- 1Faculty of Forestry and Environmental Management, University of New Brunswick, Fredericton, NB, Canada
- 2Département des Sciences du Bois et de la Forêt, Université Laval, Quebec, QC, Canada
The identification of climate anomalies associated with large-scale stand disturbances can help inform climate-focused forest management. In the spring and summer of 2018, an unusual spike in balsam fir (Abies balsamea) mortality was reported in multiple areas across its southern distribution range limit, from Wisconsin, United States, to New Brunswick, Canada. Such an event was previously reported in 1986 in the study region and referred to as Stillwell’s Syndrome. To identify the role of climate anomalies in the 2018 event, we used monthly climate anomalies as explanatory variables in a Random Forest model predicting the presence of mortality from air and field-based surveys across the Maritimes region of eastern Canada. Results were validated by (1) comparing common climate predictors of mortality from this model and a separate Random Forest model fitted on the 1986 event, and (2) using the 2018 model to predict areas of mortality in 1986. Both the 1986 and 2018 models identified multiple common climate anomalies. Areas with unusually high water deficit and temperatures in the previous growing season, followed by thick April snowpack and high May temperatures the same year, were associated with balsam fir mortality. Such climate anomalies have been previously associated with water stress and desiccation in trees. When using the 2018 model to map the occurrence of mortality in 1986 using historical climate data, we report a 95% accuracy in prediction (kappa = 0.88). The approach used here in conjunction with mapped records of past stand disturbances could help with understanding the impacts of future climate anomalies and adaptive management strategies to deal with these events.
1 Introduction
While mortality is an essential aspect of forest renewal, our capacity to determine specific causes is limited because of the many interacting factors that lead to tree mortality (Franklin et al., 1987). Nonetheless, improving our understanding of the drivers of tree mortality is important under a changing climate. Climate anomalies, such as lower than usual precipitation or higher than usual temperatures or a combination of both can lead to droughts which are continuing to increase the risk of tree mortality globally (Allen et al., 2010; Anderegg et al., 2013).
Tree mortality induced by drought can be caused by carbon starvation or hydraulic failure. Carbon starvation occurs when drought induced closure of stomata leads to a sustained reduction in photosynthate (McDowell et al., 2008). Reduced conductance in the xylem of a tree can occur when water loss from transpiration is not matched by water uptake in the roots, which increases xylem tension and can lead to cavitation and hydraulic failure (McDowell et al., 2011). Both hydraulic failure and carbon starvation are relevant when investigating drought-induced tree mortality, with individual tree responses often being species specific (Adams et al., 2017).
In the spring of 2018, the sudden mortality of a high number of balsam fir trees was reported in New Brunswick (NBERD, 2018), Wisconsin (2019), as well as northern areas of New Hampshire, Vermont, and Maine (Maine Forest Service, 2018; Figure 1). Trees were found dead with intact tree crowns turned bright red, next to healthy fir trees. Mortality in New Brunswick was considered high enough to warrant the government to complete a province-wide aerial survey. This mortality was unlikely to have been caused by spruce budworm (Choristoneura fumiferana), because foliage had no evidence of defoliation. Further, mortality from spruce budworm is reported to take more than four consecutive years of severe defoliation (MacLean, 1980). Anecdotal observations from initial local forest health surveys suggested various contributing factors to mortality including a bark beetle (Pityokteines sparsus), Armillaria root rot, salt exposure (NBERD, 2018; Wisconsin, 2019; Maine Forest Service, 2018). However, due to the broad scale synchronicity of observed mortality, it is likely that climatic conditions played an important role, along with local site conditions (Harvey et al., 2021; Lalande et al., 2020).
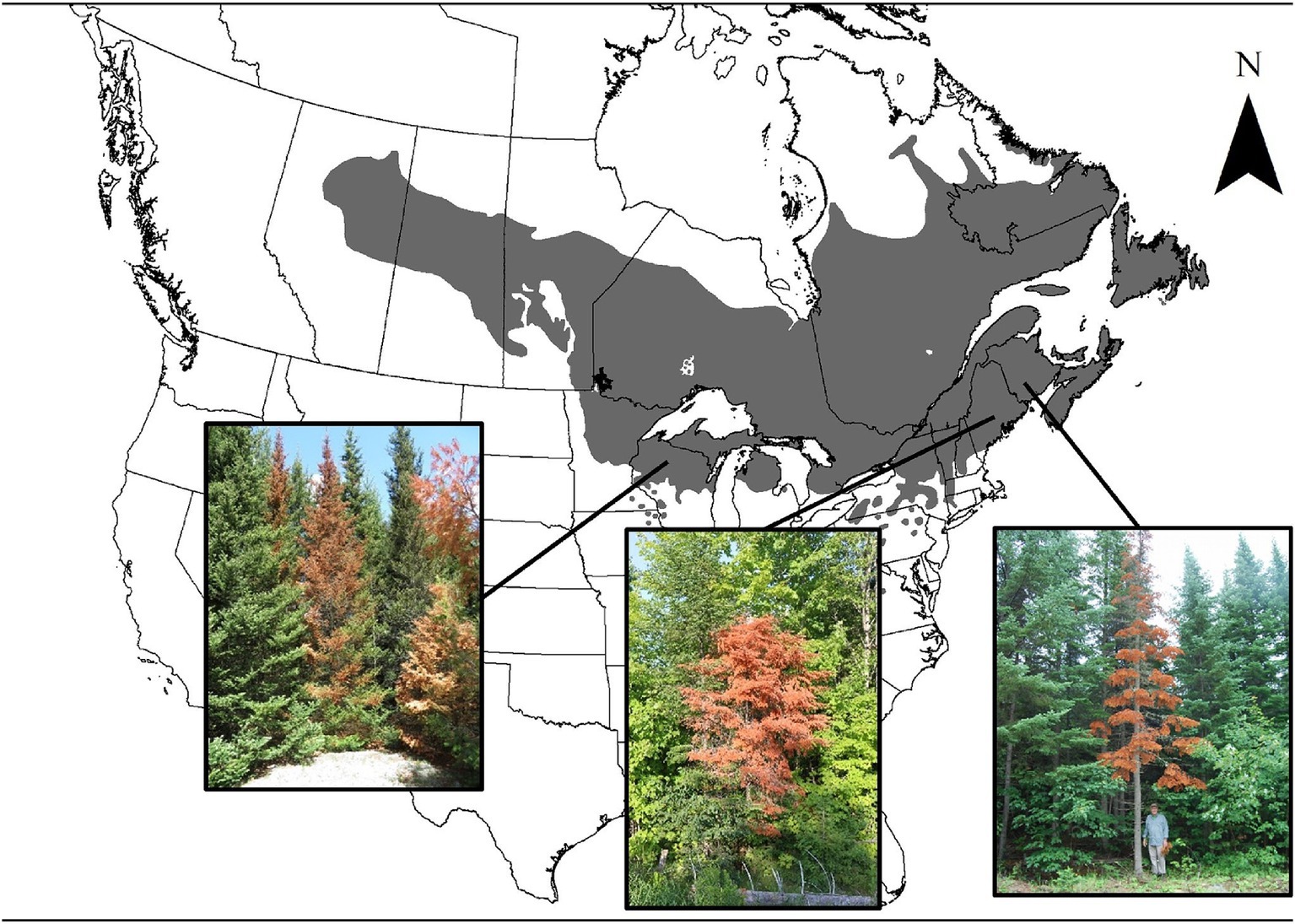
Figure 1. Location of 2018 reports of sudden fir mortality within the current distribution range of balsam fir (Natural Resources Canada, 2015). Reports included pictures shown here of the rapid reddening of intact foliage associated with sudden balsam fir mortality in Wisconsin (2019), northern areas of New Hampshire, Vermont, Maine (Maine Forest Service, 2018) and New Brunswick (NBERD, 2018). Images of the fir trees are used with permission: © Wisconsin Department of Natural Resources (left), Photo taken by Aaron Bergdahl © Maine Forest Service (center), Photo taken by Anthony Taylor © Canadian Forest Service (right).
A similar balsam fir mortality event was reported in the 1980s across the Maritimes region of eastern Canada (Magasi, 1987). Referred to as “sudden death of balsam fir trees” or “Stillwell’s syndrome,” this event was mapped in 1986 due to its high severity that year (Magasi, 1987). Although sudden balsam fir mortality has been historically associated with biotic agents (Magasi, 1987), the rapid appearance of symptoms (bright red, intact crowns) also supports the hypothesis of hydraulic failure, likely linked to drought (Arend et al., 2021). Compared to other species in the region, balsam fir has been reported to be vulnerable to water deficit, due to losses in hydraulic conductance (D’Orangeville et al., 2013; Balducci et al., 2020; Tyree et al., 1994). Identifying the broad scale climate anomalies, such as a drought, associated with the reported sudden balsam fir mortality in 2018 could prove useful to determine the likelihood of future mortality in response to climate change (Bourque et al., 2005). Therefore, the objective of this study was to identify the role of climate anomalies on the 2018 mortality event across the Maritimes region of eastern Canada. In addition, the similarity of the 2018 and 1986 events was assessed by using our analysis of the 2018 event to back cast the location of mortality from 1986, to help independently test and verify causal drivers.
2 Methods
2.1 Study area
The provinces of New Brunswick (NB), Nova Scotia (NS) and Prince Edward Island (PEI) form the Maritime provinces of Canada. They are part of the Atlantic Maritime Ecozone, a region strongly influenced by the Atlantic Ocean resulting in a cool and moist environment. Annual precipitation is between 800 mm inland and over 1,500 mm near the coast. In the upland regions, Humo-Ferric Podzols and glacial tills are the dominant soils with underlying hard, crystalline rocks including granite and gneiss. In lowland areas nearer to the coast, deeper Luvisolic soils are formed from the underlying sedimentary bedrock (Ecological Stratification Working Group, 1996).
2.2 Response variable
Following multiple public reports of sudden balsam fir mortality across NB in the spring of 2018, NB Department of Energy and Resource Development (NBERD) undertook a province-wide aerial survey in July 2018 to identify and locate recently dead balsam fir—defined as balsam fir trees with complete crowns of bright red foliage (NBERD, 2018). There was a total of 84 flight lines covering New Brunswick with lines conducted 5 km apart in northern NB and 11 km apart in the south. Of the 115 areas with recorded mortality during this survey, 82.6% displayed less than 5% mortality, 14.8% displayed between 6 and 30% mortality while the remaining 2.6% displayed more than 30% mortality. The distribution of dead fir trees was generally scattered (43.5% of areas) or in patches (50.4%), with only 6.1% of areas showing widespread mortality. The recorded mortality was likely underestimated due to the broad flight lines used in the aerial survey and subsequent difficulty of aerial surveyors to spot and record individual tree mortality. Further, the targeted ground survey that features in the NBERD report suggests a higher presence of mortality but due to its southern bias we chose to only use the aerial survey data. There were also additional reports of sudden balsam fir mortality after the aerial survey (Drew Carleton, pers. comm.). We therefore deemed the NB absence data to be unreliable and so locations in NB with no sudden balsam fir mortality recorded from the initial aerial survey were excluded from further analysis. Aerial surveys were not flown in the other Maritime provinces due to a lack of reported sudden balsam fir mortality, but we surveyed local foresters in neighboring provinces and were able to confirm that the same extent of balsam fir mortality seen in NB was not reported in NS (Jeffrey Ogden, pers. comm.) and PEI (Mary Myers, pers. comm). For the purpose of this study, both NS and PEI were therefore defined as areas with no sudden balsam fir mortality (absence data) given the absence of reported cases in 2018 (Figure 2).
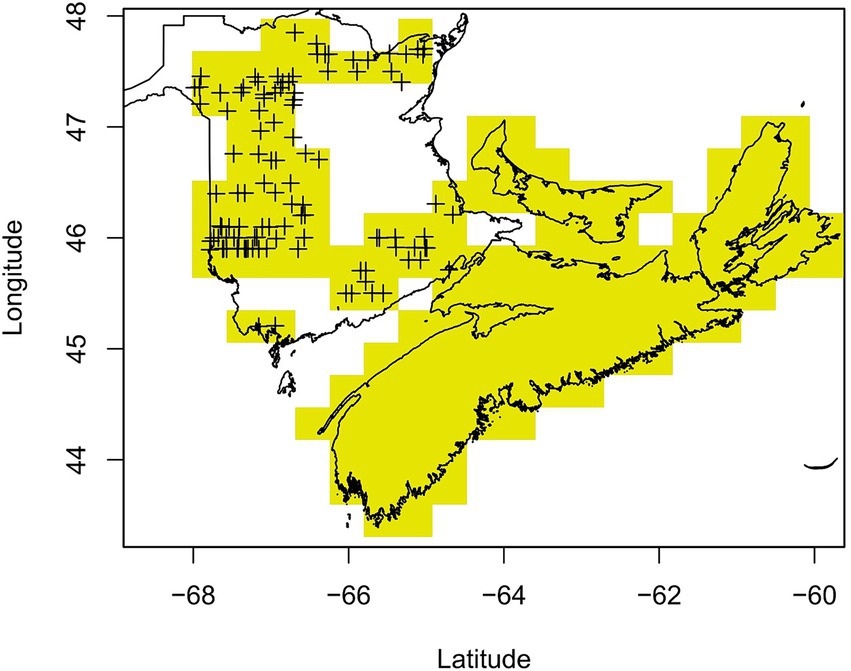
Figure 2. Individual and groups of balsam fir trees with symptoms of sudden mortality were identified from a systematic aerial survey of New Brunswick (NB) indicated by ‘+’ symbols. Areas of NB with no reported mortality from the aerial survey were omitted to avoid false negatives. Nova Scotia and Prince Edward Island had no mortality reported, which was confirmed by a survey of local foresters and became the absence data.
2.3 Climate data
Temperature, precipitation and snow depth data were acquired for the study region from Natural Resources Canada (McKenney et al., 2011). These data were derived from monthly averages of daily maximum temperature (TMAX), minimum temperature (TMIN) and the monthly sum of precipitation, interpolated at 2 km resolution from meteorological weather station data for the 1950–2018 period using thin-plate smoothing splines, implemented in the ANUSPLIN climate modeling software (McKenney et al., 2011). As deeper snow depths combined with earlier melting in spring have been positively correlated with balsam fir growth (Duchesne et al., 2012) and shown to influence crown health (Man et al., 2013), 1979–2018 monthly snow depth data were acquired from the National Center for Environmental Prediction North America Regional Reanalysis (Mesinger et al., 2006) at ~32 km resolution. Temperature and precipitation values were aggregated to a mean value that matched the coarser resolution of the snow depth data using the “raster” package in R (Hijmans, 2024).
To identify climate anomalies across the study area we developed standardized climate indices for each 32 km cell that reflected deviations from the local climate normal. Precipitation data was normalized using the Standardized Precipitation Index (SPI; McKee et al., 1993). The 69 years of precipitation data (1950–2018) were fitted to a gamma distribution and then transformed into a normal distribution with SPI values that represent the standard deviation from the median (which is a SPI value of 0). Positive SPI values indicate higher than average precipitation with negative values indicating drier than usual conditions. We also used the same process to standardize the 40 years of snow depth data (1979–2018), which we refer to as the Standardized Snow Depth Index (SSDI). Periods without any snow can be common, so we fitted a gamma distribution to the data in the same way as the precipitation data (Skaugen and Melvold, 2019). The 69 years of temperature data (1950–2018) were also standardized in a similar method as the SPI, which is referred to as the Standardized Temperature Index (STI; Zscheischler et al., 2014), but, as temperature was normally distributed we fitted the data to a log-logistic distribution. The SPI, SSDI and STI all have units of standard deviations from the long-term average. The “spei” package (Beguería and Vicente-Serrano, 2023) was used to standardize the climate data and the “raster” package (Hijmans, 2024) was used to convert the standardized climate data back to a spatial format in R.
2.4 Data preparation
The presence-absence of sudden balsam fir mortality was then scaled to the ~32 km grid cell resolution of the climate data using the gridRecords function in the “fuzzySim” package in R (Barbosa, 2016). If one or more point occurrences of sudden balsam fir mortality were in a ~ 32 km cell, then that cell was assigned as a presence cell. Only climate data from the previous 15 months prior to widespread sudden balsam fir mortality being reported was included, ranging from March 2017 to May 2018, which ensured a full growing season was investigated. This resulted in 15 monthly climate variables for TMAX, TMIN and precipitation respectively, and nine variables for snow depth (only winter and spring months were included). This gave a total of 54 monthly climate variables.
Three preliminary screening processes were used to reduce the large number of initial monthly climate variables to avoid overfitting when building our predictive model (Supplementary Table 1). First, each climate variable was averaged across presence and absence areas. Next, only climate variables that displayed a greater anomaly in presence areas were retained, thus leaving us with 32 climate variables. Second, a simple logistic regression model was built for each retained climate variable and was used to predict the presence-absence data. A Cohen’s kappa value was obtained from this prediction and only variables that had a significant logistic regression model (p < 0.05) were retained. The kappa value was chosen as the accuracy metric as it gives a more conservative measure of accuracy because it accounts for chance in a binary prediction (Cohen, 1960). Third, collinearity between the remaining 24 explanatory climate variables was determined through Pearson correlations and visualized through the “corrplot” package in R (Wei et al., 2017; Supplementary Figure 1). Each pair of climate variables was then ordered by the strength of their correlation (≥ ± 0.7) and the variable with the higher kappa value from its prediction of presence-absence data (through its logistic regression model), was retained. Faced with the unique situation of two variables, December TMAX and August precipitation, with identical kappa values, we retained August precipitation as we expected a greater influence of growing season climate on mortality. These three preliminary screening processes resulted in 11 final explanatory climate variables (which all had correlations under ±0.7) that were then used in our final modeling analysis (Supplementary Table 2). There were also 8 cells across the study area that had to be removed because of a lack of snow depth data in May, which resulted in a final total of 126 cells across the study area with 42 classed as presence cells and 84 absence cells (~33% presence).
2.5 Random forest modeling
To analyze the presence-absence of sudden balsam fir mortality based on our set of explanatory climate variables, we employed the machine learning algorithm Random Forest (RF; Breiman, 2001). RF analysis accommodates many of the violations of conventional, parametric statistics that are common for observational data, including departures from normality and homogeneity of variance, and are well suited for the analysis of ecological data (Elith, 2019). The probability of mortality reported through the random forest modeling analysis is based on the count of the number of trees across the ensemble of decision trees that vote for one of two classes of ‘presence of mortality’ or ‘absence of mortality’. The default 0.5 threshold was set to determine which class a data point falls within. The reported probability of mortality is the probability of one or more dead balsam fir trees occurring in a ~ 32 km grid cell.
The “caret” package in R (Kuhn, 2009) was used to determine the optimum mtry hyperparameter, which defines the number of explanatory variables that are randomly sampled at each node split in each decision tree of the RF model. The train function used 25 bootstrap resamples (with replacement) of the data to evaluate how different mtry values (1:11) altered the predictive performance of the RF model. Once the optimum mtry value was determined, then a final RF model was run using the “randomForest” package in R (Liaw and Wiener, 2002). The ntree (number of trees) parameter was set at the default (500) to determine when the out-of-bag error stabilized, and then rerun with the appropriate reduced number of trees (Elith, 2019). The node size, which specifies the minimum number of observations in a terminal node in each decision tree, was kept at the default for classification of 1.
2.6 Model validation
Machine learning techniques usually require data to be split into a training dataset, which is used to build a model, and the model is then validated on a withheld testing dataset (on data unseen during the training process). However, when a dataset is relatively small, as in the current analysis, then the testing/training splitting procedure can omit potentially useful data when the model is being trained. RF modeling is useful in this situation as there is an internal training and testing procedure. For each individual decision tree, a new training set is sampled with replacement, where each unique data point can be randomly chosen multiple times. This is known as a bootstrap sample and typically includes 2/3 of the original data observations (Efron and Tibshirani, 1997; Gareth et al., 2013). The remaining 1/3 that was not selected then forms the out-of-bag dataset, which is used for testing purposes. The predicted class (presence or absence of mortality) for a data point is then calculated by a majority vote from the decision trees that did not contain that specific data point (~32 km cell) in the respective bootstrap sample. The overall accuracy of the RF model is based on the proportion of out-of-bag samples that were incorrectly classified across the ensemble of decision trees and is referred to as the out-of-bag error.
The autocorrelated nature of climate data (Dormann et al., 2007) leads to spatially dependent testing and training datasets (Ploton et al., 2020). This limits our ability to properly evaluate the performance of the final RF model based on the out-of-bag error. Due to this spatial autocorrelation, we chose to use mapped records of a similar sudden balsam fir mortality event from 1986 to independently test the 2018 RF model. Areas of high, medium and low frequency of sudden balsam fir mortality were recorded in 1986, as well as areas of mortality absence (Magasi, 1987). High and medium frequency areas were combined due to the small relative size of medium frequency areas and the subsequent overlap of climate data with high frequency areas. First, we used the 2018 RF model to predict (1) areas of medium and high mortality from 1986, and (2) areas of low, medium and high frequency. Next, we fitted a separate RF model for 1986 using the same 11 monthly climate explanatory variables used in the 2018 RF model but limited the presence areas to the high and medium frequency areas only (excluding low frequency areas).
The final step investigated the partial dependence plot (PDP) for each climate variable generated for both the 2018 and 1986 RF models. A PDP gives a graphical representation of how, across the range of values for a single climate variable, the probability of the presence of sudden balsam fir mortality changes. The comparison of the PDPs meant that the level of agreement across the two RF models could be visually interpreted to determine if the effect of each climate variable on mortality probability was consistent for each mortality event. The variable importance for each RF model used the mean decrease in Gini Importance (also referred to as the Mean Decrease in Impurity). This is the average decrease in node impurity, based on each split in a decision tree for a specific variable, across all the individual decision trees that make up a random forest model (Liaw and Wiener, 2002). Inflated impurity-based variable importance related to a varied scale of measurement or number of categories was avoided because the climate explanatory variables were continuous and standardized (Strobl et al., 2007).
3 Results
3.1 Seasonal climate trends from March 2017 to May 2018
The following seasonal descriptions are based on the non-standardized mean averages for monthly climate variables across presence areas to aid interpretation (Supplementary Table 3). Spring 2017 was initially cold and dry in March with a reduced snow depth (Figure 3), then temperatures increased with April and May TMIN + 2.0°C and + 1.2°C warmer than normal. Precipitation was also higher than the average in both April (+11.1 mm) and May (+53.5 mm). Summer 2017 TMAX was warmer than normal with June, July and August +0.5, +0.4 and + 0.5°C above the average, respectively. This was combined with lower than average precipitation, dropping 43% to 42.6 mm and 38% to 34.7 mm in July and August, respectively. The warm wet spring and dry warm summer were followed by an exceptionally warm fall in 2017 with TMAX in September and October +3.2 and + 4.7°C above normal. TMIN was also warmer than the average in September at +2.6°C and October at +2.8°C. Winter 2017–2018 tended to have higher precipitation rates than usual. The initial cold conditions in December, with TMAX and TMIN -2.0°C and − 1.6°C below the average, were followed by warm conditions in February, with TMAX and TMIN + 3.5°C and + 3.0°C above the average, which resulted in a reduction in snow depth, 23% below normal. The 2018 spring leading up to widespread reports of sudden balsam fir mortality began with a warm March (+3.3°C in TMIN), followed by a cool April (−1.2°C for both TMAX and TMIN). The combination of cool temperatures and high precipitation in April (+34.2 mm) led to an exceptionally high snow depth, 94% above normal. The cold April was then followed by a warm May, with TMAX + 2°C higher than the average.
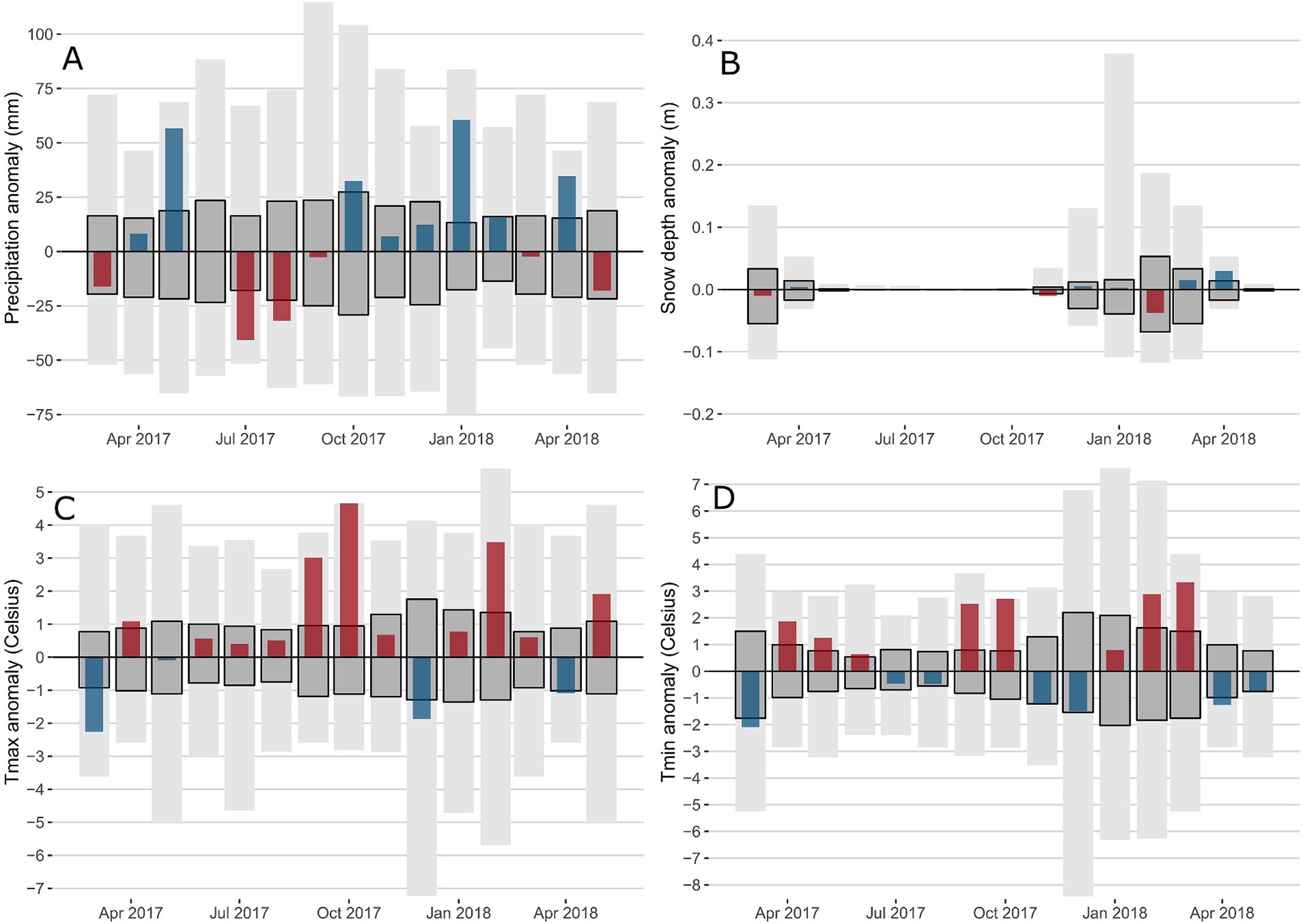
Figure 3. Monthly climatic anomalies over the 2017-2018 period for precipitation (A), snow depth (B), Tmax (C) and Tmin (D). The range of historic anomalies (maximum-minimum) is in light grey. The interquartile range of the historic time series is in dark grey. Climate data is limited to presence areas only.
3.2 The 2018 RF model
The best performing 2018 RF model (mtry = 3, ntree = 250) was accurate with an out-of-bag error rate of 1.6%. The 2018 RF model identified a series of seasonal climate anomalies which can exacerbate water stress. According to the 2018 RF model variable importance scores, the 2017 summer drought was the best predictor of mortality. Low August precipitation was ranked as the most important predictor of sudden balsam fir mortality (Table 1) with over three times the score of the 2nd highest ranked predictor. Dry conditions in March 2017 (63 mm) were also considered important in the 2018 RF model, ranking 3rd. The water deficit was likely exacerbated by unusually high summer temperatures in 2017, although only high temperatures in July were included in the RF model, which were ranked 7th. The thicker than usual snow depth in April 2018 was considered the second most important variable in the RF model with the warmer than usual TMAX in May ranked 5th.
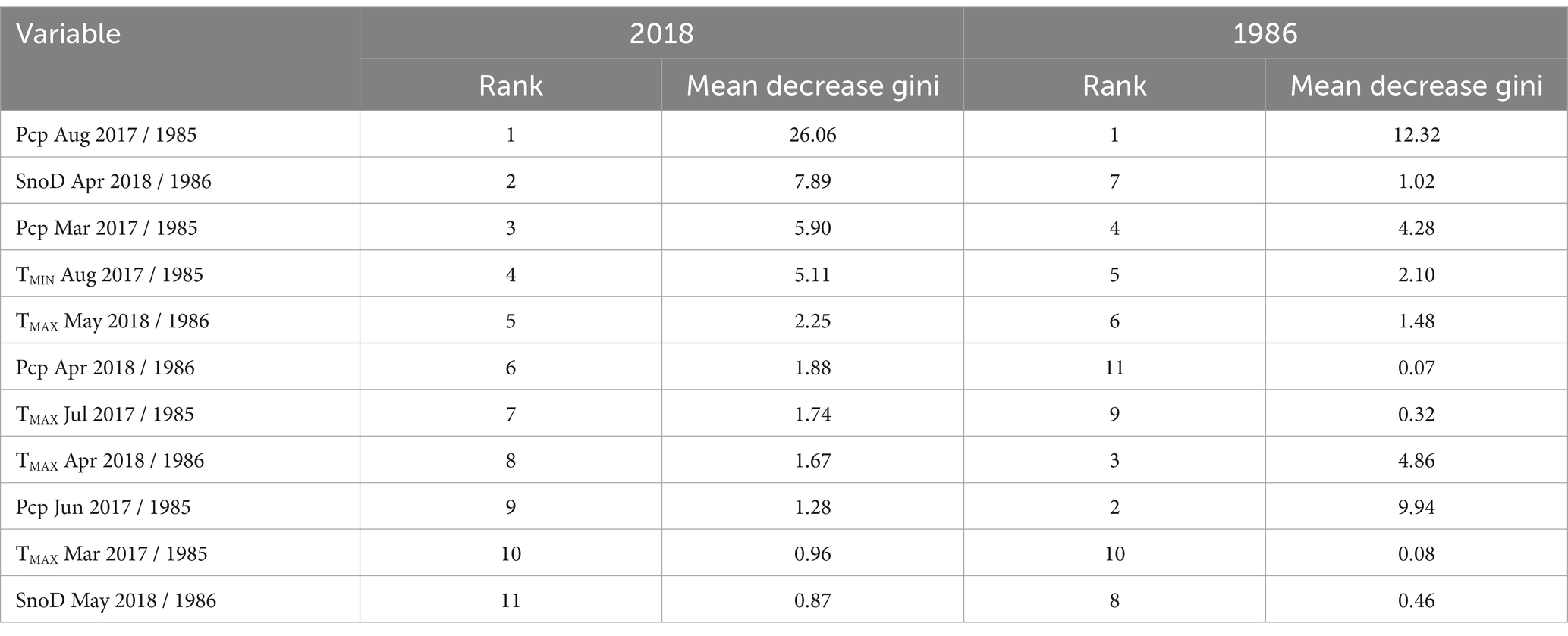
Table 1. 2018 random forest (RF) model and 1986 RF model variable importance rankings for 11 climate explanatory variables used in RF modeling (Pcp, precipitation; SnoD, snow depth; TMIN, minimum temperature; TMAX, maximum temperature).
3.3 Model validation through the 1986 event
When using the 2018 RF model to map the occurrence of mortality (medium and high frequency) in 1986 using historical climate data, we report a 95% accuracy in prediction (kappa = 0.88) with 103 out of 108 raster cells correctly classified (Figure 4). When the model was applied across the whole province (which included the low frequency as well as the medium and high frequency areas), the accuracy dropped to 89% (kappa 0.77), with higher mortality probability in areas that matched the high and medium frequency regions (Figure 4). The lower predictive ability of the 2018 RF model when areas of low frequency were included justified removing these areas from our focus with the 1986 RF model. This was further confirmed when only low frequency areas formed the presence data with the accuracy further dropping to 87% (kappa 0.69).
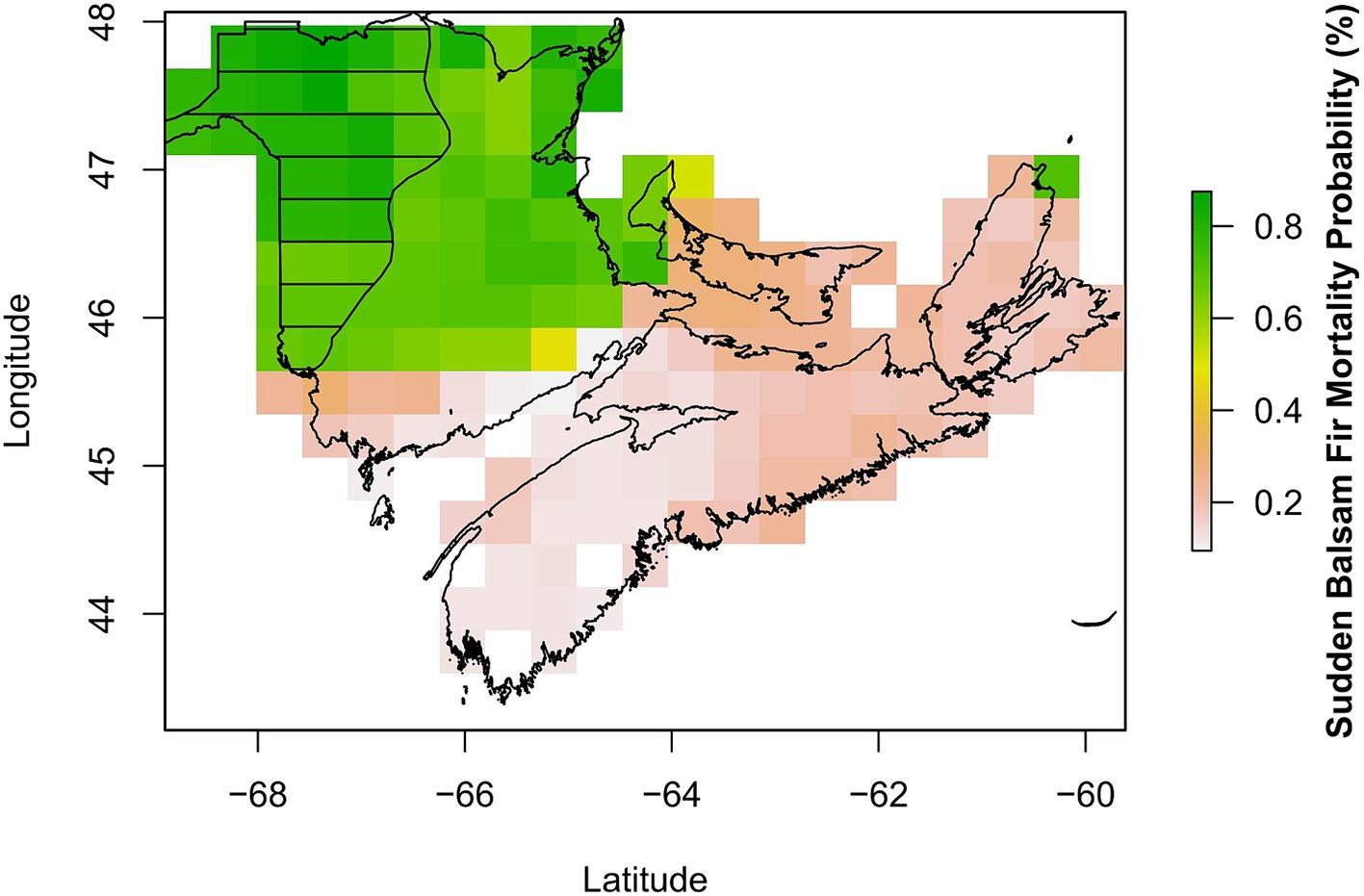
Figure 4. Observed (black horizontal lines) and predicted (coloured) sudden balsam fir mortality from 1986 in Atlantic Canada. The observations correspond to the area of combined medium and high frequency of occurrence as reported in Magasi (1987), while predictions represent the probability of mortality for one or more balsam fir trees in 1986 using the 2018 RF model.
3.4 Similarity between the 1986 and 2018 balsam fir mortality events
Both 1986 and 2018 models identified multiple common climate anomalies. The two most important climate variables in the 2018 RF model were prior August precipitation and spring snow depth, which also had similar effects on mortality probability within the 1986 RF model (Figure 5). In addition, five out of the 11 climate explanatory variables from both 2018 and 1986 RF models displayed similar effects on mortality (Supplementary Figures 3, 4). The 1986 and 2018 RF model importance score rankings also displayed important similarities. Notably, both models had prior-year August precipitation as the most important variable (Table 1). Drier conditions in March of the previous year and previous year minimum August temperatures (TMIN) also substantially increased mortality probability for both events. In the current year that mortality was reported, both events demonstrated a higher mortality probability for areas with a deeper than usual snowpack in April followed by a warmer than usual TMAX in May.
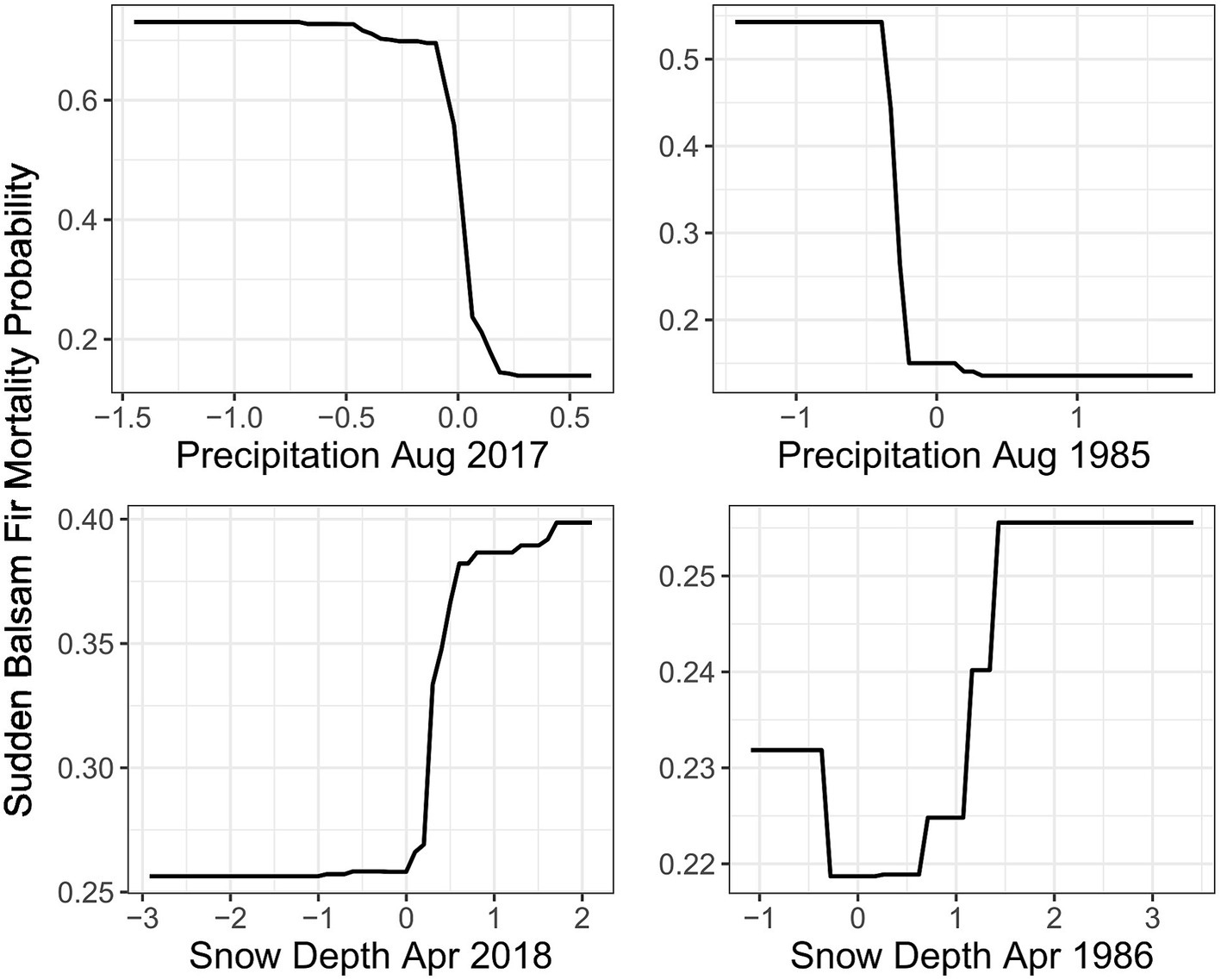
Figure 5. The Partial Dependence Plot for the two most important climate variables in the 2018 Random Forest (RF) model demonstrated a similar mortality probability trend (occurrence of one or more trees with sudden balsam fir mortality) as those from the 1986 RF model. X axis values are the standard deviations from the long term climate average represented by 0.
4 Discussion
Climate-focused forest management hinges on a better understanding of key climatic thresholds for tree species health (Law et al., 2018). Here, we took advantage of aerial mortality surveys of a recent balsam fir mortality event to model the probability of mortality as a function of climate anomalies. Not only do we find clear climatic stressors associated with mortality, but our model calibrated on the 2018 mortality was able to accurately predict the areas of balsam fir mortality from the previous event of 1986. This research demonstrates the feasibility of using recent tree dieback episodes to better understand historic episodes of tree dieback through readily available interpolated historic climate data.
The climatic drivers identified in this study revolve around water deficit. Warm spring conditions in 2017 likely induced an early start to the growing season, which is associated with an extended period of low water availability in the summer (Buermann et al., 2013). Then, the unusually dry summer, combined with temperature anomalies in the summer and fall including unusually high July maximum temperature, likely exacerbated water deficit, leading to more negative xylem tension in fir trees through higher evaporative pull (Grossiord et al., 2020) and reduced available water. The warm temperature anomalies in September and October could have prolonged the low water anomalies, while such high temperatures also raised respiration rates, reducing carbohydrate reserves in already stressed trees (Olesinski et al., 2012). Following this warm fall, fir trees likely entered winter dormancy in a water stressed condition. In the spring, the unusually high snow depth would have maintained low soil temperatures while air temperatures were rapidly increasing. Such conditions typically exacerbate water stress. Indeed, air warming is associated with recovery of photosynthesis and respiration in boreal trees (Bergeron et al., 2007; Tanja et al., 2003), while the higher viscosity of water in cold soils and low root temperatures would have inhibited replacement of the water lost through transpiration (Kaufmann, 1975; Wang and Hoch, 2022). Foliage desiccation has been shown to be more severe in years with higher snowfall and delayed melting (Cairns, 2001), and late snowmelt has been previously associated with foliage damage in balsam fir in Ontario (Man et al., 2013). Thick spring snowpack was also associated with mortality during the 1986 event. Interestingly, 1982 was another year with a thick spring snowpack and high balsam fir mortality across the Maritimes (Magasi, 1983), but a lack of mapped occurrence data restricted our ability to include this event in our analysis.
The unusual climate anomalies observed may have also led to damages to the balsam fir hydraulic transport system through cavitation (Sperry et al., 1998), but the exhaustion of non-structural carbohydrates cannot be excluded (McDowell et al., 2008), or a combination of both (Sevanto et al., 2014). Compared to other coniferous tree species in the region, balsam fir displays higher respiration rates (Hunt et al., 1999) and a greater vulnerability to cavitation that is induced by xylem tension (Balducci et al., 2020; Sperry and Tyree, 1990; Tyree and Dixon, 1986; Tyree and Sperry, 1989). Large legacy effects from drought anomalies have been reported before for balsam fir (D’Orangeville et al., 2018; D’Orangeville et al., 2013). Combined with the high average moisture balance of the region, which inhibits drought adaptations (Jump et al., 2017; Reich et al., 2016), such traits may increase the risk of high balsam fir mortality during drought conditions. Accordingly, balsam fir is projected to display a strong decline across the Maritime provinces under future warming (Bourque et al., 2010; Dombroskie et al., 2010; Taylor et al., 2017). Our study highlights this vulnerability of balsam fir with both drought and warm spring conditions predicted to increase under future warming scenarios (Albert et al., 2023). Forest stakeholders should use this information to support the diversification away from balsam fir (as part of climate-focused forest management). Based on our results, forest stakeholders responsible for the management of balsam fir should be made aware of the negative impact of drought and warming conditions on tree water balance and health. Further, our results highlight the importance of a better understanding of species-specific climatic thresholds in the context of increasing climate anomalies.
Although it was not possible to analyze other events due to a lack of mapped records of mortality, anecdotal reports from other regions where fir mortality was reported do highlight the climate stressors identified in our modeling. Maine (US) experienced a widespread drought anomaly in 2017 (United States Drought Portal, 2017a). In Wisconsin, a drought started in September 2017, along with the delayed melting of the snowpack in April 2018 followed by a rapid transition to warm conditions in May 2018 (United States Drought Portal, 2017b; Wisconsin, 2019). A delay in spring warming with some late snowfall was also observed in Vermont in April 2018 before rapidly warming in May (Vermont Department of Forests Parks and Recreation, 2018a,b).
Individual tree mortality is a complicated process with many predisposing, inciting and contributing factors (Manion, 1981; Sinclair, 1965). While our evidence suggests that climate was the tipping point, local biotic factors such as the presence of balsam fir bark weevil (Pissodes dubius) and Armillaria root disease may have acted as predisposing factors responsible for the sporadic nature of mortality within stands. Both were anecdotally observed on dead fir trees during the 1982–1986 event (Magasi, 1987). There was also reports of a heavy cone crop for balsam fir in 2017 which could have also reduced carbohydrate reserves (Bouchard and Pernot, 2020). In addition, climate stress prior to 2017, such as sequential multiyear droughts, could have also acted as predisposing factors (Sánchez-Pinillos et al., 2021).
It is notable that the sudden mortality of balsam fir trees in 2018 occurred outside of a spruce budworm outbreak. This contrasts with the 1986 event where there had been a spruce budworm outbreak across Maritime Canada throughout the 1980s with trees in the recovery stage experiencing sudden mortality (Magasi, 1987). These trees were probably weakened by the defoliation and predisposed to the subsequent climate stress observed. In contrast, we have no documented evidence that the balsam fir that experienced mortality in 2018 had suffered injuries from specific biotic stressors across the study region. However, we cannot exclude the presence of predisposing factors, which could be secondary biotic agents such as the balsam fir bark beetle, Armillaria root rot or additional climatic stressors prior to 2017 (NBERD, 2018; Wisconsin, 2019; Maine Forest Service, 2018).
Given the projected increase in climate anomalies with climate change, spatial records of tree mortality are increasingly important to determine the importance of climatic drivers of species health. Here, the analysis of the 1986 event was made possible because of the Forest Insect and Disease Survey, a Canada-wide tree health monitoring program that ceased in 1995 (Hurley and Magasi, 1996). Such annual spatial surveys of tree diebacks and declines, coupled with historic climate data, is critical to improve our understanding of species-specific vulnerabilities across forested landscapes and to help inform climate-focused management practices.
Data availability statement
The raw data supporting the conclusions of this article will be made available by the authors, without undue reservation.
Author contributions
JB: Conceptualization, Formal analysis, Investigation, Writing – original draft, Writing – review & editing. AT: Conceptualization, Funding acquisition, Writing – original draft, Writing – review & editing. LD'O: Conceptualization, Funding acquisition, Supervision, Writing – original draft, Writing – review & editing.
Funding
The author(s) declare that financial support was received for the research, authorship, and/or publication of this article. This research project was supported by a NSERC Alliance grant (ALLRP 556150–20) to LD’O. We acknowledge support from the Natural Sciences and Engineering Research Council of Canada under the Alliance and Discovery programs. JB also received financial support from the Canadian Memorial Foundation and the New Brunswick Innovation Fund.
Acknowledgments
Kara Costanza helped to initiate the research project. Paul Cigan and Aaron Bergdahl provided images for Figure 1.
Conflict of interest
The authors declare that the research was conducted in the absence of any commercial or financial relationships that could be construed as a potential conflict of interest.
Publisher’s note
All claims expressed in this article are solely those of the authors and do not necessarily represent those of their affiliated organizations, or those of the publisher, the editors and the reviewers. Any product that may be evaluated in this article, or claim that may be made by its manufacturer, is not guaranteed or endorsed by the publisher.
Supplementary material
The Supplementary material for this article can be found online at: https://www.frontiersin.org/articles/10.3389/ffgc.2024.1472699/full#supplementary-material
References
Adams, H. D., Zeppel, M. J. B., Anderegg, W. R. L., Hartmann, H., Landhäusser, S. M., Tissue, D. T., et al. (2017). A multi-species synthesis of physiological mechanisms in drought-induced tree mortality. Nat. Ecol. Evol. 1, 1285–1291. doi: 10.1038/s41559-017-0248-x
Albert, C., Taylor, A. R., Logan, T., and D’Orangeville, L. (2023). The Acadian Forest of New Brunswick in the 21st century: what shifting heat and water balance imply for future stand dynamics and management. Environ. Rev. 31, 690–707. doi: 10.1139/er-2022-0122
Allen, C. D., Macalady, A. K., Chenchouni, H., Bachelet, D., McDowell, N., Vennetier, M., et al. (2010). A global overview of drought and heat-induced tree mortality reveals emerging climate change risks for forests. For. Ecol. Manag. Adap. Forests and Forest Manag. Chang. Climate 259, 660–684. doi: 10.1016/j.foreco.2009.09.001
Anderegg, W. R., Kane, J. M., and Anderegg, L. D. (2013). Consequences of widespread tree mortality triggered by drought and temperature stress. Nat. Clim. Chang. 3, 30–36. doi: 10.1038/nclimate1635
Arend, M., Link, R. M., Patthey, R., Hoch, G., Schuldt, B., and Kahmen, A. (2021). Rapid hydraulic collapse as cause of drought-induced mortality in conifers. Proc. Natl. Acad. Sci. 118:e2025251118. doi: 10.1073/pnas.2025251118
Balducci, L., Fierravanti, A., Rossi, S., Delzon, S., De Grandpré, L., Kneeshaw, D. D., et al. (2020). The paradox of defoliation: declining tree water status with increasing soil water content. Agric. For. Meteorol. 290:108025. doi: 10.1016/j.agrformet.2020.108025
Barbosa, A. (2016). fuzzySim: fuzzy similarity in species distributions. R package version 1.7. 8/r95.
Beguería, S., and Vicente-Serrano, S.M. (2023). Package ‘spei.’ Calc. Stand. Precip.-evapotranspiration index.Available at: https://spei.csic.es, https://github.com/sbegueria/SPEI
Bergeron, O., Margolis, H. A., Black, T. A., Coursolle, C., Dunn, A. L., Barr, A. G., et al. (2007). Comparison of carbon dioxide fluxes over three boreal black spruce forests in Canada. Glob. Chang. Biol. 13, 89–107. doi: 10.1111/j.1365-2486.2006.01281.x
Bouchard, M., and Pernot, C. (2020). Climate and size of previous cone crops contribute to large-scale synchronous cone production in balsam fir. Can. J. For. Res. 51, 638–646. doi: 10.1139/cjfr-2020-0054
Bourque, C. P.-A., Cox, R. M., Allen, D. J., Arp, P. A., and Meng, F.-R. (2005). Spatial extent of winter thaw events in eastern North America: historical weather records in relation to yellow birch decline. Glob. Chang. Biol. 11, 1477–1492. doi: 10.1111/j.1365-2486.2005.00956.x
Bourque, C. P.-A., Hassan, Q. K., and Swift, D. (2010). Modelled potential species distribution for current and projected future climates for the Acadian Forest region of Nova Scotia. Canada: University of New Brunswick, Fredericton (NB).
Buermann, W., Bikash, P. R., Jung, M., Burn, D. H., and Reichstein, M. (2013). Earlier springs decrease peak summer productivity in north American boreal forests. Environ. Res. Lett. 8:024027. doi: 10.1088/1748-9326/8/2/024027
Cairns, D. M. (2001). Patterns of winter desiccation in krummholz forms of abies lasiocarpa at treeline sites in glacier national park, Montana, Usa. Geogr. Ann. Ser. A Phys. Geogr. 83, 157–168. doi: 10.1111/j.0435-3676.2001.00151.x
Cohen, J. (1960). A coefficient of agreement for nominal scales. Educ. Psychol. Meas. 20, 37–46. doi: 10.1177/001316446002000104
D’Orangeville, L., Côté, B., Houle, D., and Morin, H. (2013). The effects of throughfall exclusion on xylogenesis of balsam fir. Tree Physiol. 33, 516–526. doi: 10.1093/treephys/tpt027
D’Orangeville, L., Maxwell, J., Kneeshaw, D., Pederson, N., Duchesne, L., Logan, T., et al. (2018). Drought timing and local climate determine the sensitivity of eastern temperate forests to drought. Glob. Chang. Biol. 24, 2339–2351. doi: 10.1111/gcb.14096
Dombroskie, S., McKendy, M., Ruelland, C., Richards, W., Bourque, C. P.-A., and Meng, F.-R. (2010). Assessing impact of projected future climate on tree species growth and yield: development of an evaluation strategy. Mitig. Adapt. Strateg. Glob. Chang. 15, 307–320. doi: 10.1007/s11027-010-9218-1
Dormann, C., McPherson, J., Araújo, M., Bivand, R., Bolliger, J., Carl, G., et al. (2007). Methods to account for spatial autocorrelation in the analysis of species distributional data: a review. Ecography 30, 609–628. doi: 10.1111/j.2007.0906-7590.05171.x
Duchesne, L., Houle, D., and D’Orangeville, L. (2012). Influence of climate on seasonal patterns of stem increment of balsam fir in a boreal forest of Québec. Canada. Agric. For. Meteorol. 162-163, 108–114. doi: 10.1016/j.agrformet.2012.04.016
Ecological Stratification Working Group (1996). A national ecological framework for Canada. Hull, Quebec: Centre for Land and Biological Resources Research.
Efron, B., and Tibshirani, R. (1997). Improvements on cross-validation: the 632+ bootstrap method. J. Am. Stat. Assoc. 92, 548–560. doi: 10.1080/01621459.1997.10474007
Elith, J. (2019). 15-machine learning, random forests, and boosted regression trees. In L. A. Brennan, A. N. Tri, and B. G. Marcot, eds, Quantitative Analyses in Wildlife Science. Baltimore: John Hopkins University Press.
Franklin, J. F., Shugart, H. H., and Harmon, M. E. (1987). Tree death as an ecological process. Bio Sci. 37, 550–556. doi: 10.2307/1310665
Gareth, J., Daniela, W., Trevor, H., and Robert, T. (2013). An introduction to statistical learning: With applications in R. New York: Springer.
Grossiord, C., Buckley, T. N., Cernusak, L. A., Novick, K. A., Poulter, B., Siegwolf, R. T. W., et al. (2020). Plant responses to rising vapor pressure deficit. New Phytol. 226, 1550–1566. doi: 10.1111/nph.16485
Harvey, B. J., Andrus, R. A., Battaglia, M. A., Negrón, J. F., Orrego, A., and Veblen, T. T. (2021). Droughty times in Mesic places: factors associated with forest mortality vary by scale in a temperate subalpine region. Ecosphere 12:e03318. doi: 10.1002/ecs2.3318
Hijmans, R. (2024). raster: Geographic Data Analysis and Modeling. R package version 3. 6–28. Available at: https://rspatial.org/raster
Hunt, E. R., Lavigne, M. B., and Franklin, S. E. (1999). Factors controlling the decline of net primary production with stand age for balsam fir in Newfoundland assessed using an ecosystem simulation model. Ecol. Model. 122, 151–164. doi: 10.1016/S0304-3800(99)00135-0
Hurley, J. E., and Magasi, L. P. (1996). Forest pest conditions in the Maritimes in 1995, vol. M-X–199. Fredericton: Maritimes Forest Research Centre.
Jump, A. S., Ruiz-Benito, P., Greenwood, S., Allen, C. D., Kitzberger, T., Fensham, R., et al. (2017). Structural overshoot of tree growth with climate variability and the global spectrum of drought-induced forest dieback. Glob. Chang. Biol. 23, 3742–3757. doi: 10.1111/gcb.13636
Kaufmann, M. R. (1975). Leaf Water Stress in Engelmann Spruce. Plant Physiol. 56, 841–844. doi: 10.1104/pp.56.6.841
Lalande, B. M., Hughes, K., Jacobi, W. R., Tinkham, W. T., Reich, R., and Stewart, J. E. (2020). Subalpine fir mortality in Colorado is associated with stand density, warming climates and interactions among fungal diseases and the western balsam bark beetle. For. Ecol. Manag. 466:118133. doi: 10.1016/j.foreco.2020.118133
Law, D. J., Adams, H. D., Breshears, D. D., Cobb, N. S., Bradford, J. B., Zou, C. B., et al. (2018). Bioclimatic envelopes for individual demographic events driven by extremes: plant mortality from drought and warming. Int. J. Plant Sci. 180, 53–62. doi: 10.1086/700702
MacLean, D. A. (1980). Vulnerability of fir-spruce stands during uncontrolled spruce budworm outbreaks: a review and discussion. For. Chron. 56, 213–221. doi: 10.5558/tfc56213-5
Magasi, L. P. (1983). Forest pest conditions in the Maritimes in 1982, vol. M-X–141. Fredericton, NB: Maritimes Forest Research Centre.
Magasi, L. P. (1987). Forest pest conditions in the Maritimes in 1986, vol. M-X–161. Fredericton, NB: Maritimes Forest Research Centre.
Maine Forest Service (2018). Forest & Shade Tree-Insect & Disease Conditions for Maine. Augusta, Maine: Maine Department of Agriculture, Conservation and Forestry.
Man, R., Colombo, S., Kayahara, G. J., Duckett, S., Velasquez, R., and Dang, Q.-L. (2013). A case of extensive conifer needle browning in northwestern Ontario in 2012: winter drying or freezing damage? For. Chron. 89, 675–680. doi: 10.5558/tfc2013-120
McDowell, N. G., Beerling, D. J., Breshears, D. D., Fisher, R. A., Raffa, K. F., and Stitt, M. (2011). The interdependence of mechanisms underlying climate-driven vegetation mortality. Trends Ecol. Evol. 26, 523–532. doi: 10.1016/j.tree.2011.06.003
McDowell, N., Pockman, W. T., Allen, C. D., Breshears, D. D., Cobb, N., Kolb, T., et al. (2008). Mechanisms of plant survival and mortality during drought: why do some plants survive while others succumb to drought? New Phytol. 178, 719–739. doi: 10.1111/j.1469-8137.2008.02436.x
McKee, T.B., Doesken, N.J., and Kleist, J., (1993). “The relationship of drought frequency and duration to time scales,” in: Proceedings of the 8th Conference on Applied Climatology. Boston, MA, USA, pp. 179–183.
McKenney, D. W., Hutchinson, M. F., Papadopol, P., Lawrence, K. M., Pedlar, J. H., Campbell, K. L., et al. (2011). Customized spatial climate models for North America. AMS J. 92, 1611–1622. doi: 10.1175/2011BAMS3132.1
Mesinger, F., DiMego, G., Kalnay, E., Mitchell, K., Shafran, P. C., Ebisuzaki, W., et al. (2006). North American regional reanalysis. Bull. Am. Meteorol. Soc. 87, 343–360. doi: 10.1175/BAMS-87-3-343
Natural Resources Canada , (2015). Balsam fir [WWW document]. Balsam Fir. Available at: https://tidcf.nrcan.gc.ca/en/trees/factsheet/80 (accessed on 4.10.22).
NBERD (2018). Summary of Forest Pest conditions in New Brunswick in 2018. New Brunswick: New Brunswick Department of Energy and Resources Development Forest Planning & stewardship branch.
Olesinski, J., Krasowski, M. J., Lavigne, M. B., Kershaw, J. A., and Bernier, P. Y. (2012). Fine root production varies with climate in balsam fir (Abies balsamea). Can. J. For. Res. 42, 364–374. doi: 10.1139/x11-194
Ploton, P., Mortier, F., Réjou-Méchain, M., Barbier, N., Picard, N., Rossi, V., et al. (2020). Spatial validation reveals poor predictive performance of large-scale ecological mapping models. Nat. Commun. 11:4540. doi: 10.1038/s41467-020-18321-y
Reich, R. M., Lundquist, J. E., and Hughes, K. (2016). Host-environment mismatches associated with subalpine fir decline in Colorado. J. For. Res. 27, 1177–1189. doi: 10.1007/s11676-016-0234-1
Sánchez-Pinillos, M., D’Orangeville, L., Boulanger, Y., Comeau, P., Wang, J., Taylor, A. R., et al. (2021). Sequential droughts: a silent trigger of boreal forest mortality. Glob. Chang. Biol. 28, 542–556. doi: 10.1111/gcb.15913
Sevanto, S., Mcdowell, N. G., Dickman, L. T., Pangle, R., and Pockman, W. T. (2014). How do trees die? A test of the hydraulic failure and carbon starvation hypotheses. Plant Cell Environ. 37, 153–161. doi: 10.1111/pce.12141
Sinclair, W. (1965). Comparisons of recent declines of white ash, oaks, and sugar maple in northeastern woodlands. Cornell Plant 20, 62–67.
Skaugen, T., and Melvold, K. (2019). Modeling the snow depth variability with a high-resolution Lidar data set and nonlinear terrain dependency. Water Resour. Res. 55, 9689–9704. doi: 10.1029/2019WR025030
Sperry, J. S., Adler, F. R., Campbell, G. S., and Comstock, J. P. (1998). Limitation of plant water use by rhizosphere and xylem conductance: results from a model. Plant Cell Environ. 21, 347–359. doi: 10.1046/j.1365-3040.1998.00287.x
Sperry, J. S., and Tyree, M. T. (1990). Water-stress-induced xylem embolism in three species of conifers. Plant Cell Environ. 13, 427–436. doi: 10.1111/j.1365-3040.1990.tb01319.x
Strobl, C., Boulesteix, A.-L., Zeileis, A., and Hothorn, T. (2007). Bias in random forest variable importance measures: illustrations, sources and a solution. BMC Bioinfo. 8:25. doi: 10.1186/1471-2105-8-25
Tanja, S., Berninger, F., Vesala, T., Markkanen, T., and Hari, P. (2003). Air temperature triggers the recovery of evergreen boreal forest photosynthesis in spring. Glob. Chang. Biol. 9, 1410–1426. doi: 10.1046/j.1365-2486.2003.00597.x
Taylor, A. R., Boulanger, Y., Price, D. T., Cyr, D., McGarrigle, E., Rammer, W., et al. (2017). Rapid 21st century climate change projected to shift composition and growth of Canada’s Acadian Forest region. For. Ecol. Manag. 405, 284–294. doi: 10.1016/j.foreco.2017.07.033
Tyree, M. T., Davis, S. D., and Cochard, H. (1994). Biophysical perspectives of xylem evolution: is there a tradeoff of hydraulic efficiency for vulnerability to dysfunction? IAWA J. 15, 335–360. doi: 10.1163/22941932-90001369
Tyree, M. T., and Dixon, M. A. (1986). Water stress induced cavitation and embolism in some woody plants. Physiol. Plant. 66, 397–405. doi: 10.1111/j.1399-3054.1986.tb05941.x
Tyree, M. T., and Sperry, J. S. (1989). Vulnerability of xylem to cavitation and embolism. Annu. Rev. Plant Biol. 40, 19–36. doi: 10.1146/annurev.pp.40.060189.000315
United States Drought Portal (2017a). National integrated drought information system. Available at: https://www.drought.gov/states/maine (Accessed on Sep 06, 2024).
United States Drought Portal (2017b). National Integrated Drought Information System. Available at: https://www.drought.gov/states/wisconsin (Accessed on Sep 06, 2024).
Vermont Department of Forests Parks and Recreation (2018a). Insect and disease observations— April 2018. Available from https://fpr.vermont.gov/sites/fpr/files/Forest_and_Forestry/Forest_Health/Library/2018%20Forest%20Health%20April%20Observations.pdf (Accessed on Sep 06, 2024).
Vermont Department of Forests Parks and Recreation (2018b). Insect and disease observations— May 2018. Available from https://fpr.vermont.gov/sites/fpr/files/Forest_and_Forestry/Forest_Health/Library/2018%20Forest%20Health%20May%20Observations.pdf (Accessed on Sep 06, 2024).
Wang, W., and Hoch, G. (2022). Negative effects of low root temperatures on water and carbon relations in temperate tree seedlings assessed by dual isotopic labelling. Tree Physiol. 42, 1311–1324. doi: 10.1093/treephys/tpac005
Wei, T., Simko, V., Levy, M., Xie, Y., Jin, Y., and Zemla, J. (2017). Package ‘corrplot.’. Underst. Stat. 56:e24.
Wisconsin, D. N. R. (2019). Wisconsin DNR Forest health 2018 annual report : Wisconsin Department of Natural Resources.
Keywords: drought, mortality, forest disturbance, climate change, Stillwell’s syndrome, Abies balsamea, Acadian forest
Citation: Broom J, Taylor AR and D’Orangeville L (2024) Investigating climate anomalies associated with the sudden mortality of balsam fir trees in eastern Canada. Front. For. Glob. Change. 7:1472699. doi: 10.3389/ffgc.2024.1472699
Edited by:
Christopher Asaro, Forest Health Protection, USDA Forest Service, United StatesReviewed by:
Brian Russell Sturtevant, Northern Research Station, Forest Service (USDA), United StatesKevin J. Dodds, Forest Health Protection, Forest Service (USDA), United States
Copyright © 2024 Broom, Taylor and D’Orangeville. This is an open-access article distributed under the terms of the Creative Commons Attribution License (CC BY). The use, distribution or reproduction in other forums is permitted, provided the original author(s) and the copyright owner(s) are credited and that the original publication in this journal is cited, in accordance with accepted academic practice. No use, distribution or reproduction is permitted which does not comply with these terms.
*Correspondence: Loïc D’Orangeville, bG9pYy5kb3JhbmdldmlsbGVAc2JmLnVsYXZhbC5jYQ==