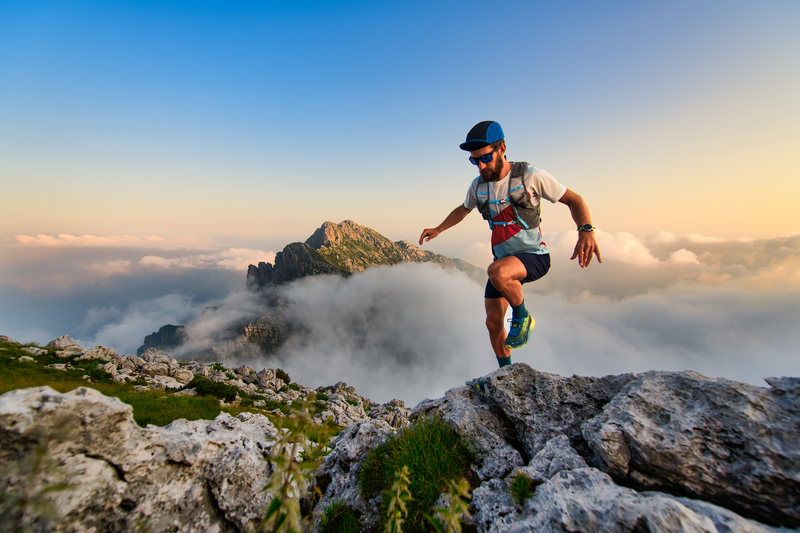
94% of researchers rate our articles as excellent or good
Learn more about the work of our research integrity team to safeguard the quality of each article we publish.
Find out more
ORIGINAL RESEARCH article
Front. For. Glob. Change , 28 October 2021
Sec. Forests and the Atmosphere
Volume 4 - 2021 | https://doi.org/10.3389/ffgc.2021.768085
This article is part of the Research Topic Forest Monitoring to Assess Forest Functioning Under Air Pollution and Climate Change View all 8 articles
The Leaf-Area-Index (LAI) is commonly used to characterize the plant canopy and is a fundamental indication of plant vitality and photosynthetic activity. The forest health status is not only vital for economical reasons, but also has a significant impact on global carbon sequestration. The LAI has a highly dynamic character among deciduous forests and is prone to significant seasonal fluctuations. Accurate continuous LAI measurements do provide valuable information on growth characteristics, but they require considerable measurement effort. In this study, we tested a novel method that would allow for continuous low-effort LAI parameterizations. For our study we used temperature measurements from 2011 to 2019 obtained at two meteorological stations: Station one is an open land station, station two is located inside a forest stand characterized by European beech (measurements were undertaken as part of the ICP Forests program), both are located in Klausen Leopoldsdorf (Austria). We chose the difference in daily maximum temperature between the two sites for our LAI parametrization (LAIpar) since the forest canopy has a significant impact on local radiation conditions. We were able to identify phenological events such as leaf unfolding, the end of leaf growth, and the beginning and end of defoliation by examining at the average course of the year for LAIpar. The resultant LAIpar values were compared to annual values derived from hemispheric photographs taken near the stand temperature sensor. For the years 2011–2017, we found a strong correlation of 0.93 between LAI measures and LAIpar, which dropped to 0.69 after adding the year 2018 and 0.32 after adding 2019. We further compared the phenological events obtained from LAIpar to phenological observations. The impact of forests on their site climate, according to our findings, can be utilized to identify phenological and growth characteristics. The proposed method, however, is not a replacement for conventional LAI measurements.
The forest canopy is an important interface between the terrestrial ecosystem and the atmosphere allowing for an exchange of energy, water, and carbon dioxide (Bonan, 1993). This interface may also be affected by climate change as previous studies have shown that the occurrence of phenological phases has shifted due to temperature changes (Chmielewski and Rötzer, 2001; Cleland et al., 2007). That being said the determination and quantification of phenological features and forest canopy extent are non-trivial and oftentimes elaborate:
Cleland et al. (2007) discussed limitations and opportunities of different methods to monitor plant phenology. The traditional method for detecting phenological phases relies on the utilization of observers (oftentimes volunteers) that register days that are associated with changes in phenological phases such as leaf unfolding during spring, bud formation, flowering, or leaf coloring and defoliation in late summer and fall. The phenological network provides conditions and thresholds to identify the various phases. One of the main problems arising when using data from phenological observations is the low spatial and temporal resolution. Phenological data can also be obtained using eddy-covariance flux measurements. As atmospheric carbon dioxide oscillates on an annual cycle, the amplitude and shift in this cycle can be used as an indicator of plant phenology. The collected data is however site-specific and in general, the setup for eddy-covariance monitoring is very expensive. Other ground and near-surface methods include digital camera sensing, spectral radiometers, and cameras carried by Unmanned Aerial Vehicles (Berra and Gaulton, 2021). The availability of reliable data is especially crucial for the evaluation and improvement of emerging, generally less complex technologies like phenology modeling and remote sensing. Phenology modeling allows not only for future estimates but also for the reconstruction of time series into the past where data is scarce. Limitations arise because the number of physiological processes described in the models is limited. Berra and Gaulton (2021) reviewed monitoring of forest phenology based on remote sensing which is oftentimes referred to as the Land-Surface-Phenology (LSP). Plant phenology shifts occur at a rate of 1 day per decade on average, however, the usual uncertainties from satellite LSP are much larger. This suggests that utilizing satellite data to track phenological changes still has considerable limitations.
The forest canopy extent can be described by numerous different parameters, with the most commonly used being the Leaf-Area-Index (LAI) which is defined as the projected area of leaves per unit of ground (Ross, 2012). LAI was shown to affect local temperature and vapor pressure deficit conditions, creating a characteristic forest microclimate (Von Arx et al., 2013). De Frenne et al. (2013) suggested that microclimatic buffering in temperature might help to decelerate a species turnover to the benefit of species from warmer regions (thermophilization) which is expected to occur due to macroclimate warming caused by climate change whilst mentioning that high closure in forest canopy might decrease the occurrence of light-demanding understory plants in forests. In a more recent approach, De Frenne et al. (2019) described the microclimatic buffering to be a potentially useful functioning to protect biodiversity. When deciding on a location and time for a field campaign, the LAI's dynamical nature must be taken into account: The main driver of spatial LAI variations between stands and years has previously been identified to be forest management, as thinning and harvesting drastically alter stand structure. Le Dantec et al. (2000). Aside from the high spatial heterogeneity, LAI is changing dramatically on various time scales. Besides year-to-year differences in the extent and natural seasonal variations especially in deciduous forests disturbances due to climatic or biotic factors can also have a major influence. Measurements of LAI are classified into direct and indirect methods. Breda (2003), Jonckheere et al. (2004), and Weiss et al. (2004) reviewed advantages and disadvantages of different measurement techniques in detail. Direct methods vary in their sampling destructiveness. Destructive methods can involve the removal of leaves from one or more model trees. Obtained LAI values are then up-scaled under the assumption of homogeneity within the stand. For a less destructive direct measurement littertraps can be placed and evaluated. The main disadvantage of direct methods is that they are in general time-consuming and labor-intensive and therefore only partially applicable for detailed long time monitoring. However, they are still needed for the calibration and validation of indirect methods. Indirect methods can be divided into contact and non-contact measurements. One commonly used indirect contact measurement is the utilization of allometric relationships between LAI and other parameters such as the sapwood area at breast height. These relationships however are not universally applicable since they are dependent on stand characteristics (Mencuccini and Grace, 1995; Le Dantec et al., 2000). An important group of non-contact indirect measurement methods are Beer's law-based optical methods such as hemispherical photographs. The setup for this method is usually a high-resolution digital camera equipped with a wide-angle fisheye lens mounted on a self-leveling system. The manually taken images are analyzed using software packages. Yan et al. (2019) described the three main issues of optical measurements to be the Leaf angle-distribution (LAD), clumping effects, and woody components. Moreover, Zheng and Moskal (2009) noted that a potential error source is the manual setting of exposure values if a camera is being used. Another issue when using photographs is that they only provide two-dimensional information. LiDAR measurements offer point cloud information, that could be used to retrieve three-dimensional data (Yan et al., 2019). It is distincted between three different LiDAR setups: terrestrial laser scanner (TLS), airborne laser scanner (ALS), and space-borne laser scanner (SLS). The latter of which is particularly relevant for generating LAI information on a global scale (Tang et al., 2014). The LAI can also be derived from satellite data utilizing vegetation reflectance properties. Typically, in that case, satellite-derived vegetation indices such as the normalized difference vegetation index (NDVI) (Rouse et al., 1974) or the enhanced vegetation index (EVI) (Huete et al., 2002) are being used to compute LAI estimates. Potithep et al. (2013) stated that seasonal changes are the most likely source of uncertainty in broadleaf forests and that it still requires in situ data of not just LAI but also vegetation indices to improve satellite-based LAI estimates. They further compared the determined LAI values to in situ measurements using NDVI and EVI data from MODIS images and discovered that EVI estimates produced better outcomes, particularly during the leaf growth phase. Non-green leaves cause mistakes in both VIs (from the saturation to the leaf fall period).
The objective of this study is to examine the modification of temperature inside a beech stand in comparison to open land conditions and analyze to which extent microclimatic differences can be used to quantify the status of the forest canopy. The presented method provides an LAI parametrization on a day-to-day basis which allows depicting seasonal changes. Furthermore, year-to-year differences in the occurrence and duration of phenological phases can be assessed. As stand data used in this study is collected as part of the ICP forests monitoring program similar time-series data are available in several European countries. Replicating the analysis might not only allow for detailed comparisons between different regions but moreover, results could be used as a reference when analyzing phenological phases determined using remote sensing technology. The novelty of this method is the generation of detailed phenological data on an operational basis.
This study focuses on a beech stand (Fagus sylvatica L.) in Klausen-Leopoldsdorf (Austria, 48°07′16″N 16°02′52″E, 510 msl) during the years 2011–2019. This site is a core plot of the Level II Monitoring initiative (de Vries et al., 2003; Neumann and Kindermann, 2016). Level II monitoring is an initiative of the ICP Forests program which started in 1995. In Austria 16 intensive observation areas were established. Six of which were selected as core plots, where additional parameters are being measured. Among others, the core plots supply data on meteorological conditions inside the forest stand. We used daily maximum temperature (TF) inside the forest stand which was measured in agreement with the programme standards using a temperature sensor placed 2 meters above ground at measurement intervals of 15 min.
As a reference for open land conditions we used daily maximum temperature (TR) provided by the Austrian National Weather Service (ZAMG). The station is part of the national weather monitoring service (TAWES) and located in open land also in Klausen-Leopoldsdorf in proximity to the Level II site (48°03′6.84″N 16°00′2.159″E, 389msl). Measurements are recorded in an interval of 10 min.
An overview of both stations and the research area can be found in Figure 1, maximum temperature characteristics for both stations can be found in Table 1. It is important to note that the lapse rate between the two stations has not been taken into account since it has no significant influence on the results and findings of our study.
Figure 1. Overview of the research area. Red markers showcase the position of the two measurement sites.
For the determination of LAI values we used hemispherical photographs which were also conducted as part of the ICP Forests program. The photographs were taken in the near vicinity of stand temperature measurements. To get more representative LAI estimations, photographs were taken at 16 different photographic points in a 10 × 10 meter grid. We used a Canon EOS 500 D camera fixed on a 1.3 m tripod which was leveled and aligned with the magnetic North. We used the programme WinSCANOPY 2009a with the recommended settings to analyze images. Photographs were obtained once a year between the 3 and 29. August. The obtained LAI (LAIhem) was on average at 1.96 with an overall standard deviation of 0.33. The maximum LAIhem of 3.41 was found in 2012 where the standard deviation between the photographic points was with 0.41 also at maximum. The minimum value of 1.26 was found in 2011. The standard deviation between the photographic points was lowest in 2012 with a value of 0.08.
Phenological observations of leaf unfolding and leaf fall for Fagus sylvatica L. were also used for comparison. Since no data from fixed observation points was available we calculated the median day of observation in an area stretching in West-East direction from 14°45′57.9492″O to 17°9′38, 7″O and stretching in North-South direction from 48°30′35.334″N to 46°59′12.0084″N. Observations were provided by the ZAMG and obtained as part of the PhenoWatch programme (Templ et al., 2018). The point in time when at least three parts of the observed tree leaves have completely unfolded and in their final form (but not size) was classified as leaf unfolding. Leaf fall was defined as the point at which roughly half of the crown had defoliated.
For this analysis Python version 3.7 was used (Van Rossum and Drake, 2009) in addition with the packages numpy (Harris et al., 2020), pandas (Wes McKinney, 2010; pandas development team, 2020) and scipy (Virtanen et al., 2020) for data analysis as well as matplotlib (Hunter, 2007) and seaborn (Waskom, 2021) for visualization.
Forest canopy closing affects stand radiation conditions. As a result, the daily maximum temperature, which is highly dependent on incoming radiation, changes. To quantify this effect, we calculated the difference in daily maximum temperature inside the stand (TF) and at the reference site (TR) as DMAX:
To achieve an LAI parametrization that is consistent with the expected foliage course in deciduous forests (low closure during the winter, increased during the spring, maximum closure during the summer, and decrease at the end of the growing season), we implemented a sign convention and defined the parameterized LAI (LAIpar) as:
In order to study the average seasonal variation of LAIpar and compare the obtained curve with the phenological observations of leaf unfolding and leaf fall, the median of LAIpar was calculated for each individual day of the year over all available years. In addition, Gompertz function was used to further explore foliage features, particularly during the growth and defoliation phases:
[y, LAIpar normalized between −1 and 1; t, time (expressed as day of the year) normalized between -1 and 1; A, upper asymptote; β, x-axis placement parameter; κ, rate of change of shape (Rossi et al., 2003).] To determine the parameters A,β and κ the curve_ fit function implemented in the scipy package was used which fits a function based on non-linear least squares. To determine the inflection point (tip) the second derivative has to be set equal to zero, leading to:
and thus it can be found where tip = β/κ (Rossi et al., 2006). This analysis was performed for two growth phases individually: leaf growth (timespan day 1–day 240) and defoliation (timespan day 160–day 366). The timespans were chosen so that each one contains the transition from one constant state to another constant state which is essential for the Gompertz Fit (for the leaf growth phase from no foliage to maximum foliage, for the defoliation phase from maximum foliage to no foliage).
In order to further investigate diminished heating inside the forest stand we normed DMAX using TR:
As described above, we calculated and plotted the median course of the year. For our analysis, we mainly focused on DMAXN during summertime, since the moderating effect of forest cover is especially of interest during the warm season.
Contrary to the LAIpar values that are available once a day, LAIhem values are obtained only once a year. In order to extract one LAIpar value for annual comparison, we decided on using the LAIpar values obtained at the exact days that the hemispheric photographs were taken. This was preferred over using weekly or monthly averages, as it is important to compare LAIpar at similar radiation conditions (Preferably no rain and fair weather conditions) which should be the case on the days the hemispheric photographs were taken. To allow a reasonable comparison between LAIpar (that is essentially a temperature difference) and LAIhem we standardized both annual timeseries using:
Where in this case x represents the individual measurement, xmean the mean value and xstd the standard deviation of x calculated from the total time series. We observed an extreme drop in performance when we included data from the year 2019 where an outlier in LAIpar occurred. Hence, we decided to include plots and statistics for the time spans 2011–2017, 2011–2018, and 2011–2019 for comparisons.
The median course of the year for DMAX can be found in the Supplementary Material (Supplementary Figure S1). The plot was sectioned into two different foliage phases: The "Maximum foliage phase" ranging from 8.June to 27.August (160–day 240) and the "No foliage phase" ranging from the 20.November to the 4.April (325–day 95). This process is relatively subjective and only gives approximate dates since it was based solely on the form of the curve. The median value of DMAX is at −2.55°C with a standard deviation of 0.89°C. The median during the no foliage phase in late autumn, winter and early spring is at −1.92°C with a standard deviation of 0.27°C. At the maximum foliage phase the median is at −3.86°C with a standard deviation of 0.33°C. The median course of the year for LAIpar can be found in Figure 2. Due to the definition of this parameter standard deviation values are the same as for DMAX, median values differ only in sign. The fit determined by applying the gompertz function to LAIpar values including measurement values can be seen in Figure 3, the function parameters can be found in Table 2. Characteristic days namely the day of maximum leaf growth (day 135) and maximum defoliation (day 289) were marked by vertical lines in the plot. LAIpar values and characteristic days for individual years were calculated and plotted. They can be found in the Supplementary Figure S2, Table S1 (for the years 2011–2015) respectively Supplementary Figure S3 (for the years 2016-2019).
Figure 3. Gompertz function for LAIpar to determine the point in time of maximum leaf growth and maximum defoliation (vertical black lines).
During the measurement period the median value for DMAXN is at −0, 17 with a variance of 0, 42. During the no foliage phase a median of −0, 35 with a variance of 1, 05 was obtained. It is important to note that high variation during this phase can partly be caused by maximum temperature approaching 0°C on some days. During the maximum foliage phase the median of DMAXN remained relatively constant at –0,15 (with a variance of 0.0002). The constancy during the maximum foliage phase is especially of intereset, since formula 5 can be rearranged to:
Formula 7 emphasizes that DMAXN describes the effective moderating effect the forest canopy has on forest maximum temperatures. When determining DMAXN for the maximum foliage phase of individual years, minimal variation was found ranging from –0,17 (2016) to –0,12 (2017). Detailed averages and variances for the maximum foliage phase in each individual year can be found in Table 3. Because the value of DMAXN during the maximum foliage phase showed minimal variation over the whole measurement period and variation was low between individual years, we tested if we could use formula 7 to approximate TF using TR and a constant DMAXN of –0,15. A comparison of observed and approximated TF values for the maximum foliage phase can be found in Figure 4.
A comparison between standardized LAIhem and LAIpar values can be found in Figure 5. Boxplots were used to depict the variation of LAIhem for the different photographic points. The comparison of annual values can be found in Table 4. To further investigate the influence of the year 2019 three scatter plots were created, showing the relationship between annual average LAIhem and LAIpar for the timespans 2011–2017, 2011–2018, and 2011–2019, which can be found in Figure 6. Corresponding regression parameters as well as calculated Spearman correlations can be found in Table 5. Spearman correlation between LAIhem and LAIpar was very strong obtaining a value of 0.93 with a p-value of 0.006 during the time span 2011–2017. A moderate correlation of 0.69 with a p-value of 0.021 was found during 2011–2018. During 2011-2019 correlation became negligible obtaining a value of 0.32 with a p-value of 0.757.
Figure 5. Comparison of LAIhem and LAIpar values throughout the years. Boxplots show the distribution of LAIhem values obtained at all 16 photographic points. Both parameters were standardized to allow a simpler comparison.
By analyzing the median course of the year for LAIpar (Figure 2) we were able to identify two constant foliage phases: The “Maximum foliage phase” during which the forest canopy is fully developed and no defoliation has yet taken place and the “No foliage phase” for which the constant low temperature difference can be interpreted as the blocking of solar radiation by the branches when defoliation is completed. Comparing the timing of the end of the no foliage phase with observed leaf unfolding showed a delay, with the no foliage phase ending on day 95 and the observed leaf unfolding being marked as day 108. However, this was expected as leaf unfolding was defined within the PhenoWatch program as the day where at least three parts of the crown leaves have already unfolded and have their final shapes and LAIpar will potentially also react to smaller disturbances such as bud formation and unrolling. For leaf fall a similar problem arises. As the observed leaf fall is at day 280 whereas the no foliage phase starts 45 days later. Per definition, this observation occurs when at least 50% of the crown is defoliated. However, the remaining crown is still blocking radiation and therefore affects DMAX, respectively, LAIpar. This could be the reason why leaf fall was observed before the beginning of the no foliage phase. The R2 for the Gompertz function fit was at 0.68 during the leaf growth phase and at 0.63 for the defoliation phase. We compared the occurrence of the main phenological events as well as duration of phenological phases determined using the presented method to the results of previous studies on Fagus sylvatica L. foliage throughout the year:
• Global radiation profiles used by Wang et al. (2004), obtained in a stand in Hesse, France throughout the years 1996–2001.
• Moderate Resolution Imaging Spectrometer (MODIS) used by Wang et al. (2005) in a stand in Hesse, France throughout the years 2001–2003.
• Hemispherical photographs and visual assesments of bud development used by Bequet et al. (2011) in 10 European beech stands distributed in Flanders Belgium in 2008 for a detailed analysis of leaf development phases.
A detailed comparison of characteristic days and duration of different foliage phases obtained via the four methods can be found in Table 6. Since the data sets come from very different climatic regions and from different years, this comparison is only used to check if characteristic days determined using the presented method are plausible. The determined day for the end of the no foliage phase using the LAIpar method is prior to the day determined using hemispherical photographs or radiation profiles and subsequent to the day determined using MODIS. Wang et al. (2005) argued that an early onset date for MODIS is found because it detects the onset of greens rather than a species specific event. It can not be ruled out that greening has an effect on the local temperature conditions and thereby also on LAIpar however the magnitude of this influence is unclear. The start of the maximum foliage phase was late in comparison to the day determined using radiation profiles and early in comparison to the day obtained using hemispheric photographs. The start of the no foliage phase was approximately the same as determined by MODIS. Considering the high variation of the determined phenological events between the different methods, the presented LAIpar method leads to plausible results. Bigger differences occurred when comparing the duration of specific phases. Where duration of the leaf growth phase was remarkably longer than duration obtained using the radiation model and duration of the maximum foliage phase remarkably shorter. Overall our findings suggest that LAIpar can be used to determine when considerable microclimatic changes are arising from foliage change within a deciduous forest.
Table 6. Comparison of foliage course of the year for Fagus sylvatica L. using the presented LAIpar method, radiation profiles (Wang et al., 2004), MODIS (Wang et al., 2005), and hemispheric photographs (Bequet et al., 2011).
We found the relationship between the observed and approximated TF to be highly significant and concluded that DMAXN can provide insight into stand-specific microclimate conditions and can be used to highlight the recreational function of forest land during summer. As an example, a hypothetical temperature prediction of 35°C for open land corresponds to a lower maximum temperature by 5, 25°C inside the stand. For individual years this reduction would range between 4.2°C (2017) and 5.95°C (2016).
Diminished daytime heating inside forest stands is of particular interest in regions where climate predictions suggest that the frequency of heat days and heatwaves is increasing. DMAXN is presumed to vary depending on stand characteristics such as forest type, dominant species, altitude, and slope, which are known to have a considerable impact on temperature moderation inside forest stands (Renaud and Rebetez, 2009; Ferrez et al., 2011; Renaud et al., 2011).
Correlations were very strong during the time span 2011–2017. During the timespan 2011–2018 correlation was moderate. We identified a few possible reasons for the decline in correlation when including the year 2019 to our analysis:
• Inhomogeneities in the radiation conditions. Since we evaluated the temperature difference at the exact day the hemispheric photographs were taken it is possible that radiation conditions varied between the years. Aggregating over more days by building averages for 1 week, month or within the maximum foliage phase however does not necessary lead to a more robust parametrization since radiation conditions can differ drastically.
• Spatial inhomogeneities in comparison to the area where LAIhem is measured. Temperature and LAIhem are both point measurements, however through temperature mixing the LAIpar contains not only information on the forest canopy status directly above the measurements but moreover within a nearby region (depending on local conditions such as wind).
• Malfunctioning of the stand temperature sensor. Since measurements malfunctioned after the 22nd of September in 2019 issues with the temperature sensor inside the stand could cause the extreme low value of LAIpar.
Since LAIhem measurements are only available annually and the period of the analysis is limited to seven years, caution should be exercised when interpreting the results of this section. On the one hand, the correlations during 2011–2017 and 2011–2018 seem to be promising, on the other hand, the drop in correlation when adding data from 2019 cannot be disregarded. To assess how well LAIpar is suitable as an LAI parameterization, the collection of more LAI data is needed, preferably also during different foliage phases. Our results suggest that LAIpar cannot replace conventional LAI methods but can provide interesting insights on stand foliage and microclimate conditions.
The raw data supporting the conclusions of this article will be made available by the authors, without undue reservation.
AZ and SS designed the study. AZ analyzed the data. KG provided stand data to the study. GS provided data of phenological observations to the study. All authors contributed to the article and approved the submitted version.
The authors declare that the research was conducted in the absence of any commercial or financial relationships that could be construed as a potential conflict of interest.
All claims expressed in this article are solely those of the authors and do not necessarily represent those of their affiliated organizations, or those of the publisher, the editors and the reviewers. Any product that may be evaluated in this article, or claim that may be made by its manufacturer, is not guaranteed or endorsed by the publisher.
We would like to thank Michael Schellmann Ing. for taking the hemispheric photographs and Gerald Schnabel for evaluating the photoaphs and extracting the corresponding LAIhem values. Additionally we would like to thank Ferdinand Kristfel the former coordinater of the ICP Forests programme in Austria.
The Supplementary Material for this article can be found online at: https://www.frontiersin.org/articles/10.3389/ffgc.2021.768085/full#supplementary-material
DMAX, difference in daily maximum temperature; DMAXN, normed difference in daily maximum temperature; ICP Forests, International Co-operative Program on Assessment and Monitoring of Air Pollution Effects on Forests; LAI, Leaf-Area-Index; LAIhem, Leaf-Area-Index obtained using hemispherical photographs; LAIpar, Leaf-Area-Index obtained using the difference in maximum temperature; TF, daily maximum temperature inside the stand; TR, daily maximum temperature at the reference station.
Bequet, R., Campioli, M., Kint, V., Vansteenkiste, D., Muys, B., and Ceulemans, R. (2011). Leaf area index development in temperate oak and beech forests is driven by stand characteristics and weather conditions. Trees 25, 935–946. doi: 10.1007/s00468-011-0568-4
Berra, E. F., and Gaulton, R. (2021). Remote sensing of temperate and boreal forest phenology: a review of progress, challenges and opportunities in the intercomparison of in-situ and satellite phenological metrics. For. Ecol. Manage. 480:118663. doi: 10.1016/j.foreco.2020.118663
Bonan, G. B. (1993). Importance of leaf area index and forest type when estimating photosynthesis in boreal forests. Remote Sens. Environ. 43, 303–314. doi: 10.1016/0034-4257(93)90072-6
Breda, N. J. (2003). Ground-based measurements of leaf area index: a review of methods, instruments and current controversies. J. Exp. Bot. 54, 2403–2417. doi: 10.1093/jxb/erg263
Chmielewski, F.-M., and Rötzer, T. (2001). Response of tree phenology to climate change across europe. Agric. For. Meteorol. 108, 101–112. doi: 10.1016/S0168-1923(01)00233-7
Cleland, E. E., Chuine, I., Menzel, A., Mooney, H. A., and Schwartz, M. D. (2007). Shifting plant phenology in response to global change. Trends Ecol. Evolut. 22, 357–365. doi: 10.1016/j.tree.2007.04.003
De Frenne, P., Rodríguez-Sánchez, F., Coomes, D. A., Baeten, L., Verstraeten, G., Vellend, M., et al. (2013). Microclimate moderates plant responses to macroclimate warming. Proc. Natl. Acad. Sci. U.S.A. 110, 18561–18565. doi: 10.1073/pnas.1311190110
De Frenne, P., Zellweger, F., Rodriguez-Sanchez, F., Scheffers, B. R., Hylander, K., Luoto, M., et al. (2019). Global buffering of temperatures under forest canopies. Nat. Ecol. Evolut. 3, 744–749. doi: 10.1038/s41559-019-0842-1
de Vries, W., Vel, E., Reinds, G. J., Deelstra, H., Klap, J. M., Leeters, E. E. J. M., et al. (2003). Intensive monitoring of forest ecosystems in Europe: 1. Objectives, set-up and evaluation strategy. For. Ecol. Manage. 174, 77–95. doi: 10.1016/S0378-1127(02)00029-4
Ferrez, J., Davison, A., and Rebetez, M. (2011). Extreme temperature analysis under forest cover compared to an open field. Agric. For. Meteorol. 151, 992–1001. doi: 10.1016/j.agrformet.2011.03.005
Harris, C. R., Millman, K. J., van der Walt, S. J., Gommers, R., Virtanen, P., Cournapeau, D., et al. (2020). Array programming with NumPy. Nature 585, 357–362. doi: 10.1038/s41586-020-2649-2
Huete, A., Didan, K., Miura, T., Rodriguez, E. P., Gao, X., and Ferreira, L. G. (2002). Overview of the radiometric and biophysical performance of the modis vegetation indices. Remote Sens. Environ. 83, 195–213. doi: 10.1016/S0034-4257(02)00096-2
Hunter, J. D. (2007). Matplotlib: a 2d graphics environment. Comput. Sci. Eng. 9, 90–95. doi: 10.1109/MCSE.2007.55
Jonckheere, I., Fleck, S., Nackaerts, K., Muys, B., Coppin, P., Weiss, M., et al. (2004). Review of methods for in situ leaf area index determination: part i. theories, sensors and hemispherical photography. Agric. For. Meteorol. 121, 19–35. doi: 10.1016/j.agrformet.2003.08.027
Le Dantec, V., Dufrêne, E., and Saugier, B. (2000). Interannual and spatial variation in maximum leaf area index of temperate deciduous stands. For. Ecol. Manage. 134, 71–81. doi: 10.1016/S0378-1127(99)00246-7
McKinney, W. (2010). “Data structures for statistical computing in python,” in Proceedings of the 9th Python in Science Conference, eds S. van der Walt and J. Millman (Austin, TX: SciPy), 56–61.
Mencuccini, M., and Grace, J. (1995). Climate influences the leaf area/sapwood area ratio in scots pine. Tree Physiol. 15, 1–10. doi: 10.1093/treephys/15.1.1
Neumann, M., and Kindermann, G. (2016). Waldzustandsmonitoring in sterreich 20 Jahre Intensivbeobachtungsflchen (Level II). Vienna: BFW.
Pandas Development Team, T. (2020). pandas-dev/pandas: pandas. Available online at: https://pandas.pydata.org/about/citing.html
Potithep, S., Nagai, S., Nasahara, K. N., Muraoka, H., and Suzuki, R. (2013). Two separate periods of the lai-vis relationships using in situ measurements in a deciduous broadleaf forest. Agric. For. Meteorol. 169, 148–155. doi: 10.1016/j.agrformet.2012.09.003
Renaud, V., Innes, J., Dobbertin, M., and Rebetez, M. (2011). Comparison between open-site and below-canopy climatic conditions in switzerland for different types of forests over 10 years (1998- 2007). Theor. Appl. Climatol. 105, 119–127. doi: 10.1007/s00704-010-0361-0
Renaud, V., and Rebetez, M. (2009). Comparison between open-site and below-canopy climatic conditions in switzerland during the exceptionally hot summer of 2003. Agric. For. Meteorol. 149, 873–880. doi: 10.1016/j.agrformet.2008.11.006
Ross, J. (2012). The Radiation Regime and Architecture of Plant Stands, Vol. 3. The Hague: Springer Science & Business Media.
Rossi, S., Deslauriers, A., Anfodillo, T., Morin, H., Saracino, A., Motta, R., et al. (2006). Conifers in cold environments synchronize maximum growth rate of tree-ring formation with day length. New Phytol. 170, 301–310. doi: 10.1111/j.1469-8137.2006.01660.x
Rossi, S., Deslauriers, A., and Morin, H. (2003). Application of the gompertz equation for the study of xylem cell development. Dendrochronologia 21, 33–39. doi: 10.1078/1125-7865-00034
Rouse, J. W., Haas, R. H., Schell, J. A., Deering, D. W., et al. (1974). Monitoring Vegetation Systems in the Great Plains With Erts. Washington, DC: NASA Special Publication.
Tang, H., Brolly, M., Zhao, F., Strahler, A. H., Schaaf, C. L., Ganguly, S., et al. (2014). Deriving and validating leaf area index (lai) at multiple spatial scales through lidar remote sensing: a case study in sierra national forest, ca. Remote Sens. Environ. 143:131–141. doi: 10.1016/j.rse.2013.12.007
Templ, B., Koch, E., Bolmgren, K., Ungersböck, M., Paul, A., Scheifinger, H., et al. (2018). Pan european phenological database (pep725): a single point of access for european data. Int. J. Biometeorol. 62, 1109–1113. doi: 10.1007/s00484-018-1512-8
Virtanen, P., Gommers, R., Oliphant, T. E., Haberland, M., Reddy, T., Cournapeau, D., et al. (2020). SciPy 1.0: fundamental algorithms for scientific computing in python. Nat. Methods 17, 261–272. doi: 10.1038/s41592-020-0772-5
Von Arx, G., Graf Pannatier, E., Thimonier, A., and Rebetez, M. (2013). Microclimate in forests with varying leaf area index and soil moisture: potential implications for seedling establishment in a changing climate. J. Ecol. 101, 1201–1213. doi: 10.1111/1365-2745.12121
Wang, Q., Tenhunen, J., Dinh, N. Q., Reichstein, M., Otieno, D., Granier, A., et al. (2005). Evaluation of seasonal variation of modis derived leaf area index at two european deciduous broadleaf forest sites. Remote Sens. Environ. 96, 475–484. doi: 10.1016/j.rse.2005.04.003
Wang, Q., Tenhunen, J., Granier, A., Reichstein, M., Bouriaud, O., Nguyen, D., et al. (2004). Long-term variations in leaf area index and light extinction in a fagus sylvatica stand as estimated from global radiation profiles. Theor. Appl. Climatol. 79, 225–238. doi: 10.1007/s00704-004-0074-3
Waskom, M. L. (2021). seaborn: statistical data visualization. J. Open Source Softw. 6, 3021. doi: 10.21105/joss.03021
Weiss, M., Baret, F., Smith, G., Jonckheere, I., and Coppin, P. (2004). Review of methods for in situ leaf area index (lai) determination: part ii. estimation of lai, errors and sampling. Agric. Forest Meteorol. 121, 37–53. doi: 10.1016/j.agrformet.2003.08.001
Yan, G., Hu, R., Luo, J., Weiss, M., Jiang, H., Mu, X., et al. (2019). Review of indirect optical measurements of leaf area index: recent advances, challenges, and perspectives. Agric. For. Meteorol. 265, 390–411. doi: 10.1016/j.agrformet.2018.11.033
Keywords: leaf area index, phenology, foliage, microclimate, forest climate, fagus sylvatica
Citation: Zolles A, Schueler S, Gartner K and Scheifinger G (2021) Continuous Parameterization of Leaf Area Index and Phenological Phases Within Deciduous Forests Based on Temperature Measurements. Front. For. Glob. Change 4:768085. doi: 10.3389/ffgc.2021.768085
Received: 31 August 2021; Accepted: 04 October 2021;
Published: 28 October 2021.
Edited by:
Arthur Gessler, Snow and Landscape Research (WSL), SwitzerlandCopyright © 2021 Zolles, Schueler, Gartner and Scheifinger. This is an open-access article distributed under the terms of the Creative Commons Attribution License (CC BY). The use, distribution or reproduction in other forums is permitted, provided the original author(s) and the copyright owner(s) are credited and that the original publication in this journal is cited, in accordance with accepted academic practice. No use, distribution or reproduction is permitted which does not comply with these terms.
*Correspondence: Anita Zolles, anita.zolles@bfw.gv.at
Disclaimer: All claims expressed in this article are solely those of the authors and do not necessarily represent those of their affiliated organizations, or those of the publisher, the editors and the reviewers. Any product that may be evaluated in this article or claim that may be made by its manufacturer is not guaranteed or endorsed by the publisher.
Research integrity at Frontiers
Learn more about the work of our research integrity team to safeguard the quality of each article we publish.