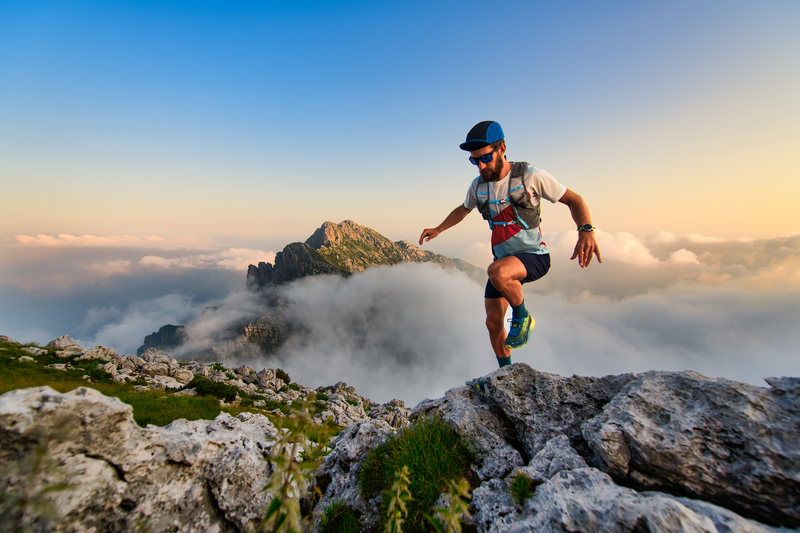
94% of researchers rate our articles as excellent or good
Learn more about the work of our research integrity team to safeguard the quality of each article we publish.
Find out more
ORIGINAL RESEARCH article
Front. For. Glob. Change
Sec. Forest Ecophysiology
Volume 8 - 2025 | doi: 10.3389/ffgc.2025.1557824
The final, formatted version of the article will be published soon.
You have multiple emails registered with Frontiers:
Please enter your email address:
If you already have an account, please login
You don't have a Frontiers account ? You can register here
In the context of climate change, assessing the adaptive potential of species and populations is crucial for developing effective conservation strategies. Changes in plant gene expression play a significant role in the adaptation process to climate change. This study aims to explore the adaptive responses of the conifer species Chamaecyparis hodginsii (the name has been revised from Fokienia hodginsii) to climate change and analyze the molecular-level reactions of these long-lived trees to climatic shifts. It seeks to understand their phenotypic responses to climate change, identify key environmental factors driving adaptive gene expression, and provide information for transplantation conservation strategies based on genetic adaptability. By conducting mixed-tissue RNA sequencing on samples from multiple provenances and employing redundancy analysis (RDA), weighted gene coexpression network analysis (WGCNA), and partial least squares path modeling (PLS-PM), the study assesses the impact of climatic variables on gene expression and phenotype. It identifies key gene groups associated with environmental responses and elucidates the complex relationships between environmental factors, functional gene groups, and phenotypic traits. The findings reveal that C. hodginsii adapts to environmental stresses by regulating specific gene activities related to morphological trait adjustments. Moreover, environmental factors such as the impact on tree architecture emphasize the importance of Precipitation Seasonality, Isothermality, and Precipitation of Driest Quarter for adapting to climate stresses. This research not only unveils the complex adaptive responses of C. hodginsii to climate change but also provides critical insights for the management and conservation of long-lived tree species facing climate change threats.
Keywords: coniferous tree, environmental changes, Gene Expression, Chamaecyparis hodginsii, Adaptability, Seed experiments
Received: 09 Jan 2025; Accepted: 03 Mar 2025.
Copyright: © 2025 He, Zhu, Song, Wu, Lin, Xu, Zhang, Zhang, Rong, Liu, Chen, Zheng and Chen. This is an open-access article distributed under the terms of the Creative Commons Attribution License (CC BY). The use, distribution or reproduction in other forums is permitted, provided the original author(s) or licensor are credited and that the original publication in this journal is cited, in accordance with accepted academic practice. No use, distribution or reproduction is permitted which does not comply with these terms.
* Correspondence:
Pengkai Zhu, Fujian Agriculture and Forestry University, Fuzhou, China
Lingyan Chen, Fujian Agriculture and Forestry University, Fuzhou, China
Disclaimer: All claims expressed in this article are solely those of the authors and do not necessarily represent those of their affiliated organizations, or those of the publisher, the editors and the reviewers. Any product that may be evaluated in this article or claim that may be made by its manufacturer is not guaranteed or endorsed by the publisher.
Research integrity at Frontiers
Learn more about the work of our research integrity team to safeguard the quality of each article we publish.