- 1Maize Research Institute, Guangxi Academy of Agricultural Sciences, Nanning, Guangxi, China
- 2Guangxi Academy of Agricultural Sciences, Nanning, Guangxi, China
Maize (Zea mays L.) is the most important food security crop worldwide. Northern corn leaf blight (NCLB), caused by Exserohilum turcicum, severely reduces production causing millions of dollars in losses worldwide. Therefore, this study aimed to identify significant QTLs associated with NCLB by utilizing next-generation sequencing-based bulked-segregant analysis (BSA). Parental lines GML71 (resistant) and Gui A10341 (susceptible) were used to develop segregating population F2. Two bulks with 30 plants each were further selected from the segregating population for sequencing along with the parental lines. High throughput sequencing data was used for BSA. We identified 10 QTLs on Chr 1, Chr 2, Chr 3, and Chr 5 with 265 non-synonymous SNPs. Moreover, based on annotation information, we identified 27 candidate genes in the QTL regions. The candidate genes associated with disease resistance include AATP1, At4g24790, STICHEL-like 2, BI O 3-BIO1, ZAR1, SECA2, ABCG25, LECRK54, MKK7, MKK9, RLK902, and DEAD-box ATP-dependent RNA helicase. The annotation information suggested their involvement in disease resistance-related pathways, including protein phosphorylation, cytoplasmic vesicle, protein serine/threonine kinase activity, and ATP binding pathways. Our study provides a substantial addition to the available information regarding QTLs associated with NCLB, and further functional verification of identified candidate genes can broaden the scope of understanding the NCLB resistance mechanism in maize.
Introduction
Maize (Zea mays L.) is a significant food security crop worldwide, fulfilling human and animal nutrition requirements (Tilman et al., 2011; Stevens and Madani, 2016; Cole et al., 2018). The gradual increase in global population is rapidly impacting food demand, as are the environmental impacts on crops (Tilman et al., 2011). China is among the leading maize producer and consumers, with a 21% share in global maize production (Yang et al., 2019a). The adversities of climate change tend to increase the intensity of both biotic and abiotic factors influencing crop yield (Li et al., 2017; Vaughan et al., 2018; Onyekachi et al., 2019). Maize is prone to multiple pathogens inducing rust, northern corn leaf blight, southern corn leaf blight, and leaf streak, which negatively affect photosynthesis activities. Without effective disease management strategies, the yield losses can reach about 70% (Romero, 2016). Moreover, host resistance is considered an effective long-term control compared to temporary chemical control with potential hazards of chemical pollution (Johnson, 1981; Venter, 2007; Singh et al., 2016; Nazir et al., 2021a).
Genetic control in crops can be categorized as qualitative and quantitative resistance. Qualitative resistance is induced by major resistance genes and is generally genotype-specific with less durable resistance (Parlevliet, 2002; Darino et al., 2016; Galiano-Carneiro and Miedaner, 2017). Several studies have demonstrated the identification of key resistance genes against rust and northern corn leaf blight in maize, including Rp and Ht1, Ht2, Ht3, HtM, and HtN genes (Darino et al., 2016; Galiano-Carneiro and Miedaner, 2017). The effectiveness of qualitative resistance tends to decline with the rapid evolution of pathogens (Ji et al., 2010). However, quantitative resistance controlled by several genes is considered the most durable against biotic stresses (Miedaner et al., 2020). Quantitative resistance is predominant in maize, with durable resistance to several races of pathogens (Wisser et al., 2006; Yang et al., 2017; Navarro et al., 2021). Therefore, it is pertinent to explore the genetic variation for effective disease control to attain sustainable crop production.
Northern corn leaf blight (NCLB) is a frequently occurring disease in maize in humid temperate and tropical climates (Ceballos et al., 1991). Minute chlorotic flecks generally characterize NCLB initially, and grey-green elliptical lesions form irregular areas of dead tissues at the mature stage (Wise, 2011). Exserohilum turcicum, a filamentous hemibiotrophic fungus, is the causal pathogen for NCLB in maize (Wu et al., 2014). Several studies have characterized the evolution and mode of infection and the physiological impact of E. turcicum in maize (Leonard and Suggs, 1974; Chung et al., 2010; Ohm et al., 2012; Kotze et al., 2019; Silveira et al., 2019). A study by Wu et al., (Wu et al., 2014), identified and characterized several miRNAs in response to E. turcicum in maize. Similarly, another study by Zang et al., (Zang et al., 2020), identified ERF transcriptions factor regulating responses against E. turcicum in maize. Moreover, ERF genes are involved in several critical pathways, such as ethylene, salicylic acid, and jasmonic acid regulating disease resistance (Xu et al., 2007; Xie et al., 2019).
Climate change, cultivation patterns, susceptible genotypes, and strong pathogenicity of E. turcicum are the major reasons for the increased occurrence of NCLB (Xia et al., 2020; Ma et al., 2022). Improving genetic makeup to develop more resistant cultivars is necessary to overcome food security challenges. With the advancement in technology, several methods have been extensively utilized to identify and characterize QTLs (quantitative trait loci) associated with biotic and abiotic phenomena. Moreover, next-generation sequencing technologies have accelerated the conventional breeding for robust identification of key biological regulators associated with the specific trait (He et al., 2020; Nazir et al., 2020; Nazir et al., 2021b). However, bulk segregant analysis (BSA) remains popular for its effectiveness and robustness in identifying key differences between two distinct characters (Karim et al., 2020).
The present study focused on the identification and characterization of major QTLs associated with NCLB in maize by utilizing a segregating population (F2) developed from GML71 (resistant parent) and Gui A10341 (susceptible parent). The genomic DNA from 30 plants of each category resistant and susceptible (identified after inoculation) was pooled for downstream analysis. QTLs with candidate genes related to Corn spot resistance were identified and annotated by combining the phenotype with genotype to provide a genetic basis for NCLB disease in two contrasting parental genotypes using Next-generation sequencing (NGS)-based bulked-segregant analysis (BSA).
Materials and methods
Plant material
Segregating population F2 was developed using two parental lines, GML71 and Gui A10341. GML71 is resistant to NCLB, while Gui A10341 is highly susceptible to NCLB. In spring 2019, two parental lines were planted in the Mingyang base and crossed to obtain F1 and sampled in two replicates to extract DNA during the jointing period. In the autumn of 2019, F1 was planted, and 283 fruit ears were obtained. In the spring of 2020, F2 was planted with a total of 283 individuals, and the disease resistance was investigated after artificial inoculation of E. turcicum (Pass.) LeonardetSuggs. Samples from 30 high-sensitive and 30 resistant lines were collected and bulked for each extreme for resequencing and BSA (bulk segregant analysis).
Pathogenicity tests using colonized sorghum grains as inoculum
White sorghum grains, soaked overnight in a 250 ml flask, were used for inoculum preparation. After 24 h, water was drained, and flasks were covered with cotton and aluminum foils and autoclaved at 121°C. Spores of E. turcicum isolate NGIB16-13 were harvested and adjusted to a concentration of 105 spores ml−1. Flasks containing the sterilized sorghum were aseptically inoculated with 4 ml spore suspension and incubated at room temperature for 5 days. After incubation, the colonized grains were stored in a refrigerator (4°C) and later used for the inoculations.
The whorl of 21-day-old maize plants was inoculated with three colonized sorghum grains and covered with clean nylon bags for 48 h. Control plants were inoculated with sterile, non-inoculated sorghum. Four plants from two pots were evaluated for disease severity using the 1 to 9 (where 1 indicates no occurrence of disease, and nine indicates 100% incidence of disease) scale described earlier (Galiano-Carneiro et al., 2021).
DNA extraction, library construction, and sequencing
Allele frequency estimates in BSA analysis depend on the variation in segregant samples and sequencing technology. The variation due to segregant samples can be minimized by either increasing the number of segregants or bulk size (Magwene et al., 2011). In this study, a total of 60 young leaves of F2 individuals (30 from resistance and susceptible pool each) along with two parental lines were collected, and genomic DNA was extracted using the cetyltrimethylammonium bromide (CTAB) method. The isolated DNA was quantified using a Qubit2.0 Fluorometer (Thermo, CA, United States). Equal amounts of DNA from the resistant and susceptible individuals were mixed to prepare the resistant pool (named D3) and susceptible pool (named D4). The DNA extracted from parental lines was also prepared for library construction, named D1 (GML71) and D2 (Gui A10341). The samples were sonicated to generate ∼350-bp fragments using an M220 instrument (Covaris, Woburn, MA, United States). Then DNA fragments were end-polished, A-tailed, and ligated with the adapter for PCR amplification (Lybaybio, Tianjin, China). Finally, PCR products were purified and analyzed for size distribution by an Agilent2100 Bioanalyzer and quantified using real-time PCR. Libraries, after quality inspection, were loaded onto an Illumina sequencing platform (Illumina, Inc., San Diego, CA, United States) for Hiseq X10 PE150 sequencing.
Data processing and analysis
Sequencing data were aligned to previously published genome B73 using BWA (Li, 2013). Before alignment, raw data were processed for quality control by removing reads with ≥10% unidentified nucleotides, Phred quality <5, and not aligned >10reads. GATK pipeline was used for SNP calling (do et al., 2016). The read-depth information for the SNP index was estimated according to the method of Takagi et al. (Takagi et al., 2013) using a sliding window. The difference in the SNP index of the two pools was calculated as the delta SNP index.
We used some commonly used BSA analyses with multiple testing using SNP-index, Euclidean Distance (ED) (de la Fuente Cantó and Vigouroux, 2022), and G-statistics (Magwene et al., 2011). If there is an apparent major QTL that controls the corresponding trait, the significant effect intervals obtained by each method should not differ much; If there is no apparent major effect site, the results obtained by each analysis method may be different. SNP index (∆SNP) refers to the subtraction of the alternate allele frequency value of the low bulk from the high bulk (∆SNP) (Takagi et al., 2013). G-statistics takes advantage of a log-likelihood statistic LOD between allele frequencies (Zhang et al., 2019), while Hill et al., predicted Euclidean distance (ED) between two vectors defined by the frequencies of the alternate and the reference alleles in the high and low bulks to identify the region of interest (Hill et al., 2013). We estimated the overlapping QTLs from each test for quality results, and only common QTLs were carried out for further analysis.
Based on ∆SNP, G-statistics, and ED, we narrowed down the candidate region associated with NCLB resistance, and to further understand the genes in candidate regions; we conducted GO enrichment analysis on genes (http://www.geneontology.org/).
Candidate gene identification and qRT-PCR based verification
Candidate genes concerning NCLB were further screened from each QTL interval using GO and KEGG enrichment information. Candidate genes with GO terms associated with disease resistance were subjected to qRT-PCR to check their expression pattern in contrasting genotypes (resistant and susceptible). Total RNA was extracted from fresh leaves and roots using TRIzol® Plus RNA Purification Kit (Invitrogen, CA) based on the manufacturer’s instructions. Approximately 1 μg RNA was reversely synthesized into cDNA using the iScriptTM Synthesis Kit (Quanta BioSciences, MD). The qRT-PCR was carried out in an Eppendorf real-time PCR equipment using a 5 μl cDNA template (diluted 1/100), 5 μl primers (2.4 M), and 10 μl SYBR green mixture (Promega, Madison, WI). Histone 3 was used as the internal control, and the relative expression levels of the ORP gene were calculated by the 2−ΔΔCt method (Livak and Schmittgen, 2001).
Results
Genotyping and SNP filtering
To comprehend NCLB regulatory mechanisms in maize, we utilized two advanced lines, GML71 and Gui A10341 (Mo et al., 2019), to develop an F2 segregating population. GML71 is resistant, while Gui A10341 is highly susceptible to NCLB (Figure 1). The segregating population consisted of 283 individuals and was further categorized for both extremes, i.e., resistant and susceptible. The bulked samples from both resistant (D3) and susceptible (D4) groups, along with parental lines (GML71 as D1 and Gui A10341 as D2), were re-sequenced using Hiseq x10 technology. A total of 37.56 Gb of clean data was obtained, with 95.84% of reads showing an average score of Q20 and 89.78% of the reads showing an average score of Q30 (Supplementary Table S1). The GC contents in D1, D2, D3, and D4 were 47.94%, 47.98%, 55.82%, and 53.09%, respectively. The sequenced samples were aligned to the reference genome B73. The alignment details have been provided in Supplementary Table S2.
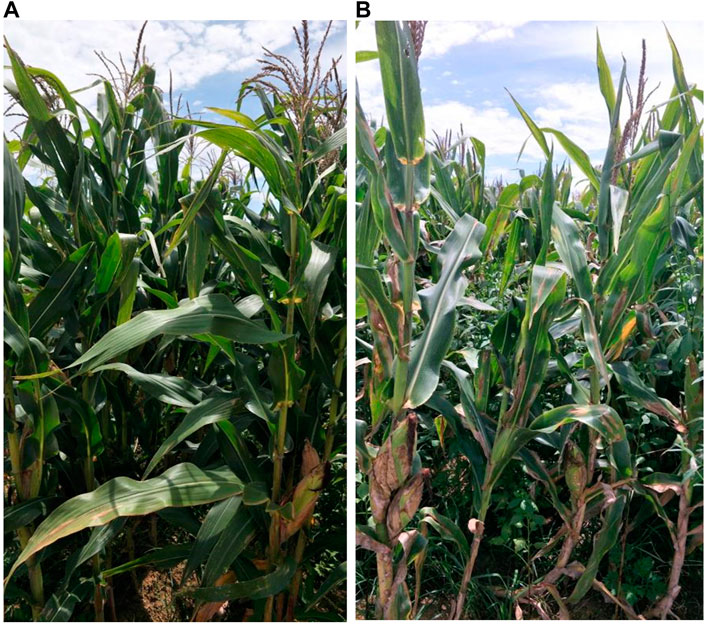
FIGURE 1. Morphological description of parental lines (A) GML71 (Resistant) and (B) Gui A10341 (susceptible).
BSA mapping
To explore and identify the molecular markers associated with NCLB disease resistance in maize, SNP-indices of each locus in D3 and D4 bulks were estimated using quality-filtered SNPs. The high-quality SNPs were classified as having a quality score ≥100 with a read depth ≥10. The average SNP-index in D3 and D4 bulks were estimated using a 2-Mb genomic interval with 10-Kb sliding windows. The Δ (SNP-index) between D3 and D4 bulks was also estimated and plotted for all the chromosomes of the maize genome (Figure 2). Similarly, Fisher’s exact test was also performed for the D3 and D4 bulks at each SNP locus, and the average p-values for SNPs located in each sliding window were calculated and log-transformed (Figure 3). Moreover, Euclidean distance and G statistics were also performed to further verify identified peak signals, and both produced similar QTL detection plots (Figures 3B,C). G-statistics indicate the allelic effect on quantitative traits (de la Fuente Cantó and Vigouroux, 2022).
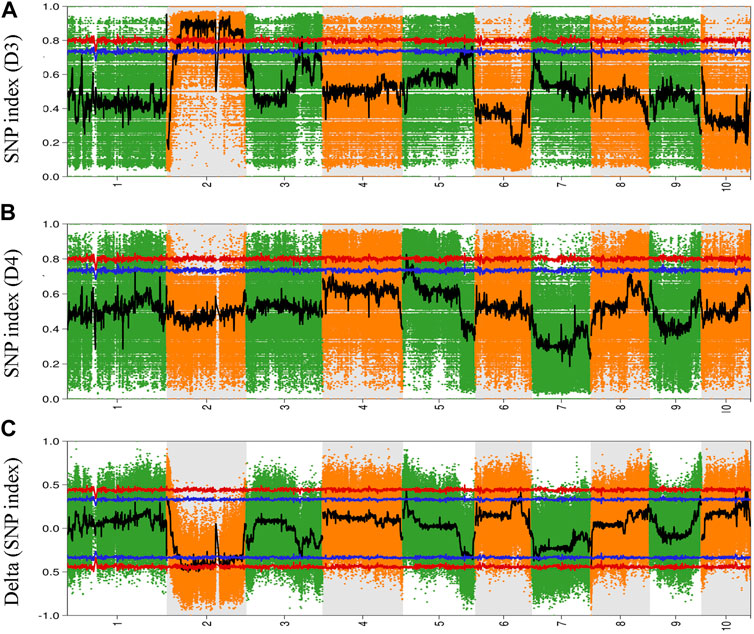
FIGURE 2. Genome-wide distribution of SNP-index in (A) D3 bulk and (B) D4 bulk (C) Delta (SNP-index) between De bulk and D4 bulk. The abscissa is the chromosome name, the colored dots represent the calculated SNP-index (or ΔSNP-index value, and the black line is the fitted SNP-index or ΔSNP-index value. The red line represents the threshold line with a confidence level of 0.99; the blue line represents the threshold line with a confidence level of 0.95.
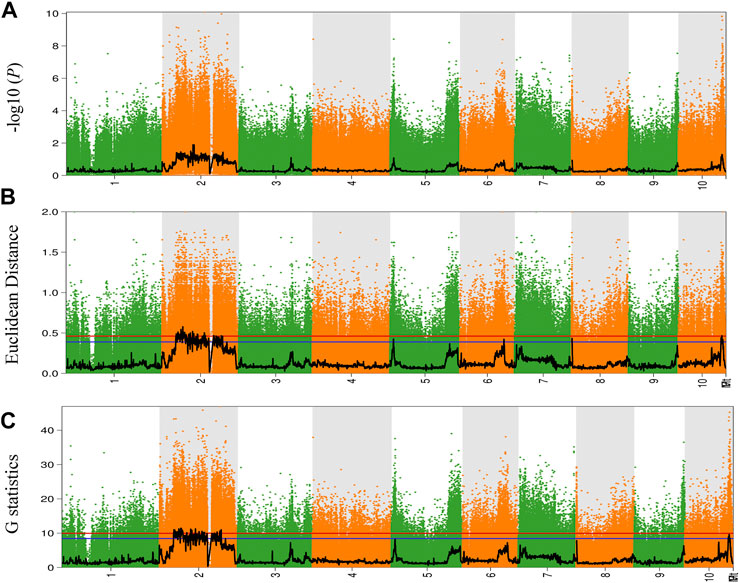
FIGURE 3. The results of the (A) -log10(P), (B) Euclidean distance (ED), and (C) G-statistics algorithm. The abscissa is the chromosome name; the colored dots represent the calculated SNP-index index (or ΔSNP-index value, and the black line is the fitted SNP-index or ΔSNP-index value. The red line represents the threshold line with a confidence level of 0.99; the blue line represents the threshold line with a confidence level of 0.95.
Based on the Δ (SNP-index) statistics, we identified multiple peaks on Chr 2, Chr 5, Chr 6, Chr 9, and Chr 11. However, the most significant variation was observed on Chr 2 (Figure 2C). Therefore, we considered regions on Chr 2 as a hotspot for NCLB control in maize. To identify the significant QTLs and genes present in the QTLs, we set criteria of 99% confidence interval and identified a total of eight QTLs associated with D3 bulk on Chr 1, 2, and 3 (Supplementary Table S3). We named these QTLs as Q-Chr-number. For instance, Q2-1 denotes the first QTL identified on Chr 2. Similarly, we identified two QTLs on Chr five associated with D4 bulk (Supplementary Table S4). Q2-3, Q2-4, and Q2-5 were identified with interval size greater than 24 Mb, while the remaining QTLs were identified with less than 5.5 Mb intervals. Detailed SNPs statistics have been provided in Supplementary Tables S1, S2. Q1-1 spanned a 2.012 Mb region on chromosome 1 starting from 3,05,020,001 to 307,041,717. The region contained 48 genes. Five QTLs Q2-1, Q2-2, Q2-3, Q2-4, and Q2-5 were 5.45Mb, 3.09Mb, 24.01Mb, 85.19Mb, and 73.01Mb, respectively (Table 1).
Candidate genes and enrichment analysis
We further characterized each QTL and identified genes residing in the QTL regions. A total of 48, 131, 74, 405, 716, 1844, 19, 36, 82, and 69 genes were identified in Q1-1, Q2-1, Q2-2, Q2-3, Q2-4, Q2-5, Q3-1, Q3-2, Q5-1, and Q5-2, respectively. The identified genes were further subjected to GO-term ontology and KEGG pathways analysis (Supplementary Figures S1, S2). GO terms, including Signal transduction, hormone regulation, and pathogenesis, were enriched in D3 bulk (Supplementary Figure S1), while phosphorylation, phosphorus metabolic process, protein phosphorylation, and signal transduction were enriched in D4 bulk (Supplementary Figure S2). KEGG pathways enriched in D3 bulk were identified as MAPK signaling, sulfur metabolism, ABC transporters, and phenylalanine metabolism, while MAPK signaling, phosphonate metabolism, signal transduction, and sugar metabolism were enriched in D4 bulk. We identified 99,481 and 3,924 significant SNPs in D3 and D4 bulk, respectively. The identified SNPs were further screened for non-synonymous SNPs as candidate sites. 250 non-synonymous and frameshift SNPs were identified in D3 bulk, and 15 non-synonymous SNPs were identified in D4 bulk.
After SNP screening, we identified 6, 6, 30, 52, 151, 2, 4, 11, and 4 non-synonymous SNPs in Q2-1, Q2-2, Q2-3, Q2-4, Q2-5, Q3-1, Q3-2, Q5-1, and Q5-2, respectively. ZmWAK-RLK1 gene has been previously characterized for involvement in NCLB in maize (Hurni et al., 2015; Yang et al., 2017; Yang et al., 2019b; Yang et al., 2021a). The annotation information regarding ZmWAK-RLK1 suggested involvement in several pathways, including protein phosphorylation, cytoplasmic vesicle, protein serine/threonine kinase activity, and ATP binding pathway in this study (Li et al., 2020). Therefore, we further screened genes associated with non-synonymous SNPs using annotation information and identified 27 genes on chromosomes 2 and 5 (Table 2). Q2-5 contains 14 annotated genes associated with pathways related to protein phosphorylation, cytoplasmic vesicle, protein serine/threonine kinase activity, and ATP binding pathway. Q2-5 genes associated with disease resistance encode cyclin11, receptor-like kinase, Putative leucyl-tRNA synthetase, Protein kinase superfamily protein, leucine-rich repeat receptor-like serine, Protein kinase superfamily protein, LRR receptor-like serine/threonine-protein kinase, Serine/threonine-protein kinase UCNL, ATP-dependent DNA helicase, Probable inactive receptor kinase, CHROMATIN REMODELING 5, ATP-dependent DNA helicase chloroplastic, Wall-associated kinase 2-like protein, and ATP-dependent DNA helicase. Mitogen-activated protein kinase 9, Mitogen-activated protein kinase 9, Atypical receptor-like kinase MARK, and DEAD-box ATP-dependent RNA helicase 21 were identified in Q5-1. Moreover, QTLs Q2-4, Q2-5, and Q5-1 were identified with multiple genes associated with disease resistance pathways; therefore, we consider these QTLs as candidates for further functional verification. Further molecular insight into the functions of genes associated with candidate QTLs can provide a comprehensive overview of quantitative disease resistance against NCLB in maize.
Expression profile of candidate genes
To understand the regulation patterns of candidate genes in two contrasting parental genotypes (GML71 is resistant, while Gui A10341), we performed qRT-PCR for all the candidate genes. The primers for each candidate gene are listed in Supplementary Table S5. The expression profile of each parent was considerably different when the expression was compared after the inoculation of NCLB. Among 27 candidate genes, 14 depicted up-regulated expression patterns under disease inoculation in tolerant genotype Gui A10341, while six showed down-regulated expression patterns (Figure 4). Zm00001d003209, Zm00001d003266, Zm00001d003430, Zm00001d004592, Zm00001d004635, Zm00001d005829, Zm00001d006017, Zm00001d006695, Zm00001d006917, Zm00001d007417, Zm00001d013418, Zm00001d013423, and Zm00001d013430 were among the 14 up-regulated genes in resistant genotype. Zm00001d013453In comparison, susceptible genotype GML71 was observed with up-regulated expression pattern of only four genes (Zm00001d005986, Zm00001d006695, Zm00001d013423, Zm00001d013453). The differential expression pattern after inoculation is highly suggestive that these genes are excellent candidates for further function verification and use in breeding programs for NCLB resistance.
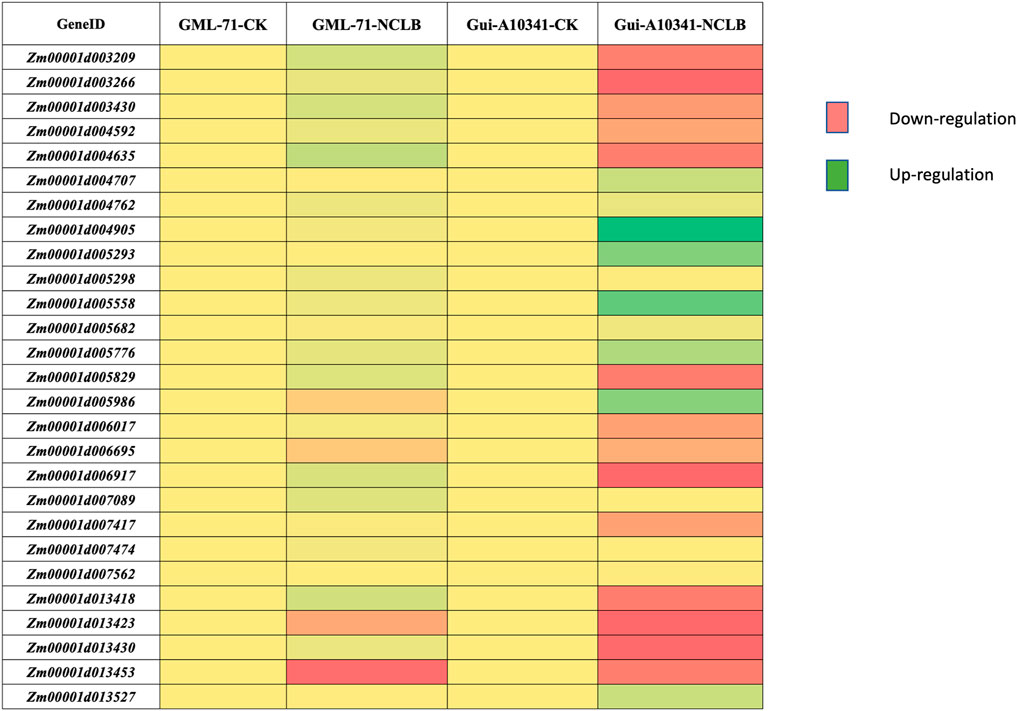
FIGURE 4. Expression profile of candidate genes in two contrasting parental genotypes, GML-71 and Gui A10341. The expression pattern was estimated before (check = CK) and after NCLB inoculation (NCLB) in both genotypes.
Discussion
NCLB, caused by Exserohilum turcicum, is a frequently occurring foliar disease of maize, threatening more than 50% yield losses in tropic and sub-tropic environments (Blandino et al., 2012). Resistant cultivars are the effective control for NCLB (Technow et al., 2013). Multiple studies have depicted chemical control as an effective measure for NCLB control (Blandino et al., 2012; Zhang et al., 2013; Camera et al., 2019; De Rossi et al., 2022). However, chemical and biological control increase the cost of production along with environmental hazards (Alabouvette et al., 2006; Fry, 2012; Hirooka and Ishii, 2013). Recent advances in omics with high-density molecular markers have enabled plant breeders to successfully understand and manipulate molecular markers to the genetic architecture of modern cultivars (Collard and Mackill, 2008; Nazir et al., 2021b; Sarfraz et al., 2021). The present study successfully utilized bulk segregant analysis to identify molecular markers associated with NCLB.
Previously reports suggested a complex genetic architecture of NCLB with multiple QTLs spanning multiple chromosomes (Wisser et al., 2006; Poland et al., 2011; Van Inghelandt et al., 2012). Poland et. al., identified 29 QTLs related to NCLB throughout the genome (Poland et al., 2011). However, each QTL depicted a minor effect in contribution towards NCLB resistance. Recently, with the advancement of technology, the BSA method supported by genomic and transcriptomic data has been proven to be a robust and efficient platform identification of molecular markers and associated genes related to a particular trait in several crops, including rice, maize, brassica, wheat, and cotton (Mansfeld and Grumet, 2018; Gyawali et al., 2019; Mu et al., 2019; Yang et al., 2021b; Wang et al., 2021). In the present study we did not perform a phenotypic evaluation on the complete F2 population. This would have allowed a chi-square analysis to predict the number of QTLs responsible for the variation of disease score in the population. We instead utilized resistance and susceptible bulks as plant materials and identified 10 QTLs potentially associated with the NCLB resistance mechanism in maize. These QTLs were identified on Chr 1, Chr 2, Chr 3, and Chr 5. A similar work by Li et. al., (Li et al., 2020), reported 502 non-synonymous SNPs in six QTLs related to NCLB on Chr eight and identified seven putative candidate genes involved in NCLB control. We identified 27 candidate genes in seven QTLs combined with SNP-index and annotation information.
Both qualitative and quantitative inheritance patterns of NCLB resistance have been reported in maize (Galiano-Carneiro and Miedaner, 2017). Identification and utilization of NCLB resistance controlled by a single gene, such as Ht1, Ht2, and Ht3, is ineffective for long-term resistance. Several physiological phenomena have been confirmed associated with the functions of these genes, including inhibition of chlorotic spots, epiphytotic control, and signal transduction (Welz and Geiger, 2000; Hurni et al., 2015; Wang et al., 2018). Moreover, several biological pathways associated with disease resistance in maize have been identified, such as protein phosphorylation, cytoplasmic vesicle, protein serine/threonine kinase activity, and ATP binding pathway (Li et al., 2020). Quantitative resistance with the cumulative effect of several genes is necessary for durable resistance against NCLB (Li et al., 2020). Three genes, AATP1 (AAA-ATPase ASD mitochondrial), At4g24790 (STICHEL-like 2), EMB2768 (Tyrosine--tRNA ligase chloroplastic), were identified as candidate genes in Q1-1, Q1-2, and Q3-2, respectively. A previous study by Liu et al., (Liu et al., 2020), reported activation of OsAAA-ATPase1 during blast infection in rice, suggesting a key role of OsAAA-ATPase1 salicylic acid-mediated defense responses against fungus M. oryzae. Moreover, ATPase genes are known for their active role in hypersensitive response (HR) toward plant pathogens (Dmitriev et al., 2017; Weiß and Winkelmann, 2017; Waititu et al., 2021). Nitric oxide (NO) regulates developmental and stress-mediated responses in plants by activating NO-signaling pathways (León, 2022) associated with Tyrosine-protein. Similarly, STICHEL-like 2 has been reported to be involved in response to biotic and abiotic stress responses (Hu et al., 2015; Wang et al., 2015; Hejri et al., 2021).
We identified four candidate genes in the QTL Q2-4, including BI O 3-BIO1, ZAR1, SECA2, ABCG25, and LECRK54. BI O 3-BIO1 regulates biotin synthesis, an essential cofactor for enzymes catalyzing carboxylation, decarboxylation, and transcarboxylation reactions (Wang et al., 2020). Functional characterization of bio2 mutant in Arabidopsis thaliana suggested biotin decreased ROS (reactive oxygen species) accumulation under stress conditions (Wang et al., 2020). ZAR1-RKS1 complex, through direct interaction with RKS1, triggers ZAR1 activation and disease resistance in A. thaliana (Wang et al., 2019a). Several reports suggest a resistance response mediated by ZAR1 (Lewis et al., 2010; Wang et al., 2019b; Hu et al., 2020; Chen et al., 2021). For instance, Wang et al., (Wang et al., 2019b), characterized the ZAR1-RKS1 complex, which causes terminal cell death and mediates rapid stress-induced transcriptional activation of defense genes in plants.
Characterization of Q2-5 identified 12 genes associated with disease resistance response. These genes included At4g37250, At1g09620, PBL28, XA21, STY17, RGI3, UCNL, At5g10020, CHR5, At3g02060, WAK3, and ESD4. While, MKK7, MKK9, RLK902, Os03g0708600, and CPK9 were identified as candidate genes from two QTLs on Chr 5. The annotation information suggested the involvement of these genes in disease resistance responses. Numerous QTL studies have reported QTLs associated with NCLB in maize. In the present study, the resolution was significantly improved by using high-density SNP markers, and the improved resolution can facilitate a comparison of our results with disease-associated QTL and genes reported previously. For example, Poland et al., (Poland et al., 2011), identified four QTLs on Chr 5 with one putative candidate gene GRMZM2G024612 associated with NCLB resistance. Our results comprehend quantitative resistance response towards NCLB by identifying several QTLs spanning three chromosomes with multiple genes associated with the NCLB resistance mechanism. Another study by Chen et al., (Chen et al., 2016), identified a stable QTL on chromosome 8 with one putative candidate gene. Xie et al., (Xia et al., 2020), reported five QTLs associated with NCLB resistance on chromosomes 1, 2, 4, 8, and 9. Another genomic region important for NCLB resistance was identified in chromosome 8, where two associated genes (Ht2 and Htn1) were identified (Zaitlin et al., 1992; Hurni et al., 2015).
We identified ten QTLs on Chr 1, Chr 2, Chr 3, and Chr 5 with a 99% confidence interval associated with NCLB resistance. Moreover, we screened 265 non-synonymous SNP-containing genes and narrowed them down to 27 candidate genes with differential expression patterns in NCLB contrasting genotypes (susceptible and resistant). Our study provides a genetic basis for quantitative disease resistance against NCLB in maize. Further functional characterization of candidate genes based on the provided information can yield significant insights into the NCLB resistance mechanisms in maize.
Data availability statement
The raw sequencing data can be found in NCBI SRA under the project number PRJNA875291 (https://www.ncbi.nlm.nih.gov/bioproject/?term=PRJNA875291).
Author contributions
Conceptualization, RZ, RM, CZ, XW, MY, JQ, HT and KH; Data curation, RZ and AH; Formal analysis, RZ, AH and RM; Funding acquisition, HT and KH; Investigation, AH, RM, CZ and XW; Methodology, XW, MY, JQ, HT and KH; Project administration, RM, MY, JQ and HT; Resources, CZ and JQ; Software, CZ; Supervision, XW; Validation, MY; Visualization, XW, MY and JQ; Writing—original draft, HT and KH; Writing—review and editing, KH.
Funding
This work was supported by The Science and Technology Development Fund of Guangxi Academy of Agricultural Sciences “Research on QTL mapping of genes related to resistance to northern corn leaf blight in maize” (Gui Nongke 2019ZX09), “Dynamic Changes of Pigment Accumulation During Grain Development of Black Waxy Maize”, Fundamental Research Project of Guangxi Academy of Agricultural Sciences (Gui Nongke 2020YM90), Guangxi key research and development plan “Demonstration and promotion of characteristic fresh corn varieties and research and development of supporting storage and processing technology” (Guike AB21196052), National key R&D project “Cultivation of new varieties of tropical and subtropical stress-resistant high-yield and barren-tolerant maize” (2018YFD0100105), National key research and development project “Guangxi maize germplasm improvement and strong dominant hybrids innovation (2016YFD01011206-6).
Conflict of interest
The authors declare that the research was conducted in the absence of any commercial or financial relationships that could be construed as a potential conflict of interest.
Publisher’s note
All claims expressed in this article are solely those of the authors and do not necessarily represent those of their affiliated organizations, or those of the publisher, the editors and the reviewers. Any product that may be evaluated in this article, or claim that may be made by its manufacturer, is not guaranteed or endorsed by the publisher.
Supplementary material
The Supplementary Material for this article can be found online at: https://www.frontiersin.org/articles/10.3389/fgene.2022.1038948/full#supplementary-material
References
Alabouvette, C., Olivain, C., and Steinberg, C. (2006). Biological control of plant diseases: The European situation. Eur. J. Plant Pathol. 114, 329–341. doi:10.1007/s10658-005-0233-0
Blandino, M., Galeazzi, M., Savoia, W., and Reyneri, A. (2012). Timing of azoxystrobin+ propiconazole application on maize to control northern corn leaf blight and maximize grain yield. Field Crops Res. 139, 20–29. doi:10.1016/j.fcr.2012.09.014
Camera, J. N., Forcelini, C. A., Koefender, J., Golle, D. P., Schoffel, A., and Deuner, C. C. (2019). Reaction of maize hybrids to Northern corn leaf blight and common rust, and chemical control of Northern corn leaf blight. Arquivos do Instituto Biológico, 86. doi:10.1590/1808-1657000082018
Ceballos, H., Deutsch, J., and Gutierrez, H. (1991). Recurrent selection for resistance to Exserohilum turcicum in eight subtropical maize populations. Crop Sci. 31, 964–971. doi:10.2135/cropsci1991.0011183x003100040025x
Chen, G., Wang, X., Long, S., Jaqueth, J., Li, B., Yan, J., et al. (2016). Mapping of QTL conferring resistance to northern corn leaf blight using high-density SNPs in maize. Mol. Breed. 36, 4–9. doi:10.1007/s11032-015-0421-3
Chen, J., Li, M., Liu, L., Chen, G., and Fu, Z. Q. (2021). ZAR1 resistosome and helper NLRs: Bringing in calcium and inducing cell death. Mol. Plant 14, 1234–1236. doi:10.1016/j.molp.2021.06.026
Chung, C.-L., Longfellow, J. M., Walsh, E. K., Kerdieh, Z., Van Esbroeck, G., Balint-Kurti, P., et al. (2010). Resistance loci affecting distinct stages of fungal pathogenesis: Use of introgression lines for QTL mapping and characterization in the maize-setosphaeria turcica pathosystem. BMC Plant Biol. 10, 103. doi:10.1186/1471-2229-10-103
Cole, M. B., Augustin, M. A., Robertson, M. J., and Manners, J. M. (2018). The science of food security. NPJ Sci. Food 2, 14–18. doi:10.1038/s41538-018-0021-9
Collard, B. C., and Mackill, D. J. (2008). Marker-assisted selection: An approach for precision plant breeding in the twenty-first century. Philos. Trans. R. Soc. Lond. B Biol. Sci. 363, 557–572. doi:10.1098/rstb.2007.2170
Darino, M. A., Rochi, L., Lia, V. V., Kreff, E. D., Pergolesi, M. F., Ingala, L. R., et al. (2016). Virulence characterization and identification of maize lines resistant to Puccinia sorghi Schwein. Present in the Argentine corn belt region. Plant Dis. 100, 770–776. doi:10.1094/PDIS-06-15-0639-RE
de la Fuente Cantó, C., and Vigouroux, Y. (2022). Evaluation of nine statistics to identify QTLs in bulk segregant analysis using next generation sequencing approaches. BMC genomics 23, 490–512. doi:10.1186/s12864-022-08718-y
De Rossi, R., Guerra, F., Plazas, M., Vuletic, E., Brücher, E., Guerra, G., et al. (2022). Crop damage, economic losses, and the economic damage threshold for northern corn leaf blight. Crop Prot. 154, 105901. doi:10.1016/j.cropro.2021.105901
Dmitriev, A. A., Krasnov, G. S., Rozhmina, T. A., Novakovskiy, R. O., Snezhkina, A. V., Fedorova, M. S., et al. (2017). Differential gene expression in response to Fusarium oxysporum infection in resistant and susceptible genotypes of flax (Linum usitatissimum L.). BMC Plant Biol. 17, 253–340. doi:10.1186/s12870-017-1192-2
do, Valle, Í, F., Giampieri, E., Simonetti, G., Padella, A., Manfrini, M., et al. (2016). Optimized pipeline of MuTect and GATK tools to improve the detection of somatic single nucleotide polymorphisms in whole-exome sequencing data. BMC Bioinforma. 17, 27–35. doi:10.1186/s12859-016-1190-7
Galiano-Carneiro, A. L., Kessel, B., Presterl, T., and Miedaner, T. (2021). Intercontinental trials reveal stable QTL for Northern corn leaf blight resistance in Europe and in Brazil. Theor. Appl. Genet. 134, 63–79. doi:10.1007/s00122-020-03682-1
Galiano-Carneiro, A. L., and Miedaner, T. (2017). Genetics of resistance and pathogenicity in the maize/Setosphaeria turcica pathosystem and implications for breeding. Front. Plant Sci. 8, 1490. doi:10.3389/fpls.2017.01490
Gyawali, A., Shrestha, V., Guill, K. E., Flint-Garcia, S., and Beissinger, T. M. (2019). Single-plant GWAS coupled with bulk segregant analysis allows rapid identification and corroboration of plant-height candidate SNPs. BMC Plant Biol. 19, 412–415. doi:10.1186/s12870-019-2000-y
He, S., Wang, P., Zhang, Y.-M., Dai, P., Nazir, M. F., Jia, Y., et al. (2020). Introgression leads to genomic divergence and responsible for important traits in upland cotton. Front. Plant Sci. 11, 929. doi:10.3389/fpls.2020.00929
Hejri, S., Salimi, A., Malboobi, M. A., and Fatehi, F. (2021). Comparative proteome analyses of rhizomania resistant transgenic sugar beets based on RNA silencing mechanism. Gm. Crops Food 12, 419–433. doi:10.1080/21645698.2021.1954467
Hill, J. T., Demarest, B. L., Bisgrove, B. W., Gorsi, B., Su, Y.-C., and Yost, H. J. (2013). Mmappr: Mutation mapping analysis pipeline for pooled RNA-seq. Genome Res. 23, 687–697. doi:10.1101/gr.146936.112
Hirooka, T., and Ishii, H. (2013). Chemical control of plant diseases. J. Gen. Plant Pathol. 79, 390–401. doi:10.1007/s10327-013-0470-6
Hu, M., Qi, J., Bi, G., and Zhou, J.-M. (2020). Bacterial effectors induce oligomerization of immune receptor ZAR1 in vivo. Mol. Plant 13, 793–801. doi:10.1016/j.molp.2020.03.004
Hu, X., Wu, L., Zhao, F., Zhang, D., Li, N., Zhu, G., et al. (2015). Phosphoproteomic analysis of the response of maize leaves to drought, heat and their combination stress. Front. Plant Sci. 6, 298. doi:10.3389/fpls.2015.00298
Hurni, S., Scheuermann, D., Krattinger, S. G., Kessel, B., Wicker, T., Herren, G., et al. (2015). The maize disease resistance gene Htn1 against northern corn leaf blight encodes a wall-associated receptor-like kinase. Proc. Natl. Acad. Sci. U. S. A. 112, 8780–8785. doi:10.1073/pnas.1502522112
Ji, W., He, H., Zhao, S., Yan, S., and Fu, D. (2010). Identification of physiological races of Setosphaeria turcica in maize-producing region of Heilongjiang. J. Maize Sci. 18, 128–134.
Johnson, R. (1981). Durable resistance: Definition of, genetic control, and attainment in plant breeding. Phytopathology 71, 567–568. doi:10.1094/phyto-71-567
Karim, M., Dakouri, A., Zhang, Y., Chen, Q., Peng, G., Strelkov, S. E., et al. (2020). Two clubroot-resistance genes, Rcr3 and Rcr9wa, mapped in Brassica rapa using bulk segregant RNA sequencing. Int. J. Mol. Sci. 21, 5033. doi:10.3390/ijms21145033
Kotze, R., Van der Merwe, C., Crampton, B., and Kritzinger, Q. (2019). A histological assessment of the infection strategy of Exserohilum turcicum in maize. Plant Pathol. 68, 504–512. doi:10.1111/ppa.12961
León, J. (2022). Protein tyrosine nitration in plant nitric oxide signaling. Front. Plant Sci. 13, 859374. doi:10.3389/fpls.2022.859374
Leonard, K., and Suggs, E. G. (1974). Setosphaeria prolata, the ascigerous state of Exserohilum prolatum. Mycologia 66, 281–297. doi:10.2307/3758363
Lewis, J. D., Wu, R., Guttman, D. S., and Desveaux, D. (2010). Allele-specific virulence attenuation of the Pseudomonas syringae HopZ1a type III effector via the Arabidopsis ZAR1 resistance protein. PLoS Genet. 6, 1000894. doi:10.1371/journal.pgen.1000894
Li, C., Ling, F., Su, G., Sun, W., Liu, H., Su, Y., et al. (2020). Location and mapping of the NCLB resistance genes in maize by bulked segregant analysis (BSA) using whole genome re-sequencing. Mol. Breed. 40, 92–12. doi:10.1007/s11032-020-01171-3
Li, H. Aligning sequence reads, clone sequences and assembly contigs with BWA-MEM. https://arxiv.org/abs/1303.3997 2013.
Li, P., Cao, W., Fang, H., Xu, S., Yin, S., Zhang, Y., et al. (2017). Transcriptomic profiling of the maize (Zea mays L.) leaf response to abiotic stresses at the seedling stage. Front. Plant Sci. 8, 290. doi:10.3389/fpls.2017.00290
Liu, X., Inoue, H., Tang, X., Tan, Y., Xu, X., Wang, C., et al. (2020). Rice OsAAA-ATPase1 is induced during blast infection in a salicylic acid-dependent manner, and promotes blast fungus resistance. Int. J. Mol. Sci. 21, 1443. doi:10.3390/ijms21041443
Livak, K. J., and Schmittgen, T. D. (2001). Analysis of relative gene expression data using real-time quantitative PCR and the 2(-Delta Delta C(T)) Method. methods 25, 402–408. doi:10.1006/meth.2001.1262
Ma, Z., Hui, H., Huang, Y., Yao, Y., Sun, Y., Liu, B., et al. (2022). Evaluation of maize hybrids for identifying resistance to northern corn leaf blight in northeast China. Plant Dis. 106, 1003–1008. doi:10.1094/PDIS-09-21-1914-RE
Magwene, P. M., Willis, J. H., and Kelly, J. K. (2011). The statistics of bulk segregant analysis using next generation sequencing. PLoS Comput. Biol. 7, 1002255. doi:10.1371/journal.pcbi.1002255
Mansfeld, B. N., and Grumet, R. Q. T. Lseqr (2018). QTLseqr: An R package for bulk segregant analysis with next-generation sequencing. Plant Genome 11, 180006. doi:10.3835/plantgenome2018.01.0006
Miedaner, T., Boeven, A. L. G.-C., Gaikpa, D. S., Kistner, M. B., and Grote, C. P. (2020). Genomics-assisted breeding for quantitative disease resistances in small-grain cereals and maize. Int. J. Mol. Sci. 21, 9717. doi:10.3390/ijms21249717
Mo, R., Huang, K., Huang, A., Wei, X., He, N., Zou, C., et al. (2019). Analysis on combining ability of main agronomic traits of 20 low nitrogen tolerance maize inbred lines from CIMMYT. Southwest China J. Agric. Sci. 32, 2732–2739.
Mu, J., Huang, S., Liu, S., Zeng, Q., Dai, M., Wang, Q., et al. (2019). Genetic architecture of wheat stripe rust resistance revealed by combining QTL mapping using SNP-based genetic maps and bulked segregant analysis. Theor. Appl. Genet. 132, 443–455. doi:10.1007/s00122-018-3231-2
Navarro, B. L., Ramos Romero, L., Kistner, M. B., Iglesias, J., and Von Tiedemann, A. (2021). Assessment of physiological races of Exserohilum turcicum isolates from maize in Argentina and Brazil. Trop. plant Pathol. 46, 371–380. doi:10.1007/s40858-020-00417-x
Nazir, M. F., He, S., Ahmed, H., Sarfraz, Z., Jia, Y., Li, H., et al. (2021). Genomic insight into the divergence and adaptive potential of a forgotten landrace G. hirsutum L. purpurascens. J. Genet. Genomics 48, 473–484. doi:10.1016/j.jgg.2021.04.009
Nazir, M. F., Jia, Y., Ahmed, H., He, S., Iqbal, M. S., Sarfraz, Z., et al. (2020). Genomic insight into differentiation and selection sweeps in the improvement of upland cotton. Plants 9, 711. doi:10.3390/plants9060711
Nazir, M. F., Sarfraz, Z., Mangi, N., Nawaz Shah, M. K., Mahmood, T., Mahmood, T., et al. (2021). Post-anthesis mobilization of stem assimilates in wheat under induced stress. Sustainability 13, 5940. doi:10.3390/su13115940
Ohm, R. A., Feau, N., Henrissat, B., Schoch, C. L., Horwitz, B. A., Barry, K. W., et al. (2012). Diverse lifestyles and strategies of plant pathogenesis encoded in the genomes of eighteen Dothideomycetes fungi. PLoS Pathog. 8, 1003037. doi:10.1371/journal.ppat.1003037
Onyekachi, O. G., Boniface, O. O., Gemlack, N. F., and Nicholas, N. (2019). The effect of climate change on abiotic plant stress: A review. Abiotic biotic stress plants 17. doi:10.5772/intechopen.82681
Parlevliet, J. E. (2002). Durability of resistance against fungal, bacterial and viral pathogens; present situation. Euphytica 124, 147–156. doi:10.1023/a:1015601731446
Poland, J. A., Bradbury, P. J., Buckler, E. S., and Nelson, R. J. (2011). Genome-wide nested association mapping of quantitative resistance to northern leaf blight in maize. Proc. Natl. Acad. Sci. U. S. A. 108, 6893–6898. doi:10.1073/pnas.1010894108
Romero, L. R. (2016). Occurrence and importance of foliar diseases on maize (Zea mays L.) in Central Europe. Ph. D. thesis. Georg-August-Universität Göttingen. Göttingen, Germany,
Sarfraz, Z., Iqbal, M. S., Geng, X., Iqbal, M. S., Nazir, M. F., Ahmed, H., et al. (2021). GWAS mediated elucidation of heterosis for metric traits in cotton (Gossypium hirsutum L.) across multiple environments. Front. Plant Sci. 12, 565552. doi:10.3389/fpls.2021.565552
Silveira, P., Milagres, P., Corrêa, E., Aucique-Pérez, C., Wordell Filho, J., and Rodrigues, F. (2019). Changes in leaf gas exchange, chlorophyll a fluorescence, and antioxidants in maize leaves infected by Exserohilum turcicum. Biol. plant. 63, 643–653. doi:10.32615/bp.2019.130
Singh, R. P., Singh, P. K., Rutkoski, J., Hodson, D. P., He, X., Jørgensen, L. N., et al. (2016). Disease impact on wheat yield potential and prospects of genetic control. Annu. Rev. Phytopathol. 54, 303–322. doi:10.1146/annurev-phyto-080615-095835
Stevens, T., and Madani, K. (2016). Future climate impacts on maize farming and food security in Malawi. Sci. Rep. 6, 36241–36314. doi:10.1038/srep36241
Takagi, H., Abe, A., Yoshida, K., Kosugi, S., Natsume, S., Mitsuoka, C., et al. (2013). QTL-seq: Rapid mapping of quantitative trait loci in rice by whole genome resequencing of DNA from two bulked populations. Plant J. 74, 174–183. doi:10.1111/tpj.12105
Technow, F., Bürger, A., and Melchinger, A. E. (2013). Genomic prediction of northern corn leaf blight resistance in maize with combined or separated training sets for heterotic groups. G3 3, 197–203. doi:10.1534/g3.112.004630
Tilman, D., Balzer, C., Hill, J., and Befort, B. L. (2011). Global food demand and the sustainable intensification of agriculture. Proc. Natl. Acad. Sci. U. S. A. 108, 20260–20264. doi:10.1073/pnas.1116437108
Van Inghelandt, D., Melchinger, A. E., Martinant, J.-P., and Stich, B. (2012). Genome-wide association mapping of flowering time and northern corn leaf blight (Setosphaeria turcica) resistance in a vast commercial maize germplasm set. BMC Plant Biol. 12, 56–15. doi:10.1186/1471-2229-12-56
Vaughan, M. M., Block, A., Christensen, S. A., Allen, L. H., and Schmelz, E. A. (2018). The effects of climate change associated abiotic stresses on maize phytochemical defenses. Phytochem. Rev. 17, 37–49. doi:10.1007/s11101-017-9508-2
Venter, M. (2007). Synthetic promoters: Genetic control through cis engineering. Trends Plant Sci. 12, 118–124. doi:10.1016/j.tplants.2007.01.002
Waititu, J. K., Zhang, X., Chen, T., Zhang, C., Zhao, Y., and Wang, H. (2021). Transcriptome analysis of tolerant and susceptible maize genotypes reveals novel insights about the molecular mechanisms underlying drought responses in leaves. Int. J. Mol. Sci. 22, 6980. doi:10.3390/ijms22136980
Wang, H., Yan, H., Du, H., Chao, M., Gao, Z., and Yu, D. (2015). Mapping quantitative trait loci associated with soybean resistance to common cutworm and soybean compensatory growth after defoliation using SNP marker-based genome-wide association analysis. Mol. Breed. 35, 168–215. doi:10.1007/s11032-015-0360-z
Wang, J., Hu, M., Wang, J., Qi, J., Han, Z., Wang, G., et al. (2019). Reconstitution and structure of a plant NLR resistosome conferring immunity. Science 364, 5870. doi:10.1126/science.aav5870
Wang, J., Wang, J., Hu, M., Wu, S., Qi, J., Wang, G., et al. (2019). Ligand-triggered allosteric ADP release primes a plant NLR complex. Science 364, 5868. doi:10.1126/science.aav5868
Wang, J., Xu, Z., Yang, J., Lu, X., Zhou, Z., Zhang, C., et al. (2018). qNCLB7. 02, a novel QTL for resistance to Northern corn leaf blight in maize. Mol. Breed. 38, 54–12. doi:10.1007/s11032-017-0770-1
Wang, Y., Wang, M., Ye, X., Liu, H., Takano, T., Tsugama, D., et al. (2020). Biotin plays an important role in Arabidopsis thaliana seedlings under carbonate stress. Plant Sci. 300, 110639. doi:10.1016/j.plantsci.2020.110639
Wang, Z., Yu, A., Li, F., Xu, W., Han, B., Cheng, X., et al. (2021). Bulked segregant analysis reveals candidate genes responsible for dwarf formation in woody oilseed crop castor bean. Sci. Rep. 11, 6277–6315. doi:10.1038/s41598-021-85644-1
Weiß, S., and Winkelmann, T. (2017). Transcriptome profiling in leaves representing aboveground parts of apple replant disease affected Malus domestica ‘M26’plants. Sci. Hortic. 222, 111–125. doi:10.1016/j.scienta.2017.05.012
Welz, H., and Geiger, H. (2000). Genes for resistance to northern corn leaf blight in diverse maize populations. Plant Breed. 119, 1–14. doi:10.1046/j.1439-0523.2000.00462.x
Wise, K. (2011). Diseases of corn: Northern corn leaf blight. Purdue University Extension Publication. Purdue University. West Lafayette, IN, USA,
Wisser, R. J., Balint-Kurti, P. J., and Nelson, R. J. (2006). The genetic architecture of disease resistance in maize: A synthesis of published studies. Phytopathology 96, 120–129. doi:10.1094/PHYTO-96-0120
Wu, F., Shu, J., and Jin, W. (2014). Identification and validation of miRNAs associated with the resistance of maize (Zea mays L.) to Exserohilum turcicum. PloS one 9, 87251. doi:10.1371/journal.pone.0087251
Xia, H., Gao, W., Qu, J., Dai, L., Gao, Y., Lu, S., et al. (2020). Genetic mapping of northern corn leaf blight-resistant quantitative trait loci in maize. Medicine 99, e21326. doi:10.1097/MD.0000000000021326
Xie, Z., Nolan, T. M., Jiang, H., and Yin, Y. (2019). AP2/ERF transcription factor regulatory networks in hormone and abiotic stress responses in Arabidopsis. Front. Plant Sci. 10, 228. doi:10.3389/fpls.2019.00228
Xu, Z.-S., Xia, L.-Q., Chen, M., Cheng, X.-G., Zhang, R.-Y., Li, L.-C., et al. (2007). Isolation and molecular characterization of the Triticum aestivum L. ethylene-responsive factor 1 (TaERF1) that increases multiple stress tolerance. Plant Mol. Biol. 65, 719–732. doi:10.1007/s11103-007-9237-9
Yang, L., Lei, L., Li, P., Wang, J., Wang, C., Yang, F., et al. (2021). Identification of candidate genes conferring cold tolerance to rice (Oryza sativa L.) at the bud-bursting stage using bulk segregant analysis sequencing and linkage mapping. Front. Plant Sci. 12, 647239. doi:10.3389/fpls.2021.647239
Yang, P., Praz, C., Li, B., Singla, J., Robert, C. A., Kessel, B., et al. (2019). Fungal resistance mediated by maize wall associated kinase Zm WAK-RLK 1 correlates with reduced benzoxazinoid content. New Phytol. 221, 976–987. doi:10.1111/nph.15419
Yang, P., Scheuermann, D., Kessel, B., Koller, T., Greenwood, J. R., Hurni, S., et al. (2021). Alleles of a wall associated kinase gene account for three of the major northern corn leaf blight resistance loci in maize. Plant J. 106, 526–535. doi:10.1111/tpj.15183
Yang, Q., Balint-Kurti, P., and Xu, M. (2017). Quantitative disease resistance: Dissection and adoption in maize. Mol. Plant 10, 402–413. doi:10.1016/j.molp.2017.02.004
Yang, Y., Xu, W., Hou, P., Liu, G., Liu, W., Wang, Y., et al. (2019). Improving maize grain yield by matching maize growth and solar radiation. Sci. Rep. 9, 3635–3711. doi:10.1038/s41598-019-40081-z
Zaitlin, D., DeMars, S., and Gupta, M. (1992). Linkage of a second gene for NCLB resistance to molecular markers in maize. Maize Genet. Coop. Newsl. 66, 69–70.
Zang, Z., Lv, Y., Liu, S., Yang, W., Ci, J., Ren, X., et al. (2020). A novel ERF transcription factor, ZmERF105, positively regulates maize resistance to Exserohilum turcicum. Front. Plant Sci. 11, 850. doi:10.3389/fpls.2020.00850
Zhang, G., Wang, F., Qin, J., Wang, D., Zhang, J., Zhang, Y., et al. (2013). Efficacy assessment of antifungal metabolites from Chaetomium globosum No. 05, a new biocontrol agent, against Setosphaeria turcica. Biol. Control 64, 90–98. doi:10.1016/j.biocontrol.2012.10.005
Keywords: northern corn leaf blight, disease resistance, bulk segregant analysis, SNP, QTL
Citation: Zhai R, Huang A, Mo R, Zou C, Wei X, Yang M, Tan H, Huang K and Qin J (2022) SNP-based bulk segregant analysis revealed disease resistance QTLs associated with northern corn leaf blight in maize. Front. Genet. 13:1038948. doi: 10.3389/fgene.2022.1038948
Received: 07 September 2022; Accepted: 31 October 2022;
Published: 25 November 2022.
Edited by:
Satinder Kaur, Punjab Agricultural University, IndiaReviewed by:
Guriqbal Singh Dhillon, Thapar Institute of Engineering & Technology, IndiaYogesh Vikal, Punjab Agricultural University, India
Uday Chand Jha, Indian Institute of Pulses Research (ICAR), India
Copyright © 2022 Zhai, Huang, Mo, Zou, Wei, Yang, Tan, Huang and Qin. This is an open-access article distributed under the terms of the Creative Commons Attribution License (CC BY). The use, distribution or reproduction in other forums is permitted, provided the original author(s) and the copyright owner(s) are credited and that the original publication in this journal is cited, in accordance with accepted academic practice. No use, distribution or reproduction is permitted which does not comply with these terms.
*Correspondence: Kaijian Huang, aGtqaWFuQGd4YWFzLm5ldA==; Jie Qin, cWluamllQGd4YWFzLm5ldA==