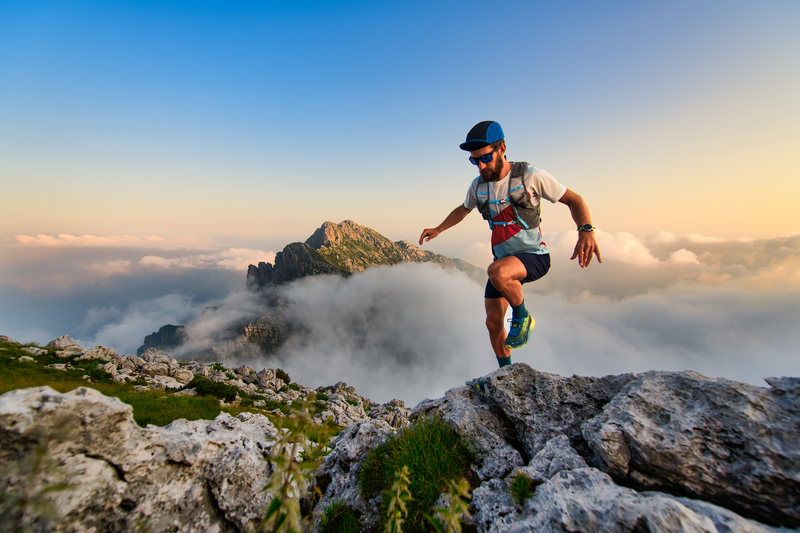
95% of researchers rate our articles as excellent or good
Learn more about the work of our research integrity team to safeguard the quality of each article we publish.
Find out more
ORIGINAL RESEARCH article
Front. For. Glob. Change , 12 July 2021
Sec. Forests and the Atmosphere
Volume 4 - 2021 | https://doi.org/10.3389/ffgc.2021.689836
This article is part of the Research Topic Forest Monitoring to Assess Forest Functioning Under Air Pollution and Climate Change View all 8 articles
Resource allocation to different plant tissues is likely to be affected by high investment into fruit production during mast years. However, there is a large knowledge gap concerning species-specific differences in resource dynamics. We investigated the influence of mast years on stem growth, leaf production, and leaf carbon (C), nitrogen (N), and phosphorus (P) concentrations and contents in Fagus sylvatica, Quercus petraea, and Q. robur at continental and climate region scales using long-term data from the International Co-operative Programme on Assessment and Monitoring of Air Pollution Effects on Forests (ICP Forests) and similar datasets. We discussed the results in the light of opposing resource dynamics hypotheses: (i) resource accumulation before mast years and exhaustion after mast years (resource storage hypothesis), (ii) shifting resources from vegetative to generative compartments (resource switching hypothesis), and (iii) investing resources concurrently in both vegetative and generative compartments (resource matching hypothesis). Linear mixed-effects modelling (LMM) showed that both stem growth and leaf production were negatively influenced by weather conditions which simultaneously lead to high fruit production. Thus, the impact of generative on vegetative growth is intermixed with effects of environmental factors. Superposed epoch analyses and LMM showed that for mast behaviour in F. sylvatica, there are indicators supporting the resource storage and the resource switching hypotheses. Before mast years, resources were accumulated, while during mast years resources switched from vegetative to generative tissues with reduced stem and leaf growth. For the Quercus species, stem growth was reduced after mast years, which supports the resource storage hypothesis. LMM showed that leaf C concentrations did not change with increasing fruit production in neither species. Leaf N and P concentrations increased in F. sylvatica, but not in Quercus species. Leaf N and P contents decreased with increasing fruit production in all species, as did leaf C content in F. sylvatica. Overall, our findings suggest different resource dynamics strategies in F. sylvatica and Quercus species, which might lead to differences in their adaptive capacity to a changing climate.
Synchronised occurrence of mass seed and fruit production in stands and forests is a reproductive phenomenon of many long-lived plant species (Nilsson and Wästljung, 1987; Kelly, 1994; Kelly and Sork, 2002; Nussbaumer et al., 2018; Vacchiano et al., 2018). Years with mass seeding, so called mast years, have an impact on wood production, tree vitality, and natural regeneration, as well as on the biogeochemical cycles of carbon (C) and nutrients (Eichhorn et al., 2008; Drobyshev et al., 2010). In the light of recent climate change, an increase in frequency of climatic and biotic extreme events impacting tree functioning is observed, including C resource dynamics at large scales (Sala et al., 2012). In addition to these cascading effects of extreme events, mast years repeatedly impact resource dynamics, which may, in combination with extreme events, impair long-term tree performance. Furthermore, mast years play an important role in ecosystems in many ways, e.g., they provide an additional food source for forest wildlife, for example for red deer (Picard et al., 1991), wild boar (Henry and Conley, 1972; Wohlgemuth et al., 2016) or bird species (Herrera et al., 1994). It can even impact the migration pattern of birds, for example bramblings which are known to winter further north after beech mast years in Middle Europe (Jenni, 1987). Mast years can also have a bearing on human health due to denser rodent populations (Ogawa et al., 2017). Infection pressure of zoonoses typically increases 1 or 2 years after a mast year, depending on the life cycles of the vector species. This potentially leads to higher infection rates of e.g., Lyme Borreliosis or diseases induced by Hanta viruses (Vapalahti et al., 2003; Ostfeld, 2013; Brugger et al., 2018).
According to Herrera et al. (1998) and Koenig et al. (2003), mast behaviour is a result of individual annual variability in seed production and synchrony between individuals. Different causes for mast behaviour are currently debated. Ultimate hypotheses concentrate on the evolutionary advantages of mast behaviour, while proximate hypotheses explain the mechanisms of mast behaviour, including resource dynamics (Kelly, 1994; Pearse et al., 2016). The underlying assumption for ultimate hypotheses is that it is favourable for trees in a stand to synchronise their flowering to reduce the costs for seed production, which always includes economy of scale effects. The most commonly discussed ultimate hypotheses are the predator satiation hypothesis, the pollination efficiency hypothesis, and the environmental prediction hypothesis (Pearse et al., 2016). Concepts to investigate and explain allocation shifts of resources, such as C and nutrients, belong to the proximate hypotheses and are summarised in terms of resource dynamics (Kelly and Sork, 2002; Crone and Rapp, 2014; Pearse et al., 2016; Figure 1). The most commonly discussed proximate hypotheses and mechanisms are the resource budget hypothesis (also known as resource budget model) which includes: (i) the resource storage hypothesis with the underlying mechanisms resource accumulation and resource depletion and (ii) the resource switching hypothesis. An additional common hypothesis is (iii) the resource matching hypothesis (Janzen, 1971; Kelly, 1994; Kelly and Sork, 2002; Monks and Kelly, 2006; Hacket-Pain et al., 2015; Pearse et al., 2016; Bogdziewicz et al., 2020a). (i) The resource storage hypothesis assumes that a strong flowering/fruiting year will only occur after years with no or low fruit production in which resource accumulation/resource storage above a certain threshold occurs (Isagi et al., 1997; Satake and Iwasa, 2000; Han et al., 2014; Bogdziewicz et al., 2020a). Synchrony between trees is controlled by one or more additional factors, such as pollination efficiency or weather cues (Rees et al., 2002; Pearse et al., 2016). Resource depletion is likely to occur following a mast year due to the size of the reproductive effort masting requires (Janzen, 1971; Hacket-Pain et al., 2015; Pearse et al., 2016). (ii) The resource switching hypothesis states that a variable fraction of a relatively constant annual resource budget is allocated to reproduction (Pearse et al., 2016). Therefore, resources are primarily allocated to generative instead of vegetative tissue during a mast year, leading to a reduction of vegetative growth in favour of fruit production (Kelly, 1994; Pearse et al., 2016; Bogdziewicz et al., 2020a). (iii) The resource matching hypothesis, in contrast, assumes that the occurrence of mast years is triggered by favourable environmental conditions and, thus, larger resource availability (Kelly and Sork, 2002; Monks and Kelly, 2006; Pearse et al., 2016). Under those conditions, sufficient resources would be obtainable for both vegetative and generative functioning. Accordingly, it is expected that vegetative growth is not reduced by generative growth, but can even be enhanced (Kelly, 1994; Pearse et al., 2016).
Figure 1. Proximate mast hypotheses concerning resource dynamics: The resource storage hypothesis states that resources have to be accumulated before high amounts of fruits can be produced (resource accumulation). In the wake of the mast year, resources are depleted (resource depletion) and have to be accumulated again before another mast year occurs. The resource switching hypothesis assumes that during the mast year, resources are shifted from vegetative to generative growth. The resource matching hypothesis states that in environmentally favourable years, vegetative and generative growth are equally enhanced. ?: Resource dynamics in mast years can vary. Red arrows: Stem growth of the current year. Year –1 = 1 year before mast year, Year 0 = mast year, Year + 1 = 1 year after mast year. Original artwork by Anita Nussbaumer.
Fruit, wood and, to some extent, also leaf production are partly controlled by weather conditions, before and during the growing season. Fagus sylvatica L. is known to be a masting pollen producer. This means that flower buds are not produced every year, which can be seen in the lack of airborne pollen in the following spring (Geburek et al., 2012). Mast years in F. sylvatica typically occur after a summer with low temperatures and high precipitation rates 2 years prior to the mast year, and a warm and dry summer 1 year prior to the mast year (Piovesan and Adams, 2001; Drobyshev et al., 2010, 2014; Hacket-Pain et al., 2015; Bogdziewicz et al., 2017; Vacchiano et al., 2017; Lebourgeois et al., 2018; Nussbaumer et al., 2018). Additionally, warm and dry conditions during the flowering period in the mast year itself are important for masting success (Kasprzyk et al., 2014; Nussbaumer et al., 2018). Stem growth in F. sylvatica is positively influenced by high precipitation sums during (early) spring and summer of the current year (Lebourgeois et al., 2005; Piovesan et al., 2008; Ježík et al., 2011; Michelot et al., 2012; Siegmund et al., 2016; Mund et al., 2020), but negatively impacted by high summer temperatures in the current year (Michelot et al., 2012) and dry summer conditions in the previous year (Piovesan et al., 2008; Seidling et al., 2012). Similar to stem growth, high leaf production in beech is dependent on low summer temperatures in the previous year and high precipitation sums during the growing season (Müller-Haubold et al., 2013). According to Geburek et al. (2012), Quercus species produce high amounts of pollen every year and are so-called non-masting pollen producers. In contrast to F. sylvatica, Quercus species are therefore prepared to produce high amounts of fruits every year. Mast years in Q. robur L. and Q. petraea (MATT.) LIEBL. mainly follow favourable weather conditions during the flowering season, with weather conditions in previous years having little impact (Bogdziewicz et al., 2017; Lebourgeois et al., 2018; Nussbaumer et al., 2018). Stem growth in Q. robur and Q. petraea is positively impacted by high precipitation sums in the previous autumn (Michelot et al., 2012), and negatively influenced by cold and dry winters (Lebourgeois et al., 2004; Rozas, 2005; Rozas et al., 2009; Michelot et al., 2012), a warm March (Mérian et al., 2011), and a cold or dry May and summer (Lebourgeois et al., 2004; Rozas, 2005; Mérian et al., 2011). In contrast to stem growth, the impact of weather conditions on leaf production of Q. robur and Q. petraea has not been specifically investigated in earlier studies.
The impact of mast years on wood production has been investigated in several recent studies at a regional to national scale, and species-specific differences were indicated. Stem increment of F. sylvatica was shown to be reduced in years with high beechnut production in Germany (Eichhorn et al., 2008), South Sweden (Drobyshev et al., 2010), France (Lebourgeois et al., 2018), and Switzerland (Braun et al., 2017). In contrast, stem growth of Q. robur and Q. petraea increased during mast years in France (Q. robur and Q. petraea; Lebourgeois et al., 2018) and in the Volga region in Russia (Q. robur; Askeyev et al., 2005), and was even enhanced in the subsequent year (Askeyev et al., 2005).
Changes in allocation dynamics of C and nutrients, such as nitrogen (N) and phosphorus (P), during mast years have been widely investigated. When looking at the results of such studies it must be taken into account that C and nutrient concentrations depend, inter alia, on leaf size, which can also be impacted by high fruit production. Therefore, a distinction has to be made between nutrient concentrations (mg/gleaf) and nutrient contents (mass per leaf). Hoch et al. (2013) showed that in F. sylvatica, stored C is invested into leaves and flowers but not into fruits. This shows that during mast years, currently assimilated C is mainly allocated to fruits and less to leaves (Ichie et al., 2013; Han and Kabeya, 2017). Two studies on leaf N concentration in F. sylvatica showed a reduction during mast years (Han et al., 2011; Müller-Haubold et al., 2015). Han et al. (2014) further found that in Fagus crenata (Blume), N concentrations of leaves and branches were lower in the year following a mast year. In contrast, Braun et al. (2020b) found that in European beech, leaf N and P concentrations increased during mast years, while N and P contents were reduced. Abe et al. (2016) and Yasumura et al. (2006) found that fruit production in F. crenata required high amounts of N, but neither leaf N content nor N concentration was reduced during mast years (Yasumura et al., 2006). Jonard et al. (2009) hypothesised that leaf P concentrations may be reduced during mast years in F. sylvatica, Q. robur, and Q. petraea in France, Walloon and Luxembourg. Braun et al. (2020b) found for F. sylvatica that leaf P concentrations were higher in trees with high fruit production, and Fernández-Martínez et al. (2017) found a similar pattern for deciduous species genera across Europe. These findings suggest that N and P are both essential nutrients for fruit production and, thus, can act as a limiting factor for mast year occurrence.
Recent studies show that the frequency of mast years increased within the last decades in F. sylvatica, Q. robur and Q. petraea (all species: Nussbaumer et al., 2016; beech: Bogdziewicz et al., 2020b, oak: Caignard et al., 2017). Climate scenarios show that weather extremes, such as summer heat waves, prolonged droughts, or late frost events will most probably increase in the next few decades (IPCC, 2013, 2019). Such climatic extreme events potentially lead to stress in forest ecosystems. High fruit production, which may be seen as a biotic extreme event, can act as an additional stress factor impacting resource dynamics. In a new study on the impact of the 2018 summer drought on F. sylvatica in Switzerland, Nussbaumer et al. (2020) found that prolonged summer heat waves and droughts during fruit development lead to fruit abortion. There is thus evidence that some tree species may struggle to reproduce under the projected climate scenarios for the next 50–100 years. Therefore, it is crucial to better understand the mechanisms of resource dynamics in forest tree species in order to recognise potential stress reactions, and to support decision making for future forestry, from both ecological and economic perspectives. A recent review on forest C allocation modelling under climate change revealed that the reproductive pool and its dynamics is often ignored due to patchy knowledge of the governing processes (Merganičová et al., 2019). Therefore, large-scale species-specific studies can strongly contribute to the improvement of forest ecosystem modelling.
In this study, we investigated F. sylvatica, Q. robur, and Q. petraea across Europe. We evaluated data from European-wide long-term forest monitoring datasets. The analyses were carried out at stand and individual tree level, investigating fruit and leaf production, stem growth, and leaf nutrient concentrations. In an earlier study, Nussbaumer et al. (2018) showed that the impact of external and internal conditions on fruit production differ regionally and, therefore, data was analysed at continental as well as climate region scale.
The main objective of our study was to shed light on the impact of masting on vegetative growth and assign our findings to the commonly discussed proximate hypotheses and mechanisms of resource dynamics. We investigated the following issues:
(i) Which common resource dynamics mechanisms are involved in fruit and leaf production, nutrient allocation and stem growth of the investigated species?
(ii) How spatially consistent are these mechanisms at a continental scale?
(iii) How do weather conditions and fruit production control stem growth and leaf production of the investigated species?
In this study, we investigated generative and vegetative growth in the most common European deciduous tree species Fagus sylvatica L. (hereafter beech), Quercus robur L. and Q. petraea (MATT.) LIEBL. (hereafter oak). For all species, flowering begins between early April and early May, depending on the geographical region. Fruits are developed over the summer of the same year. Fruit fall starts around late September and continues until late October. In beech, the growing season starts in April and ends in late summer, and in oak, stem growth starts in early April and continues until October.
We analysed data from intensive monitoring plots of the International Co-operative Programme on Assessment and Monitoring of Air Pollution Effects on Forests (ICP Forests), carried out under the UNECE Convention on Long-Range Transboundary Air Pollution (UNECE and ICP Forests Programme Co-ordinating Centre, 2016). Furthermore, we included data from an eddy covariance site in the Jastrebarsko forest in Croatia (Anić et al., 2018) and from long-term monitoring plots of the Institute of Applied Plant Biology (IAP) in Switzerland (Braun and Flückiger, 2013). The plots explored in this study cover Mediterranean, temperate, continental and alpine climates, and are distributed from 5.5° W to 24° E and 41.5° N to 57° N, with an altitudinal range of 0–1,500 m a.s.l (Supplementary Figures 1, 2 and Supplementary Tables 1A,B).
We used the gridded meteorological observation E-OBS dataset (Version 17.0) of the European Climate Assessment and Dataset (ECA&D) which provides daily measurements with a spatial resolution of 0.25° (Haylock et al., 2008). We calculated the long-term means (1996–2015) for 2 months mean temperatures and precipitation sums. For the statistical analyses we calculated deviations from the 2 months mean temperatures and precipitation sums for the months July to December of the previous year, and for January to August of the current year, according to equations 1 and 2 (see below).
Within the ICP Forests network, measurements and assessments are carried out at individual tree or plot level, and the two additional datasets from Croatia and Switzerland consist of plot level measurements. For our analyses, we used litterfall, fresh leaf and stem growth measurements (litterfall: Ukonmaanaho et al., 2016; fresh leaf: Rautio et al., 2016; stem growth: Dobbertin and Neumann, 2016; additional datasets: Braun and Flückiger, 2013; Anić et al., 2018; Supplementary Tables 1A,B; list of used variables: Supplementary Table 2). The data cover the periods 1994–2018 (litterfall), 1994–2017 (fresh leaves), and 1995–2017 (stem growth; Supplementary Tables 1A,B). We used the variables: (i) annual fruit biomass (hereafter fruit production) and annual leaf biomass (hereafter leaf production) from the litterfall survey, (ii) dry mass of 100 leaves and leaf C, N and P concentrations from the fresh leaf sampling survey, and the products of dry mass of 100 leaves and C, N and P concentrations, hereafter leaf C, N and P contents, and (iii) average annual basal area increment per plot (BAIann) from the growth survey.
All data from ICP Forests were collected by members of the ICP Forests, and the additional data were gathered in similar ways. (i) Litterfall was sampled continuously with litter traps with a minimum size of 0.18 m2 (Ukonmaanaho et al., 2016). After drying at a maximum of 70°C, the samples were sorted into pre-defined fractions, such as leaves and fruits of the main tree species (Ukonmaanaho et al., 2016). The samples were pooled into annual volumes of leaves (leafann) and fruits (fruitann) per plot. We excluded years if litterfall was collected for less than 280 days per year, or if litterfall was not collected during the leaf and fruit fall period. (ii) Fresh leaves were harvested from the canopy at the end of the growing season (usually late July to mid-August) before leaf senescence, generally every second year, although at some sites, samples were collected annually. For the chemical analysis of C, N and P, subsamples were taken from the total volumes of the leaves, and the dry mass of 100 leaves was measured (Rautio et al., 2016). We calculated C, N and P contents as products of mass of 100 leaves and C, N and P concentrations. (iii) Stem diameter or stem circumference at breast height (1.3 m above ground) was measured on approximatively 10 dominant or codominant trees per plot either several times during the year or once a year during dormancy (October to early March, depending on altitude; Dobbertin and Neumann, 2016). At the French sites, tree-ring widths were used, which we did not standardise for age trends as we investigated only short periods. At the Croatian eddy-flux site, measurements were performed at all trees with a stem diameter above 2 cm (Anić et al., 2018). We calculated annual BAI of individual trees as the difference in basal area during dormancy between two consecutive years. To calculate an average value per plot (BAIann) for each species, we averaged all available BAI of individual trees per plot and species.
We used long-term monthly mean temperature and precipitation sums of the E-OBS dataset (Haylock et al., 2008) to assign the plots to climate regions using the Ward method (CLUSTER procedure in SAS 9.4; SAS Institute Inc., Cary, NC, United States). The resulting three clusters are: the (i) warm temperate region (warmest and driest conditions), the (ii) moderate temperate region (intermediate temperate conditions), and the (iii) cool temperate region (coldest and wettest conditions; all clusters according to Köppen, 1936; Supplementary Figures 1, 2).
Figure 2. Results from averaged linear mixed-effects models assessing the effects of weather cues and fruit production (fruitann) on basal area increment (BAIann) and leaf production (leafann), and the effects of weather conditions on fruitann. (A) Fagus sylvatica, (B) Quercus sp. Weather conditions were used as deviations from the 2-monthly long-term mean temperatures and precipitation sums of the previous and current year. Full model: Includes all measured years on all plots, low fruiting model: Includes only years with less than 10% of maximum fruit production, fruitann model includes all measured years on all plots. + : Increase, –: Decrease, symbol size shows parameter importance in the averaged models. mR2: Marginal R2, cR2: Conditional R2.
We analysed the two oak species both individually and as one genus. Since it cannot be excluded that the two oak species are unwittingly mixed in the collectors or during sorting, we discuss only the results of the analysis of the genus Quercus sp. The results for the individual oak species, defined by the main tree species on plot, can be found in the Supplementary Material (Supplementary Figure 8 and Supplementary Tables 9–12).
The analysed plots were measured for 3–20 years (beech, median: 8 years) and 3–18 years (oak, median: 9 years). Dry mass of 100 leaves, leaf C, N and P concentrations, and leaf C, N and P contents were measured for 3–11 years (beech, median: 7 years) and 3–15 years (oak, median: 7 years). Median frequency of years with 60% of the maximum of measured fruit biomass per plot (= mast years) was 28% on the beech plots and 25% on the oak plots. Mean coefficients of variation per plot (ratio of standard deviation and long-term mean; Koenig et al., 2003) did not differ between the species (beech: 140%, oak: 130%).
We investigated: (i) the influence of fruitann and weather conditions on BAIann and leafann, and the influence of the same weather parameters on fruitann with linear mixed effects models (LMM). We used these results to better interpret the results from the subsequent superposed epoch analyses [(SEA, see (ii)] investigating the influence of years with high fruitann on BAIann and leafann. To explore underlying resource dynamics mechanisms we analysed (ii) the temporal variation of BAIann and leafann before, during and after years with high fruitann with SEA, and iii) the changes in dry mass of 100 leaves, leaf C, N and P concentrations, and leaf C, N and P contents with increasing fruitann using LMM.
(i) We performed LMM to investigate the influence of fruitann and weather parameters on BAIann and leafann, as well as the influence of weather parameters on fruitann. We included deviations from the 2-monthly mean temperatures and precipitation sums from July of the previous year to August of the current year in the model. We did not find multicollinearity between the independent variables (variance inflation factor <5, “vif” function from the R package “car”; Burnham and Anderson, 2002). We tested two LMM to explore the influence of fruitann and weather conditions on BAIann and leafann: (a) including all measured years (full model), and (b) including only years with less than 10% of the maximum of fruitann (low fruiting model):
where y is BAIann or leafann.
For the analysis of the influence of weather parameters on fruitann we used:
In both equations T is mean temperature, P is precipitation sum, lag1 is the value of the previous year, lag0 is the value of the current year, and “plot” is a random factor. We used the R function “dredge” from the R package “MuMIn” (Barton, 2017) to calculate all possible models of (a) and (b) with up to five variables. We applied the corrected Akaike information criterion (AICC; Burnham and Anderson, 2002) to define the best fitting models, and calculated average models of all models with deviation <2 from the best AICC. In each LMM we included a correction term for temporal autocorrelation and evaluated the models via marginal and conditional R2 (Nakagawa and Schielzeth, 2013). The marginal R2 explains the effect of the fixed effects on the dependent variable, and the conditional R2 explains the accuracy of the whole model, including the random effects.
(ii) To test for temporal correlation, i.e., the correlations between fruitann and related weather conditions in a focal (year with high fruitann) and vegetative growth (BAIann and leafann) in the same year and the years before and after the focal year, we used SEA for all spatial classes. We tested the SEA for various cut-off points for focal years (60, 70, 80, and 90% of the maximum of fruitann) and found that the results only differ slightly between the thresholds (Supplementary Figures 5, 6 and Supplementary Tables 3, 4). Hereafter, we present results using the 60% threshold (Supplementary Figures 3, 4). We focused on the period of the two preceding and two subsequent years of the focal year, resulting in 5-year time series. The SEA function estimates whether the means of individual years within the 5-year epochs centred on the focal year are different from equivalent years from randomly selected 5-year epochs via bootstrap resampling (Bunn et al., 2018). SEA allow the comparison of focal years in different time series, exclude noise from other potentially influential factors with similar time scales, and is not restricted to normally distributed data (Prager and Hoenig, 1989; Bunn et al., 2018; Park et al., 2019).
For the SEA, we used a subset to meet the model requirements, i.e., five consecutive measurement years, the third of which is a mast year. This resulted in 18% (beech) and 17% (oak) of all measured years being mast years. Standard errors and confidence intervals of the SEA for all analyses at a 60% threshold can be found in Supplementary Tables 6, 10. Beech is considered a species with a basic 2 years mast cycle (Matthews, 1955; Braun et al., 2020a; Nussbaumer et al., 2020). In our beech data, such a pattern was partly present but not dominant (Supplementary Figure 3). Cases of mast years 2 years before or after the focal years occurred in nine (Year–2; 41%) and six (Year+2; 27%) out of 22 SEA series in the European dataset. In the warm temperate region there were four cases for both Year–2 and Year+2 (57%) out of seven series, in the moderate temperate region there were four (Year–2; 44%) and one (Year+2; 11%) cases out of 9 series, and in the cool temperate region there was one case for both Year–2 and Year+2 out of six series (17%).
(iii) We applied LMM for the investigation of the effect of fruitann on dry mass of 100 leaves, leaf C, N and P concentrations, and leaf C, N and P contents, and used “plot” as a random factor (Pinheiro et al., 2020):
where y equals dry mass of 100 leaves, leaf C, N and P concentrations, or leaf C, N, and P contents. We performed the analyses for Europe and the climate regions if at least 20 measurements were available. As described in (i) we included a correction term for temporal autocorrelation in the LMMs and evaluated the models via marginal and conditional R2.
We performed statistical analyses using R, version 3.5.1 (R Core Team, 2019), i.e., SEA using the function “sea” from the R package “dplR” (Bunn et al., 2018) and linear mixed-effects modelling (LMM) using the function “lme” from the R package “nlme” (Pinheiro et al., 2020).
We transformed values of BAIann, leafann, and fruitann into percentage of maximum values per plot to be able to quantify the effect size. We used a significance level of α = 0.05, and considered results with p-values between 0.05 and 0.10 as marginally non-significant.
In the analysis of weather conditions and fruitann impacting BAIann, fruitann of beech was a main explanatory variable in the full model and showed a strong negative impact on stem growth (LMM, full model: n = 210, marginal R2 = 0.139, conditional R2 = 0.324; Figure 2A). In both the full and the low fruiting models, low mean temperatures in July/August of the previous year, high precipitation sums in July/August of the current year and, to a lesser amount, high precipitation sums in January/February of the current year were the most important weather conditions for enhanced BAIann (LMM, low fruiting model: n = 114, marginal R2 = 0.150, conditional R2 = 0.362; Figure 2A).
leafann of beech was primarily influenced by high temperatures in September/October of the previous year, high temperatures in January/February and July/August of the current year, and low temperatures in March/April of the current year (LMM, full model: n = 210, marginal R2 = 0.201, conditional R2 = 0.302; low fruiting model: n = 114, marginal R2 = 0.207, conditional R2 = 0.372; Figure 2A).
The best explaining weather conditions leading to high fruitann were high mean temperatures in July/August of the previous year, low mean temperatures from September to December of the previous year, high mean temperatures and, to a lower amount, also precipitation sums in March/April of the current year (LMM, n = 210, marginal R2 = 0.380, conditional R2 = 0.469; Figure 2A).
BAIann of beech at European scale was reduced by 14.2% during mast years (SEA, n = 22, p0 < 0.001) and enhanced by 7.6 and 6.6% in the preceding years (SEA, n = 22, p–2 = 0.026, p–1 = 0.036; Figure 3B and Table 1). At climate region scale, this pattern was also present, although the effects were generally weaker, and in the cool temperate region, BAIann was not significantly lower during mast years.
Figure 3. Superposed epoch analyses (SEA) for Europe and climate regions: (A) basal area increment in Fagus sylvatica, (B) leaf biomass in Fagus sylvatica, (C) basal area increment in Quercus sp., (D) leaf biomass in Quercus sp. SEA investigate the relations of a parameter in focal years (Year 0) to the same parameter in the previous and subsequent years (Year–2 = 2 years before mast year, Year–1 = 1 year before mast year, Year 0 = mast year, Year + 1 = 1 year after mast year, Year + 2 = 2 years after mast year). All values in percent of the maximum value per plot. Threshold level for mast year definition: 60% of the maximum of measured fruit biomass per plot. For better visibility the curves are displayed slightly offset. BAI: Basal area increment.
Table 1. Results (p-values and effect size in %) of the superposed epoch analyses for Europe and climate regions investigating the temporal correlation between fruit production and vegetative growth (basal area increment and leaf production).
leafann of beech at European scale was reduced by 4.4% during mast years (SEA, n = 53, p0 = 0.027) and 1 year before mast years, it was enhanced by 6% (SEA, n = 53, p–1 = 0.005; Figure 3B and Table 1). In the cool temperate region, this pattern was also present, but in the warm temperate and the moderate temperate region, leafann was not significantly reduced during mast years. Dry mass of 100 leaves decreased with increasing fruitann at European scale (LMM, 240 observations on 36 plots, marginal R2 = 0.188, conditional R2 = 0.397, p < 0.0001; Figure 4A and Supplementary Table 8) and in all climate regions (Supplementary Table 8). Leaf C concentration was not affected by enhanced fruitann (Figure 5A and Supplementary Table 7). Leaf N and P concentrations increased with increasing fruitann at European scale (LMM, N: 242 observations on 37 plots, marginal R2 = 0.020, conditional R2 = 0.517, p = 0.0007; P: 250 observations on 38 plots, marginal R2 = 0.010, conditional R2 = 0.669, p = 0.0077; Figures 5C,E and Supplementary Table 7) and in the warm temperate region (Supplementary Table 7). Leaf C, N, and P contents decreased with increasing fruitann in Europe (LMM, C: 108 observations on 32 plots, R2 = 0.225, conditional R2 = 0.225, p < 0.0001; N: 238 observations on 36 plots, marginal R2 = 0.100, conditional R2 = 0.229, p < 0.0001; P: 239 observations on 36 plots, marginal R2 = 0.071, conditional R2 = 0.409, p < 0.0001; Figures 6A,C,E and Supplementary Table 8).
Figure 4. Scatter plots of dry mass of 100 leaves (g) vs. fruit production in percentage of maximum values per plot at European scale. (A) Fagus sylvatica, (B) Quercus sp. Red line: Response curve of the linear mixed-effects modelling, dashed line: Standard errors, mR2: Marginal R2, cR2: Conditional R2.
Figure 5. Scatter plots of leaf carbon concentration in g/100g, nitrogen and phosphorus concentrations in mg/g vs. fruit production in percentage of maximum values per plot at European scale. (A,B) Carbon concentration, (C,D) nitrogen concentration, (E,F) phosphorus concentration, (A,C,E) Fagus sylvatica, (B,D,F) Quercus sp. Red line: Response curve of the linear mixed-effects modelling, dashed line: Standard errors, mR2: Marginal R2, cR2: Conditional R2.
Figure 6. Scatter plots of leaf carbon mass in 100 leaves (= content; g/100 leaves), nitrogen and phosphorus contents (mg/100 leaves) vs. fruit production in percentage of maximum values per plot at European scale. (A,B) carbon content, (C,D) nitrogen content, (E,F) phosphorus content, (A,C,E) Fagus sylvatica, (B,D,F) Quercus sp. Red line: Response curve of the linear mixed-effects modelling, dashed line: Standard errors, mR2: Marginal R2, cR2: Conditional R2.
fruitann of beech 1 year before and after mast years was very low, but 2 years before and after, it was not significantly lower than during mast years (SEA, Supplementary Figures 5A, 7A and Supplementary Tables 3, 5).
Concerning a suggested 2 years mast cycle, there was no significant effect of mast year occurrence 2 years before and after the focal years: Neither was fruitann enhanced nor BAIann or leafann reduced except for leafann in the cool temperate region (Figures 3A,B, Table 1, Supplementary Figures 5A, 6A, 7A, and Supplementary Tables 3–5).
The most important weather conditions for enhanced BAIann of the oak species in both the full and the low fruiting models were low mean temperatures in July/August of the previous and the current year, and high mean temperatures in March/April of the current year. Additionally, in the full model precipitation sums were high in July/August of the current year. In the low fruiting model, mean temperatures were lower than average in September/October of the previous year, and precipitation sums were lower in July/August of the previous year (LMM, full model: n = 320, marginal R2 = 0.189, conditional R2 = 0.226; low fruiting model: n = 186, marginal R2 = 0.191, conditional R2 = 0.191; Figure 2B). fruitann was not part of the best fitting models.
Weather conditions controlling leafann of the oak species were high precipitation sums in July/August in the previous year and high temperatures in July/August of the current year in both the full and the low fruiting model. In the full model, high precipitation sums in March/April and low temperatures in May/June of the current year were also favourable for leaf production (LMM, full model: n = 320, marginal R2 = 0.038, conditional R2 = 0.176; Figure 2B). In the low fruiting model, high precipitation sums in September/October of the previous year and low precipitation sums in May/June of the current year were important drivers for leaf production as well (low fruiting model: n = 186, marginal R2 = 0.028, conditional R2 = 0.032; Figure 2B).
The weather conditions leading to high fruitann in oak were low mean temperatures in July/August of the previous year, high mean temperatures from September to December of the previous year, low precipitation sums in March/April of the current year, and high precipitation sums in July/August of the current year (LMM, n = 320, marginal R2 = 0.163, conditional R2 = 0.163; Figure 2B).
At European scale, BAIann of the oak species was not affected in mast years, but in the year before mast years, BAIann was enhanced by 6.9% (SEA, n = 26, p–1 = 0.018; Figure 3C and Table 1). In the 2 years after mast years, BAIann was marginally non-significantly reduced by 4.5 and 3.7% (SEA, n = 26, p+1 = 0.061, p+2 = 0.092; Figure 3C and Table 1). In the warm temperate region, the same pattern was present, but in the moderate temperate region there was no reduction in BAIann in the years after the mast year.
leafann of the oak species did not change during the investigated 5-years periods (SEA, Figure 3D and Table 1). Dry mass of 100 leaves significantly decreased with increasing fruitann at European scale (LMM, 228 observations on 33 plots, marginal R2 = 0.024, conditional R2 = 0.319, p = 0.0108; Figure 4B and Supplementary Table 8) and in the warm temperate region (Supplementary Table 8). Leaf C, N, and P concentrations showed no significant change with increasing fruitann (LMM, Figures 5B,D,F and Supplementary Table 7). Leaf C content showed no significant change with increasing fruitann (LMM, Figure 6B and Supplementary Table 8). Leaf N and P contents decreased with increasing fruitann in Europe (LMM, N: 248 observations on 33 plots, marginal R2 = 0.015, conditional R2 = 0.278, p = 0.0350; P: 239 observations on 36 plots, marginal R2 = 0.022, conditional R2 = 0.420, p = 0.0038; Figures 6D,F and Supplementary Table 8).
fruitann of the oak species was significantly reduced in the 2 years before and 1 year after a mast year (SEA, Supplementary Figures 5B, 7B and Supplementary Tables 3, 5).
Our results show that years with high fruit production are partly controlled by weather conditions which simultaneously might reduce stem growth and leaf production. The effect of high fruit production on stem growth is therefore likely to be intermixed with the effect of weather conditions as was shown in a previous study by Hacket-Pain et al. (2018) for beech. The best fitting weather models we found for leaf production showed a similar effect for all investigated species (Figure 2), although for oak, the weather conditions partly varied between the full model and the low-fruiting model. Consequently, the results of the superposed epoch analyses include the effect of mast years with its triggering weather parameters on vegetative growth rather than the effect of high fruit production alone.
Our findings show that the impact of mast years on vegetative growth is species-specific. Although we found evidence for the resource storage hypothesis for all investigated species (Janzen, 1971; Kelly, 1994; Hacket-Pain et al., 2015; Pearse et al., 2016), the effect of mast years on resource allocation to generative compartments was more accentuated for beech than for oak. Reduced stem growth and leaf production in beech during mast years indicates resource switching. Higher vegetative growth in the years prior to mast years suggests resource accumulation in beech (Figures 3A,B and Table 1). Increased leaf production might lead to higher photosynthetic capacity, which is likely to enable higher accumulation of available nutrients and C in the years before mast years. However, surplus C is mostly assimilated as non-structural C and might not be available for fruit production in a following mast year (Fatichi et al., 2014). This is also in accordance with the findings by Hoch et al. (2013); Ichie et al. (2013), and Han and Kabeya (2017) who found that C in beech fruits mostly stems from currently assimilated C. In oak, total mass of leaves was not reduced in mast years which shows that oak is able to compensate for reduced mass of individual leaves and presumably also for leaf C content by producing more leaves (Figures 3D, 4B, 6B and Table 1). In contrast to beech, leaf production was not enhanced before the mast year and therefore, resource accumulation appears to not take place (Figure 3D and Table 1). However, high stem growth in oak in the years before the mast year shows that resources were available for increased growth (Figure 3C). Reduced stem growth in oak in the years after the mast year is indicative of resource depletion, but this effect was moderate (Figure 3C). The absence of an immediate effect of high fruit production on stem growth shows that oak is able to invest additional resources for fruit production without resource switching, and follows different resource allocation mechanisms than beech.
Increasing fruit production had no effect on leaf C concentrations in all species and on leaf N and P concentrations in oak. In beech, leaf N and P concentrations increased with increasing fruit production (Figure 5 and Supplementary Table 7). This is in contrast with Han et al. (2011) and Müller-Haubold et al. (2015) who found that beech leaf N concentrations decreased with higher fruit production in beech, and with Jonard et al. (2009) who suggested that P concentrations are likely to be reduced in mast years in our investigated species. Our findings can be explained as a concentration effect of smaller leaf mass in mast years (Figure 4A and Supplementary Table 8), which is also described in Braun et al. (2020b), but could also be a sign for better nutrient supply. However, we found a decrease of leaf C, N and P contents with increasing fruit production, except for leaf C content in the oak species which was not affected by fruit production (Figure 6 and Supplementary Table 8). Hence the increase of N and P concentrations in beech could be an effect of reduced leaf C content when in mast years C is less invested in leaf production as seen in the reduced mass of 100 leaves. This assumption does not contradict Ichie et al. (2013) and Han and Kabeya (2017) who found for beech that currently assimilated C is used for fruit production, and Hoch et al. (2013) who showed that for leaf production, stored C is used. It seems that in beech mast years, overall C allocation to leaves is reduced even if stored C is available. This could be a sign for resource switching from leaves to fruits in beech mast years. The reduction in N and P contents in oak is also likely to be evidence for resource switching as, at the same time, C content was not reduced. Furthermore, the reduction of N content with increasing fruit production supports the assumption of Pearse et al. (2016) that N is the most likely candidate for resource depletion in connection with mast years as it is a crucial nutrient for fruit production.
There was no evidence for the resource matching hypothesis in either species. For the oak species, this is in contrast to earlier studies which found an increase in stem growth during mast years in France (Q. petraea and Q. robur: Lebourgeois et al., 2018) and the Volga region in Russia (Q. robur: Askeyev et al., 2005). For beech, however, a reduction in stem growth during mast years has been described in several previous studies, which is in contrast with the resource matching hypothesis (Eichhorn et al., 2008; Drobyshev et al., 2010; Lebourgeois et al., 2018).
For beech, we found an immediate negative correlation between high fruiting levels and leaf production in Europe and in the cool temperate region (Figure 3B and Table 1), and a simultaneous reduction in dry mass of 100 leaves (Figure 4A and Supplementary Table 8). Autumn weather conditions in the previous year and spring weather conditions in the focal year had an opposing effect on leaf and fruit production which might be part of this effect. Our results further suggest that in cool and wet conditions, beech has difficulty compensating for smaller leaves in years with high fruit production. This is in accordance with earlier observations in European beech (Müller-Haubold et al., 2015; Braun et al., 2020b). A negative effect of fruit production on leaf production was also recently found in a subset of the ICP Forests Level II plots (Swiss beech stands; Nussbaumer et al., 2020). However, in the warmer climate regions, beech leaf production was not significantly reduced during mast years. These findings are in accordance with Dobbertin (2005) who found for Swiss beech trees that resources allocated to vegetative tissues are primarily invested in leaves rather than stem growth. The reduction of stem growth in beech in the warm temperate and moderate temperate region corresponds with earlier regional studies in Germany (Eichhorn et al., 2008) and France (Lebourgeois et al., 2018). Studies on weather conditions controlling stem growth have shown that wet (and cool) conditions are favourable for beech stem growth, especially during the growing season (Dittmar et al., 2003; Lebourgeois et al., 2004; Rozas, 2005; Piovesan et al., 2008; Ježík et al., 2011; Mérian et al., 2011; Michelot et al., 2012; Scharnweber et al., 2013; Siegmund et al., 2016; Mund et al., 2020; Figure 2A). In our study, plots in the cool temperate region showed no change in stem growth with increasing fruit production. This is in contrast to previous findings by Drobyshev et al. (2010, 2014) on beech stem growth in a comparable climate region (South Sweden) where stem growth was compromised by high fruit production. For oak, stem growth was positively influenced by the same weather conditions in summer of the recent year as for successful mast years (Bogdziewicz et al., 2017; Lebourgeois et al., 2018; Nussbaumer et al., 2018). This may explain the lack of an immediate negative impact of fruit production on stem growth in oak.
Current climate models predict that, while temperatures will very likely increase throughout all seasons, annual precipitation sums will not necessarily be reduced in Europe (Representative Concentration Pathways RCP 8.5; IPCC, 2013, 2019). However, precipitation sums in summer are likely to be reduced up to 40%, while winter precipitation is likely to increase up to 30% in the next few decades, leading to a higher risk for summer droughts (IPCC, 2013, 2019). In our study, we found that for both species, high precipitation sums during summer and, to a lesser amount for beech, before the start of the growth season, are crucial for high stem growth. This is also in accordance with earlier studies (Dittmar et al., 2003; Lebourgeois et al., 2005; Piovesan et al., 2008; Michelot et al., 2012; Scharnweber et al., 2013). Furthermore, summer droughts and prolonged heatwaves can lead to years with mast failure in beech, i.e., years with successful pollination in spring but failing fruit development during summer. This has already been observed at beech stands in Switzerland during the very hot and dry summer 2018 (Nussbaumer et al., 2020). For oak, on the other hand, high summer temperatures have not yet had a negative impact on fruit production (Shibata et al., 2020). Although studies on the possible impact of recent climate change show that seed production increased with increasing temperatures in the last few decades (all species: Nussbaumer et al., 2016; beech: Bogdziewicz et al., 2020b, oak: Caignard et al., 2017), this trend may be reversed for beech in the future.
Our results further suggest that beech may face problems with vegetative as well as generative growth in future climates. However, it cannot be concluded from our results that oak will have similar problems. Especially in cool and humid regions, leaf production in beech was lower during years with high fruit production and, therefore, it can be assumed that photosynthesis is also reduced, leading to decreasing C accumulation. Recent studies on the impact of the 2018 extreme summer drought in large parts of Europe show that under hot and dry conditions beech is prone to early browning and leaf loss in mid-summer (Schuldt et al., 2020). Furthermore, as stated above, we found that, similar to earlier studies, wet spring and summer conditions have a positive impact on beech stem growth (Dittmar et al., 2003; Lebourgeois et al., 2004; Rozas, 2005; Piovesan et al., 2008; Ježík et al., 2011; Mérian et al., 2011; Michelot et al., 2012; Scharnweber et al., 2013; Siegmund et al., 2016; Mund et al., 2020; Figure 2). For oak in our study, we found that high temperatures in spring and low precipitation sums in both the summer before a mast year and the mast year itself are favourable for stem growth. The first finding is consistent with previous studies, while the latter is in contrast (Lebourgeois et al., 2004, 2005; Rozas, 2005; Rozas et al., 2009; Mérian et al., 2011; Michelot et al., 2012; Siegmund et al., 2016; Mund et al., 2020; Figure 2B). However, the results in those studies did not always agree with each other. This may be evidential of a greater dependency of oak on regional climatic conditions than beech. In accordance with this assumption, Mérian et al. (2011) found that for oak, weather cues influencing stem growth changed on a longitudinal gradient. Therefore, we assume that in some regions, the adaptation potential of oak to future climates is higher than in others. This agrees with a recent common garden experiment which investigates the influence of local conditions on adaptation capacity to future climates (Bert et al., 2020). Our findings are further in accordance with results of other studies investigating the survival potential of tree species in Europe in the next few decades (Hanewinkel et al., 2013; Sáenz-Romero et al., 2017). They found a higher adaptive potential for oak than for beech as, in general, oak is better adapted to warmer and drier conditions than beech.
For beech, we found evidence for resource accumulation before mast years, and for resource switching from vegetative to generative tissue during the mast year. For oak, resource depletion was present in the years after the mast year, but in the mast year itself, resource allocation to vegetative tissues was not different from other years. For leaf N, P, and C in all species we suggest that resource switching is present in years with high fruit production. While leaf concentrations were either enhanced (N and P in beech) or unaffected by increasing fruit production (C in beech, C, N, and P in oak), N and P contents were reduced in all species, and C content was reduced in beech. Our findings confirm our hypothesis that beech and oak have partly different resource allocation strategies. Climate change is likely to lead to severe changes in environmental conditions, influencing resilience against extreme events, which will affect mast patterns in many tree species. We found that for beech, fruit production, together with high summer temperatures in the previous year, can act as a stress factor with reduced vegetative growth in years with enhanced generative growth, while oak is able to allocate resources to generative and vegetative growth simultaneously. We further found differences in stem growth, leaf production and leaf nutrient concentrations in beech mast years and in leaf nutrient content in oak mast years between climate regions. Therefore, we suggest that, although mast years often occur in synchrony over large areas, caution is advised when performing spatial extrapolation of specific findings. In our study, we analysed long-term monitoring data which can be used to investigate correlations between variables rather than questions of cause and effect. Therefore, future studies on mast behaviour should concentrate on experimental data, e.g., by exploration of different provenances such as common garden experiments. Furthermore, direct measurements of leaf chemistry and stem growth processes could be used to better understand resource allocation strategies.
The datasets presented in this article are not readily available because the datasets are available through the Programme Co-ordinating Centre (PCC) of ICP Forests database upon request. Requests to access the datasets should be directed to Programme Co-ordinating Centre (PCC) of ICP Forests; https://icp-forests.net/page/data-requests; cGNjLWljcGZvcmVzdHNAdGh1ZW5lbi5kZQ==. For the additional Croatian dataset please contact HM (aHJ2b2plbUBzdW1pbnMuaHI=); for the additional Swiss dataset please contact the Institute of Applied Plant Biology (https://iap.ch).
AN and PW designed the study. AN, SB, BC, SE, MI, FJ, FL, TL, HM, MN, MZOS, TP, PRa, PRo, TS, MS, VŠ, AT, LU, AV, LV, MW, and PW provided samples and data. AN assembled and analysed the data, performed the modelling, and wrote the manuscript. All authors, including AG and AR, contributed to the reviewing and editing of the manuscript.
The data collection was co-financed by governmental bodies of the participating countries and by the European Commission under regulations (EEC) No. 2158/86, Forest Focus (EC) No. 2152/200, and FutMon (EC) LIFE07 ENV/D/218. It was further co-financed by the Swiss State Secretariat for Education, Research and Innovation (SERI C11.0140) within the Cost Action FP0903 “Climate Change and Forest Mitigation and Adaptation in a Polluted Environment” and with Croatian Science Foundation projects EFFEctivity (HRZZ-UIP-2013-11-2492) and MODFLUX (HRZZ-IP-2019-04-6325).
The authors declare that the research was conducted in the absence of any commercial or financial relationships that could be construed as a potential conflict of interest.
We are grateful to the late Matthias Dobbertin who initiated this evaluation. The study was based on several surveys with data from the UNECE ICP Forests Collaborative Database (see www.icp-forests.net, data request 91) and national databases. In particular, data from the countries listed in Supplementary Tables 1A,B were used. We would like to thank the numerous field observation teams that collected the field observations and measurements, the laboratory technicians, the experts in the participating countries, as well as the Expert Panels on Growth, Litterfall, Foliar and Crown Condition that harmonised the applied methods, especially Anna Brechbühl, Noureddine Hajjar, Christian Hug, and Oliver Schramm (WSL, Swiss Federal Institute for Forest, Snow and Landscape Research, Switzerland), Sabine Braun (Institute of Applied Plant Biology, Switzerland), Luc Croisé (Office National des Forêts, Direction Technique et Commerciale Bois, France), Johannes Eichhorn (Nordwestdeutsche Forstliche Versuchsanstalt NW-FVA, Germany), Nadine Eickenscheidt (Landesamt für Natur, Umwelt und Verbraucherschutz Nordrhein-Westfalen LANUV, Germany), Gianfranco Fabbio (Agricultural Research Council, Research Unit for Forest Monitoring and Planning, Italy), Alfred Fürst (Institute for Forest Protection, Austria), Rainer Hentschel (Landesbetrieb Forst Brandenburg, Germany), Hubert Jochheim (Leibniz-Zentrum für Agrarlandschaftsforschung ZALF, Germany), Miklós Manninger (NARIC Forest Research Institute, Hungary), María José Manzano Serrano (ESMA Estudios Medioambientales S.L., Spain), Jan Martin (Landesforst Mecklenburg-Vorpommern, Germany), Giorgio Matteucci (Istituto per i Sistemi Agricoli e Forestali del Mediterraneo, Italy), Claude Parini (Administration de la Nature et des Forêts, Luxembourg), Ekaterina Pavlova (University of Forestry, Bulgaria), Alexander Russ (Landeskompetenzzentrum Forst Eberswalde LFE, Germany), Hans-Werner Schroeck (Forschungsanstalt für Waldökologie und Forstwirtschaft Rheinland-Pfalz, Germany), Iben Margrete Thomsen (Department of Geosciences and Natural Resource Management, University of Copenhagen, Denmark), and Slavka Tothova (National Forest Centre and Forest Research Institute, Slovakia). This study is part of the dissertation by AN (Nussbaumer, 2021).
The Supplementary Material for this article can be found online at: https://www.frontiersin.org/articles/10.3389/ffgc.2021.689836/full#supplementary-material
BAIann, average annual basal area increment per plot; fruitann, annual volumes of fruits (kg/ha) per plot; ICP Forests, International Co-operative Program on Assessment and Monitoring of Air Pollution Effects on Forests; leafann, annual volumes of leaves (kg/ha) per plot; LMM, linear mixed-effects modelling; SEA, superposed epoch analyses.
Abe, T., Tachiki, Y., Kon, H., Nagasaka, A., Onodera, K., Minamino, K., et al. (2016). Parameterisation and validation of a resource budget model for masting using spatiotemporal flowering data of individual trees. Ecol. Lett. 19, 1129–1139. doi: 10.1111/ele.12651
Anić, M., Ostrogovic Sever, M. Z., Alberti, G., Balenovic, I., Paladinic, E., and Peressotti, A. (2018). Eddy covariance vs biometric based estimates of net primary productivity of pedunculate oak (Quercus robur L) forest in Croatia during ten years. Forests 9:764. doi: 10.3390/f9120764
Askeyev, O. V., Tischin, D., Sparks, T. H., and Askeyev, I. V. (2005). The effect of climate on the phenology, acorn crop and radial increment of pedunculate oak (Quercus robur) in the middle Volga region, Tatarstan, Russia. Int. J. Biometeorol. 49, 262–266. doi: 10.1007/s00484-004-0233-3
Bert, D., Lebourgeois, F., Ponton, S., Musch, B., and Ducousso, A. (2020). Which oak provenances for the 22nd century in Western Europe? Dendroclimatology in common gardens. PLoS One 15:e0234583. doi: 10.1371/journal.pone.0234583
Bogdziewicz, M., Ascoli, D., Hacket-Pain, A. J., Koenig, W. D., Pearse, I., Pesendorfer, M. B., et al. (2020a). From theory to experiments for testing the proximate mechanisms of mast seeding: an agenda for an experimental ecology. Ecol. Lett. 23, 210–220. doi: 10.1111/ele.13442
Bogdziewicz, M., Kelly, D., Thomas, P. A., Lageard, J. G. A., and Hacket-Pain, A. (2020b). Climate warming disrupts mast seeding and its fitness benefits in European beech. Nat. Plants 6, 88–94. doi: 10.1038/s41477-020-0592-8
Bogdziewicz, M., Szymkowiak, J., Kasprzyk, I., Grewling, L., Borowski, Z., Borycka, K., et al. (2017). Masting in wind-pollinated trees: system-specific roles of weather and pollination dynamics in driving seed production. Ecology 98, 2615–2625. doi: 10.1002/ecy.1951
Braun, S., de Witte, L. C., and Hopf, S. E. (2020a). Auswirkungen des Trockensommers 2018 auf Flächen der Interkantonalen Walddauerbeobachtung. Schweiz. Z. Forstwes. 171, 270–280. doi: 10.3188/szf.2020.0270
Braun, S., Schindler, C., and Rihm, B. (2020b). Foliar nutrient concentrations of European beech in Switzerland: relations with nitrogen deposition, ozone, climate and soil chemistry. Front. For. Glob. Change 3:33. doi: 10.3389/ffgc.2020.00033
Braun, S., Schindler, C., and Rihm, B. (2017). Growth trends of beech and Norway spruce in Switzerland: the role of nitrogen deposition, ozone, mineral nutrition and climate. Sci. Total Environ. 599–600, 637–646. doi: 10.1016/j.scitotenv.2017.04.230
Brugger, K., Walter, M., Chitimia-Dobler, L., Dobler, G., and Rubel, F. (2018). Forecasting next season’s Ixodes ricinus nymphal density: the example of southern Germany 2018. Exp. Appl. Acarol. 75, 281–288. doi: 10.1007/s10493-018-0267-6
Bunn, A., Korpela, M., Biondi, F., Campelo, F., Mérian, P., Qeadan, F., et al. (2018). dplR: Dendrochronology Program Library in R. R Package Version 1.6.9. Avaliable online at https://CRAN.R-project.org/package=dplR (accessed February 15, 2019).
Burnham, K. P., and Anderson, D. R. (2002). Model Selection and Multimodel Inference: A Practical Information-Theoretic Approach. New York, NY: Springer Verlag.
Caignard, T., Kremer, A., Firmat, C., Nicolas, M., Venner, S., and Delzon, S. (2017). Increasing spring temperatures favor oak seed production in temperate areas. Sci. Rep. 7:8555. doi: 10.1038/s41598-017-09172-7
Crone, E. E., and Rapp, J. M. (2014). Resource depletion, pollen coupling, and the ecology of mast seeding. Ann. N. Y. Acad. Sci. 1322, 21–34. doi: 10.1111/nyas.12465
Dittmar, C., Zech, W., and Elling, W. (2003). Growth variations of common beech (Fagus sylvatica L.) under different climatic and environmental conditions in Europe – a dendroecological study. For. Ecol. Manag. 173, 63–78. doi: 10.1016/s0378-1127(01)00816-7
Dobbertin, M. (2005). Tree growth as indicator of tree vitality and of tree reaction to environmental stress: a review. Eur. J. For. Res. 124, 319–333. doi: 10.1007/s10342-005-0085-3
Dobbertin, M., and Neumann, M. (2016). “Part V: Tree Growth,” in Manual on Methods and Criteria for Harmonized Sampling, Assessment, Monitoring and Analysis of the Effects of Air Pollution on Forests, eds UNECE and ICP Forests Programme Co-ordinating Centre (Eberswalde: Thünen Institute of Forest Ecosystems).
Drobyshev, I., Niklasson, M., Mazerolle, M. J., and Bergeron, Y. (2014). Reconstruction of a 253-year long mast record of European beech reveals its association with large scale temperature variability and no long-term trend in mast frequencies. Agric. For. Meteor. 192-193, 9–17. doi: 10.1016/j.agrformet.2014.02.010
Drobyshev, I., Övergaard, R., Saygin, I., Niklasson, M., Hickler, T., Karlsson, M., et al. (2010). Masting behaviour and dendrochronology of European beech (Fagus sylvatica L) in southern Sweden. For. Ecol. Manag. 259, 2160–2171. doi: 10.1016/j.foreco.2010.01.037
Eichhorn, J., Dammann, I., Schönfelder, E., Albrecht, M., Beck, W., and Paar, U. (2008). “Assessment of drought resistance of beech exemplified by the 2003 extreme weather conditions,” in Ergebnisse Angewandter Forschung zur BUCHE, eds Nordwestdeutsche Forstliche and Versuchsanstalt (Göttingen: Universitätsverlag Göttingen), 109–134.
Fatichi, S., Leuzinger, S., and Körner, C. (2014). Moving beyond photosynthesis: from carbon source to sink-driven vegetation modeling. New Phytol. 201, 1086–1095. doi: 10.1111/nph.12614
Fernández-Martínez, M., Vicca, S., Janssens, I. A., Espelta, J. M., and Peñuelas, J. (2017). The role of nutrients, productivity and climate in determining tree fruit production in European forests. New Phytol. 213, 669–679. doi: 10.1111/nph.14193
Geburek, T., Hiess, K., Litschauer, R., and Milasowsky, N. (2012). Temporal pollen pattern in temperate trees: expedience or fate? Oikos 121, 1603–1612. doi: 10.1111/j.1600-0706.2011.20140.x
Hacket-Pain, A. J., Ascoli, D., Vacchiano, G., Biondi, F., Cavin, L., Conedera, M., et al. (2018). Climatically controlled reproduction drives interannual growth variability in a temperate tree species. Ecol. Lett. 21, 1833–1844. doi: 10.1111/ele.13158
Hacket-Pain, A. J., Friend, A. D., Lageard, J. G. A., and Thomas, P. A. (2015). The influence of masting phenomenon on growth–climate relationships in trees: explaining the influence of previous summers’ climate on ring width. Tree Physiol. 35, 319–330. doi: 10.1093/treephys/tpv007
Han, Q., and Kabeya, D. (2017). Recent developments in understanding mast seeding in relation to dynamics of carbon and nitrogen resources in temperate trees. Ecol. Res. 32, 771–778. doi: 10.1007/s11284-017-1494-8
Han, Q., Kabeya, D., and Hoch, G. (2011). Leaf traits, shoot growth and seed production in mature Fagus sylvatica trees after 8 years of CO2 enrichment. Ann. Bot. 107, 1405–1411. doi: 10.1093/aob/mcr082
Han, Q., Kabeya, D., Iio, A., Inagaki, Y., and Kakubari, Y. (2014). Nitrogen storage dynamics are affected by masting events in Fagus crenata. Oecologia 174, 679–687. doi: 10.1007/s00442-013-2824-3
Hanewinkel, M., Cullmann, D. A., Schelhaas, M. J., Nabuurs, G. J., and Zimmermann, N. E. (2013). Climate change may cause severe loss in the economic value of European forest land. Nat. Clim. Chang. 3, 203–207. doi: 10.1038/nclimate1687
Haylock, M. R., Hofstra, N., Tank, A. M. G. K., Klok, E. J., Jones, P. D., and New, M. (2008). A European daily high-resolution gridded dataset of surface temperature and precipitation. J. Geophys. Res. 113:D20119.
Henry, V. G., and Conley, R. H. (1972). Fall foods of european wild hogs in the southern appalachians. J. Wildl. Manag. 36, 854–860. doi: 10.2307/3799440
Herrera, C. M., Jordano, P., Guitián, J., and Traveset, A. (1998). Annual variability in seed production by woody plants and the masting concept: reassessment of principles and relationship to pollination and seed dispersal. Am. Nat. 152, 576–594. doi: 10.2307/2463358
Herrera, C. M., Jordano, P., Lopez-Soria, L., and Amat, J. A. (1994). Recruitment of a mast-fruiting, bird-dispersed tree: bridging frugivore activity and seedling establishment. Ecol. Monogr. 64, 315–344. doi: 10.2307/2937165
Hoch, G., Siegwolf, R. T. W., Keel, S. G., Körner, C., and Han, Q. M. (2013). Fruit production in three masting tree species does not rely on stored carbon reserves. Oecologia 171, 653–662. doi: 10.1007/s00442-012-2579-2
Ichie, T., Igarashi, S., Yoshida, S., Kenzo, T., Masaki, T., and Tayasu, I. (2013). Are stored carbohydrates necessary for seed production in temperate deciduous trees? J. Ecol. 101, 525–531. doi: 10.1111/1365-2745.12038
IPCC (2013). Climate Change 2013: The Physical Science Basis Contribution of Working Group I to the Fifth Assessment Report of the Intergovernmental Panel on Climate Change. Cambridge: Cambridge University Press.
IPCC (2019). “Summary for Policymakers,” in IPCC Special Report on the Ocean and Cryosphere in a Changing Climate, eds H.-O. Pörtner, D. C. Roberts, V. Masson-Delmotte, P. Zhai, M. Tignor, E. Poloczanska, et al. (Geneva: IPCC).
Isagi, Y., Sugimura, K., Sumida, A., and Ito, H. (1997). How does masting happen and synchronize? J. Theoret. Biol. 187, 231–239. doi: 10.1006/jtbi.1997.0442
Janzen, D. H. (1971). Seed predation by animals. Annu. Rev. Ecol. Evol. Syst. 2, 465–492. doi: 10.1146/annurev.es.02.110171.002341
Jenni, L. (1987). Mass concentrations of bramblings Fringilla montifringilla in Europe 1900-1983: their dependence upon beech mast and the effect of snow-cover. J. Avian Biol. 18, 84–94. doi: 10.2307/3676843
Ježík, M., Blaženec, M., Strelcova, K., and Ditmarova, L. (2011). The impact of the 2003–2008 weather variability on intra-annual stem diameter changes of beech trees at a submontane site in central Slovakia. Dendrochronologia 29, 227–235. doi: 10.1016/j.dendro.2011.01.009
Jonard, M., André, F., Dambrine, E., Ponette, Q., and Ulrich, E. (2009). Temporal trends in the foliar nutritional status of the French, Walloon and Luxembourg broad-leaved plots of forest monitoring. Ann. For. Sci. 66, 412–422. doi: 10.1051/forest/2009014
Kasprzyk, I., Ortyl, B., and Dulska-Jez, A. (2014). Relationships among weather parameters, airborne pollen and seed crops of Fagus and Quercus in Poland. Agric. For. Meteor. 197, 111–122. doi: 10.1016/j.agrformet.2014.05.015
Kelly, D. (1994). The evolutionary ecology of mast seeding. Tree Trends Ecol. Evol. 9, 465–470. doi: 10.1016/0169-5347(94)90310-7
Kelly, D., and Sork, V. L. (2002). Mast seeding in perennial plants: why, how, where? Annu. Rev. Ecol. Evol. Syst. 33, 427–447. doi: 10.1146/annurev.ecolsys.33.020602.095433
Koenig, W. D., Kelly, D., Sork, V. L., Duncan, R. P., Elkinton, J. S., Peltonen, M. S., et al. (2003). Dissecting components of population-level variation in seed production and the evolution of masting behavior. Oikos 102, 581–591. doi: 10.1034/j.1600-0706.2003.12272.x
Köppen, W. (1936). “Das geographische System der Klimate (The geographic system of climates),” in Handbuch der Klimatologie, ed. G. Geiger (Berlin: Gebrüder Borntraeger).
Lebourgeois, F., Bréda, N., Ulrich, E., and Granier, A. (2005). Climate-tree-growth relationships of European beech (Fagus sylvatica L) in the French Permanent Plot Network (RENECOFOR). Trees 19, 385–401. doi: 10.1007/s00468-004-0397-9
Lebourgeois, F., Cousseau, G., and Ducos, Y. (2004). Climate-tree-growth relationships of Quercus petraea Mill. stand in the Forest of Bercé (“Futaie des Clos”, Sarthe, France). Ann. For. Sci. 61, 361–372. doi: 10.1051/forest:2004029
Lebourgeois, F., Delpierre, N., Dufrêne, E., Cecchini, S., Macé, S., Croisé, L., et al. (2018). Assessing the roles of temperature, carbon inputs and airborne pollen as drivers of fructification in European temperate deciduous forests. Eur. J. For. Res. 137, 249–365.
Matthews, J. D. (1955). The influence of weather on the frequency of beech mast years in England. Forestry 28, 107–116. doi: 10.1093/forestry/28.2.107
Merganičová, K., Merganic, J., Lehtonen, A., Vacchiano, G., Ostrogovic Sever, M. Z., Augustynczik, A. L. D., et al. (2019). Forest carbon allocation modelling under climate change. Tree Physiol. 39, 1937–1960. doi: 10.1093/treephys/tpz105
Mérian, P., Bontemps, J. D., Bergès, L., and Lebourgeois, F. (2011). Spatial variation and temporal instability in climate-growth relationships of sessile oak (Quercus petraea [Matt.] Liebl.) under temperate conditions. Plant Ecol. 212, 1855–1871. doi: 10.1007/s11258-011-9959-2
Michelot, A., Bréda, N., Damesin, C., and Dufrêne, E. (2012). Differing growth responses to climatic variations and soil water deficits of Fagus sylvatica, Quercus petraea and Pinus sylvestris in a temperate forest. For. Ecol. Manag. 265, 161–171. doi: 10.1016/j.foreco.2011.10.024
Monks, A., and Kelly, D. (2006). Testing the resource-matching hypothesis in the mast seeding tree Nothofagus truncata (Fagaceae). Austral Ecol. 31, 366–375. doi: 10.1111/j.1442-9993.2006.01565.x
Müller-Haubold, H., Hertel, D., and Leuschner, C. (2015). Climatic drivers of mast fruiting in european beech and resulting C and N Allocation Shifts. Ecosystems 18, 1083–1100. doi: 10.1007/s10021-015-9885-6
Müller-Haubold, H., Hertel, D., Seidel, D., Knutzen, F., and Leuschner, C. (2013). Climate responses of aboveground productivity and allocation in Fagus sylvatica: a transect study in mature forests. Ecosystems 16, 1498–1516. doi: 10.1007/s10021-013-9698-4
Mund, M., Herbst, M., Knohl, A., Matthäus, B., Schumacher, J., Schall, P., et al. (2020). It is not just a ‘trade-off’: indication for sink- and source-limitation to vegetative and regenerative growth in an old-growth beech forest. New Phytol. 226, 111–125. doi: 10.1111/nph.16408
Nakagawa, S., and Schielzeth, H. (2013). A general and simple method for obtaining R2 from generalized linear mixed-effects models. Methods Ecol. Evol. 4, 133–142. doi: 10.1111/j.2041-210x.2012.00261.x
Nilsson, S. G., and Wästljung, U. (1987). Seed predation and cross-pollination in mast-seeding beech (Fagus sylvatica) patches. Ecology 68, 260–265. doi: 10.2307/1939256
Nussbaumer, A. (2021). Mast Behaviour in European Forest Tree Species: Triggers, Inhibitors, and Resource Dynamics Mechanisms. Dissertation. Zurich: ETH Zurich, doi: 10.3929/ethz-b-000480828
Nussbaumer, A., Meusburger, K., Schmitt, M., Waldner, P., Gehrig, R., Haeni, M., et al. (2020). Extreme summer heat and drought leads to early fruit abortion in European beech. Sci. Rep. 10:5334. doi: 10.1038/s41598-020-62073-0
Nussbaumer, A., Waldner, P., Apuhtin, V., Aytar, F., Benham, S., Bussotti, F., et al. (2018). Impact of weather cues and resource dynamics on mast occurrence in the main forest tree species in Europe. For. Ecol. Manag. 429, 336–350. doi: 10.1016/j.foreco.2018.07.011
Nussbaumer, A., Waldner, P., Etzold, S., Gessler, A., Benham, S., Thomsen, I. M., et al. (2016). Patterns of mast fruiting of common beech, sessile and common oak, Norway spruce and Scots pine in Central and Northern Europe. For. Ecol. Manag. 363, 237–251. doi: 10.1016/j.foreco.2015.12.033
Ogawa, R., Mortelliti, A., Witham, J. W., and Hunter, M. L. Jr. (2017). Demographic mechanisms linking tree seeds and rodent population fluctuations: insights from a 33-year study. J. Mammal. 98, 419–427. doi: 10.1093/jmammal/gyw200
Ostfeld, R. S. (2013). “Ecology of lyme disease,” in Fundamentals of Ecosystem Science, eds K. C. Weathers, D. L. Strayer, and G. E. Likens (Cambridge, MA: Academic Press, Inc), 243–251. doi: 10.1016/b978-0-08-091680-4.00013-5
Park, C. S., Vogel, E., Larson, L. M., Myers, S. S., Daniel, M., and Biggs, B.-A. (2019). The global effect of extreme weather events on nutrient supply: a superposed epoch analysis. Lancet Planet. Health 3, 429–438.
Pearse, I. S., Koenig, W. D., and Kelly, D. (2016). Mechanisms of mast seeding: resources, weather, cues, and selection. New Phytol. 212, 546–562. doi: 10.1111/nph.14114
Picard, J. F., Oleffe, P., and Boisaubert, B. (1991). Influence of oak mast on feeding behaviour of red deer (Cervus elaphus L). Ann. For. Sci. 48, 547–559. doi: 10.1051/forest:19910505
Pinheiro, J., Bates, D., DebRoy, S., Sarkar, D., and R Core Team (2020). nlme: Linear and Nonlinear Mixed Effects Models. R Package Version 3.1-148. Avaliable online at: https://CRAN.R-project.org/package=nlme (accessed June 30, 2020).
Piovesan, G., and Adams, J. M. (2001). Masting behaviour in beech: linking reproduction and climatic variation. Can. J. Bot. 79, 1039–1047. doi: 10.1139/cjb-79-9-1039
Piovesan, G., Biondi, F., Di Filippo, A., Alessandrini, A., and Maugeri, M. (2008). Drought-driven growth reduction in old beech (Fagus sylvatica L.) forests of the central Apennines. Italy. Glob. Chang. Biol. 14, 1–17.
Prager, M. H., and Hoenig, J. M. (1989). Superposed epoch analysis: a randomization test of environmental effects on recruitment with application to Chub mackerel. Trans. Am. Fish. Soc. 118, 608–618. doi: 10.1577/1548-8659(1989)118<0608:seaart>2.3.co;2
R Core Team (2019). R: A Language and Environment for Statistical Computing. Vienna: R Foundation for Statistical Computing.
Rautio, P., Fürst, A., Stefan, K., Raitio, H., and Bartels, U. (2016). “Part XII: sampling and analysis of needles and leaves,” in Manual on Methods and Criteria for Harmonized Sampling, Assessment, Monitoring and Analysis of the Effects of air Pollution on Forests, eds UNECE and ICP Forests Programme Co-ordinating Centre (Eberswalde: Thünen Institute of Forest Ecosystems).
Rees, M., Kelly, D., and Bjørnstad, O. N. (2002). Snow tussocks, chaos, and the evolution of mast seeding. Am. Nat. 160, 44–59. doi: 10.2307/3078997
Rozas, V. (2005). Dendrochronology of pedunculate oak (Quercus robur L) in an old-growth pollarded woodland in northern Spain: tree-ring growth responses to climate. Ann. For. Sci. 62, 209–218. doi: 10.1051/forest:2005012
Rozas, V., Lamas, S., and García-González, I. (2009). Differential tree-growth responses to local and large-scale climatic variation in two Pinus and two Quercus species in northwest Spain. Ecoscience 16, 299–310. doi: 10.2980/16-3-3212
Sáenz-Romero, C., Lamy, J. B., Ducousso, A., Musch, B., Ehrenmann, F., Delzon, S., et al. (2017). Adaptive and plastic responses of Quercus petraea populations to climate across Europe. Glob. Chang. Biol. 23, 2831–2847.
Sala, A., Woodruff, D. R., and Meinzer, F. C. (2012). Carbon dynamics in trees: feast or famine? Tree Physiol. 32, 764–775. doi: 10.1093/treephys/tpr143
Satake, A., and Iwasa, Y. (2000). Pollen coupling of forest trees: forming synchronized and periodic reproduction out of chaos. J. Theoret. Biol. 203, 63–84. doi: 10.1006/jtbi.1999.1066
Scharnweber, T., Manthey, M., and Wilmking, M. (2013). Differential radial growth patterns between beech (Fagus sylvatica L.) and oak (Quercus robur L.) on periodically waterlogged soils. Tree Physiol. 33, 425–437. doi: 10.1093/treephys/tpt020
Schuldt, B., Buras, A., Arend, M., Vitasse, Y., Beierkuhnlein, C., Damm, A., et al. (2020). A first assessment of the impact of the extreme 2018 summer drought on Central European forests. Basic Appl. Ecol. 45, 86–103. doi: 10.1016/j.baae.2020.04.003
Seidling, W., Ziche, D., and Beck, W. (2012). Climate responses and interrelations of stem increment and crown transparency in Norway spruce, Scots pine, and common beech. For. Ecol. Manag. 284, 196–204. doi: 10.1016/j.foreco.2012.07.015
Shibata, M., Masaki, T., Yagihashi, Y., and Shimada, T. (2020). Decadal changes in masting behaviour of oak trees with rising temperature. J. Ecol. 108, 1088–1100. doi: 10.1111/1365-2745.13337
Siegmund, J. F., Sanders, T. G. M., Heinrich, I., van der Maaten, E., Simard, S., Helle, G., et al. (2016). Meteorological drivers of extremes in daily stem radius variations of beech, oak, and pine in Northeastern Germany: an event coincidence analysis. Front. Plant Sci. 7:733. doi: 10.3389/fpls.2016.00733
Ukonmaanaho, L., Pitman, R., Bastrup-Birk, A., Breda, N., and Rautio, P. (2016). “Part XIII: Sampling and Analysis of Litterfall,” in Manual on Methods and Criteria for Harmonized Sampling, Assessment, Monitoring and Analysis of the Effects of air Pollution on Forests, eds UNECE and ICP Forests Programme Co-ordinating Centre (Eberswalde: Thünen Institute of Forest Ecosystems).
UNECE</snm>, and ICP Forests Programme Co-ordinating Centre (2016). Manual on Methods and Criteria for Harmonized Sampling, Assessment, Monitoring and Analysis of the Effects of Air Pollution on Forests. Eberswalde: Thünen Institute of Forest Ecosystems.
Vacchiano, G., Ascoli, D., Berzaghi, F., Lucas-Borja, M. E., Caignard, T., Collalti, A., et al. (2018). Reproducing reproduction: how to simulate mast seeding in forest models. Ecol. Modell. 376, 40–53. doi: 10.1016/j.ecolmodel.2018.03.004
Vacchiano, G., Hacket-Pain, A., Turco, M., Motta, R., Maringer, J., Conedera, M., et al. (2017). Spatial patterns and broad-scale weather cues of beech mast seeding in Europe. New Phytol. 215, 595–608. doi: 10.1111/nph.14600
Vapalahti, O., Mustonen, J., Lundkvist, Å, Henttonen, H., Plyusnin, A., and Vaheri, A. (2003). Hantavirus infections in Europe. Lancet Infect. Dis. 3, 653–661.
Wohlgemuth, T., Nussbaumer, A., Burkart, A., and Bollmann, K. (2016). Eichenmast und Wildschweine. Zür. Wald 2/16, 28–30.
Keywords: climate change, Fagus sylvatica, long-term monitoring, mast fruiting, Quercus petraea, Quercus robur, resource dynamics
Citation: Nussbaumer A, Gessler A, Benham S, de Cinti B, Etzold S, Ingerslev M, Jacob F, Lebourgeois F, Levanic T, Marjanović H, Nicolas M, Ostrogović Sever MZ, Priwitzer T, Rautio P, Roskams P, Sanders TGM, Schmitt M, Šrámek V, Thimonier A, Ukonmaanaho L, Verstraeten A, Vesterdal L, Wagner M, Waldner P and Rigling A (2021) Contrasting Resource Dynamics in Mast Years for European Beech and Oak—A Continental Scale Analysis. Front. For. Glob. Change 4:689836. doi: 10.3389/ffgc.2021.689836
Received: 01 April 2021; Accepted: 18 June 2021;
Published: 12 July 2021.
Edited by:
Alessandra De Marco, Italian National Agency for New Technologies, Energy and Sustainable Economic Development (ENEA), ItalyReviewed by:
Sabine Braun, Institute for Applied Plant Biology AG, SwitzerlandCopyright © 2021 Nussbaumer, Gessler, Benham, de Cinti, Etzold, Ingerslev, Jacob, Lebourgeois, Levanic, Marjanović, Nicolas, Ostrogović Sever, Priwitzer, Rautio, Roskams, Sanders, Schmitt, Šrámek, Thimonier, Ukonmaanaho, Verstraeten, Vesterdal, Wagner, Waldner and Rigling. This is an open-access article distributed under the terms of the Creative Commons Attribution License (CC BY). The use, distribution or reproduction in other forums is permitted, provided the original author(s) and the copyright owner(s) are credited and that the original publication in this journal is cited, in accordance with accepted academic practice. No use, distribution or reproduction is permitted which does not comply with these terms.
*Correspondence: Anita Nussbaumer, YW5pdGEubnVzc2JhdW1lckB3c2wuY2g=
Disclaimer: All claims expressed in this article are solely those of the authors and do not necessarily represent those of their affiliated organizations, or those of the publisher, the editors and the reviewers. Any product that may be evaluated in this article or claim that may be made by its manufacturer is not guaranteed or endorsed by the publisher.
Research integrity at Frontiers
Learn more about the work of our research integrity team to safeguard the quality of each article we publish.