- 1School for Environment and Sustainability, University of Michigan, Ann Arbor, MI, United States
- 2Department of Aerospace Engineering, University of Michigan, Ann Arbor, MI, United States
- 3Department of Ecology and Evolutionary Biology, University of Michigan, Ann Arbor, MI, United States
- 4Departamento de Biología, Universidad de Puerto Rico, Recinto de Utuado, Utuado, Puerto Rico
Concerns over the capacity of the world’s existing agricultural land to provide food for the global population under climate change and continued biodiversity loss have set the stage for a prevailing narrative of inherent tradeoffs with agricultural production. Coffee, a major export of tropical countries, offers a unique opportunity to examine how different management practices can lead to a variety of outcomes in food security, ecosystem services, and biodiversity conservation. Our study examined this intersection to identify tradeoffs and synergies using compiled data from Puerto Rico. At the island level, we analyzed data on coffee yield and planted area under shade or sun management. At the farm level, we analyzed management variables (percent shade cover, maximum canopy height, ground cover, and food crop richness), non-provisioning ecosystem services variables (total farm carbon storage, soil organic carbon storage, coffee plant carbon biomass, and hurricane resistance and resilience), and biodiversity variables (ant, bird, and lizard richness and abundance). At the island level, we found that planted area was the most significant predictor of total production, suggesting no obvious tradeoff between production and shade management in coffee farms. At the farm level, canopy cover of shade trees was negatively correlated with ground cover and positively correlated with food crop richness, suggesting a synergy between agroforestry and subsistence food production. We detected mostly synergies associated with ecosystem services, biodiversity conservation, and agroforestry management and no tradeoffs among ecosystem service and biodiversity parameters. Shade canopy cover significantly increased total carbon storage, coffee plant biomass, hurricane resistance, and bird species richness. Shade canopy height had a similar positive effect on total farm carbon storage while food crop richness had a positive effect on farm resilience following Hurricane Maria. Ground cover was positively associated with soil carbon storage and pest-controlling lizard abundance. Tradeoffs related to agroforestry management included an inverse relationship between ground cover and hurricane resistance and more dominance of an invasive ant species in farms with higher shade canopies. We discuss the implications of practicing agroforestry principles in this smallholder coffee system and highlight opportunities to contribute to more diversified food production systems that support biodiversity and ecosystem services.
Introduction
Home to almost 3 million inhabitants, Puerto Rico produces specialty coffee with congenial flavor profiles that are a product of both the dedicated livelihoods of smallholder farmers and the cultural significance of the drink. Ever entwined, the history of coffee and agricultural production in Puerto Rico has evolved alongside ever-changing social-political environments and colonial transitions since the 16th century. Under present-day jurisdiction by the U.S. Government, agriculture for local consumption has greatly diminished and the island has grown increasingly reliant on imported food and processed supermarket goods (Diaz, 2016) leaving food prices and supply vulnerable to disruptions from natural disasters and top-down trade policies such as the Jones Act (García-López, 2018; Rodríguez-Díaz, 2018). In addition, many of the agricultural support programs in Puerto Rico cater more to large scale, high-input farming operations (Perfecto et al., 2009; Félix and Holt-Giménez, 2017) than those that are more agroecological and aligned with the principles of food sovereignty, according to farmers (Diaz and Hunsberger, 2018). Compared to “sun,” or conventional coffee growing operations, agroecological, or “shade,” coffee farms are often managed as agroforestry systems with a variety of shade trees (e.g., Inga spp., Andira inermis, Guarea spp.), fruit trees, and food crops (e.g., citrus, avocado, plantains, and a variety of tubers) grown for sale or consumption by farming households in addition to coffee (Rice, 2008; Cerda et al., 2017; Perfecto et al., 2019; Stratton et al., 2020).
The conservation and ecosystem service benefits of agroforestry management in coffee production include in-farm biodiversity conservation (Philpott et al., 2006; Bos et al., 2007; Hajian-Forooshani et al., 2014; Cely-Santos and Philpott, 2019), the creation of a high-quality agroecological matrix that facilitates migration of wildlife between forest fragments (Perfecto et al., 2007, 2019; Jha et al., 2014; Perfecto and Vandermeer, 2015), increased soil drainage and water retention (Lin, 2010; Cannavo et al., 2011), partial mitigation of temperature and climate fluctuations (Campanha et al., 2004; Lin, 2007; de Souza et al., 2012; Jha et al., 2014; Rice, 2018) improved soil quality (de Souza et al., 2012; Thomazini et al., 2015), enhanced carbon storage (De Beenhouwer et al., 2016; Denu et al., 2016), and reduced incidence of coffee pests and pathogens (López-Bravo et al., 2012; Rice, 2018; Vandermeer and Perfecto, 2019). Previous studies have also reported co-benefits, or synergies, between ecosystem services and biodiversity conservation such as greater carbon storage and higher tree diversity in coffee farms under agroforestry management than in more intensively managed coffee systems (Häger, 2012; De Beenhouwer et al., 2016; Cerda et al., 2017; Guillemot et al., 2018).
Along with the synergies, however, tradeoffs in agroforestry coffee production compared to conventional production may also exist in the form of increased disease spread (Schroth et al., 2000; Avelino et al., 2020; Durand-Bessart et al., 2020), reduced or more variable coffee yields (Campanha et al., 2004; Vaast et al., 2016; Durand-Bessart et al., 2020), and changes in the predator-prey dynamics of pests and biological control agents that are still under study (Staver et al., 2001; Hajian-Forooshani et al., 2016; Beilhe et al., 2020). Yet, one of the greatest threats to Puerto Rico’s coffee-growing operations comes from climate change and amplified hurricane frequency and severity. Under mid-high climate warming scenarios, Fain et al. (2018) predicted an acceleration of warming and drying trends in the coffee-producing region of Puerto Rico and a 47% loss of areas with optimal temperature, precipitation, and soil conditions for growing coffee. Additionally, other climate change effect models predict substantial increases in the intensity and frequency of the most intense tropical cyclones in the Atlantic (Elsner et al., 2008). Following the landfall of Hurricane Maria in September of 2017 (Category 4), sustained wind speeds of 155 mph (National Weather Service, 2017) inflicted widespread damage to shade trees and surrounding forest, as expected for a hurricane of this caliber (Perfecto et al., 2019), along with significant disruption to the island’s weak infrastructure and the lives of vulnerable communities (Morris et al., 2018; Kwasinski et al., 2019; Orengo-Aguayo et al., 2019; Ma and Smith, 2020; Yabe et al., 2020). Interviews with farmers also revealed a devastating loss of the coffee harvest, severely damaged coffee plants, and damage to most other crops as well (Perfecto et al., 2019), including those intended for local consumption and distribution. With widespread devastation to social, economic, and ecological systems in Puerto Rico, the damage inflicted by Hurricane Maria underscores the link between biodiversity conservation, ecosystem services, and food security within the coffee agroforestry system.
In this study, we leveraged a variety of compiled data from Puerto Rico at the island and farm levels to identify trade-offs and synergies in the agroforestry system compared to conventional production. At the island level, we analyzed data on coffee yield and planted area under shade or sun management. At the farm level, we analyzed continuous management variables (percent shade cover, maximum canopy height, percent ground cover, and food crop richness), ecosystem services variables (total farm carbon storage, soil organic carbon storage, coffee plant carbon biomass, and hurricane resistance and resilience [determined by NDVI]), and biodiversity variables (ant, bird, and lizard abundance). At the regional scale, we predicted that farms classified as sun coffee operations would produce higher coffee yields than the more shaded, agroforestry coffee systems. At the local scale, however, we predicted that more shaded, agriculturally diverse coffee farms would be more strongly associated with ecosystem service provisioning (e.g., hurricane resistance and recovery, carbon storage) and biodiversity (e.g., anole lizards, birds, and ants). We discuss the implications of producing Puerto Rican coffee with agroforestry practices and highlight opportunities for maximizing biodiversity, ecosystem services, and food security based on our findings. Additional understanding of the associated tradeoffs and synergies in this system generated by our study will help inform further development of agroforestry management strategies that support both ecosystem functioning and local livelihoods.
Materials and methods
Study area
Our study was conducted in Puerto Rico with regional data (from the Caribbean Climate Hub, US Department of Agriculture)1 spanning most of the island’s geography and local data collection concentrated in the central coffee-producing region on the central and western part of the Cordillera Central. Average annual temperature and precipitation within the region range from 22 to 26°C, and from 904 to 2,439 mm, respectively. According to the Web Soil Survey (NRCS, 2005), soil taxonomy is predominantly Ultisol with some farms under Inceptisol and Oxisol classification. Following the Holdridge Life Zones classification, the study area includes subtropical moist forest, subtropical wet forest, and lower montane wet forest. Coffee farms in this study included a range of management strategies from more conventional (i.e., no shade trees or low density of shade trees) to more agroecological (i.e., medium to high density of shade trees) and produced coffee as their primary crop (mostly Coffea arabica but some Coffea canephora). Many of the farms also produce plantain (Musa x paradisiaca L.) and orange [Citrus sinensis (L.) Osbeck]. According to the USDA 2017 Census of Agriculture (USDA-NASS, 2020), 76 percent of the farms are family-owned and with sizes of 7 ha or less.
Data sources
In this study, two data sets were used with analysis divided into regional and local levels because production data was not available at the local scale. For regional-scale coffee yields (island-wide), we used the USDA Caribbean Climate Hub data set (see below). For local-scale vegetation and carbon storage metrics, we used data collected for 68 farms within a 100 m2 plot (10 m × 10 m). Locations for the 100 m2 plots were selected by speaking to farmers directly about their land and walking the extensive trail systems to get a sense of the abundance and distribution of features. Plots were established in locations qualitatively determined to be representative of the overall farm. From those 68 farms, we selected 25 farms throughout the coffee production region of Puerto Rico (effectively from the municipality of Orocovis in the center to Las Marias in the west). These 25 farms were selected as representative of the management types, based on shade cover and geographic position, and sampled for the biodiversity of ants and anole lizards. Bird data was obtained from a separate data set of bird surveys conducted on 67 farms in the same region from which the 68 farms were sampled, though not necessarily on all those same farms. We matched the first and last names of the farmers from the bird data set to unique regional codes recorded in the vegetation and carbon estimate data which allowed us to merge bird survey data for 46 farms into a compiled data set that included all of our other variables (Figure 1).
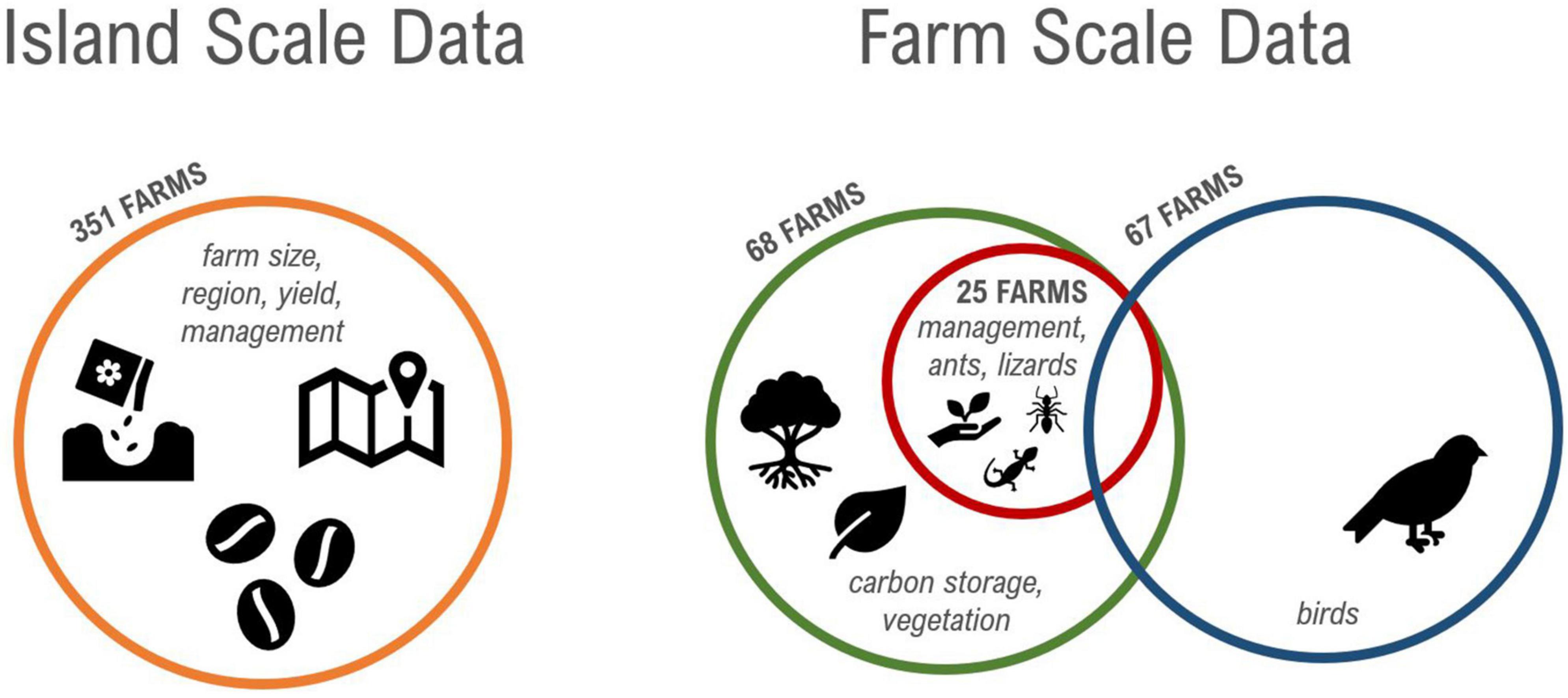
Figure 1. Graphic showing overlap of datasets used in this study. Island scale data came from the USDA dataset of 352 coffee farms across Puerto Rico and included data only on farm size, region, yield, and management (discreet measures). Farm scale data was pieced together from three existing datasets from different studies and included data on carbon storage, vegetation, ants, lizards, birds, and management (continuous measures). These datasets overlapped for some farms, but not all.
Regional data
Island-wide coffee production data (n = 351) was obtained from the USDA Caribbean Climate Hub (2016) based on surveys with farmers and included: municipality, scientific name (Coffea spp.), management type (a binary classification: shade or sun-grown), production (kg), the area planted with coffee (ha), harvested area (ha), and geographic point locations for the most recent year available (2016) (Figure 2). Yield was calculated by dividing values for coffee production (kg) by area harvested (ha) from the Climate Hub dataset.
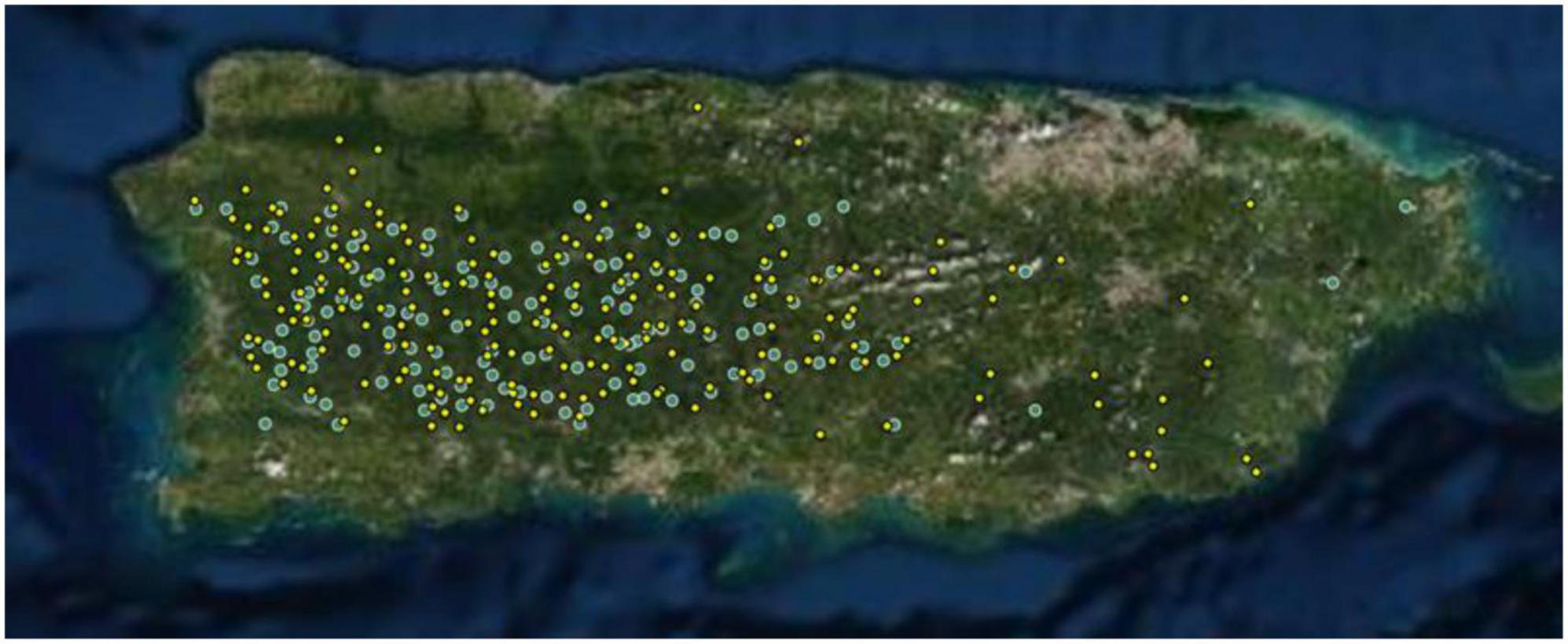
Figure 2. Spatial distribution of shaded (green circles) and unshaded (yellow circles) coffee farms in Puerto Rico from the Caribbean Climate Hub (n = 351).
Regional data statistical analysis
Island-level yield data were analyzed in R version 1.3.1093 (R Core Team, 2020). Instances of 0 kg harvest on a non-zero harvested area (N = 58) were omitted as well as the top 3 yield outliers of the square-root transformed normally distributed dataset (z-score > 3). We ran the linear mixed model analysis using the lme4 package version 1.1-26 (Bates et al., 2014) including coffee yield as the dependent variable and added fixed effects of planted area and sun or shade management. We included the region in which farms were located as a random effect. The model specification was as follows: Yield (ha) ∼ Planted Area (ha) + Management Type (sun or shade) + (1| Farm Region). Significance was calculated using the lmerTest package (Kunzetsova et al., 2017), which applies Satterthwaite’s method to estimate degrees of freedom and generate p-values for mixed models.
Local data
Ant sampling
Ant sampling was conducted in the 25 farms selected for this study. During December 2018 and January 2019, we visited each of the farms and placed five tuna fish baits directly on the stem (or stems) of each of the 20 coffee plants selected randomly from the 10 m × 10 m plot, which was chosen to reflect the basic management style of the farm. Thus, we placed a total of 100 arboreal baits in a representative area of 100 m2 on each of 25 farms, waited for 40 mins, and then checked each bait for ants, recording the species present at each bait (no counts of numbers of foragers). For further ant sampling details, see Perfecto and Vandermeer (2020b).
Lizard sampling
Lizards were sampled in the same 25 farms and the same 20 randomly selected plants where the ants were sampled. Sampling took place monthly from May 2019 to July 2019. Two observers visited each coffee plant, slowly approaching the bush from opposite sides, and spent 2 mins carefully searching all branches for lizards. The numbers and identities of the lizards observed were recorded for each plant.
Bird surveys
From the original 68 farms used to conduct the vegetation survey to estimate carbon storage (see below), 58 farms were selected as representative of the habitat types, based on shade cover and geographic position. Three avian surveys were conducted at each of the 58 farms between March and June 2018. This period encompassed the breeding season of avian resident species in Puerto Rico (Gleffe et al., 2006), thereby minimizing changes in detectability throughout the season (Thompson, 2002). Birds were surveyed using fixed-radius point counts in which two observers recorded all bird species detected visually or by sound, within 50 m of the center of the station for 10 mins (for a more detailed methodology see Irizarry et al., 2021). Though the survey points were not conducted within the exact 100 m2 plots used for the rest of the data collection, the first and last names of farmers were used to match survey locations to farm sites representing the same general area. All surveys were conducted between 0600 and 1000 h under favorable weather conditions.
Vegetation data and farm management
Vegetation data were obtained for the same 25 farms and 100 m2 plots used for the ant and lizard sampling. Farm vegetation was assessed between February and August 2018: about 5 months after Category 4 hurricanes Irma and Maria made landfall in September 2017. The 100 m2 plots were established to avoid lot edges and ensure representation of the general farm management. Respective GPS coordinates were recorded at the center of each plot. All trees, coffee shrubs, and Musa plants with a diameter at breast height (DBH, measured 1.3 m above the ground) > 3 cm were measured and identified. When trees could not be identified in the field, samples were taken for identification in the laboratory.
Percent canopy cover was measured using the application Canopy App (University of New Hampshire, 2014; Brush et al., 2017; Barreiros, 2019; Perfecto and Vandermeer, 2020a) and averaging five measurements: one at the center of the plot and each of the four plot corners. Given that sites were selected to be characteristic of overall farm management, we used the average shade across the plot to be representative of the shade from tree canopies (excluding coffee plants) on the farm overall. Maximum canopy height was recorded in each plot and ground cover was estimated for the farm by randomly placing a 1 m × 1 m square at five different locations (center and four corners) in the 100 m2 plot and recording the percentage of bare ground. Food crop richness was also surveyed within each 100 m2 plot based on the number of crops, including fruit trees, that were recorded from each plot.
Hurricane damage and recovery rate
To quantify the impact of the hurricanes and the recovery from them at the farm scale, we used the NDVI pre and post-hurricane to create a variety of metrics (Figure 3A). Post-hurricane NDVI was calculated in Google Earth Engine (Gorelick et al., 2017) from the USGS Landsat 8 Surface Reflectance Image Collection by first applying a 1 km buffer around the center point of the 100 m2 plots within the 25 representative farms for which vegetation, ant, and lizard surveys were conducted. A cloud mask was applied to the images by searching through the points where the “Pixel_qa” band (a pixel quality assessment band provided by the Surface Reflectance image collection) in Landsat had a corresponding value of 322, which indicated clear terrain and low confidence cloud and cirrus interference.
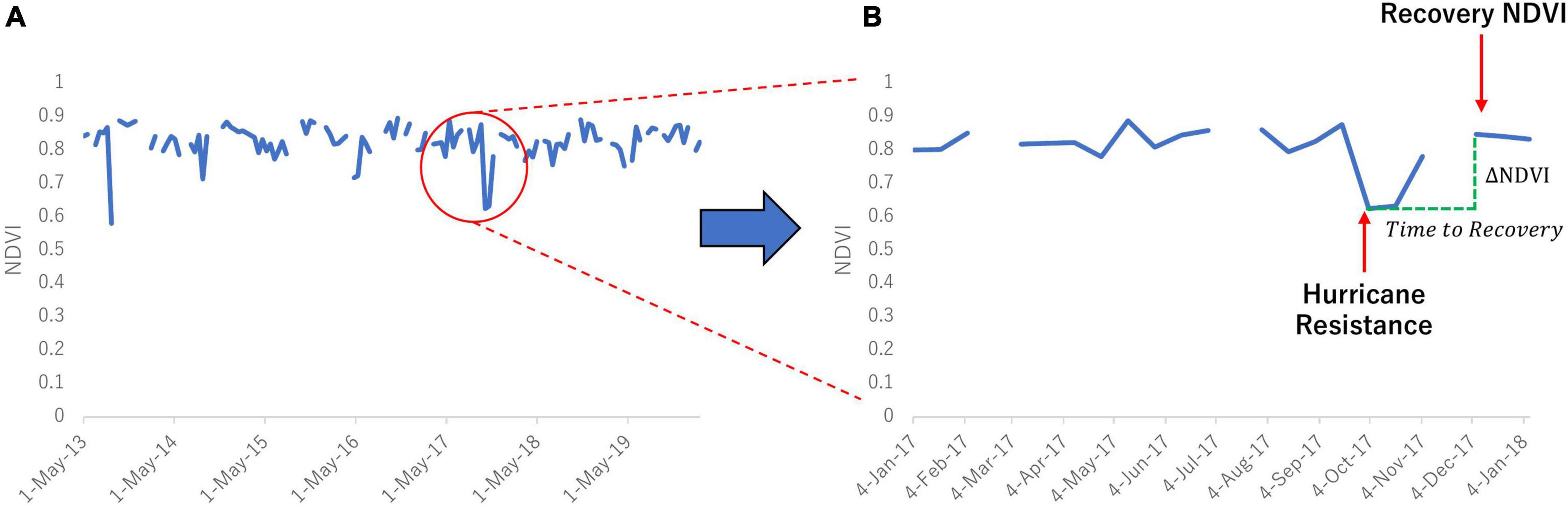
Figure 3. (A) Mean NDVI time series from 25 farms in Puerto Rico from 2013 to 2019. (B) Mean NDVI time series in the year that Hurricane Maria hit the island, showing the drop in NDVI.
For the hurricane impact analysis, we defined five variables: Hurricane Resistance, Recovery NDVI, Time to Recovery, Recovery Velocity, and ΔNDVI (Figure 3B). In step with Hu and Smith (2018), analysis of damage from Hurricane Maria, we interpreted the differences between pre-and post-hurricane NDVI values to indicate damage to a farm associated with Hurricane Maria. The lowest NDVI value following Hurricane Maria’s landfall in Puerto Rico between September and October 2017 was selected as the “Hurricane Resistance” variable. Conceptually, Hurricane Resistance captures the deviation from pre-hurricane NDVI levels and indicates the ability of a given farm to withstand or resist, hurricane damage. Therefore, a lower Hurricane Resistance value suggests that the farm can better resist hurricane damage, while a higher value suggests that the farm is more susceptible to hurricane damage. In the NDVI time series chart, we observed that NDVI dropped immediately following the hurricane, then gradually increased back up to a higher value (Recovery NDVI) before resuming seasonal fluctuation patterns. The Time to Recovery variable was defined as the period between the lowest NDVI value and the Recovery NDVI (highest NDVI value post-hurricane). Because the Landsat 8 satellite has a visiting frequency of 16 days, Time to Recovery values were discretized to 48, 64, and 80 days. ΔNDVI was defined as the difference between Recovery NDVI and the lowest NDVI value. Recovery velocity was defined as 30ΔNDVI/timeto. The multiplication factor of 30 was included to convert the units from (NDVI)/day to (NDVI)/month. Conceptually, Recovery Velocity captures hurricane resilience by measuring how quickly a given farm can repopulate green areas and return to its natural state after incurring damage from Hurricane Maria. The higher the recovery velocity, the more resilient the farm is. See Vargas de Mendonça (2021) for a repository of the code and data used in the NDVI analysis.
Carbon storage
Carbon storage data was obtained from the same study of 68 coffee farms in the central rural region of Puerto Rico. Data was collected following hurricane Maria between February and August 2018. Above and below ground carbon stocks were estimated for all citrus trees, shade trees, coffee shrubs, and banana plants within the 100 m2 plots (see Supplementary Table 1). For trees and shrubs with the main stem that forked below 1.3 m DBH (naturally or by pruning), all secondary stems were measured. Secondary stem basal areas were added to calculate individual basal area (BA). Soil organic carbon content was estimated from soil core samples taken from the same plots and added to the total above and below ground plant carbon stock estimates to calculate the total farm carbon storage. Though carbon storage in other systems is impacted by pruning of both coffee and shade trees at 5–6 and 1-year intervals, respectively, pruning in Puerto Rico is generally more infrequent for coffee and even more rare for shade trees due to the high cost of labor on the island.
Allometric equations were used to estimate aboveground biomass (AGB) and coarse root biomass (CRB). For AGB of shade trees, citrus trees, and bananas and plantains (Musa ×paradisiaca), validated models from the literature were applied (see Supplementary Table 1). To estimate AGB of coffee shrubs, an allometric model based on BA was developed using destructive sampling of 29 coffee shrubs. The coarse root biomass density (CRB) for shade trees, citrus trees, and coffee shrubs was estimated using a model developed by Cairns et al. (1997). To convert AGB and CRB into our measure of total carbon in the biomass (AGCB and CRCB, respectively), the carbon content was assumed to be 50% for woody plants (Brown, 1997) and 46% for Musa plants (Danarto and Hapsari, 2016). All C stocks were expressed in Mg C ha–1.
Local data statistical analysis
Since we did not have access to yield data for the same farms from which we collected ecosystem service and biodiversity data, we examined relationships with management variables (i.e., percent canopy cover, maximum canopy height, ground cover, and crop richness) to look for potential trade-offs and synergies. Specifically, we divided our analyses into three conceptual groups: (1) relationships among management variables, (2) management effects on ecosystem services and biodiversity, and (3) relationships among ecosystem services and biodiversity. Management effects on ecosystem services and biodiversity were analyzed through a series of linear regressions with management variables as the independent variable. We explored interactions among an informed selection of the ecosystem service and biodiversity variables through a correlation analysis that could capture significant nonlinear relationships and used non-parametric Spearman rank correlations because many of our variables were not normally distributed (see Supplementary Information for a table of results). Given that this study synthesized data from multiple studies, using a generalized linear mixed model approach was not feasible due to the low overlap in farm data for the explanatory variables of interest, hence our use of correlations.
Results
Yield and agroforestry versus conventional production at the regional level
In our linear mixed model that incorporated the area planted with coffee, management type did not have a significant effect on coffee yields (p = 0.165, df = 237). Additionally, planted area arose as a significant predictor of yield (p = 0.094, df = 234) suggesting that there was no clear relationship between yield and management type after accounting for regional variation and farm size.
Management variables, ecosystem services, and biodiversity at the local level
Relationships among management variables
Among the four management variables we analyzed (percent canopy cover, maximum canopy height, ground cover, and food crop richness), two significant associations arose (Figure 4). Percent canopy cover from shade trees was positively associated with food crop richness (r = 0.289, p = 0.015), including bananas, tubers, citrus, peppers, plantains, pumpkin, legumes, and sweet potatoes in addition to coffee, indicating a possible synergy between agroforestry management and farm-level food production. Food crop richness within a single 100 m2 plot varied from 0 to 3 different crops (excluding coffee) for all farms surveyed for carbon storage. Percent canopy cover was negatively associated with percent ground cover (r = −0.235, p = 0.025). However, ground cover was a problematic variable given that it could be driven by direct management practices (i.e., weeding, herbicide application) or an associated effect of management (i.e., a very dense canopy cover).
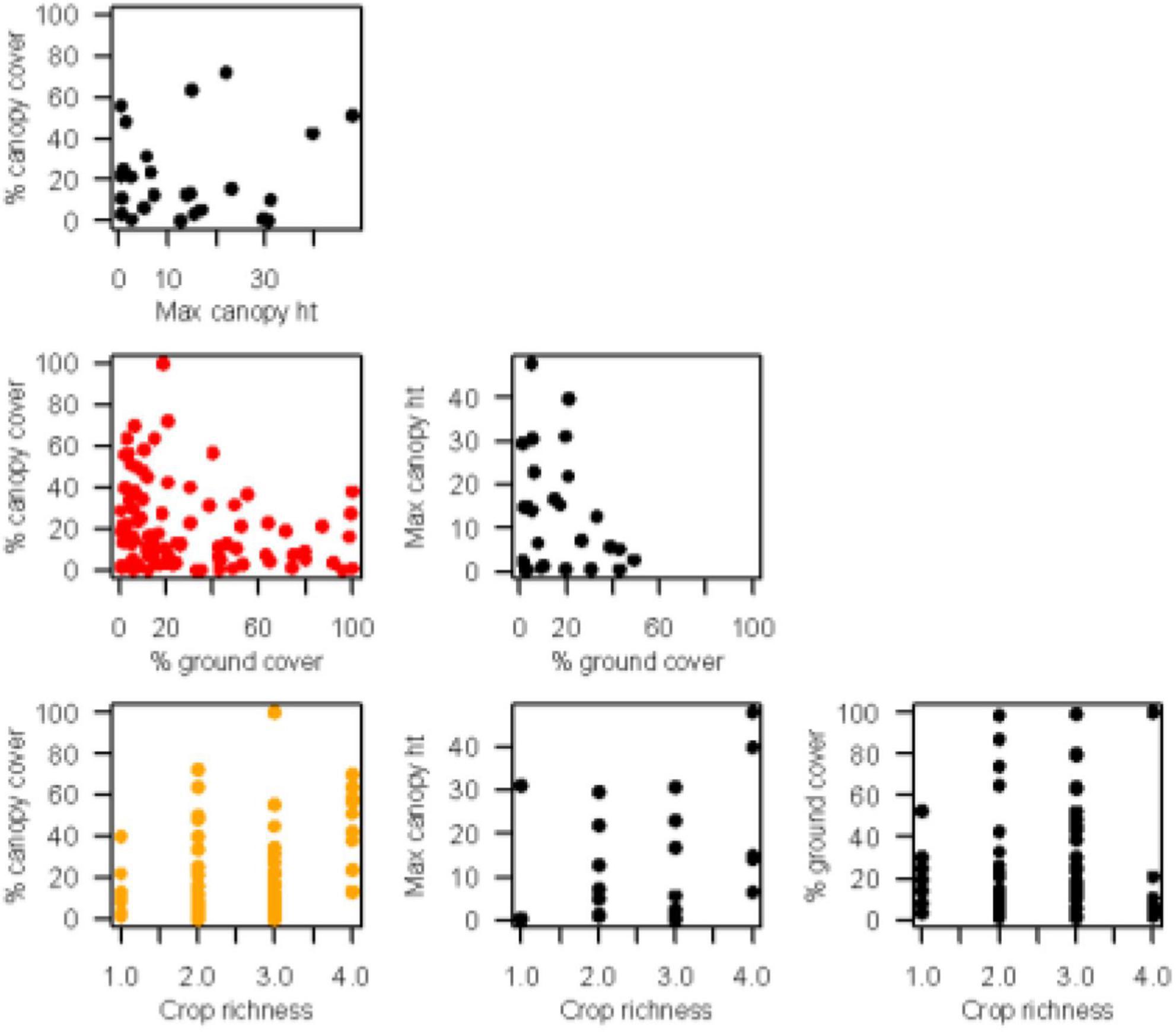
Figure 4. Shows the Spearman Rank correlations for management variables considered in the coffee system studied. Black data points indicate non-significant relationships, yellow data points indicate a positive significant relationship, and red data points indicate a significant negative relationship (p < 0.05).
Management effects on ecosystem services and biodiversity
Our results suggest that agroforestry management variables (i.e., percent canopy cover, maximum canopy height, ground cover, and food crop richness) had mostly positive effects on ecosystem services (summarized in Figures 5, 6). Higher percent canopy cover was associated with higher total coffee plant carbon storage (R2 = 0.138, p = 0.001), total farm carbon storage (R2 = 0.140, p = 0.001), and hurricane resistance (R2 = 0.201, p = 0.021). Higher maximum canopy height was also positively associated with total farm carbon storage (R2 = 0.250, p = 0.009). While more ground cover did provide soil carbon storage benefits (R2 = 0.104, p = 0.006), it was negatively associated with hurricane resistance (R2 = 0.194, p = 0.024), though this could be partially explained by the negative relationship between shade and ground cover, therefore indicating that shaded areas were able to better resist against hurricane damages. Finally, food crop richness was positively associated with higher hurricane resilience (quicker recovery to pre-hurricane NDVI values) (R2 = 0.243, p = 0.016).
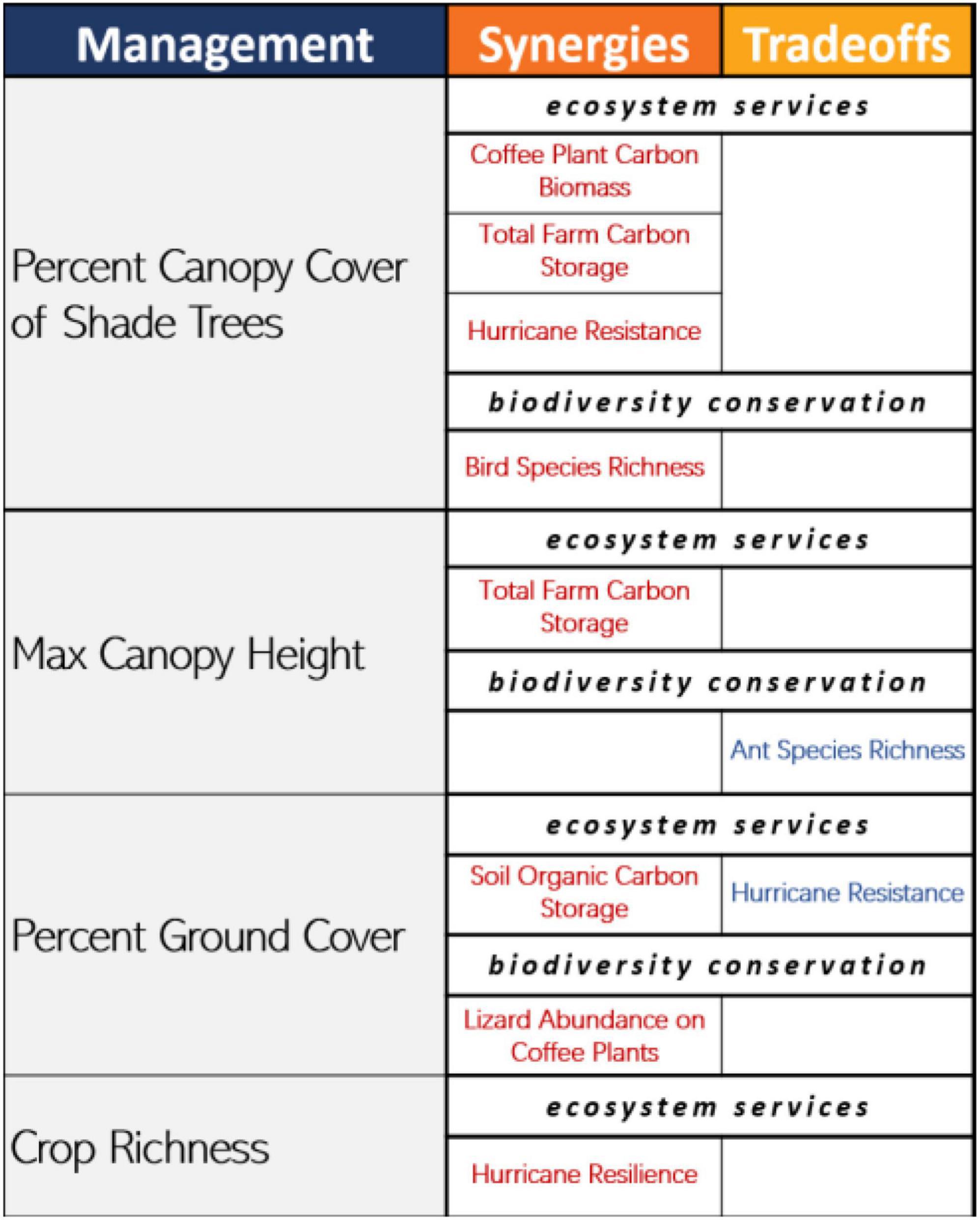
Figure 5. Summary table of management effects on ecosystem services and biodiversity. Red indicates a positive relationship and blue indicates a negative relationship. This panel contains a scatter plot matrix of the correlations condensed in this table.
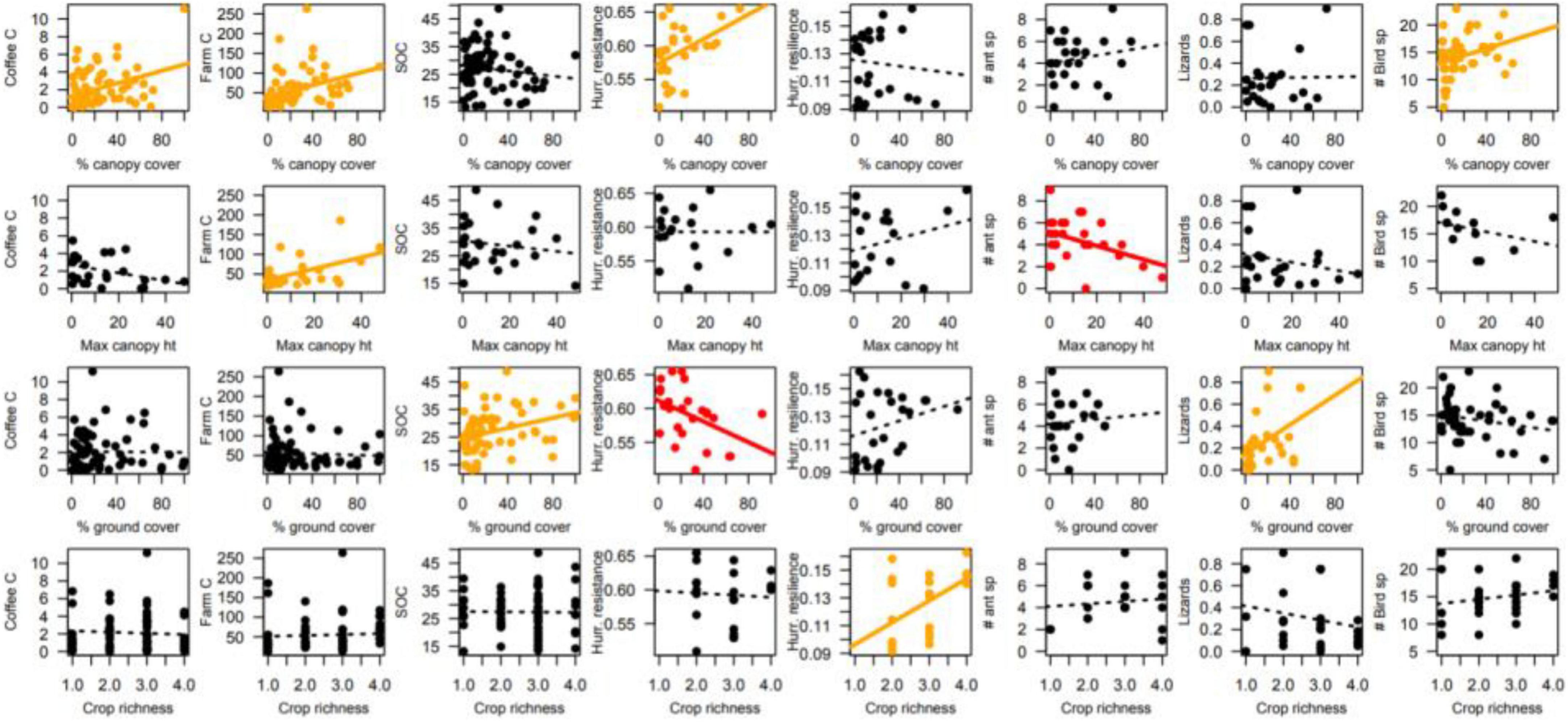
Figure 6. Scatter plots for all of the management effects on ecosystem service and biodiversity conservation studied. Black indicates a non-significant relationship, red indicates a negative significant relationship, and yellow indicates a positive significant relationship (p < 0.05).
Management practice metrics including percent shade canopy cover, maximum canopy height, and percent ground cover had clear positive effects on some biodiversity variables with only one potential tradeoff. Bird species richness tended to be higher in farms with higher percent shade cover (R2 = 0.094, p = 0.035). Notably, many of the tropical bird species observed on the farms were species endemic to Puerto Rico including the Puerto Rican Emerald (Riccordia maugeaus), Puerto Rican Flycatcher (Myiarchus antillarum), Puerto Rican Lizard-Cuckoo (Coccyzus vieilloti), Puerto Rican Oriole (Icterus portoricensis), Puerto Rican Spindalis (Spindalis portoricensis), Puerto Rican Tody (Todus mexicanus), Puerto Rican Tanager (Nesospingus speculiferus), Puerto Rican Vireo (Vireo latimeri), Puerto Rican Woodpecker (Melanerpes portoricensis), and the Puerto Rican Bullfinch (Loxigilla portoricensis). Anole abundance tended to be higher on farms with more ground cover (R2 = 0.177, p = 0.031). Ant species richness observed on coffee plants decreased with higher shade canopies (R2 = 0.165, p = 0.039) which could be considered a tradeoff, but the effect on individual species of invasive ants (i.e., Wasmannia auropunctata, Solenopsis invicta, Monomorium floricola, and Tapinoma melanocephalum) was mixed.
Relationships among ecosystem services and biodiversity conservation
Among the ecosystem service and biodiversity variables we analyzed, several statistically significant synergies arose with no evidence of tradeoffs (Figure 7). Total farm carbon storage was positively related to both soil organic carbon storage (r = 0.341, p = 0.003) and total coffee plant carbon storage (r = 0.330, p = 0.004) which was not surprising given the contribution of soil carbon and coffee plant carbon to the calculation of overall farm-level carbon storage. Conversely, the positive association between SOC and coffee plant carbon biomass (r = 0.239, p = 0.04425), two distinct metrics, is ecologically meaningful and suggestive of synergy between ecosystem services and robust coffee bushes that may produce favorable yields. Interestingly, preliminary data exploration did not indicate a negative relationship between coffee plant carbon biomass and shade tree carbon biomass which could imply a lack of appreciable competition for light and water. Total farm carbon storage was also positively associated with hurricane resilience, a metric that indicates how fast the farm recovered after the hurricane impact (r = 0.435, p = 0.037), suggesting that cultivated areas with more woody vegetation tended to re-establish more quickly following Hurricane Maria. Evidence of a crossover synergy between ecosystem services and biodiversity was supported by the positive association of soil organic carbon and lizard abundance on individual coffee plants (r = 0.443, p = 0.018).
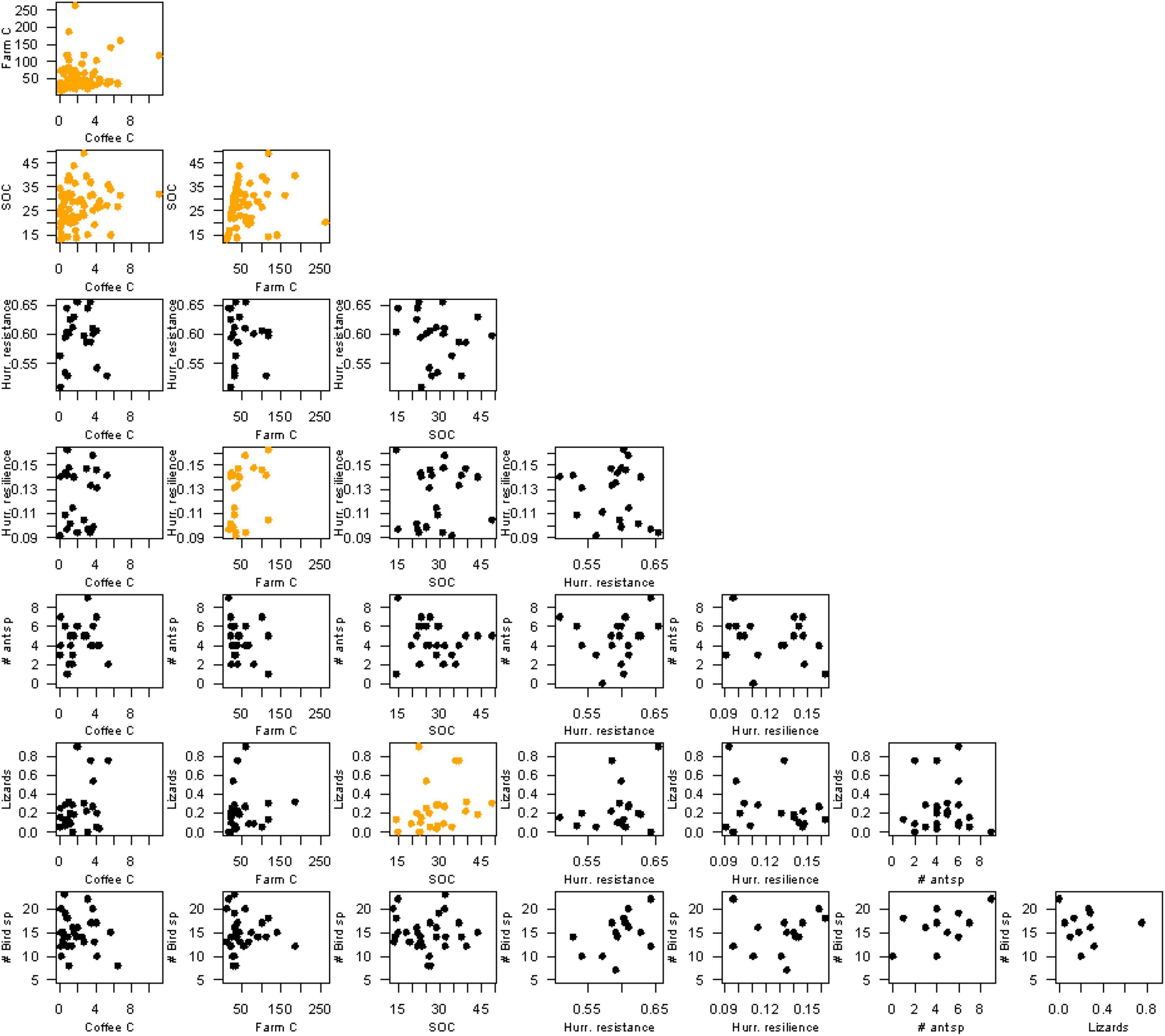
Figure 7. Scatter plots for all of the ecosystem service and biodiversity conservation variables in the coffee system studied. Black data points indicate non-significant relationships and yellow data points indicate a positive significant relationship (p < 0.05).
Discussion
Relationship between coffee yield and agroforestry versus conventional production at the regional level
At the regional scale, there was no obvious tradeoff in yield between agroforestry and conventional coffee production. Even though the farms in our study spanned a large geographic area subject to regional microclimates and varied in operational size from around 240 ha to less than one ha, we found no significant difference between the yields for sun and shade coffee. This is in contrast to previous studies such as a meta-analysis of 26 locations by Jezeer et al. (2017) which reported lower yields in shaded agroforestry coffee production compared to conventionally produced coffee, despite higher cost-efficiency. Though we could not directly analyze trends between shade level and yield per hectare without a continuous measure of shade, the literature suggests there may be a positive effect on yield at intermediate levels of shade (Elevitch et al., 2009; Piato et al., 2020) or that some of the variation may be driven by other factors such as coffee plant density (Wang et al., 2015). Growing coffee in a shaded agroforestry environment may also increase the quality which can have a substantial net benefit for the price (Bote and Struik, 2011), especially when coupled with certifications such as Bird Friendly, Rainforest Alliance, Fair Trade, or other economic incentives that directly benefit farmers (Perfecto et al., 2005; Philpott et al., 2007). For example, Iverson et al. (2019) propose a multifunctional approach specific to coffee farms in Puerto Rico that combines certification premiums with carbon payments to increase the profitability of more biodiverse farms. Without a clear tradeoff in yield at the regional scale, our results also provide local-scale evidence of benefits to biodiversity conservation and ecosystem services associated with higher shade cover that could be incorporated into economic policies supportive of local livelihoods.
Local synergies between management, ecosystem services, and biodiversity conservation
Our study illustrates synergies between shade cover and a variety of ecosystem services and biodiversity variables such as bird richness, carbon storage in soil and plant biomass, and resistance to hurricane damage with no evidence of tradeoffs among our ecosystem service and biodiversity conservation measures. At the local scale, we found that farms with more shade cover and higher shade tree canopies (continuous measures of agroforestry management), were associated with more biodiversity and ecosystem service provisioning. Coffee bushes under more dense shade tree canopies tended to have greater biomass both above and below ground, similar to findings by Campanha et al. (2004), suggesting no obvious tradeoff with coffee plant growth and perhaps even yield with certain shade tree intercroppings (Boreux et al., 2016). This idea is supported by research from Soto-Pinto et al. (2000) who reported an increase in coffee yield up to 38% shade cover and no effect of shade on yield up to 50% shade. Our study also identified a synergy between soil organic carbon storage and coffee plant carbon storage that could be driven by a combination of higher microbial activity, more nutrient release in the soil, and greater water infiltration contributing to higher soil quality, as Cannavo et al. (2011) and de Souza et al. (2012) reported for coffee agroforestry systems in Brazil and Costa Rica. This could enable larger coffee plants to grow and potentially contribute to higher yields or different flavor profiles, though the relationship between coffee plant size, bean quality, and yield depends on how resources are allocated within the plant (Steiman et al., 2011; Bote and Jan, 2016). With the high cost of labor limiting the pruning of shade trees in Puerto Rico, coffee plants under agroforestry management may have the capacity to follow these trends of greater yield at intermediate shade levels and benefits to bean quality at high shade levels.
Though our island-wide data showed no relationship between management type and yield, a relationship between yield and shade may have been detected with a continuous measure of shade or another measure of productivity such as yield per coffee plant. The synergy between agroforestry management and biodiversity conservation was most apparent for birds in our study (many endemic to Puerto Rico), with more shade cover being associated with higher bird species richness as other studies have reported (Philpott and Bichier, 2012; Buechley et al., 2015), though we did not examine community composition. Our study also detected a positive relationship between percent ground cover and lizard abundance which could be the result of lizards using herbaceous vegetation in the coffee farms for cover from predators. In another study conducted in Puerto Rico, Monagan et al. (2017) reported a negative relationship between coffee intensification and lizard abundance, with higher lizard abundance in shade coffee farms compared to sun coffee farms. We did not find such a relationship. On the contrary, our study found more lizards in farms with more ground cover, which was correlated with lower shade cover. It is possible, however, that increased ground cover from low-lying vegetation found in the less shaded farms facilitated lizard movement between coffee plants thereby increasing their detected abundance.
The synergies between shade, ground cover, and carbon storage reported in our study highlight the opportunity for agroforestry management to play a role in climate change mitigation efforts that seek agents for carbon storage, including plant and soil biomass. Additional synergies may also be realized by policies that prioritize land with high biodiversity value, such as the more shaded farms in our study, which offer higher carbon storage benefits than conventional systems (Reside et al., 2017; Middendorp et al., 2018). Agroforestry management of coffee farms, therefore, could support efforts to mitigate climate change through carbon sequestration while bolstering the stability of coffee production in the face of a warming climate by including shade trees that minimize heat stress and reduce high-velocity winds from hurricanes for which the risk is greatest during the harvest season (Lin, 2007; de Souza et al., 2012; Rice, 2018). The benefits of agroforestry management to long-term coffee production and farmer livelihoods are further compounded by the synergies between shade cover and resistance to hurricane damage and that of food crop richness and hurricane resilience. Regional studies have identified land cover type, terrain, slope orientation, and vegetation complexity as significant determinants of hurricane damage severity that could be mediated by agroforestry management practices such as planting shade trees to slow hurricane winds and protect lower strata coffee bushes (Philpott et al., 2008; Hu and Smith, 2018; Mariño et al., 2018). Our analysis suggests that more shaded farms suffered less damage from Hurricane Maria’s impact and farms growing a variety of crops recovered faster. Considering the contribution of percent canopy cover to lower annual soil erosion rates in other systems (Zuazo and Pleguezuelo, 2009), agroforestry presents dual benefits for coffee farming in Puerto Rico, including higher resistance to hurricanes and lower damage due to erosion. Factors such as tree size, root depth, cohesion, and mean tensile root strength supported by management decisions to include shade trees or additional crops may offer opportunities for maximizing soil conservation and protection against hurricanes (De Baets et al., 2008; Ali, 2010; Hwang et al., 2015).
Local tradeoffs between management, ecosystem services, and biodiversity conservation
Our analysis identified only three negative relationships: between percent canopy cover and percent ground cover; percent ground cover and hurricane resistance; and maximum canopy height and ant richness. The negative relationship between percent canopy cover and ground cover is not surprising since heavier shade can help regulate the herbaceous vegetation in the understory. Indeed, weed control is frequently cited as one of the benefits of shade cover in coffee agroforestry systems (Staver et al., 2001, 2020; Soto-Pinto et al., 2002). In this study, we included ground cover as a management variable that indirectly measures the control of weeds by either chemical or mechanical methods because of the heavy use of herbicides among Puerto Rican coffee farmers (informal conversations with farmers). Despite that, we detected a negative relationship between percent canopy cover and percent ground cover, suggesting that ground cover is a result of the shade level rather than an indirect measurement of weed management. We also found that hurricane resistance decreased with ground cover, however, this could simply be a reflection of the negative relationship between shade and ground cover. Since farms that had higher shade levels also had higher hurricane resistance and lower ground cover, it follows that we would detect a negative relationship between ground cover and hurricane resistance.
The decrease in ant richness with taller shade canopies could also be considered an ecosystem service tradeoff partially explained by the dominance of the invasive “fire ant” Wasmannia auropunctata, which disrupts farmworkers with its painful bite and is commonly observed on citrus trees intercropped with coffee plants. Though Wasmannia are a known biocontrol agent of the coffee berry borer and the coffee leaf miner, both prolific pests in Puerto Rico, they have also been found to deter Anole lizards, another generalist pest predator, with their bite (Perfecto and Vandermeer, 2020a; Perfecto et al., 2021). It is not fully understood how Wasmannia interacts with other species in the ant community, such as Solenopsis invicta, and how those interactions are mediated by management practices such as agroforestry (Perfecto and Vandermeer, 2020b), but our results indicated that less shaded farms with more groundcover had more Anoles. Therefore, though our findings suggest a tradeoff between ant species richness and shade, future research on the interactions between biocontrol agents and coffee pests in Puerto Rico could inform low-input management strategies that minimize the effect of the coffee berry borer and leaf miner while supporting biodiversity. Due to the lack of yield data for the farms, we surveyed for ants, lizards, birds, and vegetation, we were not able to examine direct relationships between yield and our biodiversity and ecosystem service measures. Future studies could collect this data through relatively simple questionnaires that could be combined with other tools such as the Household Dietary Diversity Survey (Swindale and Bilinsky, 2006) to explore further linkages between management, yield, and food security.
Food security and agroforestry management
Our study also illustrated relationships that may indicate potential synergies between agroforestry management and food security in Puerto Rico. Though we did not include direct metrics of food security, we believe that smallholder food crop production is an important dimension of the food security discussion. Furthermore, reporting on food crops in coffee farms challenges the false perception among many agroecologists and food security advocates in Puerto Rico that coffee production does not contribute at all to food production since coffee is not a food crop and may take productive land away from growing food. Within the farms included in our study, we found higher food crop richness, including bananas, tubers, citrus, peppers, plantains, pumpkin, legumes, and peas in coffee farms with more shade cover suggesting that agroforestry management may also be a means to provide households with local food resources while supporting local biodiversity. This idea is consistent with Frison et al. (2011) and Jha et al. (2014) who argue that agrobiodiversity resulting from management strategies such as agroforestry, can provide sustainable social, political, and economic benefits to food security while delivering “agricultural intensification without simplification.” Informal surveys of walking trails within farms (data not included in this article) revealed a great diversity of fruit trees and food crops such as guava, breadfruit, coconut, mango, loquat, soursop, avocado, and star apple which may supplement the diets of farmers and their families, though further research is needed to quantify the nutritional value of local production and understand its role in household consumption, as some studies have already done in other farming communities (Remans et al., 2011; Blesh and Wittman, 2015; Valencia et al., 2019; Stratton et al., 2020). In addition, it is important to consider that fruits and tubers comprise much of the non-coffee crops grown on the farms in this study, so additional subsistence would need to be purchased or planted to ensure a complete diet that meets micro-and macronutrient needs. Nevertheless, our results support the idea that coffee farms under agroforestry management do have the potential to reduce some level of food insecurity in farming households and provide fresh, potentially organic produce at a low cost. Given that some farms in our study produced up to 12 food crops in addition to coffee, opportunities also exist to leverage the spatial analysis power of Geographic Information Systems (GIS) software to map out potential distribution pathways for small-scale production of food crops on the island. This, combined with qualitative interviews with farmers, could build on the literature connecting agroforestry farming practices to food sovereignty in agricultural communities.
Conclusion
In sum, our analyses present exploratory insights into the synergies and tradeoffs between agroforestry management, biodiversity conservation, and ecosystem services at regional and local scales of coffee production in Puerto Rico. Though several of the associations we report for management, ecosystem service, and biodiversity data are limited to correlations and relationships between measured proxies, we intend to provide a basis for future, more direct studies of the complex, interconnected processes in this system that may be less restricted by the availability of existing data. In our study, we found few tradeoffs and several synergies resulting from shaded coffee production, including benefits to carbon storage, resistance and resilience to hurricane damage, and bird and lizard conservation. At the island level, we found no evidence of a significant tradeoff in yield between shade and sun-managed coffee farms after isolating the effects of planted area and region. Among ecosystem services and biodiversity conservation, we identified synergies among carbon storage sinks, between total farm carbon storage and hurricane resilience, and between pest-controlling lizard abundance and soil organic carbon storage with no evidence of tradeoffs. Though the explained variance in our analysis remained relatively low in many cases, the significant results (p < 0.05) we report illustrate broadly associations with plausible mechanisms for which we encourage further exploration of biodiversity and ecosystem services in agroforestry systems. Finally, we discussed the advantages of agroforestry management to household and regional food security and highlighted opportunities and the need for future research in supporting areas such as food sovereignty.
Data availability statement
Publicly available datasets were analyzed in this study. This data can be found here: https://caribbeanclimatehub.org/estadisticasagricolas/.
Author contributions
IM and IP conceived of the research framework and question. IM and JV reviewed relevant literature for the manuscript. IM, JV, JL-P, and ZH-F synthesized the data and produced figures based on the analyses with feedback from IM and IP. All authors discussed the results and contributed to the final manuscript.
Funding
This research was supported by USDA grant numbers, NIFA/USDA 2017-67019-26292 and NIFA/USDA 2018-67030-28239.
Acknowledgments
We are grateful to the many farmers in Puerto Rico for participating in this study and allowing us to visit their farms. We thank S. Brines and I. Peng from the Puerto Rico Drone Project team in the Environmental Spatial Analysis Lab at the University of Michigan for sharing their resources and support with the remote sensing analysis, A. White in the Department of Ecology and Evolutionary Biology for commentary and discussion on food security in Puerto Rico, and Amarilys Irizarry, Isa Marie Acosta, Koralis Reyes, and Warren Irizarry for help establishing plots in the 25 farms and collecting field data. Landsat-8 imagery was provided courtesy of the U.S. Geological Survey.
Conflict of interest
The authors declare that the research was conducted in the absence of any commercial or financial relationships that could be construed as a potential conflict of interest.
Publisher’s note
All claims expressed in this article are solely those of the authors and do not necessarily represent those of their affiliated organizations, or those of the publisher, the editors and the reviewers. Any product that may be evaluated in this article, or claim that may be made by its manufacturer, is not guaranteed or endorsed by the publisher.
Supplementary material
The Supplementary Material for this article can be found online at: https://www.frontiersin.org/articles/10.3389/ffgc.2022.690164/full#supplementary-material
Footnotes
References
Ali, F. (2010). Use of vegetation for slope protection: Root mechanical properties of some tropical plants. Int. J. Phys. Sci. 5, 496–506.
Avelino, J., Vilchez, S., Segura-Escobar, M. B., Brenes-Loaiza, M. A., Virginio Filho, E. D. M., and Casanoves, F. (2020). Shade tree Chloroleucon eurycyclum promotes coffee leaf rust by reducing uredospore wash-off by rain. Crop Prot. 129:105038. doi: 10.1016/j.cropro.2019.105038
Barreiros, J. L. (2019). Efeito do Manejo Florestal Sustentável Sobre a Deposição de Serapilheira em uma Floresta de Terra Firme na Amazônia brasileira [Effect of Sustainable Forest Management on Litter Deposition in a Terra Firme Forest in the Brazilian Amazon] (Dissertation). Manaus: Universidade do Estado do Amazonas.
Bates, D., Maechler, M., Bolker, B., and Walker, S. (2014). Ime4: Linear Mixed-Effects Moels Using Eigen and S4. R Package Version 1.0.-6.
Beilhe, L. B., Roudine, S., Perez, J. A. Q., Allinne, C., Daout, D., Mauxion, R., et al. (2020). Pest-regulating networks of the coffee berry borer (Hypothenemus hampei) in agroforestry systems. Crop Prot. 131:105036. doi: 10.1016/j.cropro.2019.105036
Blesh, J., and Wittman, H. (2015). “Brasilience:” assessing resilience in land reform settlements in the Brazilian Cerrado. Hum. Ecol. 43, 531–546. doi: 10.1007/s10745-015-9770-0
Boreux, V., Vaast, P., Madappa, L. P., Cheppudira, K. G., Garcia, C., and Ghazoul, J. (2016). Agroforestry coffee production increased by native shade trees, irrigation, and liming. Agron. Sustain. Dev. 36:42. doi: 10.1007/s13593-016-0377-7
Bos, M. M., Höhn, P., Saleh, S., Büche, B., Buchori, D., Steffan-Dewenter, I., et al. (2007). “Insect diversity responses to forest conversion and agroforestry management,” in Stability of Tropical Rainforest Margins, eds T. Tscharntke, C. Leuschner, M. Zeller, E. Guhardja, and A. Bidin (Berlin: Springer), 277–294. doi: 10.1007/978-3-540-30290-2_14
Bote, A. D., and Jan, V. (2016). Branch growth dynamics, photosynthesis, yield and bean size distribution in response to fruit load manipulation in coffee trees. Trees 30, 1275–1285. doi: 10.1007/s00468-016-1365-x
Bote, A. D., and Struik, P. C. (2011). Effects of shade on growth, production and quality of coffee (Coffea arabica) in Ethiopia. J. Horticult. For. 3, 336–341.
Brown, S. (1997). Estimating Biomass and Biomass Change of Tropical Forests: A Primer. FAO Forest Paper 134. Rome: Food and Agriculture Organization.
Brush, J. S., Racelis, A., and Brush, T. (2017). The breeding bird community of a remnant urban woodland in McAllen, Texas. Texas Ornithol. Soc. 85:8.
Buechley, E. R., Şekercioǧlu, ÇH., Atickem, A., Gebremichael, G., Ndungu, J. K., Mahamued, B. A., et al. (2015). Importance of Ethiopian shade coffee farms for forest bird conservation. Biol. Conserv. 188, 50–60. doi: 10.1016/j.biocon.2015.01.011
Cairns, M. A., Brown, S., Helmer, E. H., and Baumgardner, G. A. (1997). Root biomass allocation in the world’s upland forests. Oecologia 111, 1–11. doi: 10.1007/s004420050201
Campanha, M. M., Santos, R. H. S., De Freitas, G. B., Martinez, H. E. P., Garcia, S. L. R., and Finger, F. L. (2004). Growth and yield of coffee plants in agroforestry and monoculture systems in Minas Gerais, Brazil. Agrofor. Syst. 63, 75–82. doi: 10.1023/B:AGFO.0000049435.22512.2d
Cannavo, P., Sansoulet, J., Harmand, J. M., Siles, P., Dreyer, E., and Vaast, P. (2011). Agroforestry associating coffee and Inga densiflora results in complementarity for water uptake and decreases deep drainage in Costa Rica. Agricult. Ecosyst. Environ. 140, 1–13. doi: 10.1016/j.agee.2010.11.005
Caribbean Climate Hub (2016). Explore the Agricultural Diversity of Puerto Rico. Available online at: https://caribbeanclimatehub.org/estadisticasagricolas/ (accessed June 24, 2020).
Cely-Santos, M., and Philpott, S. M. (2019). Local and landscape habitat influences on bee diversity in agricultural landscapes in Anolaima, Colombia. J. Insect Conserv. 23, 133–146. doi: 10.1007/s10841-018-00122-w
Cerda, R., Allinne, C., Gary, C., Tixier, P., Harvey, C. A., Krolczyk, L., et al. (2017). Effects of shade, altitude and management on multiple ecosystem services in coffee agroecosystems. Eur. J. Agron. 82, 308–319. doi: 10.1016/j.eja.2016.09.019
Danarto, S. A., and Hapsari, L. (2016). Biomass and carbon stock estimation inventory of Indonesian bananas (Musa spp.) and its potential role for land rehabilitation. Biotropia 22, 102–108. doi: 10.11598/btb.2015.22.2.376
De Baets, S., Poesen, J., Reubens, B., Wemans, K., De Baerdemaeker, J., and Muys, B. (2008). Root tensile strength and root distribution of typical Mediterranean plant species and their contribution to soil shear strength. Plant Soil 305, 207–226. doi: 10.1007/s11104-008-9553-0
De Beenhouwer, M., Geeraert, L., Mertens, J., Van Geel, M., Aerts, R., Vanderhaegen, K., et al. (2016). Biodiversity and carbon storage co-benefits of coffee agroforestry across a gradient of increasing management intensity in the SW Ethiopian highlands. Agricult. Ecosyst. Environ. 222, 193–199. doi: 10.1016/j.agee.2016.02.017
de Souza, H. N., de Goede, R. G., Brussaard, L., Cardoso, I. M., Duarte, E. M., Fernandes, R. B., et al. (2012). Protective shade, tree diversity and soil properties in coffee agroforestry systems in the Atlantic Rainforest biome. Agricult. Ecosyst. Environ. 146, 179–196. doi: 10.1016/j.agee.2011.11.007
Denu, D., Platts, P. J., Kelbessa, E., Gole, T. W., and Marchant, R. (2016). The role of traditional coffee management in forest conservation and carbon storage in the Jimma Highlands, Ethiopia. For. Trees Livelihoods 25, 226–238. doi: 10.1080/14728028.2016.1192004
Diaz, I. I. (2016). Coffee Agroecology, Food Sovereignty, and Agrarian Livelihoods in Puerto Rico. Electronic Thesis and Dissertation Repository. 4229. London, ON: Western University.
Diaz, I. I., and Hunsberger, C. (2018). Can agroecological coffee be part of a food sovereignty strategy in Puerto Rico? Geoforum 97, 84–94. doi: 10.1016/j.geoforum.2018.10.016
Durand-Bessart, C., Tixier, P., Quinteros, A., Andreotti, F., Rapidel, B., Tauvel, C., et al. (2020). Analysis of interactions amongst shade trees, coffee foliar diseases and coffee yield in multistrata agroforestry systems. Crop Prot. 133:105137. doi: 10.1016/j.cropro.2020.105137
Elevitch, C. R., Idol, T., Friday, J. B., Lepczyk, C., Easton Smith, V., and Nelson, S. C. (2009). Shade-Grown Coffee for Hawaii. Holualoa: Permanent Agriculture Resources.
Elsner, J. B., Kossin, J. P., and Jagger, T. H. (2008). The increasing intensity of the strongest tropical cyclones. Nature 455, 92–95. doi: 10.1038/nature07234
Fain, S. J., Quiñones, M., Álvarez-Berríos, N. L., Parés-Ramos, I. K., and Gould, W. A. (2018). Climate change and coffee: Assessing vulnerability by modeling future climate suitability in the Caribbean island of Puerto Rico. Clim. Change 146, 175–186. doi: 10.1007/s10584-017-1949-5
Félix, G. F., and Holt-Giménez, E. (2017). Hurricane maría: An agroecological turning point for puerto rico? Food First Backgrounders Issue Briefs, 23. 1–4.
Frison, E. A., Cherfas, J., and Hodgkin, T. (2011). Agricultural biodiversity is essential for a sustainable improvement in food and nutrition security. Sustainability 3, 238–253. doi: 10.3390/su3010238
García-López, G. A. (2018). The multiple layers of environmental injustice in contexts of (un) natural disasters: The case of Puerto Rico Post-Hurricane Maria. Environ. Justice 11, 101–108. doi: 10.1089/env.2017.0045
Gleffe, J. D., Collazo, J. A., Groom, M. J., and Miranda-Castro, L. (2006). Avian reproduction and the conservation value of shaded coffee plantations. Ornitol. Neotrop. 17, 271–282.
Gorelick, N., Hancher, M., Dixon, M., Ilyushchenko, S., Thau, D., and Moore, R. (2017). Google earth engine: Planetary-scale geospatial analysis for everyone. Remote Sens. Environ. 202, 18–27. doi: 10.1016/j.rse.2017.06.031
Guillemot, J., Le Maire, G., Munishamappa, M., Charbonnier, F., and Vaast, P. (2018). Native coffee agroforestry in the Western Ghats of India maintains higher carbon storage and tree diversity compared to exotic agroforestry. Agricult. Ecosyst. Environ. 265, 461–469. doi: 10.1016/j.agee.2018.06.002
Häger, A. (2012). The effects of management and plant diversity on carbon storage in coffee agroforestry systems in Costa Rica. Agrofor. Syst. 86, 159–174. doi: 10.1007/s10457-012-9545-1
Hajian-Forooshani, Z., Gonthier, D. J., Marín, L., Iverson, A. L., and Perfecto, I. (2014). Changes in species diversity of arboreal spiders in Mexican coffee agroecosystems: Untangling the web of local and landscape influences driving diversity. PeerJ 2:e623. doi: 10.7717/peerj.623
Hajian-Forooshani, Z., Rivera Salinas, I. S., Jiménez-Soto, E., Perfecto, I., and Vandermeer, J. (2016). Impact of regionally distinct agroecosystem communities on the potential for autonomous control of the coffee leaf rust. J. Environ. Entomol. 45, 1521–1526. doi: 10.1093/ee/nvw125
Hu, T., and Smith, R. B. (2018). The impact of hurricane maria on the vegetation of dominica and puerto rico using multispectral remote sensing. Remote Sens. 10:827. doi: 10.3390/rs10060827
Hwang, T., Band, L. E., Hales, T. C., Miniat, C. F., Vose, J. M., Bolstad, P. V., et al. (2015). Simulating vegetation controls on hurricane-induced shallow landslides with a distributed ecohydrological model. J. Geophys. Res. Biogeosci. 120, 361–378. doi: 10.1002/2014JG002824
Irizarry, A. D., Collazo, J. A., Vandermeer, J., and Perfecto, I. (2021). Coffee plantations hurricanes and avian resiliency: Insights from local occupancy, colonization and extinction rates in Puerto Rico. Glob. Ecol. Conserv. 27:e01579. doi: 10.1016/j.gecco.2021.e01579
Iverson, A. L., Gonthier, D. J., Pak, D., Ennis, K. K., Burnham, R. J., Perfecto, I., et al. (2019). A multifunctional approach for achieving simultaneous biodiversity conservation and farmer livelihood in coffee agroecosystems. Biol. Conserv. 238:108179. doi: 10.1016/j.biocon.2019.07.024
Jezeer, R. E., Verweij, P. A., Santos, M. J., and Boot, R. G. (2017). Shaded coffee and cocoa–double dividend for biodiversity and small-scale farmers. Ecol. Econ. 140, 136–145. doi: 10.1016/j.ecolecon.2017.04.019
Jha, S., Bacon, C. M., Philpott, S. M., Ernesto Mendez, V., Läderach, P., and Rice, R. A. (2014). Shade coffee: Update on a disappearing refuge for biodiversity. Bioscience 64, 416–428. doi: 10.1093/biosci/biu038
Kunzetsova, A., Brockhoff, P. B., and Christensen, R. H. B. (2017). lmerTest package: Tests in linear mixed effect models. J. Stat. Softw. 82, 1–26. doi: 10.18637/jss.v082.i13
Kwasinski, A., Andrade, F., Castro-Sitiriche, M. J., and O’Neill-Carrillo, E. (2019). Hurricane maria effects on puerto rico electric power infrastructure. IEEE Power Energy Technol. Syst. J. 6, 85–94. doi: 10.1109/JPETS.2019.2900293
Lin, B. B. (2007). Agroforestry management as an adaptive strategy against potential microclimate extremes in coffee agriculture. Agric. For. Meteorol. 144, 85–94. doi: 10.1016/j.agrformet.2006.12.009
Lin, B. B. (2010). The role of agroforestry in reducing water loss through soil evaporation and crop transpiration in coffee agroecosystems. Agric. For. Meteorol. 150, 510–518. doi: 10.1016/j.agrformet.2009.11.010
López-Bravo, D. F., Virginio-Filho, E. D. M., and Avelino, J. (2012). Shade is conducive to coffee rust as compared to full sun exposure under standardized fruit load conditions. Crop Prot. 38, 21–29. doi: 10.1016/j.cropro.2012.03.011
Ma, C., and Smith, T. (2020). Vulnerability of renters and low-income households to storm damage: Evidence from Hurricane Maria in Puerto Rico. Am. J. Public Health 110, 196–202. doi: 10.2105/AJPH.2019.305438
Mariño, Y. A., García-Peña, J. M., Vega, V. J., Verle-Rodrigues, J. C., and Bayman, P. (2018). After the fall: Did coffee plants in Puerto Rico survive the 2017 hurricanes? Agricult. Ecosyst. Environ. 266, 10–16. doi: 10.1016/j.agee.2018.07.011
Middendorp, R. S., Vanacker, V., and Lambin, E. F. (2018). Impacts of shaded agroforestry management on carbon sequestration, biodiversity and farmers income in cocoa production landscapes. Landsc. Ecol. 33, 1953–1974. doi: 10.1007/s10980-018-0714-0
Monagan, Jr I. V, Morris, J. R., Davis Rabosky, A. R., Perfecto, I., and Vandermeer, J. (2017). Anolis lizards as biocontrol agents in mainland and island agroecosystems. Ecol. Evol. 7, 2193–2203. doi: 10.1002/ece3.2806
Morris, Z. A., Hayward, R. A., and Otero, Y. (2018). The political determinants of disaster risk: Assessing the unfolding aftermath of hurricane maria for people with disabilities in Puerto Rico. Environ. Justice 11, 89–94. doi: 10.1089/env.2017.0043
National Weather Service (2017). Major Hurricane Maria. Available online at: https://www.weather.gov/sju/maria2017 (accessed September 20, 2017).
NRCS (2005). USDA Web Soil Survey. Available online at: https://websoilsurvey.sc.egov.usda.gov/ (accessed June 17, 2020).
Orengo-Aguayo, R., Stewart, R. W., de Arellano, M. A., Suárez-Kindy, J. L., and Young, J. (2019). Disaster exposure and mental health among Puerto Rican youths after Hurricane Maria. JAMA Netw. Open 2:e192619. doi: 10.1001/jamanetworkopen.2019.2619
Perfecto, I., and Vandermeer, J. (2015). Coffee Agroecology: A New Approach to Understanding Agricultural Biodiversity, Ecosystem Services and Sustainable Development. Milton Park: Routledge. doi: 10.4324/9780203526712
Perfecto, I., and Vandermeer, J. (2020a). Antagonism between Anolis spp. and Wasmannia auropunctata in coffee farms on Puerto Rico: Potential complications of biological control of the coffee berry borer. Caribbean J. Sci. 50, 43–47. doi: 10.18475/cjos.v50i1.a6
Perfecto, I., and Vandermeer, J. (2020b). The assembly and importance of a novel ecosystem: The ant community of coffee farms in Puerto Rico. Ecol. Evol. 10, 12650–12662. doi: 10.1002/ece3.6785
Perfecto, I., Armbrecht, I., Philpott, S. M., Soto-Pinto, L., and Dietsch, T. V. (2007). “Shaded coffee and the stability of rainforest margins in northern Latin America,” in Stability of Tropical Rainforest Margins, eds T. Tscharntke, C. Leuschner, M. Zeller, E. Guhardja, and A. Bidin (Berlin: Springer), 225–261. doi: 10.1007/978-3-540-30290-2_12
Perfecto, I., Hajian-Forooshani, Z., Iverson, A., Irizarry, A. D., Lugo-Perez, J., Medina, N., et al. (2019). Response of coffee farms to hurricane maria: Resistance and resilience from an extreme climatic event. Sci. Rep. 9:15668. doi: 10.1038/s41598-019-51416-1
Perfecto, I., Hajian-Forooshani, Z., White, A., and Vandermeer, J. (2021). Ecological complexity and contingency: Ants and lizards affect biological control of the coffee leaf miner in Puerto Rico. Agricult. Ecosyst. Environ. 305:107104. doi: 10.1016/j.agee.2020.107104
Perfecto, I., Vandermeer, J. H., and Wright, A. L. (2009). Nature’s Matrix: Linking Agriculture, Conservation and Food Sovereignty. Milton Park: Routledge. doi: 10.4324/9781849770132
Perfecto, I., Vandermeer, J., Mas, A., and Soto Pinto, L. (2005). Biodiversity, yield, and shade coffee certification. Ecol. Econ. 54, 435–446. doi: 10.1016/j.ecolecon.2004.10.009
Philpott, S. M., and Bichier, P. (2012). Effects of shade tree removal on birds in coffee agroecosystems in Chiapas, Mexico. Agric. Ecosyst. Environ. 149, 171–180. doi: 10.1016/j.agee.2011.02.015
Philpott, S. M., Bichier, P., Rice, R., and Greenberg, R. (2007). Field-testing ecological and economic benefits of coffee certification programs. Conserv. Biol. 21, 975–985. doi: 10.1111/j.1523-1739.2007.00728.x
Philpott, S. M., Lin, B. B., Jha, S., and Brines, S. J. (2008). A multi-scale assessment of hurricane impacts on agricultural landscapes based on land use and topographic features. Agric. Ecosyst. Environ. 128, 12–20. doi: 10.1016/j.agee.2008.04.016
Philpott, S. M., Perfecto, I., and Vandermeer, J. (2006). Effects of management intensity and season on arboreal ant diversity and abundance in coffee agroecosystems. Biodivers. Conserv. 15, 139–155. doi: 10.1007/s10531-004-4247-2
Piato, K., Lefort, F., Subía, C., Caicedo, C., Calderón, D., Pico, J., et al. (2020). Effects of shade trees on robusta coffee growth, yield and quality. A meta-analysis. Agron. Sustain. Dev. 40:38. doi: 10.1007/s13593-020-00642-3
R Core Team (2020). R: A Language and Environment for Statistical Computing. Vienna: R Foundation for Statistical Computing.
Remans, R., Flynn, D. F., DeClerck, F., Diru, W., Fanzo, J., Gaynor, K., et al. (2011). Assessing nutritional diversity of cropping systems in African villages. PLoS One 6:e21235. doi: 10.1371/journal.pone.0021235
Reside, A. E., VanDerWal, J., and Moran, C. (2017). Trade-offs in carbon storage and biodiversity conservation under climate change reveal risk to endemic species. Biol. Conserv. 207, 9–16. doi: 10.1016/j.biocon.2017.01.004
Rice, R. A. (2008). Agricultural intensification within agroforestry: The case of coffee and wood products. Agricult. Ecosyst. Environ. 128, 212–218. doi: 10.1016/j.agee.2008.06.007
Rice, R. A. (2018). Coffee in the crosshairs of climate change: Agroforestry as abatis. Agroecol. Sustain. Food Syst. 42, 1058–1076. doi: 10.1080/21683565.2018.1476428
Rodríguez-Díaz, C. E. (2018). Maria in Puerto Rico: Natural disaster in a colonial archipelago. Am. J. Public Health 108, 30–32. doi: 10.2105/AJPH.2017.304198
Segura, M., Kanninen, M., and Suárez, D. (2006). Allometric models for estimating aboveground biomass of shade trees and coffee bushes grown together. Agroforestry Syst. 68, 143–150. doi: 10.1007/s10457-006-9005-x
Schroth, G., Krauss, U., Gasparotto, L., Aguilar, J. D., and Vohland, K. (2000). Pests and diseases in agroforestry systems of the humid tropics. Agrofor. Syst. 50, 199–241. doi: 10.1023/A:1006468103914
Schroth, G., D’Angelo, S.A., Teixeira, W.G., Haag, D., and Lieberei, R. (2002). Conversion of secondary forest into agroforestry and monoculture plantations in Amazonia: Consequences for biomass, litter and soil carbon stocks after 7 years. For. Ecol. Manage. 163, 131–150. doi: 10.1016/S0378-1127(01)00537-0
Soto-Pinto, L., Perfecto, I., and Caballero-Nieto, J. (2002). Shade over coffee: Its effects on berry borer, leaf rust and spontaneous herbs in Chiapas, Mexico. Agrofor. Syst. 55, 37–45. doi: 10.1023/A:1020266709570
Soto-Pinto, L., Perfecto, I., Castillo-Hernandez, J., and Caballero-Nieto, J. (2000). Shade effect on coffee production at the northern Tzeltal zone of the state of Chiapas, Mexico. Agricult. Ecosyst. Environ. 80, 61–69. doi: 10.1016/S0167-8809(00)00134-1
Staver, C., Guharay, F., Monterroso, D., and Muschler, R. G. (2001). Designing pest-suppressive multistrata perennial crop systems: Shade-grown coffee in Central America. Agrofor. Syst. 53, 151–170. doi: 10.1023/A:1013372403359
Staver, C., Juventia, S., Navarrete, E., Navarrete, L., Sepulveda, N., and Barrios, M. (2020). Long-term response of groundcover components to organic and conventional weed control in shaded and open-sun coffee in Nicaragua. Crop Prot. 133:105150. doi: 10.1016/j.cropro.2020.105150
Steiman, S., Idol, T., Bittenbender, H. C., and Gautz, L. (2011). Shade coffee in Hawai ‘i–Exploring some aspects of quality, growth, yield, and nutrition. Sci. Horticult. 128, 152–158. doi: 10.1016/j.scienta.2011.01.011
Stratton, A. E., Kuhl, L., and Blesh, J. (2020). Ecological and nutritional functions of agroecosystems as indicators of smallholder resilience. Front. Sustain. Food Syst. 4:173. doi: 10.3389/fsufs.2020.543914
Swindale, A., and Bilinsky, P. (2006). Household Dietary Diversity Score (HDDS) for Measurement of Household Food Access: Indicator Guide (V.2). Washington, DC: Food and Nutrition Technical Assistance.
Thomazini, A., Mendonça, E. S., Cardoso, I. M., and Garbin, M. L. (2015). SOC dynamics and soil quality index of agroforestry systems in the Atlantic rainforest of Brazil. Geoderma Reg. 5, 15–24. doi: 10.1016/j.geodrs.2015.02.003
Thompson, W. L. (2002). Overview towards reliable bird surveys: Accounting for individuals present but not detected. Auk 119, 18–25. doi: 10.1093/auk/119.1.18
University of New Hampshire (2014). Canopy App (Version 1.0.3) [Mobile app]. App Store. Durham, NH: University of New Hampshire.
USDA-NASS. (2020). 2017 Census of Agriculture: Puerto Rico (2018) Island and Regional Data. Washington, DC: USDA-NASS
Vaast, P., Harmand, J. M., Rapidel, B., Jagoret, P., and Deheuvels, O. (2016). “Coffee and cocoa production in Agroforestry—a climate-smart agriculture model,” in Climate change and agriculture worldwide, ed. E. Torquebiau (Dordrecht: Springer), 209–224. doi: 10.1007/978-94-017-7462-8_16
Valencia, V., Wittman, H., and Blesh, J. (2019). Structuring markets for resilient farming systems. Agron. Sustain. Dev. 39:25. doi: 10.1007/s13593-019-0572-4
Vandermeer, J., and Perfecto, I. (2019). Hysteresis and critical transitions in a coffee agroecosystem. Proc. Natl. Acad. Sci. U.S.A. 116, 15074–15079. doi: 10.1073/pnas.1902773116
Van Noordwijk, M., Rahayu, S., Hairiah, K., Wulan, Y., Farida, A., and Verbist, B. 2002. Carbon stock assessment for a forest-to-coffee conversion landscape in Sumber-Jaya (Lampung, Indonesia): From allometric equations to land use change analysis. Sci. China 45, 75–86.
Vargas de Mendonça, J. L. (2021). Post-Hurricane NDVI Analysis in Puerto Rican Coffee Farms. Available online at: https://github.com/jlvargasme/prhurricane (accessed February 7, 2021).
Wang, N., Jassogne, L., van Asten, P. J., Mukasa, D., Wanyama, I., Kagezi, G., et al. (2015). Evaluating coffee yield gaps and important biotic, abiotic, and management factors limiting coffee production in Uganda. Eur. J. Agron. 63, 1–11. doi: 10.1016/j.eja.2014.11.003
Yabe, T., Rao, P. S. C., and Ukkusuri, S. V. (2020). Regional differences in resilience of social and physical systems: Case study of puerto rico after hurricane maria. Environ. Plann. B 48, 1042–1057. doi: 10.1177/2399808320980744
Keywords: agroforestry, ecosystem services, biodiversity conservation, food security, synergies and tradeoffs, Hurricane Maria, Puerto Rico, shade coffee
Citation: Mayorga I, Vargas de Mendonça JL, Hajian-Forooshani Z, Lugo-Perez J and Perfecto I (2022) Tradeoffs and synergies among ecosystem services, biodiversity conservation, and food production in coffee agroforestry. Front. For. Glob. Change 5:690164. doi: 10.3389/ffgc.2022.690164
Received: 02 April 2021; Accepted: 26 July 2022;
Published: 18 August 2022.
Edited by:
Anthony A. Kimaro, Consultative Group on International Agricultural Research (CGIAR), United StatesReviewed by:
Sammy Carsan, World Agroforestry Centre, KenyaErwin Dreyer, INRA Centre Nancy-Lorraine, France
Meine van Noordwijk, World Agroforestry Centre (ICRAF), Indonesia
Copyright © 2022 Mayorga, Vargas de Mendonça, Hajian-Forooshani, Lugo-Perez and Perfecto. This is an open-access article distributed under the terms of the Creative Commons Attribution License (CC BY). The use, distribution or reproduction in other forums is permitted, provided the original author(s) and the copyright owner(s) are credited and that the original publication in this journal is cited, in accordance with accepted academic practice. No use, distribution or reproduction is permitted which does not comply with these terms.
*Correspondence: Isabella Mayorga, Ym1heW9yZ2FAdW1pY2guZWR1