95% CI: 95% confidence interval. The red lines represented p < 0.05.
We included MIP1b, SDF1a, and MCSF in MVMR, the results showed that MIP1b (β = −0.027, S.E. = 0.010, p = 0.009) and MCSF (β = −0.024, S.E. = 0.011, p = 0.029) remained associated with offspring’s BW, although only the association of MIP1b surpassed the Bonferroni-corrected p-value threshold (p < 0.017). After taking into account MIP1b and MCSF, SDF1a (β = −0.031, S.E. = 0.021, p = 0.136) became non-significant (Figure 3; Supplementary Table S8). Then we try to included other potential cytokines for MVMR, MIF(β = −0.030, S.E. = 0.013, p = 0.023), SDF1a (β = −0.042, S.E. = 0.020, p = 0.039), MIP1b (β = −0.026, S.E. = 0.009, p = 0.007), MCSF(β = −0.024, S.E. = 0.011, p = 0.030) and IP10 (β = 0.047, S.E. = 0.012, p = 0.000) composed cytokine networks, which significantly associated with offspring’s BW, but only MIP1b and IP10 surpassed the Bonferroni-corrected p-value threshold (p < 0.008) (Supplementary Table S10).
FIGURE 3. MVMR analytical results of the associations between the circulation levels of cytokines and offspring’s BW, SB. Abbreviations: BW, birthweight; SB, stillbirth; 95% CI: 95% confidence interval. The blue line represented p < 0.05, the red lines represented surpassed the Bonferroni-corrected p-value threshold.
The results from univariable MR showed that each SD raised in genetically predicted levels of GROa (OR: 0.92, 95% CI: 0.87–0.97, p = 0.004) decreased PTB risk, but the association did not surpass the Bonferroni-corrected p-value threshold (p < 0.003) (Figure 2; Supplementary Table S5).
Then, we try to included other potential cytokines for MVMR. After taking into account GROa, increased Eotaxin (OR: 0.92, 95% CI: 0.86–0.99, p = 0.035) levels was associated with attenuated risk for PTB, but GROa (OR: 1.02, 95% CI: 0.93–1.12, p = 0.634) did not remain significant associated with PTB risk (Supplementary Table S11). The association of Eotaxin did not surpass the Bonferroni-corrected p-value threshold (p < 0.025).
In univariable MR analysis, each SD increased in genetically predicted levels of MCP1 (OR: 0.93, 95% CI: 0.87–1.00, p = 0.037) was associated with decressed SM risk. However, raised MCP3 (OR: 1.05, 95% CI: 1.00–1.11, p = 0.038), IL-17 (OR: 1.11, 95% CI: 1.01–1.21, p = 0.026) and IL-1b (OR: 1.17, 95% CI: 1.03–1.33, p = 0.014) were associated with increased SM risk, but each association did not surpass the Bonferroni-corrected p-value threshold (Figure 2; Supplementary Table S6).
We included MCP1, MCP3, IL-17, and IL-1b in MVMR. After taking into account MCP3, IL-17 and IL-1b, raised MCP1 (OR: 0.90, 95% CI: 0.83–0.97, p = 0.007) remained associated with decreased risk of SM, and the association surpassed the Bonferroni-corrected p-value threshold (p < 0.013), but MCP3 (OR: 1.03, 95% CI: 0.99–1.07, p = 0.197), IL-17 (OR: 1.10, 95% CI: 0.97–1.24, p = 0.161) and IL-1b (OR: 1.05, 95% CI: 0.93–1.20, p = 0.433) did not remain significant associated with SM risk (Figure 4; Supplementary Table S9). Then, we try to included other potential cytokines for MVMR, after taking into account MCP1, MCP3, IL-17, and IL-1b, raised IL-8 (OR: 1.18, 95% CI: 1.07–1.31, p = 0.002) and RANTES (OR: 1.10, 95% CI: 1.02–1.18, p = 0.017) were significantly associated with increased SM risk, but only IL-8 surpassed the Bonferroni-corrected p-value threshold (p < 0.008) (Supplementary Table S11).
FIGURE 4. MVMR analytical results of the associations between the circulation levels of cytokines and SM. Abbreviations: SM, spontaneous miscarriage; 95% CI: 95% confidence interval. The red lines represented surpassed the Bonferroni-corrected p-value threshold.
Univariable MR showed that each SD increase in genetically predicted levels of SCF (β = −0.014, S.E. = 0.005, p = 0.004), CTACK (β = −0.008, S.E. = 0.003, p = 0.025) and SDF1 (β = −0.014, S.E. = 0.005, p = 0.012) were associated with decreased number of SB, but each association did not surpass the Bonferroni-corrected p-value threshold (Figure 2; Supplementary Table S7).
Then, MVMR was performed to analyze whether CTACK, SCF, and SDF1a were associated with the risk of SB. After taking into account CTACK and SDF1a, raised SCF (β = −0.014, S.E. = 0.005, p = 0.012) remained associated with decreased number of SB, and the association surpassed the Bonferroni-corrected p-value threshold (p < 0.017), but CTACK (β = −0.006, S.E. = 0.003, p = 0.062) and SDF1a (β = −0.010, S.E. = 0.006, p = 0.104) did not remain significant associated with number of SB (Figure 3; Supplementary Table S8). Then, we try to included other potential cytokines for MVMR, no significant association was observed for other cytokines.
The MR-Egger regression intercept test showed that no evidence of horizontal pleiotropy (all p > 0.05) (Supplementary Table S12). MR-PRESSO indicated that horizontal pleiotropy may affected analyses of Eotaxin, MIF with BW; bNGF with PTB; and GCSF and MIG with SB (all p ≤ 0.05). Following outlier correction, there was no evidence for the association above (all p > 0.05) (Supplementary Table S13).
In addition, in-depth analyses showed a significant disparity in the heterogeneity between cytokines and the following pregnancy outcomes: PTB and CTACK, Eotaxin; SM and IL1ra; SB and MIG, MIP1b, RANTES. None of the other cytokines showed significant heterogeneity with adverse pregnancy outcomes (all p > 0.05) (Supplementary Table S12).
The potential mediators between cytokines and adverse pregnancy outcomes were further explored. Univariable MR showed that each SD increase in genetically predicted levels of SDF1a (β = 0.006, S.E. = 0.003, p = 0.025), MCP3 (β = 0.003, S.E. = 0.001, p = 0.039) associated with increased smoking; raised GROa (β = −0.006, S.E. = 0.003, p = 0.047), IL-17 (β = −0.013, S.E. = 0.006, p = 0.044) associated with decreased BMI; IL-1b (β = 0.016, S.E. = 0.005, p = 0.003), RANTES (β = −0.007, S.E. = 0.003, p = 0.029) associated with height, but only IL-1b associated with height surpassed the Bonferroni-corrected p-value threshold (p < 0.007) (Supplementary Table S14).
The MVMR results suggested that smoking behaviors might be responsible for MIF (β = −0.029, S.E. = 0.013, p = 0.035), MIP1b (β = −0.023, S.E. = 0.010, p = 0.028), MCSF(β = −0.031, S.E. = 0.011, p = 0.007), IP10 (β = 0.044, S.E. = 0.013, p = 0.001) linked offspring’s BW, but only MCSF and IP10 association surpassed the Bonferroni-corrected p-value threshold (p < 0.008). Smoking behaviors also might be potential mediators linking for GROa (OR: 0.91, 95% CI: 0.85–0.98, p = 0.010) linked risk of PTB, MCP1 (OR: 0.89, 95% CI: 0.82–0.96, p = 0.003) and IL-8 (OR: 1.19, 95% CI: 1.07–1.33, p = 0.003) linked SM risk, and SCF (β = −0.012, S.E. = 0.005, p = 0.031) linked number of SB, all above associations surpassed the Bonferroni corrected threshold. The SDF1a-BW (β = 0.053, S.E. = 0.016, p = 0.001) association enhanced after taking into account body mass index in MVMR, and surpassed the Bonferroni corrected threshold (Supplementary Table S15).
MR analysis was conducted to investigate whether cytokines were causally associated with BW, PTB, SM, and SB and to identify causative relations between cytokine and pregnancy outcomes. The circulation levels of MIP1b, MCSF, GROa, MCP1, SCF and involved complex cytokines network were associated with the risk of adverse pregnancy outcomes, which might be potentially mediated by smoking behaviors and BMI.
During pregnancy, the maternal immune system is reprogrammed to tolerate allogeneic (paternal) fetal antigens, in which a stepwise progression of tolerance is clearly observed. As a result of creating immune imbalances, pregnancy outcomes are jeopardized (Green and Arck, 2020; Menon, 2022). Given that cytokines influence the pregnancy outcomes, this result could have significant clinical implications (Dai et al., 2022). First, cytokine is not only useful as a tool for identifying a high-risk pregnancy but also for stratifying risk groups. Second, immunotherapy may provide for adverse pregnancy outcomes with a novel treatment option.
Numerous observational cohort studies had linked the levels of the biomarkers MIP1b, MCSF, GROa, Eotaxin, MCP3, and SCF in the regulation of embryo implantation (Gnainsky et al., 2010), improve endometrial receptivity (Gnainsky et al., 2015), premature rupture of membranes (Raba et al., 2021), recurrent miscarriage (Wu et al., 2021), reduced developmental potential of an oocyte (Tan et al., 2017), and gestational hypertension (Lan et al., 2022). IP10 has been found to promote the differentiation and migration of T cells toward Th1 and Th17 cells. This phenomenon contributes to the construction of the local proinflammatory environment of endometrial tissue and facilitates tissue remodeling and angiogenesis. Such processes can enhance the implantation of embryos based on the association with the endometrial microenvironment during the first trimester (Gervasi et al., 2012; Jia and Li, 2020; Jiang et al., 2021). MCP-1, RANTES might be predictive factors for diabetic pregnancy in early pregnancy development, which levels associated with perinatal outcome (Wender-Ozegowska et al., 2008). These findings were strongly supported by our results.
Maternal immune imbalances might be associated with a range of negative experiences, such as infection, smoking (Morales-Prieto et al., 2022; Prins et al., 2012), exposure to air pollutants (Buxton et al., 2019), symptoms of anxiety, depression, and other mood disorders (Camacho-Arroyo et al., 2021), and promotion of inflammation directly or indirectly by the reproductive tract (Kumar et al., 2021) or gut microbiota dysbiosis (Chen et al., 2019), that contribute to pregnancy outcomes. During pregnancy, changes to a woman’s physiologic state of lungs are reasons for the mother’s increased susceptibility to infectious agents. A rapid cellular and humoral immune response is evoked during infection, increasing the possibility of suffering from adverse pregnancy outcomes. Patients infected with influenza (Uchide et al., 2012), COVID-19 (Rosen et al., 2022), Zika virus (Rabelo et al., 2020), dengue virus (Nunes et al., 2019), Chlamydia trachomatis of the reproductive tract (Faris et al., 2019), HIV (Akoto et al., 2021; Morrison et al., 2018), Plasmodium falciparum (Chêne et al., 2014; Dobaño et al., 2018), and latent tuberculosis (Indrati et al., 2022) had elevated levels of MIP1b, GROa, IL-8, RANTES, and IP10, but reduced levels of Eotaxin, MCP-1 (Requena et al., 2015), associated with the inflammation in the placenta. The serum cytokine expression levels, which were associated with the type of infection, could provide predictive information about pathogen infection, mother-to-child-vertical transmission (Kumar et al., 2012; Schnittman et al., 2021), immunity protection of host (Amaral et al., 2020), and the risk of adverse pregnancy outcomes.
Maternal smoking affects functional development of placenta, and constrain the delivery of nutrients and oxygen. However, tobacco induced maternal-fetal immunoregulatory changes during pregnancy have not been studied deeply (Morales-Prieto et al., 2022). The current findings may suggest new insights into the relationship between smoking behaviors, cytokines network, and adverse pregnancy outcomes.
In addition, given that adverse pregnancy outcomes had multiple causes, secreted cytokines apparently induce a more complex network rather than act independently with maternal response. Therefore, the complex network played a role in influencing the fetal development and function. Multiple biomarkers might have a better prognostic/predictive relevance. Bodnar et al. (2020) described a unique neurodevelopmental delay atlas, suggesting that cytokine imbalance may have their origin in the neurodevelopmental disorders of a child. The effectiveness of a comprehensive risk biomarker for predicting preterm deliveries could be enhanced than a single risk factor. In addition, an apparent discrepancy among different races had been proposed (Bhat et al., 2013; Brou et al., 2012). We estimated the effects of the cytokine network on pregnancy outcomes using multivariable MR, which have not been studied deeply, and further evidence is still needed to confirm it.
Although robust study protocols and strict implementation had been performed, this study still presents some limitations. First, larger sample sizes are required for GWAS discovery to generalize conclusions. Second, genetic variants that represented a cumulative lifetime exposure were incorporated into the MR, and a larger cohort study is necessary to confirm our findings. Finally, the dissimilar findings in MR may have been caused by separate regions and differences in genetics and environment among the diverse ethnic groups.
In conclusion, disturbances in cytokine homeostasis, immune imbalances, and adverse pregnancy outcomes correlations of causality could be estimated from exposure and outcome data with the aid of more comprehensive GWAS datasets and two-sample MR study. An evaluation of the effects of cytokine networks on pregnancy outcomes was initiated in this study by using MVMR. Inflammation cells were activated abnormally and collaborated subtly, resulting in adverse perinatal outcomes. The significant clinical implications for studying the molecular mechanisms of immune imbalance-mediated adverse pregnancy outcomes and identifying high-risk maternal individuals and immunotherapy were discussed. The results could present various implications for policies and programs at the prepotency level. Independent validation studies and prospective cohort studies will be necessary to verify the conclusion.
The original contributions presented in the study are included in the article/Supplementary Material, further inquiries can be directed to the corresponding author.
ZM, HW, and YJ contributed to the conception and design of the study. HW and TJ collected the data, and HW and JHJ performed the statistical analysis. YW, ML, SH, JJX, ZC, YG, and JZ conducted literature retrieval, while HW and JHJ wrote the first draft of the manuscript. All authors contributed to the article and approved the submitted version.
This work was supported by the Natural Science Foundation of China (82060145, 81901538), Guangxi Key Research and Development Project (Grant No. Guike AB21196022), Guangxi Science and Technology Major Project (Grant No. Guike AA22412, Guike AA22398), the Natural Science Foundation of Guangxi Zhuang Autonomous Region (2021JJA140912, 2018JJB140051), Major project of Scientific Research and Technology Development Plan of Nanning (20221023), Advanced Innovation Teams and Xinghu Scholars Program of Guangxi Medical University, and Science and Technology Plan of Liuzhou (2019BJ10603).
We wish to acknowledge the participants and investigators of the UK Biobank and the FinnGen study. We also appreciate Ahola-Olli et al. and Beaumont RN et al. for making their GWAS summary-level statistics publicly available.
The authors declare that the research was conducted in the absence of any commercial or financial relationships that could be construed as a potential conflict of interest.
All claims expressed in this article are solely those of the authors and do not necessarily represent those of their affiliated organizations, or those of the publisher, the editors and the reviewers. Any product that may be evaluated in this article, or claim that may be made by its manufacturer, is not guaranteed or endorsed by the publisher.
The Supplementary Material for this article can be found online at: https://www.frontiersin.org/articles/10.3389/fgene.2023.1113804/full#supplementary-material
Ahola-Olli, A. V., Würtz, P., Havulinna, A. S., Aalto, K., Pitkänen, N., Lehtimäki, T., et al. (2017). Genome-wide association study identifies 27 loci influencing concentrations of circulating cytokines and growth factors. Am. J. Hum. Genet. 100 (1), 40–50. doi:10.1016/j.ajhg.2016.11.007
Akoto, C., Norris, S. A., and Hemelaar, J. (2021). Maternal HIV infection is associated with distinct systemic cytokine profiles throughout pregnancy in South African women. Sci. Rep.-UK 11 (1), 10079. doi:10.1038/s41598-021-89551-3
Amaral, M. S., Goulart, E., Caires-Júnior, L. C., Morales-Vicente, D. A., Soares-Schanoski, A., Gomes, R. P., et al. (2020). Differential gene expression elicited by ZIKV infection in trophoblasts from congenital Zika syndrome discordant twins. PLoS Negl. Trop. D. 14 (8), e0008424. doi:10.1371/journal.pntd.0008424
Beaumont, R. N., Warrington, N. M., Cavadino, A., Tyrrell, J., Nodzenski, M., Horikoshi, M., et al. (2018). Genome-wide association study of offspring birth weight in 86 577 women identifies five novel loci and highlights maternal genetic effects that are independent of fetal genetics. Hum. Mol. Genet. 27 (4), 742–756. doi:10.1093/hmg/ddx429
Ben, E., Matthew, L., Tessa, A., Yi, L., Peter, M., Jon, H., et al. (2020). The MRC IEU OpenGWAS data infrastructure. bioRxiv Available at: https://www.biorxiv.org/content/10.1101/2020.08.10.244293v1.
Bhat, G., Spratt, H., Tamayo, E., Saade, G., and Menon, R. (2013). 487: Multivariate adaptive regression splines analysis to predict biomarkers of spontaneous preterm birth. Am. J. Obstet. Gynecol. 208 (1), S210. doi:10.1016/j.ajog.2012.10.653
Bodnar, T. S., Raineki, C., Wertelecki, W., Yevtushok, L., Plotka, L., Granovska, I., et al. (2020). Immune network dysregulation associated with child neurodevelopmental delay: Modulatory role of prenatal alcohol exposure. J. Neuroinflamm. 17 (1), 39. doi:10.1186/s12974-020-1717-8
Brou, L., Almli, L. M., Pearce, B. D., Bhat, G., Drobek, C. O., Fortunato, S., et al. (2012). Dysregulated biomarkers induce distinct pathways in preterm birth. BJOG Int. J. Obstetrics Gynaecol. 119 (4), 458–473. doi:10.1111/j.1471-0528.2011.03266.x
Buxton, M. A., Meraz-Cruz, N., Sanchez, B. N., Gronlund, C. J., Foxman, B., Vadillo-Ortega, F., et al. (2019). Air pollution and inflammation: Findings from concurrent repeated measures of systemic and reproductive tract cytokines during term pregnancy in Mexico City. Sci. Total Environ. 681, 235–241. doi:10.1016/j.scitotenv.2019.05.041
Camacho-Arroyo, I., Flores-Ramos, M., Mancilla-Herrera, I., Cruz, F. M. C., Hernández-Ruiz, J., Diaz, G. P., et al. (2021). Chemokine profile in women with moderate to severe anxiety and depression during pregnancy. BMC Pregnancy Childb 21 (1), 807. doi:10.1186/s12884-021-04225-2
Care, A., Nevitt, S. J., Medley, N., Donegan, S., Good, L., Hampson, L., et al. (2022). Interventions to prevent spontaneous preterm birth in women with singleton pregnancy who are at high risk: Systematic review and network meta-analysis. BMJ 376, e064547. doi:10.1136/bmj-2021-064547
Carp, H. (2004). Cytokines in recurrent miscarriage. Lupus 13 (9), 630–634. doi:10.1191/09612033043lu1091oa
Chawanpaiboon, S., Vogel, J. P., Moller, A., Lumbiganon, P., Petzold, M., Hogan, D., et al. (2019). Global, regional, and national estimates of levels of preterm birth in 2014: A systematic review and modelling analysis. Lancet Glob. Health 7 (1), e37–e46. doi:10.1016/S2214-109X(18)30451-0
Chen, Y., Li, Z., Tye, K. D., Luo, H., Tang, X., Liao, Y., et al. (2019). Probiotic supplementation during human pregnancy affects the gut microbiota and immune status. Front. Cell. Infect. Mi. 9, 254. doi:10.3389/fcimb.2019.00254
Chêne, A., Briand, V., Ibitokou, S., Dechavanne, S., Massougbodji, A., Deloron, P., et al. (2014). Placental cytokine and chemokine profiles reflect pregnancy outcomes in women exposed to plasmodium falciparum infection. Infect. Immun. 82 (9), 3783–3789. doi:10.1128/IAI.01922-14
Colvin, M., McGuire, W., and Fowlie, P. W. (2004). ABC of preterm birth: Neurodevelopmental outcomes after preterm birth. BMJ. Br. Med. J. Int. ed.). 329 (7479), 1390–1393. doi:10.1136/bmj.329.7479.1390
Crump, C., Sundquist, J., Winkleby, M. A., and Sundquist, K. (2019). Preterm birth and risk of chronic kidney disease from childhood into mid-adulthood: National cohort study. BMJ 365, l1346. doi:10.1136/bmj.l1346
Dai, F., Hu, M., Zhang, Y., Zhu, R., Chen, L., Li, Z., et al. (2022). TNF-α/anti-TNF-α drugs and its effect on pregnancy outcomes. Expert Rev. Mol. Med. 24, e26. doi:10.1017/erm.2022.18
Deshmukh, H., and Way, S. S. (2019). Immunological basis for recurrent fetal loss and pregnancy complications. Annu. Rev. pathology 14 (1), 185–210. doi:10.1146/annurev-pathmechdis-012418-012743
Dobaño, C., Berthoud, T., Manaca, M. N., Nhabomba, A., Guinovart, C., Aguilar, R., et al. (2018). High production of pro-inflammatory cytokines by maternal blood mononuclear cells is associated with reduced maternal malaria but increased cord blood infection. Malar. J. 17 (1), 177. doi:10.1186/s12936-018-2317-2
Faris, R., Andersen, S. E., McCullough, A., Gourronc, F., Klingelhutz, A. J., and Weber, M. M. (2019). Chlamydia trachomatis serovars drive differential production of proinflammatory cytokines and chemokines depending on the type of cell infected. Front. Cell. Infect. Mi. 9, 399. doi:10.3389/fcimb.2019.00399
Gervasi, M., Romero, R., Bracalente, G., Erez, O., Dong, Z., Hassan, S. S., et al. (2012). Midtrimester amniotic fluid concentrations of interleukin-6 and interferon-gamma-inducible protein-10: Evidence for heterogeneity of intra-amniotic inflammation and associations with spontaneous early (<32 weeks) and late (>32 weeks) preterm delivery. J. Perinat. Med. 40 (4), 329–343. doi:10.1515/jpm-2012-0034
Gnainsky, Y., Granot, I., Aldo, P., Barash, A., Or, Y., Mor, G., et al. (2015). Biopsy-induced inflammatory conditions improve endometrial receptivity: The mechanism of action. Reprod. Camb. Engl. 149 (1), 75–85. doi:10.1530/REP-14-0395
Gnainsky, Y., Granot, I., Aldo, P. B., Barash, A., Or, Y., Schechtman, E., et al. (2010). Local injury of the endometrium induces an inflammatory response that promotes successful implantation. Fertil. Steril. 94 (6), 2030–2036. doi:10.1016/j.fertnstert.2010.02.022
Green, E. S., and Arck, P. C. (2020). Pathogenesis of preterm birth: Bidirectional inflammation in mother and fetus. Semin. Immunopathol. 42 (4), 413–429. doi:10.1007/s00281-020-00807-y
Indrati, A. R., Sumarpo, A., Atmadja, P., Wisesa, R. R., Ghozali, M., Judistiani, R. T. D., et al. (2022). Exploring alternative cytokines as potential biomarkers for latent tuberculosis infection in pregnant women. PLoS One 17 (7), e0270552. doi:10.1371/journal.pone.0270552
Jia, N., and Li, J. (2020). Human uterine decidual NK cells in women with a history of early pregnancy enhance angiogenesis and trophoblast invasion. Biomed. Res. Int. 2020, 6247526–6247527. doi:10.1155/2020/6247526
Jiang, Y., Huang, F., Chai, X., Yuan, W., Ding, H., and Wu, X. (2021). The role of IP-10 and its receptor CXCR3 in early pregnancy. Mol. Immunol. 140, 59–69. doi:10.1016/j.molimm.2021.09.013
Kumar, M., Murugesan, S., Singh, P., Saadaoui, M., Elhag, D. A., Terranegra, A., et al. (2021). Vaginal microbiota and cytokine levels predict preterm delivery in Asian women. Front. Cell. Infect. Mi. 11, 639665. doi:10.3389/fcimb.2021.639665
Kumar, S. B., Rice, C. E., Milner, D. A., Ramirez, N. C., Ackerman, W. E., Mwapasa, V., et al. (2012). Elevated cytokine and chemokine levels in the placenta are associated with in-utero HIV-1 mother-to-child transmission. AIDS 26 (6), 685–694. doi:10.1097/QAD.0b013e3283519b00
Lan, X., Guo, L., Zhu, S., Cao, Y., Niu, Y., Han, S., et al. (2022). First-trimester serum cytokine profile in pregnancies conceived after assisted reproductive Technology (ART) with subsequent pregnancy-induced hypertension. Front. Immunol. 13, 930582. doi:10.3389/fimmu.2022.930582
Makhseed, M., Raghupathy, R., Azizieh, F., Farhat, R., Hassan, N., and Bandar, A. (2000). Circulating cytokines and CD30 in normal human pregnancy and recurrent spontaneous abortions. Hum. Reprod. 15 (9), 2011–2017. doi:10.1093/humrep/15.9.2011
Menon, R. (2022). Fetal inflammatory response at the fetomaternal interface: A requirement for labor at term and preterm. Immunol. Rev. 308 (1), 149–167. doi:10.1111/imr.13075
Meuleman, T., Lashley, L. E. L. O., Dekkers, O. M., van Lith, J. M. M., Claas, F. H. J., and Bloemenkamp, K. W. M. (2015). HLA associations and hla sharing in recurrent miscarriage: A systematic review and meta-analysis. Hum. Immunol. 76 (5), 362–373. doi:10.1016/j.humimm.2015.02.004
Morales-Prieto, D. M., Fuentes-Zacarías, P., Murrieta-Coxca, J. M., Gutierrez-Samudio, R. N., Favaro, R. R., Fitzgerald, J. S., et al. (2022). Smoking for two-effects of tobacco consumption on placenta. Mol. Asp. Med. 87, 101023. doi:10.1016/j.mam.2021.101023
Morrison, C. S., Fichorova, R., Chen, P., Kwok, C., Deese, J., Yamamoto, H., et al. (2018). A longitudinal assessment of cervical inflammation and immunity associated with HIV-1 infection, hormonal contraception, and pregnancy. AIDS Res. Hum. Retrov. 34 (10), 889–899. doi:10.1089/aid.2018.0022
Nunes, P., Nogueira, R., Coelho, J., Rodrigues, F., Salomão, N., José, C., et al. (2019). A stillborn multiple organs’ investigation from a maternal DENV-4 infection: Histopathological and inflammatory mediators characterization. Viruses 11 (4), 319. doi:10.3390/v11040319
Pierce, B. L., Ahsan, H., and VanderWeele, T. J. (2011). Power and instrument strength requirements for Mendelian randomization studies using multiple genetic variants. Int. J. Epidemiol. 40 (3), 740–752. doi:10.1093/ije/dyq151
Polettini, J., Cobo, T., Kacerovsky, M., Vinturache, A. E., Laudanski, P., Peelen, M. J. C. S., et al. (2017). Biomarkers of spontaneous preterm birth: A systematic review of studies using multiplex analysis. J. Perinat. Med. 45 (1), 71–84. doi:10.1515/jpm-2016-0097
Prins, J. R. M., Hylkema, M. N. P., Erwich, J. J. H. M., Huitema, S., Dekkema, G. J., Dijkstra, F. E., et al. (2012). Smoking during pregnancy influences the maternal immune response in mice and humans. Am. J. Obstet. Gynecol. 207 (1), 76.e1–76.14. doi:10.1016/j.ajog.2012.04.017
Quenby, S., Gallos, I. D., Dhillon-Smith, R. K., Podesek, M., Stephenson, M. D., Fisher, J., et al. (2021). Miscarriage matters: The epidemiological, physical, psychological, and economic costs of early pregnancy loss. Lancet 397 (10285), 1658–1667. doi:10.1016/S0140-6736(21)00682-6
Raba, G., Kacerovsky, M., and Laudanski, P. (2021). Eotaxin-2 as a potential marker of preterm premature rupture of membranes: A prospective, cohort, multicenter study. Adv. Clin. Exp. Med. 30 (2), 197–202. doi:10.17219/acem/130609
Rabelo, K., de Souza, L. J., Salomão, N. G., Machado, L. N., Pereira, P. G., Portari, E. A., et al. (2020). Zika induces human placental damage and inflammation. Front. Immunol. 11, 2146. doi:10.3389/fimmu.2020.02146
Requena, P., Barrios, D., Robinson, L. J., Samol, P., Umbers, A. J., Wangnapi, R., et al. (2015). Proinflammatory responses and higher IL-10 production by T cells correlate with protection against malaria during pregnancy and delivery outcomes. J. Immunol. 194 (7), 3275–3285. doi:10.4049/jimmunol.1401038
Rosen, D. B., Murphy, E. A., Gejman, R. S., Capili, A., Friedlander, R. L., Rand, S., et al. (2022). Cytokine response over the course of COVID-19 infection in pregnant women. Cytokine 154, 155894. doi:10.1016/j.cyto.2022.155894
Schnittman, S. R., Byakwaga, H., Boum, Y., Kabakyenga, J., Matthews, L. T., Burdo, T. H., et al. (2021). Changes in immune activation during pregnancy and the postpartum period in treated HIV infection. Open Forum Infect. Dis. 8 (6), ofab245. doi:10.1093/ofid/ofab245
Sharabi-Nov, A., Kumar, K., Fabjan Vodušek, V., Premru Sršen, T., Tul, N., Fabjan, T., et al. (2020). Establishing a differential marker profile for pregnancy complications near delivery. Fetal diagn. Ther. 47 (6), 471–484. doi:10.1159/000502177
Tan, J., Zou, Y., Wu, X., Tian, L., Su, Q., He, J., et al. (2017). Increased SCF in follicular fluid and granulosa cells positively correlates with oocyte maturation, fertilization, and embryo quality in humans. Reprod. Sci. 24 (11), 1544–1550. doi:10.1177/1933719117697125
The Lancet Respiratory Medicine, (2022). Improving lifelong respiratory health after preterm birth. Lancet Respir. Med. 10 (2), 121. doi:10.1016/S2213-2600(22)00018-2
Uchide, N., Ohyama, K., Bessho, T., Takeichi, M., and Toyoda, H. (2012). Possible roles of proinflammatory and chemoattractive cytokines produced by human fetal membrane cells in the pathology of adverse pregnancy outcomes associated with influenza virus infection. Mediat. Inflamm. 2012, 270670. doi:10.1155/2012/270670
Wender-Ozegowska, E., Micha Owska-Wender, G., Zawiejska, A., Pietryga, M., Brazert, J., and Wender, M. (2008). Concentration of chemokines in peripheral blood in first trimester of diabetic pregnancy. Acta Obstet. gyn. scan. 87 (1), 14–19. doi:10.1080/00016340701698724
Whittaker, P. G., Taylor, A., and Lind, T. (1983). Unsuspected pregnancy loss in healthy women. Lancet (British Ed. 1 (8334), 1126–1127. doi:10.1016/s0140-6736(83)92865-9
Wilcox, A. J., Weinberg, C. R., O'Connor, J. F., Baird, D. D., Schlatterer, J. P., Canfield, R. E., et al. (1988). Incidence of early loss of pregnancy. N. Engl. J. Med. 319 (4), 189–194. doi:10.1056/NEJM198807283190401
Keywords: Mendelian randomization, cytokines, immune system, birthweight, spontaneous miscarriages, stillbirth, preterm birth
Citation: Wang H, Jiang J, Jin T, Wang Y, Li M, Huang S, Xie J, Chen Z, Guo Y, Zheng J, Jiang Y and Mo Z (2023) Associations of circulation levels of cytokines with birthweight, preterm birth, spontaneous miscarriages, and stillbirth: A Mendelian randomization analysis. Front. Genet. 14:1113804. doi: 10.3389/fgene.2023.1113804
Received: 01 December 2022; Accepted: 08 February 2023;
Published: 20 February 2023.
Edited by:
Qingzhao Yu, Louisiana State University, United StatesReviewed by:
Wentao Cao, Louisiana State University, United StatesCopyright © 2023 Wang, Jiang, Jin, Wang, Li, Huang, Xie, Chen, Guo, Zheng, Jiang and Mo. This is an open-access article distributed under the terms of the Creative Commons Attribution License (CC BY). The use, distribution or reproduction in other forums is permitted, provided the original author(s) and the copyright owner(s) are credited and that the original publication in this journal is cited, in accordance with accepted academic practice. No use, distribution or reproduction is permitted which does not comply with these terms.
*Correspondence: Zengnan Mo, bW96ZW5nbmFuQGd4bXUuZWR1LmNu
†These authors have contributed equally to this work
Disclaimer: All claims expressed in this article are solely those of the authors and do not necessarily represent those of their affiliated organizations, or those of the publisher, the editors and the reviewers. Any product that may be evaluated in this article or claim that may be made by its manufacturer is not guaranteed or endorsed by the publisher.
Research integrity at Frontiers
Learn more about the work of our research integrity team to safeguard the quality of each article we publish.