- 1Department of Colorectal Surgery, The Sixth Affiliated Hospital, Sun Yat-sen University, Guangzhou, China
- 2Guangdong Institute of Gastroenterology, Guangzhou, China
- 3Guangdong Provincial Key Laboratory of Colorectal and Pelvic Floor Diseases, The Sixth Affiliated Hospital, Sun Yat-sen University, Guangzhou, China
- 4Department of Gastrointestinal Endoscopy, The Sixth Affiliated Hospital, Sun Yat-sen University, Guangzhou, China
- 5Department of Pathology, The First Affiliated Hospital of Anhui Medical University, Hefei, China
Long non-coding RNAs (lncRNAs) remodel the tumor immune microenvironment (TIME) by regulating the functions of tumor-infiltrating immune cells. It remains uncertain the way that TIME-related lncRNAs (TRLs) influence the prognosis and immunotherapy response of colorectal cancer (CRC). Aiming at providing survival and immunotherapy response predictions, a CRC TIME-related lncRNA signature (TRLs signature) was developed and the related potential regulatory mechanisms were explored with a comprehensive analysis on gene expression profiles from 97 immune cell lines, 61 CRC cell lines and 1807 CRC patients. Stratifying CRC patients with the TRLs signature, prolonged survival was observed in the low-risk group, while the patients in the high-risk group had significantly higher pro-tumor immune cells infiltration and higher immunotherapy response rate. Through the complex TRLs-mRNA regulation network, immunoregulation pathways and immunotherapy response pathways were found to be differently activated between the groups. In conclusion, the CRC TRLs signature is capable of making prognosis and immunotherapy response predictions, which may find application in stratifying patients for immunotherapy in the bedside.
Introduction
Colorectal cancer (CRC) ranks as the third most common cancers and the second most common cause of cancer-related deaths worldwide (Siegel et al., 2019), and the overall 5-years relative survival rate for CRC patients is approximately 64% (Miller et al., 2019). Though significant advances have been made in the treatment of CRC, the recurrence rate remains high in patients received standard chemotherapy and surgery (Ahiko et al., 2021; Fang et al., 2021; Kim et al., 2021). Recently, immunotherapy has emerged as a novel treatment approach and achieved exciting results in some cancer types (Doki et al., 2022; Makker et al., 2022; Schmid et al., 2022). For CRC, anti-programmed death 1 (anti-PD-1) antibodies, such as pembrolizumab and nivolumab, and CTLA-4 inhibitor ipilimumab were approved by FDA (Pan et al., 2021). Though a subset of patients with mismatch repair deficiency or high microsatellite instability CRC benefit a lot from immune checkpoint blockade therapy (Pan et al., 2021), the overall response rate of immunotherapy remains low in all cases of CRC and there were difficulties in stratifying suitable patients for immunotherapy (Chen et al., 2021). Widely used biomarkers for immunotherapy response prediction, such as impaired DNA mismatch repair deficiency and microsatellite instability (MSI) (Cortes-Ciriano et al., 2017), only have moderate accuracy, and there are still a portion of CRC patients with MSI/mismatch repair deficiency tumors do not respond to the treatment (Gibney et al., 2016; Cohen et al., 2020). Therefore, it is of vital importance to develop effective methods to predict CRC prognosis and immunotherapy response.
In recent years, tumor microenvironment (TME) was identified to have an huge impact on the behavior and characteristics of cancer (Li et al., 2007). TME is made up of noncellular components, such as extracellular matrix and types of signaling molecules, and non-tumor cellular components, including epithelial, smooth muscle, immune cells and other types of cells in the tumor niche (Li et al., 2007; Valkenburg et al., 2018). The crosstalk between tumor cells and non-tumor cells was found taking an active part in regulating the development and therapeutic responses of cancer (Zhang et al., 2020a). Among cells of TME, different types of tumor infiltrating immune cells build up tumor immune microenvironment (TIME). Tumor infiltrating lymphocytes, such as B cells, CD4 positive T helper cells, CD8 positive cytotoxic T lymphocytes and regulatory T cells (Tregs), are communicating and cooperating with other tumor infiltrating immune cells including macrophages, natural killer cells and dendritic cells (Zhang et al., 2020b). Significantly influencing the survival and the immunotherapy response of patients (Zhang et al., 2020b), TIME is essential in the progress and the treatment of CRC.
Defined to be non-coding RNAs longer than 200 nucleotides in length (Cao, 2014), long non-coding RNA (lncRNAs) are important regulators of multiple biological processes, including cell proliferation (Xiong et al., 2019), apoptosis (Huang et al., 2019), differentiation, tumorigenesis (Bhan and Mandal, 2014), metastasis (Tian et al., 2019), cell cycle regulation (Wu et al., 2018), epithelial-mesenchymal transition (Wang et al., 2019a) and drug resistance (Wei et al., 2019) by forming RNA-RNA, RNA-DNA, RNA-protein interactions and serving as competing endogenous RNAs (ceRNA) in a variety of regulatory mechanisms (Yao et al., 2019). Actually, emerging evidence has implicated that lncRNAs are key coordinators and regulators within tumor infiltrating immune cells that build up the complex “ecosystem” of TIME, associating with recruitment, infiltration, differentiation, activation and pro-/anti-tumor function in those infiltrating immune cells (Sage et al., 2018; Xu et al., 2019; Zhang et al., 2020b; Zhang et al., 2021). By mediating and regulating important mechanisms and processes of immune response in the microenvironment (Bhan and Mandal, 2014; Zhou et al., 2019), lncRNAs within the tumor infiltrating immune cells occupy a central role in immunity regulation of the TIME, as well as in the development, progression and maintenance of many human tumors (Denaro et al., 2019), suggesting that TIME related lncRNAs (TRLs) could be potential diagnostic markers and therapeutic targets in CRC.
In this study, we developed a prognostic TRLs signature for prognosis and immunotherapy response predictions. The performance of model was validated with multiple independent cohorts, proving its potential to serve as a reliable predictor for patient survival and an indicator for immunotherapy.
Materials and methods
Data collection
Datasets of colorectal cancer cases
Transcriptome and clinical data of CRC cases were obtained from the Gene Expression Omnibus (GEO database, https://www.ncbi.nlm.nih.gov/geo/). Data collected from GEO was analyzed by Affymetrix Human Genome U133 2.0 Plus GeneChip Set platform. Clinical information and transcriptional profiles were downloaded from The Cancer Genome Atlas (TCGA, https://portal.gdc.cancer.gov/). Finally, excluding cases with incomplete clinical information, 519 cases of GSE39582 (Marisa et al., 2013) served as training cohort, 595 cases of TCGA CRC and 693 cases of GSE14333 (Jorissen et al., 2009), GSE17538 (Smith et al., 2010; Freeman et al., 2012; Williams et al., 2015; Chen et al., 2019), GSE33113 (de Sousa et al., 2011; Kemper et al., 2012), GSE37892 (Laibe et al., 2012) and GSE39084 (Kirzin et al., 2014) were used as two independent testing cohorts. The summary of clinical information of the three cohorts was shown in Table 1.
Datasets of immune cell lines and colorectal cancer cell lines
Representing 17 different immune cell types, transcriptional profiles of 97 non-treated immune cell lines of healthy volunteers are collected from GEO database (Supplementary Table S1). Transcriptional profiles of 61 CRC cell lines were obtained from Cancer Cell Line Encyclopedia (CCLE, https://sites.broadinstitute.org/ccle/datasets) project. The downloaded transcription profiles of immune cell lines and CRC cell lines were all originally analyzed by Affymetrix Human Genome U133 2.0 Plus GeneChip Set platform.
Data preprocessing
The downloaded GEO and CCLE transcriptional profiles were based on the Affymetrix Human Genome U133 2.0 Plus GeneChip Set. Probe information of the chip was reannotated by NetAffx Annotation Files (HG-U133_Plus_2 Annotations release 36, https://www.affymetrix.com/support/technical/byproduct.affx?product=hg-u133-plus), Gencode files (Long non-coding RNA gene annotation release 38, https://www.gencodegenes.org/human/) and Refseq files (Refseq H_sapiens annotation, https://ftp.ncbi.nlm.nih.gov/refseq/H_sapiens/annotation/) to find out probes that matched long non-coding RNAs, which were labeled as “lncRNA” in Gencode or “long non-coding RNA” in Refseq. Among 50,000 probes of the gene chip, only 2,287 probes had Ensembl ID or Refseq ID annotated as “lncRNA” or “long non-coding RNA”, which corresponded to 1892 unique lncRNA Ensembl IDs. Similar methods were also applied on the transcriptional profiles of TCGA to obtain the lncRNAs and their expression profiles. Finally, the shared 1724 lncRNAs were identified and the corresponding lncRNA expression matrixes were therefore established (Figure 1).
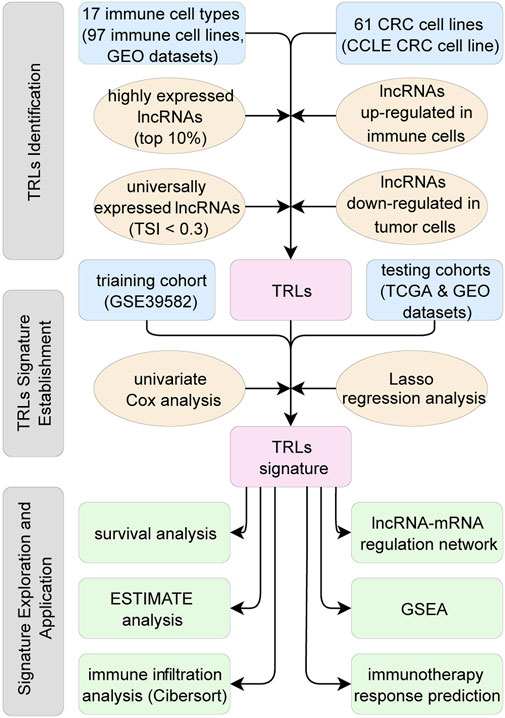
FIGURE 1. Flow chart of this study. First, TRLs of the CRC were identified. Second, TRLs signature was established utilizing Lasso regression. Third, TRLs signature was assessed on independent datasets and the related biological mechanisms were explored.
Prognostic TRLs signature development
Identification of TRLs
The following three steps built up a workflow for identifying TRLs. First, utilizing the gene transcription profiles of immune cells, the top 10% expressed lncRNAs in each immune cell type were identified as immune-related lncRNAs. Second, tissue specificity index (TSI) (Yanai et al., 2005) was calculated across 17 immune cell types to identify universally expressed immune-related lncRNAs in all the immune cells of TIME. Ranging from 0 to 1, the smaller the TSI is, the more consistent the particular lncRNA expressing across all types of immune cells. Here, lncRNAs with TSI smaller than 0.3 were selected, so that universally high-expressed lncRNAs in all types of immune cells were identified. Third, using limma package (Ritchie et al., 2015), lncRNAs upregulated in immune cell lines and downregulated in CRC cell lines (logFoldChange >1.0 and adjusted p < 0.05) were recognized as lncRNAs expressing in immune cells rather than in the tumor cells. In other words, these lncRNAs were mainly expressed in the TIME, which were regarded as TIME related lncRNAs. Step by step, universally high-expressed lncRNAs in the immune cells of TIME were identified, namely TRLs in this study (Figure 1).
Development and validation of the TRLs signature
The prognostic value of each TRL was evaluated by univariate Cox proportional hazards regression analysis with the training set. TRLs with p < 0.1 were selected as candidates for the construction of the signature. Utilizing the LASSO regression analysis (Supplementary Figure S1), the TRLs signature was established based on the training cohort, and the risk score formula was generated as follows
Independent prognostic role of the TRLs signature
To investigate whether the signature could be independent of other clinical parameters, including risk group, age, sex, stage, location of the tumor, microsatellite stability (MSS) or microsatellite instability (MSI) status and consensus molecular subtypes (CMS) (Guinney et al., 2015), univariate and multivariate Cox regression analyses were performed, and p < 0.05 were considered as statistically significant.
Differentially expressed gene (DEG) analys and gene set enrichment analysis (GSEA)
DEGs between the low-risk group and high-risk group were identified using the Limma package with age, sex and TNM stage factors adjusted (Ritchie et al., 2015). DEGs were visualized with pheatmap package in R. Log-fold-change > 0.5 and adjusted p-value < 0.05 were cutoff value for DEG analysis. Based on the results of DEG analysis and gene set collections of Molecular Signatures Database (MSigDB), GSEA was performed with clusterProfiler package (Yu et al., 2012) and HTSanalyzeR2 package (Wang et al., 2011). Pathways and gene sets from “curated gene sets” collection (C2), “ontology gene sets” collection (C5) and “immunologic signature gene sets” collection (C7) are used to perform the GSEA.
The TRLs signature lncRNA-mRNA regulation network
LncRNAs associated RNA interactions, which included information about lncRNAs and their target mRNAs in the regulatory network, were collected from four different manually-curated and experimentally-supported RNA databases, including starBase v2.0 (Li et al., 2014), LncACTdb 2.0 (Wang et al., 2019b), LncTarD (Zhao et al., 2020) and LnCeCell (Wang et al., 2021). Over 1,000 pairs of lncRNA–target mRNA involving lncRNAs in the TRLs signature were selected. Spearman correlation analysis were applied to calculate the correlation coefficients between the expression of 10 lncRNAs of the signature and the expression of their target mRNAs based on transcription profiles of immune cell lines. Selecting the top 30 most correlated target mRNAs for each lncRNA, a regulatory network of the TRLs signature was constructed and visualized with Cytoscape software (version 3.8.2). The correlated target mRNAs were analyzed with GSEA to find out the targeted pathways, process of which was the same as above.
Tumor immune infiltration analysis
Tumor purity and the infiltration level of stromal cells (StromalScore) and immune cells (ImmuneScore) were estimated by ESTIMATE package (Yoshihara et al., 2013). The fraction of tumor infiltrating immune cells in each sample, such as B cells, T cells, dendritic cells, macrophages, neutrophils and so on, were estimated by CIBERSORT algorithm (Newman et al., 2019). The fractions of 22 types of tumor infiltrating immune cells were calculated by Cibersort algorithm. Among them, nine types of immune cells playing important roles in the effect and regulation of the tumor immunology, including different types of T cells, NK cells and macrophages, were chosen to displayed in the figure. The correlations between risk score and StromalScore, ImmuneScore, tumor purity, fractions of immune cells were explored to identify whether the TRLs signature could be a reliable indicator in the CRC TIME.
TRLs signature in immunotherapy response prediction
The expression level of immune checkpoint blockade therapy associated genes, such as PD-1 (PDCD1), PD-L1 (CD274), PD-L2 (PDCD1LG2), are closely related to the response of immunotherapy. The correlations between risk score and the expression of those key genes were investigated (p < 0.05). GSE165252, a dataset containing immunotherapy response information and transcriptional profiles of pre-treatment CRC tissues, was download from GEO and served as an external dataset to verify the TRLs signature’s capacity of predicting immunotherapy treatment response. Receiver operating characteristic (ROC) curve was therefore performed and the area under the ROC curve (AUC) was also calculated by pROC package.
Statistical analysis
All statistical analyses were performed with R (version 4.1.0). T tests and Wilcoxon tests were performed for differential gene expression analyses and differential immune cell infiltration analysis. The Kaplan-Meier survival curve with log-rank test was used to compare the survival difference between the two groups. Univariate and multivariate Cox regression models were utilized to validate the prognosis value of the TRLs signature and other clinical parameters in patients of CRC. Pearson correlation analysis were applied to perform the correlation analyses of the study. DeLong test was employed to calculate the confidence intervals for the AUC values of the ROC curves.
Results
The construction of prognostic TRLs signature
Clinical data and gene expression data of 1807 CRC patients from multiple datasets were collected and divided into three cohorts (Table 1). A total of 1724 unique lncRNAs were identified from downloaded transcription profiles, and 60 lncRNAs were found to be universally high-expressed in the immune cells of TIME. Among the 60 TRLs, 18 lncRNAs were found to be prognostic markers for the survival of CRC patients and were selected for the construction of the signature. Using LASSO regression analysis (Supplementary Figure S1), a 10 TRLs signature was established, and the risk score of each patient was calculated. The corresponding coefficients of the TRLs were listed in the Supplementary Table S2. The flowchart of the whole study was showed in Figure 1.
The prognostic value of the TRLs signature
Based on the cut‐off value calculated by Youden index and the risk score of each patient, patients were divided into a high-risk group and a low-risk group in both training cohort and independent testing cohorts (Figures 2A,C,E). Kaplan-Meier curves with log-rank test (Figures 2B,D,F) and the univariate Cox regression analysis (Table 2) showed that the high‐risk patients had significant shorter disease-free survival (DFS) than the low‐risk patients in both training cohort (hazard ratio (HR) = 2.63, 95% confidence interval (CI) = 1.9–3.63, p < 0.001) and testing cohorts (testing cohort 1: HR = 1.6, 95% CI = 1.19–2.16, p = 0.002; testing cohort 2: HR = 1.64, 95% CI = 1.19–2.26, p = 0.002). Additionally, multivariate Cox regression analysis were also performed in the training and testing cohorts to examine whether the TRLs signature was an independent prognostic factor in CRC. Taking into consideration the risk group and other clinical or pathological parameters which were found significant in the previous univariate Cox regression, the results of multivariate Cox regression (Table 2) showed that risk group was an independent prognostic factor for DFS prediction in both training cohort (HR = 2.18, 95% CI = 1.53–3.12; p < 0.001) and testing cohorts (testing cohort 1: HR = 1.40, 95% CI = 1.04–1.91, p < 0.05; testing cohort 2: HR = 1.58, 95% CI = 1.14–2.18, p < 0.0001). It indicated that the TRLs signature was a promising predictor of prognosis for CRC patients, which had the potential to find clinical application.
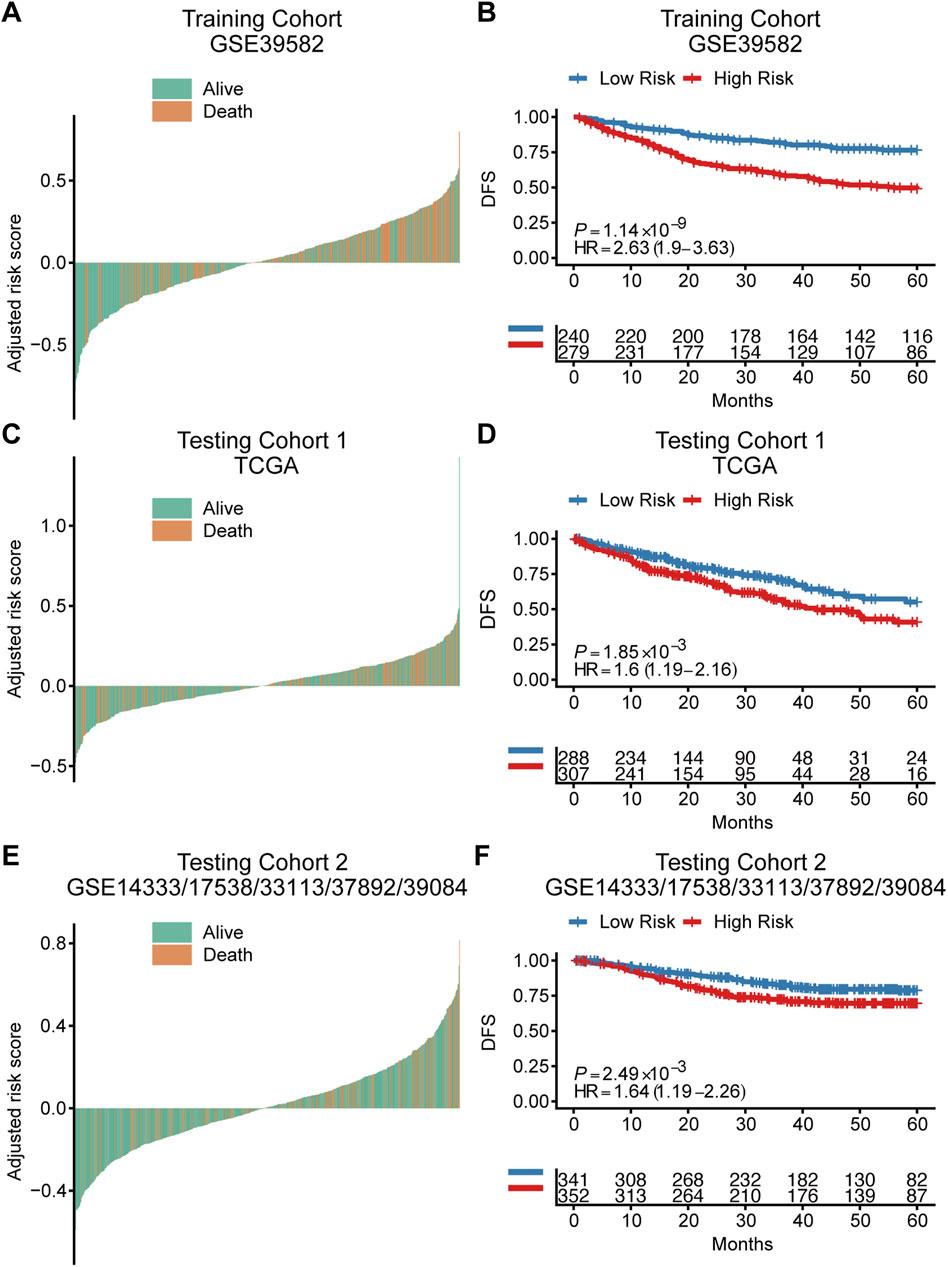
FIGURE 2. The prognostic value of the TRLs signature for colorectal cancer. Waterfall plots showed the distribution of survival status for patients of different TRLs signature risk groups in the training cohort (A), testing cohort 1 (C) and testing cohort 2 (E). Kaplan–Meier curves of DFS according to risk groups in the training cohort (B), testing cohort 1 (D) and testing cohort 2 (F). DFS: disease-free survival.
The relationship between TRLs signature and immune pathways
Between the low-risk group and high-risk group, 56 DEGs were identified. The expression of 56 DEGs, score group and corresponding clinical, molecular and pathological features of each patient were visualized with a heatmap and a volcano plot (Figure 3A; Supplementary Figure S2). Based on DEGs and the clusterProfiler package, the top 20 enriched pathways were shown in (Figure 3B). It showed that immune-related pathways involving CD8 positive T cells, CD4 positive T cells and T lymphocytes were among the top ones, suggesting that the TRLs signature risk score correlated closely with immune cells and immunity-related regulation. High-risk group were enriched in the pathway of tumor immune escape (Figure 3C), implying that immune escape might be one of the reasons contributing to the worse prognosis of the high-risk group. It was also found that low-risk group were enriched in genes sets that down-regulated in CTLA4 expressing CD4 positive cells and exhausted CD8 positive T cells, suggesting that patients of the low-risk group were not the potential target of immunotherapy (Figures 3D,E).
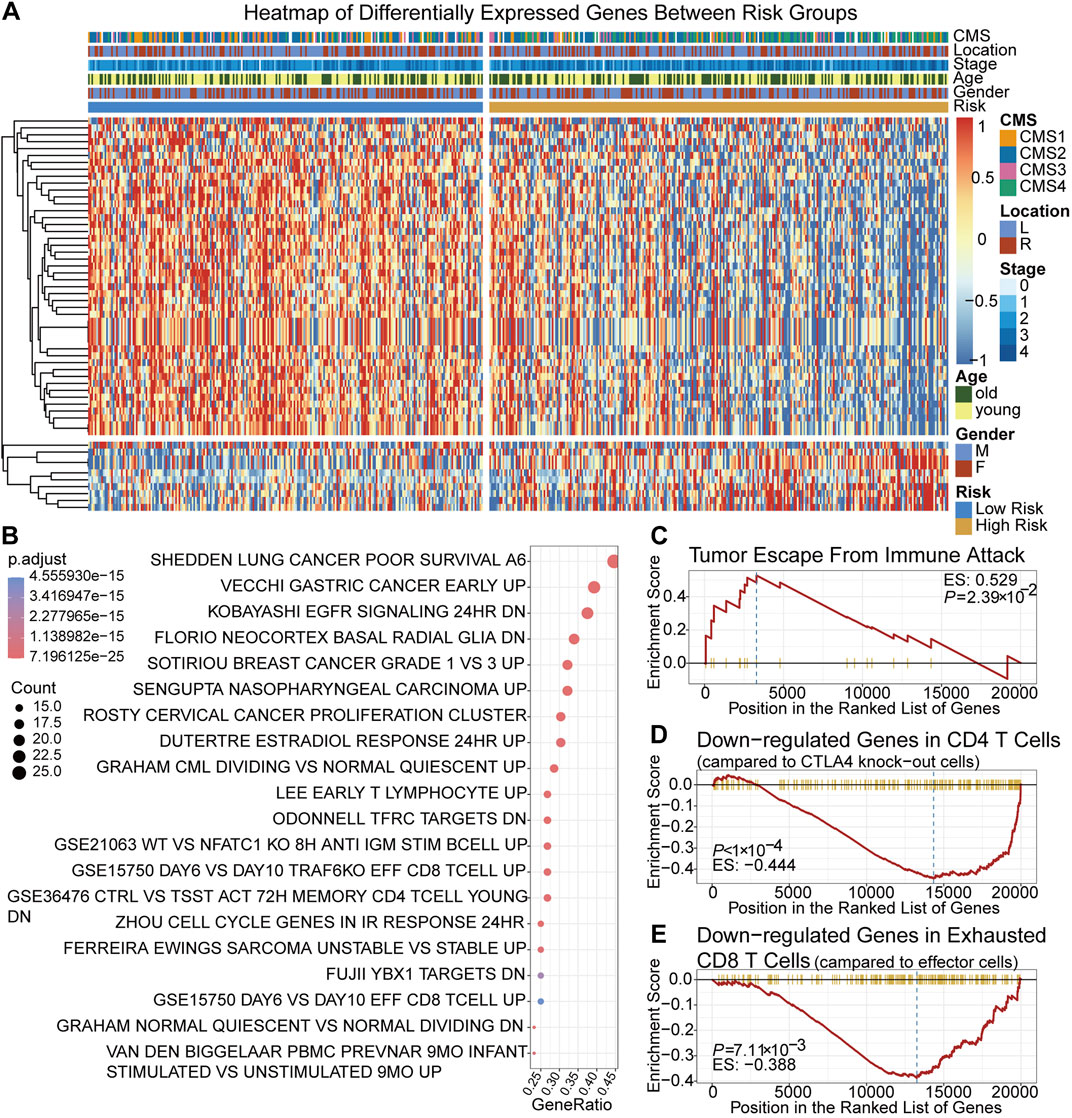
FIGURE 3. Identification and gene enrichment analysis of 56 DEGs between two risk groups. (A) A heatmap of 56 DEGs. (B) Bubble chart of the top 20 enriched MSigDB pathways of the DEGs. (C–E) Gene set enrichment plots of cancer immune escape related pathways and cancer immunotherapy related pathways. MSigDB pathways: C2, C5 and C7 pathways collection of the Molecular Signatures Database.
The complex LncRNA-mRNA regulation network
Providing an insight into the complex regulatory mechanism of 10 TRLs of the signature, the most correlated lncRNA-target mRNA in the immune cells of the TIME were visualized with a network based on four manually-curated and experimentally-supported lncRNA-target mRNA interaction databases (Figure 4). Analyzing target mRNAs with GSEA, multiple pathways related to immunoregulatory mechanisms and immune cells were enriched, indicating that TRLs of the signature exerted a great impact on the TIME and tumor-related immune response (Table 3).
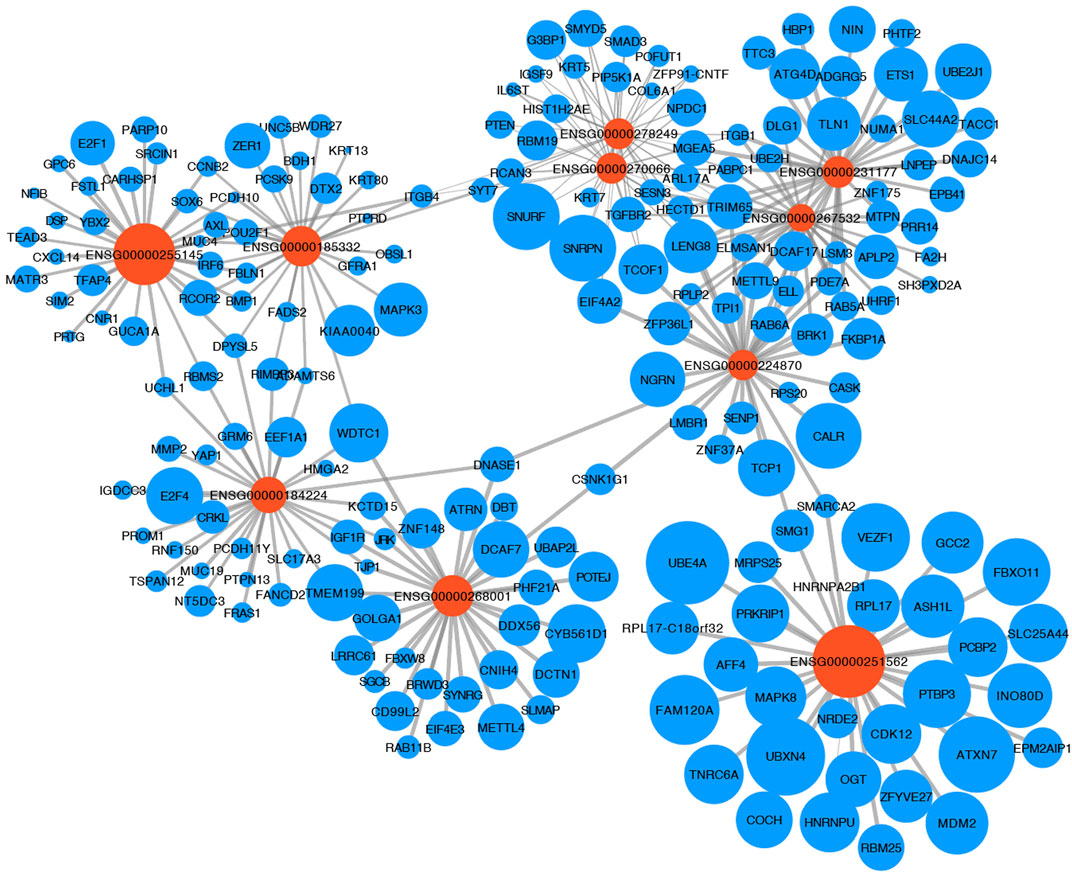
FIGURE 4. LncRNA-mRNA regulation network. The relationship between 10 TRLs of the signature (orange node) and their most correlated target mRNAs (blue nodes) was shown. The size of the nodes represented the average expression of lncRNAs and mRNAs in the immune cells, and the width of the lines represented the correlation between the expression of the lncRNAs and the expression of their targets.
Tumor immune environment characterization
Assessed with ESTIMATE algorithm, the infiltration level of stromal cells (StromalScore) and immune cells (ImmuneScore) were significantly higher in high-risk group (p < 0.05 and p < 0.001, respectively), while significant lower tumor purity was observed in the low-risk group (p < 0.001, Figures 5A–C). The results of CIBERSORT immune infiltration analysis showed that the fraction of M2 macrophages and Tregs was significantly higher in the TIME in both training and testing cohorts (Figures 5D–F). In summary, the tumor tissue of the high-risk group was associated with pro-tumor TIME and greater degree of pro-tumor immune cells infiltration.
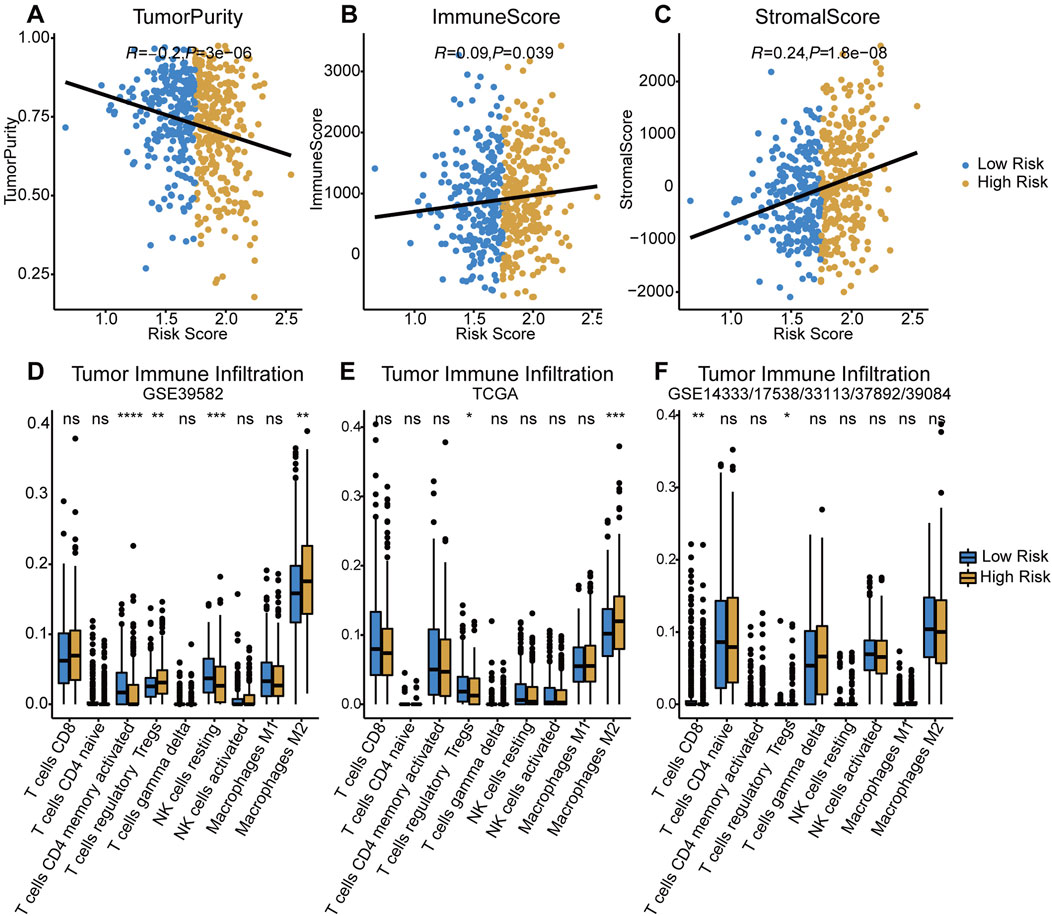
FIGURE 5. Evaluation of tumor immune infiltration in both risk groups. (A–C) Comparisons of tumor purity, immune score and stromal score between low-/high-risk groups. (D–F) Difference of tumor infiltrating immune cells in two risk groups among three cohorts. p < 0.0001 ****, p < 0.001 ***, p < 0.01 **, p < 0.05 *, not significant: ns.
The TRLs signature prediction in colorectal cancer immunotherapy
The expression of immunotherapy targets, such as PD‐1 (PDCD-1), PD‐L1 (CD274) and PD‐L2 (PDCD1LG2), were evaluated in both risk groups. Both PD‐1 and PD‐L2 were significantly upregulated in high-risk group (Figures 6A,B), suggesting the potential role of the TRLs signature in stratifying CRC patients for immune checkpoint inhibitor therapy. Meanwhile, immunotherapy dataset GSE165252, which was originally about atezolizumab (a PD-L1 inhibitor) treating esophageal adenocarcinoma, was used as an external dataset to verified the signature’s ability of making immunotherapy response predictions. As a result, an AUC value of 0.70 (95% CI = 0.51–0.88) was achieved (Figure 6C), and higher proportion of responders was also observed in the high-risk group (Figure 6D). The TRLs signature was capable of predicting immunotherapy response, suggesting that patients of the high-risk group would get more rewards from the anti-PD-1/PD-L1 therapy.
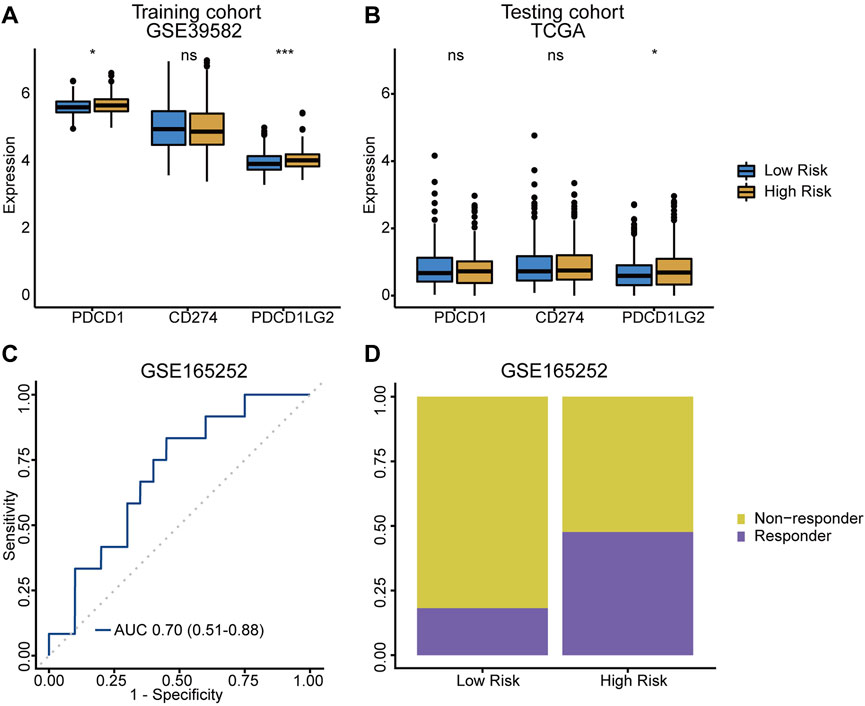
FIGURE 6. TRLs signature predicting immunotherapy response. (A,B) Difference of crucial immune checkpoint genes expression levels between low-/high-risk group. (C) The ROC curve for predicting anti‐PD-L1 immune checkpoint blockade therapy response of the TRLs signature. (D) Difference of anti‐PD-L1 immune checkpoint blockade therapy response rates between low-/high-risk groups. PDCD1: PD-1. CD274: PD-L1. PDCD1LG2: PD-L2. p < 0.001 ***, p < 0.01 **, p < 0.05 *, not significant: ns.
Discussion
In recent years, lncRNAs have attracted extensive attention and there are a great number of studies about the relationship between lncRNAs of the TIME and characteristics of the tumor, including the prognosis, TME and anti-tumor immunity. A large number of lncRNAs are expressing different patterns in types of cancer including CRC (Bhan et al., 2017; Deng et al., 2017) and regarded as a vital player in tumorigenesis, anti-cancer immune response and immunotherapy (Yu et al., 2018). The TIME and its regulation are sculpted by tumor infiltrating immune cells, and lncRNAs of the tumor infiltrating immune cells plays an important role in this procedure (Denaro et al., 2019), indicating that the lncRNAs of CRC TIME has an unique value in prognostic and guiding patient stratification for immunotherapy. Here, a TRLs signature of CRC was established and verified in independent cohorts, providing distinct survival and immunotherapy response prediction for low and high-risk groups.
By forming a complex regulation network, TRLs of the signature modified the expression patterns of multiple target genes in the tumor infiltrating immune cells of TIME, especially genes related to immunoregulatory mechanisms and pathways. A previous study reported that lncRNA MALAT1 (ENSG00000251562 of the TRLs signature) promotes tumor angiogenesis in thyroid cancer by regulating functions of macrophage in the TIME (Huang et al., 2017). Due to the difference in the gene expression pattern between the high and low-risk groups controlled by multiple TIME lncRNA-based mechanisms, the infiltration, activation, function and fate of tumor infiltrating immune cells differ between the risk groups (Huarte and Rinn, 2010; Batista and Chang, 2013; Uthaya Kumar and Williams, 2020). Here, a significantly greater degree of pro-tumor immune infiltration was found in the high-risk group, especially M2 macrophage cells and Tregs, which impaired anti-tumor immunity, promoted tumor progression and contributed to tumor immune escape and poor prognosis (Bader et al., 2020; Zeng et al., 2020). As a result, profound changes in the niche the led to significant alterations in the gene expression profiles and behaviors of the tumor, bringing about different courses and outcomes of disease between low-risk group and high-risk group.
Having the power to rewrite the regulation network in the tumor-infiltrating immune cells and the TIME (Uthaya Kumar and Williams, 2020; Wells et al., 2020), lncRNAs also take part in controlling the immune surveillance, drug resistance and the efficacy of immunotherapy (Pi et al., 2021). Many studies have shown that immune-related lncRNAs were capable of predicting the response for immune checkpoint inhibitor therapy (Jiang et al., 2020; Sun et al., 2020; Ma et al., 2021; Xu et al., 2021; Zhou et al., 2021). Consistent with the reported findings, the CRC TRLs signature provided us with immunotherapy treatment indications, showing that patients of the high-risk group were associated with higher expression of cellular receptors targeted by immune checkpoint inhibitor therapy and favorable response towards immunotherapy, which is of great help to stratify CRC patients for immunotherapy (Bateman, 2021).
There are some limitations that should be acknowledged. First, as a retrospective study, the model was trained and validated on existing datasets, indicating that TRLs signature needs to be further validate on large prospective cohorts. Second, the major limitation of the study was the lack of experimental validation. Although the model performed well in survival and immunotherapy response prediction, the underlying biological functions of the signature’s TRLs and the complicated regulation mechanisms between TRLs and their target mRNAs the in the TME were not fully understood, which should be further studied with cellular and molecular experiments.
In summary, not only provides distinct survival prediction and insights into the TIME for the two risk groups, our TRLs signature also gives doctors with immunotherapy treatment indications, suggesting that the patients of low-risk group may have a chance to live longer and patients of high-risk group could benefit more from the immunotherapy.
Data availability statement
The datasets presented in this study can be found in online repositories. The names of the repository/repositories and accession number(s) can be found in the article/Supplementary Material.
Author contributions
Study design and conception were proposed by FG and X-JW; writing and revision of paper were done by CH, DC, FG, and X-JW; data analyses and interpretation was conducted by CH and DC; data management was done by CH, M-EZ, DF, and C-L; data acquisition was done by M-YL, Z-PH, and WW. All authors interpreted the results and revised the manuscript. All authors read and approved the final manuscript.
Funding
This study was supported by the National Key Clinical Discipline, the National Natural Science Foundation of China (No. 82002221, FG), The Sixth Affiliated Hospital of Sun Yat sen University Start-up Fund for Returnees (No. R20210217202501975, FG), Guangzhou Basic and Applied Basic Research Fund (No. 202102020820, FG), the Sun Yat-sen University 100 Top Talent Scholars Program–China (No. P20190217202203617, FG), National Natural Science Foundation of China (No. 81972212, XW), Natural Science Foundation of Guangdong Province, China (No. 2019A1515010063, XW), the program of Guangdong Provincial Clinical Research Center for Digestive Diseases (2020B1111170004, XW), Guangzhou Key Research and Development Project (No. 202206080008, XW).
Conflict of interest
The authors declare that the research was conducted in the absence of any commercial or financial relationships that could be construed as a potential conflict of interest.
Publisher’s note
All claims expressed in this article are solely those of the authors and do not necessarily represent those of their affiliated organizations, or those of the publisher, the editors and the reviewers. Any product that may be evaluated in this article, or claim that may be made by its manufacturer, is not guaranteed or endorsed by the publisher.
Supplementary material
The Supplementary Material for this article can be found online at: https://www.frontiersin.org/articles/10.3389/fgene.2022.993714/full#supplementary-material
References
Ahiko, Y., Shida, D., Kudose, Y., Nakamura, Y., Moritani, K., Yamauchi, S., et al. (2021). Recurrence hazard of rectal cancer compared with colon cancer by adjuvant chemotherapy status: A nationwide study in Japan. J. Gastroenterol. 56 (4), 371–381. Epub 20210221. doi:10.1007/s00535-021-01771-6
Bader, J. E., Voss, K., and Rathmell, J. C. (2020). Targeting metabolism to improve the tumor microenvironment for cancer immunotherapy. Mol. Cell 78 (6), 1019–1033. Epub 2020/06/20. doi:10.1016/j.molcel.2020.05.034
Bateman, A. C. (2021). Immune checkpoint inhibitor therapy in colorectal cancer-the role of cellular pathology. Int. J. Surg. Pathol. 29 (6), 584–591. Epub 20210618. doi:10.1177/10668969211025844
Batista, P. J., and Chang, H. Y. (2013). Long noncoding rnas: Cellular address codes in development and disease. Cell 152 (6), 1298–1307. doi:10.1016/j.cell.2013.02.012
Bhan, A., and Mandal, S. S. (2014). Long noncoding rnas: Emerging stars in gene regulation, epigenetics and human disease. ChemMedChem 9 (9), 1932–1956. Epub 2014/03/29. doi:10.1002/cmdc.201300534
Bhan, A., Soleimani, M., and Mandal, S. S. (2017). Long noncoding rna and cancer: A new paradigm. Cancer Res. 77 (15), 3965–3981. Epub 2017/07/14. doi:10.1158/0008-5472.CAN-16-2634
Cao, J. (2014). The functional role of long non-coding rnas and epigenetics. Biol. Proced. Online 16, 11. Epub 2014/10/03. doi:10.1186/1480-9222-16-11
Chen, M. S., Lo, Y. H., Chen, X., Williams, C. S., Donnelly, J. M., Criss, Z. K., et al. (2019). Growth factor-independent 1 is a tumor suppressor gene in colorectal cancer. Mol. Cancer Res. 17 (3), 697–708. Epub 20190103. doi:10.1158/1541-7786.MCR-18-0666
Chen, S. J., Wang, S. C., and Chen, Y. C. (2021). The immunotherapy for colorectal cancer, lung cancer and pancreatic cancer. Int. J. Mol. Sci. 22 (23), 12836. Epub 20211127. doi:10.3390/ijms222312836
Cohen, R., Rousseau, B., Vidal, J., Colle, R., Diaz, L. A., and Andre, T. (2020). Immune checkpoint inhibition in colorectal cancer: Microsatellite instability and beyond. Target. Oncol. 15 (1), 11–24. doi:10.1007/s11523-019-00690-0
Cortes-Ciriano, I., Lee, S., Park, W. Y., Kim, T. M., and Park, P. J. (2017). A molecular portrait of microsatellite instability across multiple cancers. Nat. Commun. 8, 15180. Epub 20170606. doi:10.1038/ncomms15180
de Sousa, E. M. F., Colak, S., Buikhuisen, J., Koster, J., Cameron, K., de Jong, J. H., et al. (2011). Methylation of cancer-stem-cell-associated wnt target genes predicts poor prognosis in colorectal cancer patients. Cell Stem Cell 9 (5), 476–485. doi:10.1016/j.stem.2011.10.008
Denaro, N., Merlano, M. C., and Lo Nigro, C. (2019). Long noncoding rnas as regulators of cancer immunity. Mol. Oncol. 13 (1), 61–73. Epub 2018/12/01. doi:10.1002/1878-0261.12413
Deng, H., Wang, J. M., Li, M., Tang, R., Tang, K., Su, Y., et al. (2017). Long non-coding rnas: New biomarkers for prognosis and diagnosis of colon cancer. Tumour Biol. 39 (6), 1010428317706332. Epub 2017/06/24. doi:10.1177/1010428317706332
Doki, Y., Ajani, J. A., Kato, K., Xu, J., Wyrwicz, L., Motoyama, S., et al. (2022). Nivolumab combination therapy in advanced esophageal squamous-cell carcinoma. N. Engl. J. Med. 386 (5), 449–462. doi:10.1056/NEJMoa2111380
Fang, L., Yang, Z., Zhang, M., Meng, M., Feng, J., and Chen, C. (2021). Clinical characteristics and survival analysis of colorectal cancer in China: A retrospective cohort study with 13, 328 patients from southern China. Gastroenterol. Rep. 9 (6), 571–582. Epub 20211117. doi:10.1093/gastro/goab048
Freeman, T. J., Smith, J. J., Chen, X., Washington, M. K., Roland, J. T., Means, A. L., et al. (2012). Smad4-Mediated signaling inhibits intestinal neoplasia by inhibiting expression of beta-catenin. Gastroenterology 142 (3), 562–571.e2. Epub 20111122. doi:10.1053/j.gastro.2011.11.026
Gibney, G. T., Weiner, L. M., and Atkins, M. B. (2016). Predictive biomarkers for checkpoint inhibitor-based immunotherapy. Lancet. Oncol. 17 (12), e542–e551. doi:10.1016/S1470-2045(16)30406-5
Guinney, J., Dienstmann, R., Wang, X., de Reynies, A., Schlicker, A., Soneson, C., et al. (2015). The consensus molecular subtypes of colorectal cancer. Nat. Med. 21 (11), 1350–1356. Epub 2015/10/13. doi:10.1038/nm.3967
Huang, J. K., Ma, L., Song, W. H., Lu, B. Y., Huang, Y. B., Dong, H. M., et al. (2017). Lncrna-Malat1 promotes angiogenesis of thyroid cancer by modulating tumor-associated macrophage Fgf2 protein secretion. J. Cell. Biochem. 118 (12), 4821–4830. Epub 20170613. doi:10.1002/jcb.26153
Huang, W., Su, G., Huang, X., Zou, A., Wu, J., Yang, Y., et al. (2019). Long noncoding rna Pcat6 inhibits colon cancer cell apoptosis by regulating anti-apoptotic protein arc expression via Ezh2. Cell Cycle 18 (1), 69–83. Epub 2018/12/21. doi:10.1080/15384101.2018.1558872
Huarte, M., and Rinn, J. L. (2010). Large non-coding rnas: Missing links in cancer? Hum. Mol. Genet. 19 (R2), R152–R161. Epub 20100820. doi:10.1093/hmg/ddq353
Jiang, W., Zhu, D., Wang, C., and Zhu, Y. (2020). An immune relevant signature for predicting prognoses and immunotherapeutic responses in patients with muscle-invasive bladder cancer (mibc). Cancer Med. 9 (8), 2774–2790. Epub 20200225. doi:10.1002/cam4.2942
Jorissen, R. N., Gibbs, P., Christie, M., Prakash, S., Lipton, L., Desai, J., et al. (2009). Metastasis-associated gene expression changes predict poor outcomes in patients with dukes stage B and C colorectal cancer. Clin. Cancer Res. 15 (24), 7642–7651. doi:10.1158/1078-0432.CCR-09-1431
Kassambara, A., Kosinski, M., and Biecek, P. (2021). Survminer: Drawing survival curves using “Ggplot2”. R package version 0.4.9. https://CRAN.R-project.org/package=survminer.
Kemper, K., Versloot, M., Cameron, K., Colak, S., de Sousa e Melo, F., de Jong, J. H., et al. (2012). Mutations in the ras-raf Axis underlie the prognostic value of Cd133 in colorectal cancer. Clin. Cancer Res. 18 (11), 3132–3141. Epub 20120410. doi:10.1158/1078-0432.CCR-11-3066
Kim, H. G., Kim, H. S., Yang, S. Y., Han, Y. D., Cho, M. S., Hur, H., et al. (2021). Early recurrence after neoadjuvant chemoradiation therapy for locally advanced rectal cancer: Characteristics and risk factors. Asian J. Surg. 44 (1), 298–302. Epub 20200725. doi:10.1016/j.asjsur.2020.07.014
Kirzin, S., Marisa, L., Guimbaud, R., De Reynies, A., Legrain, M., Laurent-Puig, P., et al. (2014). Sporadic early-onset colorectal cancer is a specific sub-type of cancer: A morphological, molecular and Genetics study. PLoS One 9 (8), e103159. Epub 20140801. doi:10.1371/journal.pone.0103159
Laibe, S., Lagarde, A., Ferrari, A., Monges, G., Birnbaum, D., Olschwang, S., et al. (2012). A seven-gene signature aggregates a subgroup of stage ii colon cancers with stage iii. OMICS 16 (10), 560–565. Epub 20120823. doi:10.1089/omi.2012.0039
Li, H., Fan, X., and Houghton, J. (2007). Tumor microenvironment: The role of the tumor stroma in cancer. J. Cell. Biochem. 101 (4), 805–815. Epub 2007/01/18. doi:10.1002/jcb.21159
Li, J. H., Liu, S., Zhou, H., Qu, L. H., and Yang, J. H. (2014). Starbase V2.0: Decoding mirna-cerna, mirna-ncrna and protein-rna interaction networks from large-scale clip-seq data. Nucleic Acids Res. 42, D92–D97. Database issueEpub 2013/12/04. doi:10.1093/nar/gkt1248
Ma, B., Jiang, H., Luo, Y., Liao, T., Xu, W., Wang, X., et al. (2021). Tumor-infiltrating immune-related long non-coding rnas indicate prognoses and response to Pd-1 blockade in head and neck squamous cell carcinoma. Front. Immunol. 12, 692079. Epub 20211019. doi:10.3389/fimmu.2021.692079
Makker, V., Colombo, N., Casado Herraez, A., Santin, A. D., Colomba, E., Miller, D. S., et al. (2022). Lenvatinib Plus pembrolizumab for advanced endometrial cancer. N. Engl. J. Med. 386 (5), 437–448. Epub 20220119. doi:10.1056/NEJMoa2108330
Marisa, L., de Reynies, A., Duval, A., Selves, J., Gaub, M. P., Vescovo, L., et al. (2013). Gene expression classification of colon cancer into molecular subtypes: Characterization, validation, and prognostic value. PLoS Med. 10 (5), e1001453. Epub 20130521. doi:10.1371/journal.pmed.1001453
Miller, K. D., Nogueira, L., Mariotto, A. B., Rowland, J. H., Yabroff, K. R., Alfano, C. M., et al. (2019). Cancer treatment and survivorship statistics, 2019. Ca. Cancer J. Clin. 69 (5), 363–385. Epub 2019/06/12. doi:10.3322/caac.21565
Newman, A. M., Steen, C. B., Liu, C. L., Gentles, A. J., Chaudhuri, A. A., Scherer, F., et al. (2019). Determining cell type Abundance and expression from bulk tissues with digital cytometry. Nat. Biotechnol. 37 (7), 773–782. Epub 2019/05/08. doi:10.1038/s41587-019-0114-2
Pan, W., Zhao, J., Zhang, S., Chen, X., Liang, W., and Li, Q. (2021). Towards exertion of immunotherapeutics in the treatment of colorectal cancer; adverse sides, challenges, and future directions. Int. Immunopharmacol. 101, 108337. Epub 20211111. doi:10.1016/j.intimp.2021.108337
Pi, Y. N., Qi, W. C., Xia, B. R., Lou, G., and Jin, W. L. (2021). Long non-coding rnas in the tumor immune microenvironment: Biological properties and therapeutic potential. Front. Immunol. 12, 697083. doi:10.3389/fimmu.2021.697083
Ritchie, M. E., Phipson, B., Wu, D., Hu, Y., Law, C. W., Shi, W., et al. (2015). Limma powers differential expression analyses for rna-sequencing and microarray studies. Nucleic Acids Res. 43 (7), e47. Epub 2015/01/22. doi:10.1093/nar/gkv007
Sage, A., Ng, K., Marshall, E., Enfield, K., Stewart, G., Martin, S., et al. (2018). Ma24.06 long non-coding rna expression patterns delineate infiltrating immune cells in the lung tumour microenvironment. J. Thorac. Oncol. 13 (10), S443–S444. doi:10.1016/j.jtho.2018.08.524
Schmid, P., Cortes, J., Dent, R., Pusztai, L., McArthur, H., Kummel, S., et al. (2022). Event-free survival with pembrolizumab in early triple-negative breast cancer. N. Engl. J. Med. 386 (6), 556–567. doi:10.1056/NEJMoa2112651
Siegel, R. L., Miller, K. D., and Jemal, A. (2019). Cancer statistics, 2019. Ca. Cancer J. Clin. 69 (1), 7–34. Epub 2019/01/09. doi:10.3322/caac.21551
Smith, J. J., Deane, N. G., Wu, F., Merchant, N. B., Zhang, B., Jiang, A., et al. (2010). Experimentally derived metastasis gene expression profile predicts recurrence and death in patients with colon cancer. Gastroenterology 138 (3), 958–968. Epub 20091113. doi:10.1053/j.gastro.2009.11.005
Sun, J., Zhang, Z., Bao, S., Yan, C., Hou, P., Wu, N., et al. (2020). Identification of tumor immune infiltration-associated lncrnas for improving prognosis and immunotherapy response of patients with non-small cell lung cancer. J. Immunother. Cancer 8 (1), e000110. doi:10.1136/jitc-2019-000110
Tian, L., Zhao, Z. F., Xie, L., and Zhu, J. P. (2019). Taurine up-regulated 1 accelerates tumorigenesis of colon cancer by regulating mir-26a-5p/mmp14/P38 mapk/hsp27 Axis in vitro and in vivo. Life Sci. 239, 117035. Epub 2019/11/08. doi:10.1016/j.lfs.2019.117035
Uthaya Kumar, D. B., and Williams, A. (2020). Long non-coding rnas in immune regulation and their potential as therapeutic targets. Int. Immunopharmacol. 81, 106279. Epub 20200212. doi:10.1016/j.intimp.2020.106279
Valkenburg, K. C., de Groot, A. E., and Pienta, K. J. (2018). Targeting the tumour stroma to improve cancer therapy. Nat. Rev. Clin. Oncol. 15 (6), 366–381. Epub 2018/04/14. doi:10.1038/s41571-018-0007-1
Wang, L., Cho, K. B., Li, Y., Tao, G., Xie, Z., and Guo, B. (2019). Long noncoding rna (Lncrna)-Mediated competing endogenous rna networks provide novel potential biomarkers and therapeutic targets for colorectal cancer. Int. J. Mol. Sci. 20 (22), E5758. Epub 2019/11/21. doi:10.3390/ijms20225758
Wang, P., Guo, Q., Hao, Y., Liu, Q., Gao, Y., Zhi, H., et al. (2021). Lncecell: A comprehensive database of predicted lncrna-associated cerna networks at single-cell resolution. Nucleic Acids Res. 49 (D1), D125–D133. Epub 2020/11/22. doi:10.1093/nar/gkaa1017
Wang, P., Li, X., Gao, Y., Guo, Q., Wang, Y., Fang, Y., et al. (2019). Lncactdb 2.0: An updated database of experimentally supported cerna interactions curated from low- and high-throughput experiments. Nucleic Acids Res. 47 (D1), D121–D127. Epub 2018/11/27. doi:10.1093/nar/gky1144
Wang, X., Terfve, C., Rose, J. C., and Markowetz, F. (2011). Htsanalyzer: An R/bioconductor package for integrated network analysis of high-throughput screens. Bioinformatics 27 (6), 879–880. Epub 2011/01/25. doi:10.1093/bioinformatics/btr028
Wei, L., Wang, X., Lv, L., Zheng, Y., Zhang, N., and Yang, M. (2019). The emerging role of noncoding rnas in colorectal cancer chemoresistance. Cell. Oncol. 42 (6), 757–768. Epub 2019/07/31. doi:10.1007/s13402-019-00466-8
Wells, A. C., Pobezinskaya, E. L., and Pobezinsky, L. A. (2020). Non-coding rnas in Cd8 T cell biology. Mol. Immunol. 120, 67–73. Epub 20200218. doi:10.1016/j.molimm.2020.01.023
Williams, C. S., Bernard, J. K., Demory Beckler, M., Almohazey, D., Washington, M. K., Smith, J. J., et al. (2015). Erbb4 is over-expressed in human colon cancer and enhances cellular transformation. Carcinogenesis 36 (7), 710–718. Epub 20150427. doi:10.1093/carcin/bgv049
Wu, K., Xu, K., Liu, K., Huang, J., Chen, J., Zhang, J., et al. (2018). Long noncoding rna Bc200 regulates cell growth and invasion in colon cancer. Int. J. Biochem. Cell Biol. 99, 219–225. doi:10.1016/j.biocel.2018.04.001
Xiong, W., Qin, J., Cai, X., Xiong, W., Liu, Q., Li, C., et al. (2019). Overexpression Linc01082 suppresses the proliferation, migration and invasion of colon cancer. Mol. Cell. Biochem. 462 (1-2), 33–40. doi:10.1007/s11010-019-03607-7
Xu, M., Xu, X., Pan, B., Chen, X., Lin, K., Zeng, K., et al. (2019). Lncrna satb2-as1 inhibits tumor metastasis and affects the tumor immune cell microenvironment in colorectal cancer by regulating Satb2. Mol. Cancer 18 (1), 135. Epub 2019/09/08. doi:10.1186/s12943-019-1063-6
Xu, Q., Wang, Y., and Huang, W. (2021). Identification of immune-related lncrna signature for predicting immune checkpoint blockade and prognosis in hepatocellular carcinoma. Int. Immunopharmacol. 92, 107333. Epub 20210121. doi:10.1016/j.intimp.2020.107333
Yanai, I., Benjamin, H., Shmoish, M., Chalifa-Caspi, V., Shklar, M., Ophir, R., et al. (2005). Genome-wide midrange transcription profiles reveal expression level relationships in human tissue specification. Bioinformatics 21 (5), 650–659. Epub 2004/09/25. doi:10.1093/bioinformatics/bti042
Yao, R. W., Wang, Y., and Chen, L. L. (2019). Cellular functions of long noncoding rnas. Nat. Cell Biol. 21 (5), 542–551. Epub 2019/05/03. doi:10.1038/s41556-019-0311-8
Yoshihara, K., Shahmoradgoli, M., Martinez, E., Vegesna, R., Kim, H., Torres-Garcia, W., et al. (2013). Inferring tumour purity and stromal and immune cell admixture from expression data. Nat. Commun. 4, 2612. Epub 2013/10/12. doi:10.1038/ncomms3612
Yu, G., Wang, L. G., Han, Y., and He, Q. Y. (2012). Clusterprofiler: An R package for comparing biological themes among gene clusters. OMICS 16 (5), 284–287. Epub 2012/03/30. doi:10.1089/omi.2011.0118
Yu, W. D., Wang, H., He, Q. F., Xu, Y., and Wang, X. C. (2018). Long noncoding rnas in cancer-immunity cycle. J. Cell. Physiol. 233 (9), 6518–6523. Epub 2018/03/27. doi:10.1002/jcp.26568
Zeng, D., Ye, Z., Wu, J., Zhou, R., Fan, X., Wang, G., et al. (2020). Macrophage correlates with immunophenotype and predicts anti-Pd-L1 response of urothelial cancer. Theranostics 10 (15), 7002–7014. Epub 2020/06/20. doi:10.7150/thno.46176
Zhang, W. L., Liu, Y., Jiang, J., Tang, Y. J., Tang, Y. L., and Liang, X. H. (2020). Extracellular vesicle long non-coding rna-mediated crosstalk in the tumor microenvironment: Tiny molecules, huge roles. Cancer Sci. 111 (8), 2726–2735. Epub 2020/05/22. doi:10.1111/cas.14494
Zhang, Y., Liu, Q., and Liao, Q. (2020). Long noncoding rna: A dazzling dancer in tumor immune microenvironment. J. Exp. Clin. Cancer Res. 39 (1), 231. Epub 2020/11/06. doi:10.1186/s13046-020-01727-3
Zhang, Z., Yan, C., Li, K., Bao, S., Li, L., Chen, L., et al. (2021). Pan-cancer characterization of lncrna modifiers of immune microenvironment reveals clinically distinct de novo tumor subtypes. NPJ Genom. Med. 6 (1), 52. Epub 20210617. doi:10.1038/s41525-021-00215-7
Zhao, H., Shi, J., Zhang, Y., Xie, A., Yu, L., Zhang, C., et al. (2020). Lnctard: A manually-curated database of experimentally-supported functional lncrna-target regulations in human diseases. Nucleic Acids Res. 48 (D1), D118–D126. Epub 2019/11/13. doi:10.1093/nar/gkz985
Zhou, M., Zhang, Z., Bao, S., Hou, P., Yan, C., Su, J., et al. (2021). Computational recognition of lncrna signature of tumor-infiltrating B lymphocytes with potential implications in prognosis and immunotherapy of bladder cancer. Brief. Bioinform. 22 (3), bbaa047. doi:10.1093/bib/bbaa047
Zhou, Y., Zhu, Y., Xie, Y., and Ma, X. (2019). The role of long non-coding rnas in immunotherapy resistance. Front. Oncol. 9, 1292. Epub 2019/12/19. doi:10.3389/fonc.2019.01292
Glossary
lncRNA long non-coding RNA
TIME tumor immune microenvironment
CRC colorectal cancer
TRL tumor immune microenvironment related long non-coding RNA
LASSO Least absolute shrinkage and selection operator
HR hazard ratio;
CI confidence interval
PD-L1 programmed cell death 1 ligand 1
PD-L2 programmed cell death 1 ligand 2
PD-1 programmed cell death 1
TME tumor microenvironment
Treg regulatory T cell
ceRNA competing endogenous RNA
GEO Gene Expression Omnibus
TCGA The Cancer Genome Atlas
COAD colon adenocarcinoma
READ rectum adenocarcinoma
CCLE Cancer Cell Line Encyclopedia
TSI tissue specificity index
MMR mismatch repair
MSS microsatellite stability
MSI microsatellite instability
CMS consensus molecular subtypes
DEG differentially expressed gene
GSEA gene set enrichment analysis
MSigDB Molecular Signatures Database
ROC receiver operating characteristic
AUC area under the curve
DFS disease-free survival
CTLA4 cytotoxic T-lymphocyte associated protein 4.
Keywords: colorectal cancer, tumor microenvironment, tumor immune microenvironment, long non-coding RNA, immunotherapy
Citation: Hu C, Cai D, Zhong M-E, Fan D, Li C-H, Lv M-Y, Huang Z-P, Wang W, Wu X-J and Gao F (2022) Predicting prognosis and immunotherapy response among colorectal cancer patients based on a tumor immune microenvironment-related lncRNA signature. Front. Genet. 13:993714. doi: 10.3389/fgene.2022.993714
Received: 14 July 2022; Accepted: 15 August 2022;
Published: 07 September 2022.
Edited by:
Hifzur R. Siddique, Aligarh Muslim University, IndiaReviewed by:
Hamid Latifi-Navid, National Institute for Genetic Engineering and Biotechnology, IranZishan Wang, Icahn School of Medicine at Mount Sinai, United States
Gabriel B. K. Sasa, Zhejiang Sci-Tech University, China
Copyright © 2022 Hu, Cai, Zhong, Fan, Li, Lv, Huang, Wang, Wu and Gao. This is an open-access article distributed under the terms of the Creative Commons Attribution License (CC BY). The use, distribution or reproduction in other forums is permitted, provided the original author(s) and the copyright owner(s) are credited and that the original publication in this journal is cited, in accordance with accepted academic practice. No use, distribution or reproduction is permitted which does not comply with these terms.
*Correspondence: Xiao-Jian Wu, wuxjian@mail.sysu.edu.cn; Feng Gao, gaof57@mail.sysu.edu.cn
†These authors contributed equally to this study and shared first authorship.