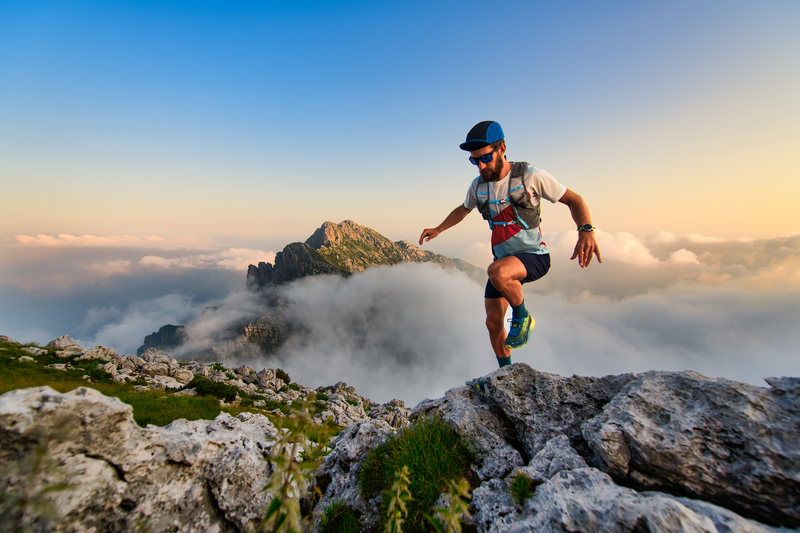
94% of researchers rate our articles as excellent or good
Learn more about the work of our research integrity team to safeguard the quality of each article we publish.
Find out more
ORIGINAL RESEARCH article
Front. For. Glob. Change
Sec. Tropical Forests
Volume 8 - 2025 | doi: 10.3389/ffgc.2025.1519432
The final, formatted version of the article will be published soon.
You have multiple emails registered with Frontiers:
Please enter your email address:
If you already have an account, please login
You don't have a Frontiers account ? You can register here
The Caatinga, a seasonally dry tropical forest in northeastern Brazil, is notable for its biodiversity and high proportion of endemic plants adapted to its semi-arid environment. Among its prominent tree species, Myracrodruon urundeuva (Aroeira) stands out due to its extensive distribution and economic value. Despite its significance, little is known about the environmental factors influencing its distribution. This study uses species distribution modeling (SDM) to assess the current and potential distribution of M. urundeuva and its habitat suitability under various climate change scenarios. Utilizing models like GLM, GAM, and BRT, and Maxent, the research analyzes georeferenced occurrence data and bioclimatic variables (selected by the variance inflation factor) from precipitation and temperature metrics. Our findings indicate that M. urundeuva is projected to experience relative stability or slight expansion in suitable habitats under future climate scenarios, including the pessimistic SSP585 scenario. However, localized habitat losses may occur, particularly in certain regions and timeframes, highlighting the complex and regionally variable impacts of climate change. This study emphasizes the need for localized and regional action plans to mitigate climate change impacts on M. urundeuva's habitats. Conservation efforts should target areas identified as stable, ensuring the species' resilience against escalating climate threats, thereby preserving one of its critical habitats within the Caatinga.
Keywords: Dry forest, species distribution models, environmental suitability maps, Future projections, Predictive biogeography
Received: 29 Oct 2024; Accepted: 28 Feb 2025.
Copyright: © 2025 da Costa, De Lima, Ferreira, de Paula, de Oliveira, da Silva, da Silva, Santana and Pereira. This is an open-access article distributed under the terms of the Creative Commons Attribution License (CC BY). The use, distribution or reproduction in other forums is permitted, provided the original author(s) or licensor are credited and that the original publication in this journal is cited, in accordance with accepted academic practice. No use, distribution or reproduction is permitted which does not comply with these terms.
* Correspondence:
Robson Borges De Lima, Universidade Estadual do Amapá, Macapá, Brazil
Disclaimer: All claims expressed in this article are solely those of the authors and do not necessarily represent those of their affiliated organizations, or those of the publisher, the editors and the reviewers. Any product that may be evaluated in this article or claim that may be made by its manufacturer is not guaranteed or endorsed by the publisher.
Research integrity at Frontiers
Learn more about the work of our research integrity team to safeguard the quality of each article we publish.