- 1School of Ecosystem and Forest Sciences, The University of Melbourne, Parkville, VIC, Australia
- 2Centre for Environmental Risk Management of Bushfires, Centre for Sustainable Ecosystem Solutions, University of Wollongong, Wollongong, NSW, Australia
- 3Hawkesbury Institute for the Environment, Western Sydney University, Penrith, NSW, Australia
Fire management agencies undertake a range of fire management strategies in an attempt to reduce the risk posed by future wildfires. This can include fuel treatments (prescribed burning and mechanical removal), suppression and community engagement. However, no agency has an unlimited budget and numerically optimal solutions can rarely be implemented or may not even exist. Agencies are trying to quantify the extent to which their management actions reduce risk across multiple values in the most cost-effective manner. In this paper, we examine the cost-effectiveness of a range of prescribed burning strategies across multiple landscapes in south-eastern Australia. Landscapes considered include vegetated areas surrounding the cities of Hobart, Melbourne, Adelaide, Canberra, and Sydney. Using a simulation approach, we examine the potential range of fires that could occur in a region with varying levels of edge and landscape prescribed burning treatment regimes. Damages to assets are measured for houses, lives, transmission lines, carbon and ecological assets. Costs of treatments are estimated from published models and all data are analyzed using multi-criteria decision analysis. Cost-effectiveness of prescribed burning varies widely between regions. Variations primarily relate to the spatial configuration of assets and natural vegetation. Regions with continuous urban interface adjacent to continuous vegetation had the most cost-effective fuel treatment strategies. In contrast, those regions with fragmented vegetation and discontinuous interfaces demonstrated the lowest cost-effectiveness of treatments. Quantifying the extent to which fuel treatments can reduce the risk to assets is vital for determining the location and extent of treatments across a landscape.
Introduction
Wildfires are a natural disturbance in many ecosystems but when they encounter human settlements/infrastructure/communities they can have devastating consequences. Recent fires around the globe (e.g., Europe, USA, Chile and Australia,) have resulted in major losses of life, property, infrastructure and caused significant environmental changes (Bowman, 2018). These problems will be exacerbated under patterns of global change where expanding urban populations are increasingly moving into flammable parts of the landscape and fire regimes will shift in response to changing climates (Gill et al., 2013; Bowman and Moreira-Muñoz, 2019; Syphard and Keeley, 2019).
Prescribed burning is a form of fuel treatment: i.e., it aims to alter the quantity and structural complexity of fine fuel in a way that moderates the rate of spread and intensity of subsequent wildfires. An individual prescribed burn will be considered effective for the period in which consequent effects on rate of spread and intensity of subsequent fire are reduced compared to untreated fuels (e.g., Penman et al., 2007; Price and Bradstock, 2012). The effectiveness of prescribed burning is potentially a function of the dynamics of surface fine fuel loads which increase with time-since-fire until an equilibrium load is reached (e.g., Penman and York, 2010; Thomas et al., 2014). However, recent research has shown that these dynamics vary between regions and fuel strata. Variations in fuel and responses to prescribed fire will vary with environmental drivers such as climate and productivity (Dixon et al., 2018; McColl-Gausden and Penman, 2019; McColl-Gausden et al., 2019). Generally, the window of effectiveness of treatment has been shown to diminish as fire weather at the time of encounter by a wildfire increases in severity (Price and Bradstock, 2012; Collins et al., 2013; Tolhurst and McCarthy, 2016). Given that there are major variations in fire weather at regional scales, particularly the upper extreme, effectiveness of prescribed burning can be expected to vary as a coupled function of vegetation type (inherent fuel dynamics structure) and climate (potential fire weather).
Generally, the primary objective of prescribed burning is to reduce risks to human and natural assets via modifications to fire behavior, although prescribed burning can be undertaken to promote ecological assets or for cultural purposes (Penman et al., 2011). The degree to which prescribed burning can potentially alter fire behavior, constitutes an important but only partial element of the overall effectiveness in mitigating risk. Other factors in concert with changes to fire behavior caused by fuel treatments will affect risk. For example, the rates of treatment, spatial distribution of treatments and configuration of developments will alter the risk mitigation outcomes for people and property, of different prescribed burning strategies (Bradstock et al., 2012b; Penman et al., 2014; Thompson et al., 2017; Florec et al., 2019; Cirulis et al., 2020).
Fire and land managers are required to design prescribed burning strategies to protect a range of assets across the landscape. Prescribed fire is most commonly used to reduce the risk to assets that are vulnerable to exposure to high intensity fires, such as people, property and major infrastructure (Penman et al., 2011, 2020; Driscoll et al., 2016). Treatments have been used to offset carbon emissions in some environments (Russell-Smith, 2016) but may increase carbon emissions in others (Bradstock et al., 2012a). Many water supply catchments exist in naturally vegetated areas and are susceptible to fire (Smith et al., 2011; Langhans et al., 2016). Fuel treatments have been used to decrease the risk of reduction in water quality and quantity (Nyman et al., 2011; Smith et al., 2011). Inappropriate fire regimes can result in significant impacts on biodiversity, although ecological burns can be used to benefit some species (Gundale et al., 2005; Bentley and Penman, 2017).
Decisions around prescribed burning are therefore complex and require consideration of the costs of implementation and the impacts on assets of interest, although relatively few studies have attempted this approach. Results from such studies vary widely with some suggesting fuel treatments near houses are the most cost-effective strategy (Penman et al., 2014; Scott et al., 2016) and others suggesting landscape treatments are more cost-effective (Florec et al., 2019) for reducing cost. Optimal strategies for human and ecological values differed in some studies (Driscoll et al., 2016), but not others (Bentley and Penman, 2017). Most studies have focused on only one or two case study regions (Bradstock et al., 2012b; Thompson et al., 2013; Driscoll et al., 2016; Bentley and Penman, 2017; Florec et al., 2019; McFayden et al., 2019; Cirulis et al., 2020) making it difficult to generalize results. The performance of prescribed burning strategies needs to be systematically assessed in relation to these variations given the likely variation in potential effectiveness of prescribed burning in influencing fire behavior, along with differing configurations of assets and management values. Specifically, we need to determine if the risk mitigation potential of differing prescribed burning strategies is likely to be robust to such variations or if the optimum strategy (i.e., most cost-effective) varies widely according to biophysical and human context.
In this study, we aim to determine the cost effectiveness of prescribed fire treatments across multiple assets and multiple landscapes. These case studies are placed across a sub-continental gradient of biophysical (e.g., temperature and rainfall) and human variation (e.g., house density and land uses). We compare varying prescribed burning treatment rates in both landscape and edge treatments (i.e,. within 1 km of houses) across forested and woodland landscapes on SE Australia. Specifically, we ask:
1. What are the most cost-effective prescribed burning strategies/solutions across multiple landscapes and assets?
2. Do these solutions vary as a function of landscape context?
Methods
Comparisons of treatment cost-effectiveness were conducted across 13 regions spanning the diversity of ecosystems in south-eastern Australia (Figure 1). Patterns of vegetation and fire regimes reflected variation in latitude (from temperate to sub-tropical), rainfall (from 250 mm in the semi-arid west to 1,500 mm in the coastal zone of the east) and altitude (the dividing range runs along the eastern coast up to an elevation of 2,000 meters above sea level. (Murphy et al., 2013). Case study regions were selected to cover the diversity of vegetation types, climates and built environments. Each case study was between 45 and 60 km rectangles with a dominant cover of native vegetation that can be treated with prescribed burning. A summary of each of the study areas appears in Supplementary Material.
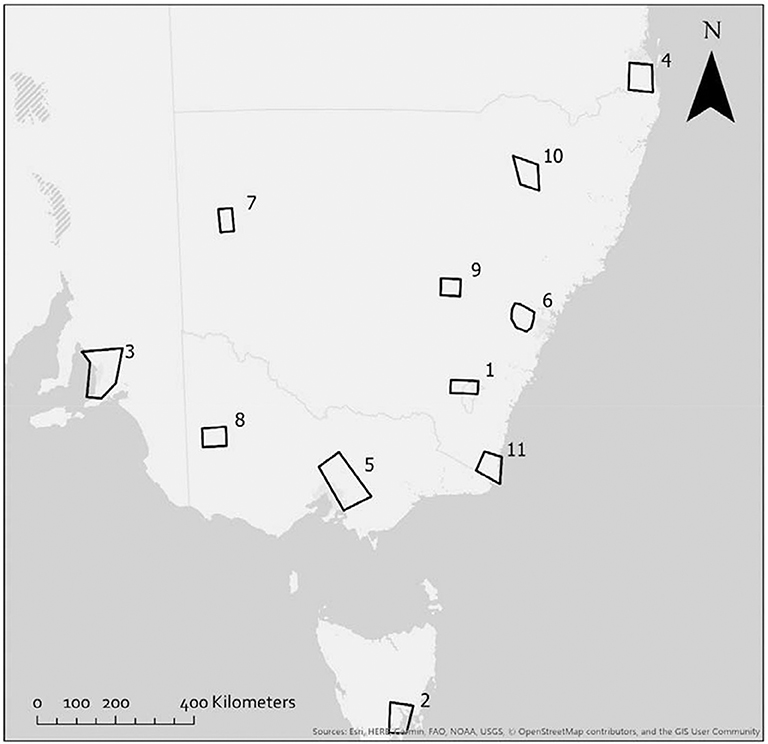
Figure 1. Case study locations in south-eastern Australia. 1, ACT Canberra; 2, TAS Hobart; 3, SA Adelaide Hills; 4, South-eastern Queensland; 5, VIC Central Highlands; 6, NSW Blue Mountains; 7, NSW Broken Hill; 8, VIC Little Desert; 9, NSW SW Slopes; 10, NSW Nandewar; 11, NSW south-east corner.
To examine the cost-effectiveness of prescribed burning on landscape values we followed the methods of Cirulis et al. (2020). The approach required four main steps. Firstly, we prepared region specific data about weather, fire history and ignition locations. Secondly, wildfires were simulated across the full spectrum of historical weather and prescribed burning scenarios giving a broad range of outputs. Thirdly, the impact on five asset types was estimated for each fire. Finally, data were analyzed using a Bayesian Decision Network to estimate the cost-effectiveness of different prescribed burning strategies relative to a do-nothing approach. From this, the most cost-effective solution for each landscape was identified. The workflow is presented in Figure 2 and described in more detail below.
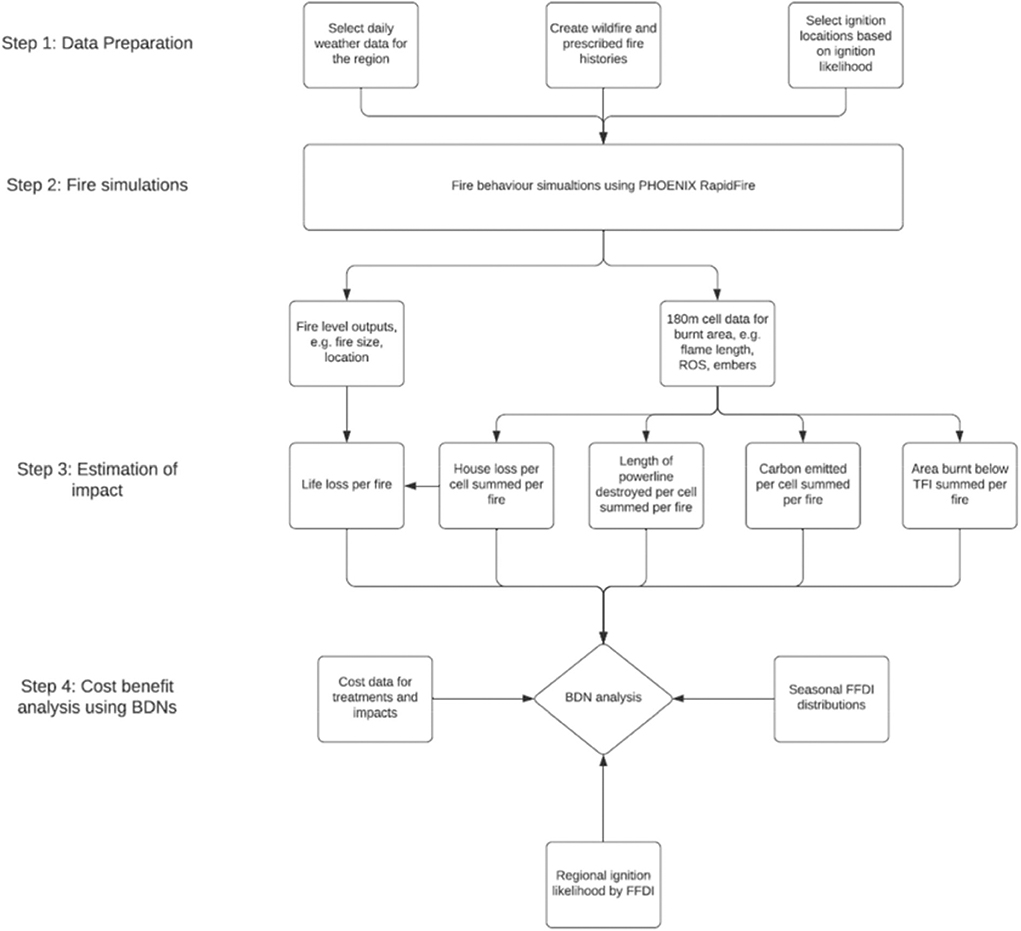
Figure 2. Workflow for the study. ROS, rate of spread; BDN, Bayesian decision network; TFI, tolerable fire interval; FFDI, forest fire danger index.
Fire Simulation
Fire simulations were undertaken using PHOENIX RapidFire v4.0.0.7 (hereafter PHOENIX; Tolhurst et al., 2008)—the operational version of the software at the time of analysis. PHOENIX is used operationally by fire agencies in eastern and southern Australia (Bentley and Penman, 2017). Other fire behavior models are available, e.g., SPARK (Hilton et al., 2015), however the spatial datasets required for them are not available for all case study landscapes. Datasets for PHOENIX are available for all six states and territories included in the study. These datasets have been developed and tested by the relevant land and fire management agencies in each state.
Two-dimensional fire growth in PHOENIX is simulated using Huygens' propagation principle of fire edge (Knight and Coleman, 1993). Two fire behavior models are used to estimate rate of spread—a modified McArthur Mk5 forest fire behavior model (McArthur, 1967; Noble et al., 1980) and a generalization of the CSIRO southern grassland fire spread model (Cheney et al., 1998). PHOENIX produces outputs of ember density, convection, intensity and flame length for each 180 m cell affected by the simulation. PHOENIX is described more fully in a range of other studies (Tolhurst et al., 2008; Paterson and Chong, 2011; Tolhurst and Chong, 2011)
PHOENIX predicts fire behavior based on inputs of weather, ignition location, fire history, vegetation, fuel accumulation, topography and natural breaks in the landscape (rivers and roads that could slow or halt the spread of fire). Data on vegetation, fuel accumulation, topography and natural breaks were provided from the relevant state fire or land management agency.
Weather values were selected from the nearest Australian Bureau of Meteorology Automatic Weather Station (AWS) records for each region. Days were selected to capture variation in fire weather based on the forest fire danger index (FFDI)—a composite measure that combines temperature, relative humidity and wind speed with a long-term drying index to predict the difficulty of fire suppression (McArthur, 1967; Noble et al., 1980). Three primary drivers can influence the FFDI—(i) strong wind, (ii) strong wind with a significant directional change or (iii) high air temperature (Cirulis et al., 2020). Within each FFDI category, up to three different days were chosen for each of these FFDI drivers resulting in a maximum of 54 different weather streams, although this number was often smaller if there were not sufficient days that fit the selection criteria (Table 1). All weather streams covered a 24-h period beginning from midnight to allow the model to generate stable and realistic estimates of diurnal fluctuations in fuel moisture based on temperature and relative humidity (Tolhurst et al., 2008). Each weather stream contained hourly data for air temperature, relative humidity, wind speed, wind direction, drought factor and curing.
Fire histories were created to represent the different fuel management scenarios. Scenarios were based on the application of prescribed burning in the landscape (hereafter landscape) or the interface zone (hereafter edge)—within 500 m of an urban interface based on definitions of Radeloff et al. (2005). Within each of the landscape and edge zones we simulated seven levels (0, 1, 2, 3, 5, 10, and 15% per annum) of treatment and considered the 49 combinations resulting from the seven levels of landscape treatment and seven levels of edge treatment. We first generated a wildfire history using a random samping with replacement procedure. We firstly selected all wildfires within the case study landscape from the fire history geodatabase to create the available wildfires. Fires were randomly selected from the available wildfires to create an annual area burnt using a moving window target, based on the historic average annual area burnt as reported in Bradstock et al. (2014). After each season all fires were replaced in the available wildfires set and the process repeated for 30 years to create a wildfire history. Treatable vegetation in each case study landscape was divided into management sized “burn blocks” (data supplied by management agencies) and allocated to either edge or landscape treatments. Areas available as edge or landscape blocks for each case study region are presented in Table 2. Prescribed burning treatments were simulated over a period of 20 years following the methods of Penman et al. (2014) which selects blocks for treatments based on time since fire—prescribed fire or wildfire. Blocks were considered available after 5 years for edge treatments, whereas in the landscape treatments ecological thresholds based on vegetation class were used. In creating a prescribed fire history, we first identified all blocks available for treatment. From this dataset we then randomly selected blocks until the desired treatment rate was achieved (± 0.1) or no more blocks were available. These steps were repeated to create a 20-year prescribed fire history for each level of landscape and edge treatments. Prescribed fire histories were then spatially merged with wildfire history to create a landscape fire history. The process was replicated 5 times for each of the 49 treatment combinations to give a total of 245 simulated fire history layers for each case study landscape.
Ignition locations were selected using a probabilistic model. Firstly, we generated 10,000 random points across the landscape. We then calculated the ignition probability for the random points based on an empirical model developed and tested across the study region (Clarke et al., 2019). From the 10,000 points, the 1,000 ignition points with the highest ignition probabilities were selected for use in the simulations. Individual fires were ignited at 1100 and allowed to propagate for up to 12 h, unless self-extinguished within this period. Fires were not simulated on successive days as the permutations were prohibitive and generally most area burned and damage from fires in these region occurs on a single day (Cunningham, 1984; Bradstock et al., 2009; Collins et al., 2016). Ignition points were randomly split into 5 groups of 200 ignitions. Each of these 200 ignitions was simulated for a single replicate of each weather category/driver combination and fuel treatment to reduce total simulation time.
Assessment of Fire Effects
The effect of fire on various assets was calculated for five management values: house loss, loss of human life, length of powerline damaged, quantity of carbon released and environmental impact measured by area burnt below minimum tolerable fire interval (TFI). Loss functions for house and life loss came from published sources (Tolhurst and Chong, 2011; Harris et al., 2012; Cirulis et al., 2020). Data for house locations were from Geocoded National Address File Database (https://www.psma.com.au/products/g-naf accessed 21 January 2019) which was combined with data from the Australian Bureau of Statistics to calculate the average number of people per household and therefore estimate population exposed to the fire. There are no published data on loss functions for power related assets. We used the intensity threshold value of 10,000 kW/m to determine damage to mapped powerlines (Cirulis et al., 2020). Carbon released was calculated from Byram's fire line intensity equation (Byram, 1959) using intensity and rate of spread values from PHOENIX to determine fuel consumed and multiplying by 0.5, the fraction of carbon in fuel (Roxburgh et al., 2006). This is a very coarse measure of carbon released but more specific could not be estimated from existing fire behavior models. Environmental impact was measured by the number of hectares area burnt by wildfire when the time since the last wildfire was less than the tolerable fire interval (TFI)—an ecological measure that considers the amount of time required between fires to maintain vegetation diversity (Kenny et al., 2004; Gosper et al., 2013). Area burnt below TFI was calculated from area burnt and existing TFI threshold mapping supplied by the management agencies.
Risk Estimation
A Bayesian Decision Network (BDN) model was used to examine prescribed burning effectiveness in altering risk. Bayesian networks (BNs) are statistical tools commonly used for risk analysis of complex environmental systems (Pollino et al., 2007; Johnson et al., 2010; Kelly et al., 2013; Sierra et al., 2018) as the outputs of the model are expressed as probabilities (Marcot et al., 2001). Variables (“nodes”) contain the joint probability distributions representing combinations of conditions (Korb and Nicholson, 2011). BDNs are extensions of BNs that include decision structures and utility costs or benefits of those decisions. Decision nodes represent discrete actions to allow for the comparison of outcomes (e.g., costs) among competing approaches. Models can be used to identify the relative cost and benefit of competing strategies.
In developing the BDN model, we followed the BN modeling guidelines of Marcot et al. (2006) and Chen and Pollino (2012). The primary steps are:
1. Construct a conceptual model of the problem;
2. Develop influence diagrams that depict the relationships within the conceptual model;
3. Populate all the conditional probability tables within the model; and
4. Specify alternative decision actions and values of utilities.
We used the conceptual model and built on the influence diagram presented in Cirulis et al. (2020). Fire weather is represented by FFDI which influences the likelihood of ignitions given the FFDI (FFDI_ignition). Ignition FFDI and the rate of edge and landscape prescribed burning influence the distribution of fire sizes. All four of these factors then influence the distribution of loss for each asset type. Utility or cost nodes were added for treatments and assets to create the BDN. Utility nodes represent the cost (or benefit) associated with each a state in either a stochastic or decision node. To measure the cost of a decision, all utility node values are summed to provide a single value for each decision or combination of decisions allowing users to identify the best or best set of management strategies (Penman and Cirulis, 2020). The same structure was used for each case study with data specific to each case study (Figure 3).
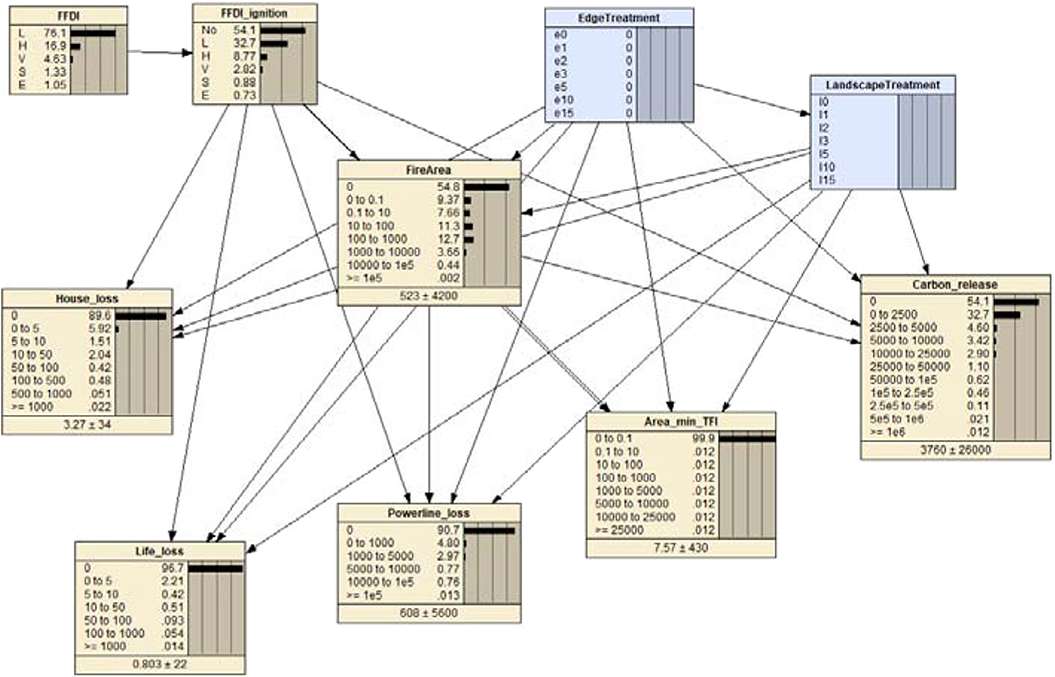
Figure 3. The BDN structure (influence diagram) used in the study. Boxes represent nodes and the average distribution of values across all decisions. Bar plots represent the average distribution of values across all decisions. Numbers next to bars represent the probability of being in the given state. Values at the base of the boxes represent the expected values plus or minus one standard deviation.
Data for the conditional probability tables (CPTs) came from either empirical data or the PHOENIX simulation study. Fire weather was calculated using the maximum daily FFDI values across the fire season for the study area using data from the relevant weather stations to populate the FFDI node. The mean ignition probability was calculated for each FFDI category using the empirical model of Clarke et al. (2019) to create the distribution in the FFDI-ignitions node. Data for each of the fire size and each of the nodes representing fire impact on assetswere taken from the simulations for each case study (as described above). Each node was discretised on a semi-log scale in an iterative fashion to get a relatively even distribution across the non-zero values.
Data for the utility nodes were calculated from a variety of sources. Treatment costs were calculated using the equations in Penman et al. (2014) which had a log-log relationship between treatment size and cost per ha of treatment. Costs for individual burns in the simulated prescribed burn history were calculated and then average annual costs for the 20-year period calculated. Costs of house loss were estimated as $500,000 per house based on the estimates of median property values across the various study areas (based on www.yourinvestmentpropertymag.com.au accessed November 2017). We did not vary cost by region as this would prioritize areas with greater property values. Life loss was estimated as $4.2 million per life based on national standards (https://www.pmc.gov.au/sites/default/files/publications/Value_of_Statistical_Life_guidance_note.pdf, accessed November 2019), accessed November 2019). Cost of powerline replacement was estimated at $120 per meter (www.energy.vic.gov.au/safety-and-emergencies/powerline-bushfire-safety-program/pb-report-indicative-costs-for-replacing-swer-lines). We did not attempt to estimate the cost of loss of power as this requires detailed modeling of electricity networks, which is beyond the scope of the paper. Carbon released was calculated using the values of Hunt (2008) who estimated a cost of $AUD 61 per ton. There is no means of translating TFI into economic values, we used a coarse value of $1,000 per ha burnt below TFI based on the economic impact of major fires on environmental values (Stephenson, 2010).
The BDN was used to calculate the cost effectiveness of each treatment level accounting for the likelihood of weather and ignitions, as well as fire behavior. In this study, we focussed on the estimated cost for each asset by treatment, as well as the annualized risk cost per treatment. This value includes the cost of implementing the treatment(s) and the summed impact across all asset types. Cost effectiveness was measured as the difference between a given treatment and the “do nothing” approach with 0% edge and landscape treatment levels. Treatments were considered to have a positive effect if the annualized risk cost was less than the “do nothing” approach.
We undertook two analyses to determine if the ordering of prescribed burning approaches among case study landscapes were consistent. Firstly, we used Spearman rank correlations to compare the ranking of the 49 treatments between the case study landscapes. If a single solution existed, we would expect the ranking of treatments to be consistent with strong correlations between all case studies. Secondly, we undertook non-metric Multi-Dimensional Scaling (nMDS) using Bray-Curtis similarity index to look for groupings in the relative costs of treatments and impacts. Total costs were standardized on a scale of 0–1, with 0 representing the minimum cost for the case study region and 1 representing the maximum cost. This was done to remove the regional variations in the magnitude of the costs. nMDS will help identify regions with similar patterns in the cost data across treatments and impacts with less emphasis on the ordering. Results were plotted so that regions with similar cost profiles were positioned close together with the distance increasing as the differences increased.
Results
Annualized risk cost ranged from $116,992 (NSW Broken Hill, L0E0) to $40,200,590 (VIC Central Highlands (L15E10) (Figure 4). Costs varied between the regions with consistently higher costs in the SA Adelaide Hills and VIC Central Highlands compared to all other case studies. Costs were lowest in NSW Broken Hill. The “do nothing” approach was the cheapest option in most case study regions with the exceptions being ACT Canberra, NSW Blue Mountains and TAS Hobart.
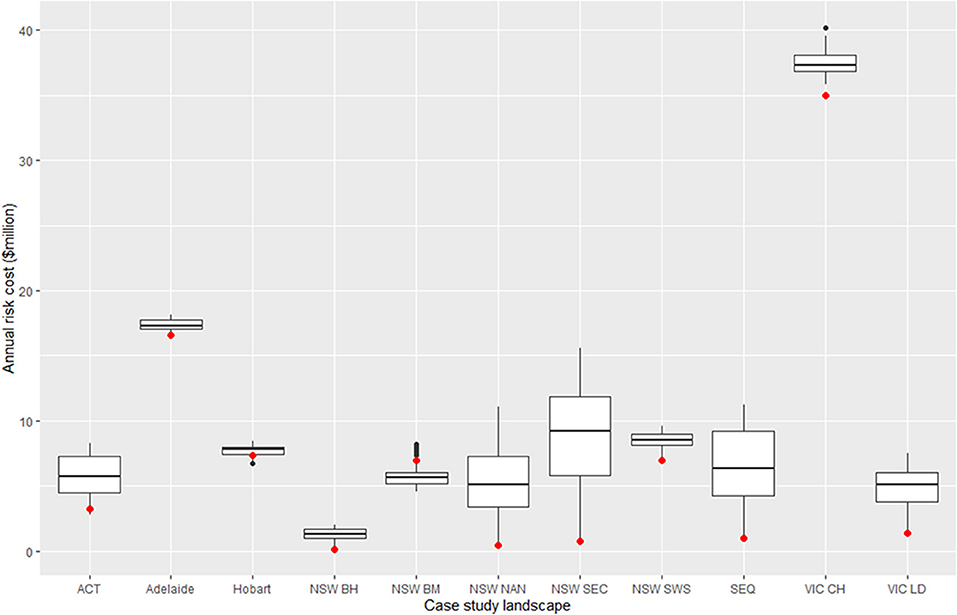
Figure 4. Annualized risk cost across all values and treatments for the case study regions across all treatment rates. Red circle indicates the “do nothing” approach L0E0. See Table 1 for case study codes.
Increasing investment in treatment costs resulted in a reduction of risk and the cost of impact in all regions (Figure 5; Supplementary B). Edge treatments reduced the impact costs to a greater extent than landscape treatments where the cost of houses or lives were the primary components of the overall costs, the exceptions being VIC Central Highlands and NSW south-west Slopes. Landscape treatments had a small effect on impact costs, e.g., ACT Canberra and NSW Blue Mountains, or no meaningful effect, e.g., SA Adelaide Hills and TAS Hobart. A cost-effective solution only occurred where the reduction in impact costs was greater than the implementation cost. In both ACT Canberra and TAS Hobart, the increase in edge treatments to 10 or 15 % resulted in cost-effective solutions. However, adding any level of landscape treatment did not result in cost-effective solutions. Almost all treatments in the NSW Blue Mountains resulted in cost-effective solutions, although the edge treatments resulted in the greatest reduction in impact cost. The most cost-effective solution in this region consisted of edge treatments rates of 5% per annum or more combined with landscape treatment rates of 1–3% per annum. In all other regions, treatments did not reduce the risk of asset loss or the reduction was less than the treatment cost (Figure 5; Supplementary B).
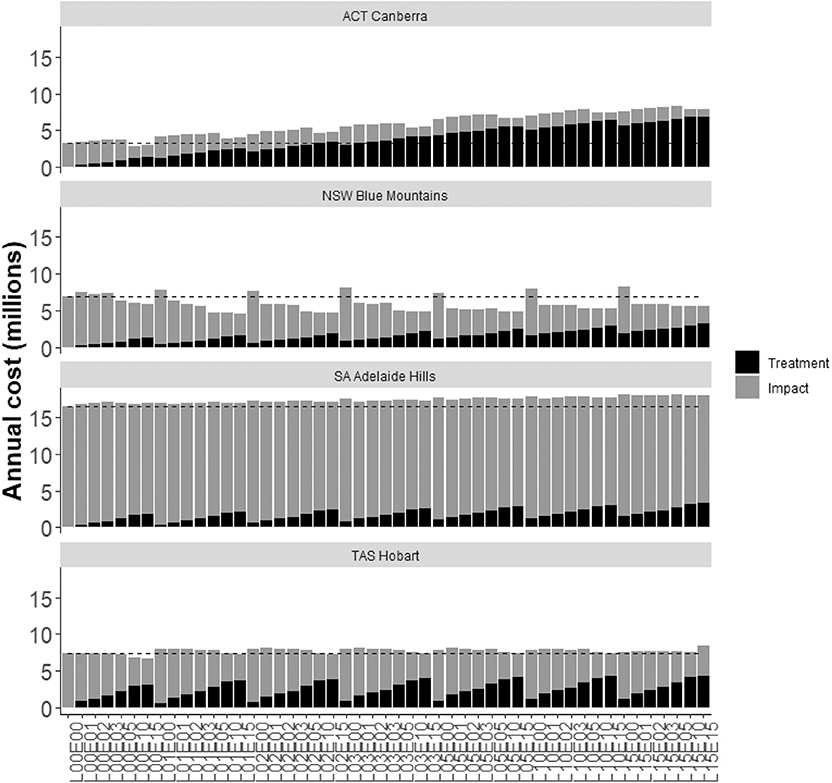
Figure 5. Annualized risk costs for four case study regions across all treatment rates. The dashed line represents the do nothing approach—columns below this line are considered cost-effective. Treatment codes are presented as LXXEYY where XX represents the annual prescribed burning treatment rate in the landscape and YY the rate in the edge blocks.
Contributions to the total cost varied between regions, although some common patterns emerged (Figure 6; Supplementary C). Unsurprisingly, as treatment rates increased the contribution to total costs increased, reaching values as high as 80% of total risk costs in the ACT Canberra. The exception being SA Adelaide Hills where treatment costs were never more than 20% of total costs. Carbon, powerlines and environmental costs contributed to <10% of total costs in all regions except SA Adelaide Hills where environmental costs alone contributed approximately 25% of total costs. Cost of the impact on lives and houses combined represented the major asset impact cost, although the relative contribution varied. In most regions, the cost of life loss exceeded the cost of house loss, except in TAS Hobart where the reverse occurred.
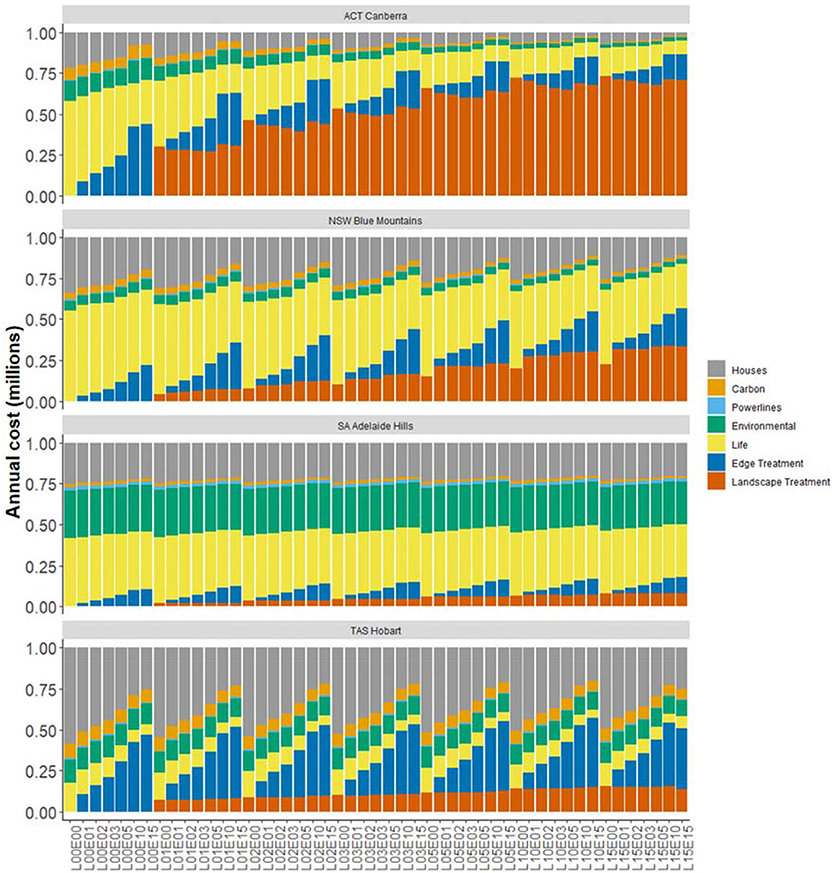
Figure 6. Relative contribution of treatments and asset risk costs for four case study regions across all treatment rates. Treatment codes are presented as LXXEYY where XX represents the annual prescribed burning treatment rate in the landscape and YY the rate in the edge blocks.
There was no single cost-effective solution for prescribed burning that was common across all regions. Rank correlations of the treatment options indicated that a large group of regions with similar solutions (Figure 7). These were SA Adelaide Hills, ACT Canberra, NSW Broken Hill, NSW Nandewar, NSW south-east corner, VIC Little Desert and VIC Central Highlands. Weaker relationships were seen between this group and the NSW south-west slopes and south-east Queensland. Ranking of treatments in TAS Hobart were not related to any region and NSW Blue Mountains showed negative correlations with most regions (Figure 7).
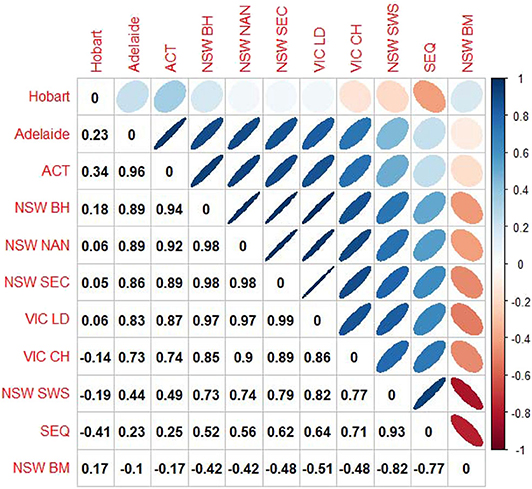
Figure 7. Rank correlations of total cost across the 49 tested prescribed burning scenarios. Color of the ellipses depict the nature of the data, with blue representing positive relationships and red negative. Shape of the ellipses represent the nature of the fit, with thin ellipses representing a strong relationship whereas broad ellipses indicate greater uncertainty in the correlation. Upper right triangle represents the correlation coefficients. Case study codes appear in Table 1.
The nMDS analysis of the normalized cost data found different patterns (Figure 8). SE Queensland and the Blue Mountains were unique in their positions. Two broad groupings were identified. The first included areas from the semi-arid zone with ACT Canberra and the NSW south-east corner. In the second group were forested areas around three capital cities (Adelaide, Hobart and the Central Highlands which lies to the NE of Melbourne) and the NSW south-west slopes.
Discussion
There was no “one size fits all” solution to prescribed burning when considering multiple assets. Edge treatments generally reduced impact costs to a greater extent than landscape treatments, but reductions in impact were not always equal to the treatment cost. Cost-effective solutions were only found in a quarter of the case study landscapes. There were three primary contributors to the total cost for all regions—treatments, houses and lives. Cost-effectiveness is likely to be driven by the spatial distribution of these assets within the landscape relative to the locations of treatments and the total value of assets within each case study landscape.
Treatment Effectiveness
Fire agencies around the world have increasingly adopted a risk management framework as no prescribed burning regime is expected to remove the risk to assets (Fernandes and Botelho, 2003; Hughes and Mercer, 2009; Penman et al., 2011; Thompson et al., 2013; Victorian DELWP, 2015). A significant residual risk to all assets remains regardless of treatment level which is consistent with previous studies (Stockmann et al., 2010; Bradstock et al., 2012b; Calkin et al., 2014; Florec et al., 2019). The treatments we simulated had only a limited influence on the size of fires in the case-study landscapes.
Landscape treatments were more likely to extend benefit to assets that occur in native vegetation within the study areas, e.g., powerlines, carbon and other environmental assets. Impact costs for these assets were correlated with fire size distributions and therefore the effectiveness of landscape treatments was likely to be related to the extent to which landscape fuel treatments reduce fire size and severity. This is a concept termed leverage: leverage potentially varies as a function of environmental variation (Loehle, 2004; Boer et al., 2009; Price et al., 2015). Only modest changes in the risk to the environmental assets were recorded across all study regions consistent with the fact that leverage is low or absent in most of these regions (Price et al., 2015).
Edge treatments were generally more effective at reducing risk compared with landscape treatments. This was consistent with a growing body on literature highlighting the ability of these treatments to reduce the risk of house and/or life loss (Safford et al., 2009; Ager et al., 2010; Gibbons et al., 2012; Penman et al., 2014; Florec et al., 2019). Results of these studies suggest that the effectiveness was due to the reduction in fire behavior immediately adjacent to the asset resulting in a direct transfer of benefit to houses and lives. Edge treatments did not alter landscape fire behavior and could not be expected to alter the risk to assets across the landscape such as environmental assets, roads and powerlines (Penman et al., 2014; Florec et al., 2019).
Cost-Effectiveness
Benefits of the treatments need to be offset by the cost of treatments, which were generally the greatest contributors to the overall cost estimation. In regions with low population densities (e.g., NSW Broken Hill, VIC Little Desert, NSW south-east corner), the treatment costs were 50–90% of the total costs. Edge treatments were generally more expensive as the risk of loss, should fires escape, is higher and therefore more resources and higher costs of treatment per hectare are required (Berry et al., 2006; Calkin and Gebert, 2006; Penman et al., 2014; Florec et al., 2019). In contrast, treating 1% of a landscape requires treatment of significantly more land than 1% of edge blocks.
Loss of houses and lives represent the greatest impact cost in case study areas with significant urban areas, e.g., NSW Blue Mountains, TAS Hobart, ACT Canberra, SA Adelaide Hills. These values are also the highest cost per unit in the study—$500K per house and $4.2 million per life. House and life loss were significantly correlated (Harris et al., 2012) as historically more than 60% of life loss during wildfires was associated with a residence (Blanchi et al., 2014). Single fires in these urban interface areas can result in large loss of houses (and at times lives) directly from the fire (Ahern and Chladhil, 1999; Blanchi et al., 2014) or indirectly through house to house transfer (Cohen and Stratton, 2008; Penman et al., 2018). In contrast, the contribution of houses and lives were relatively small in case studies with dispersed populations.
Environmental costs were a key driver in other regions, e.g., VIC Central Highlands, NSW south-west Slopes. Few studies have considered environmental and human assets (Ager et al., 2016; Bentley and Penman, 2017) and rarely have they included a risk approach as done here (Milne et al., 2014). One of the key reasons for this gap in knowledge is that it is very difficult to estimate the economic value of environmental assets over complex landscapes (Fromm, 2000). Indeed, we used a coarse economic value of $1,000 per ha based on Stephenson (2010) who studied a relatively small number of large fires. Such an evaluation assumes that all fires are equal and all have negative impacts on the environment, which is not the case for ecosystems considered in this study (Bradstock R. et al., 2012). A true risk assessment should include the positive and negative effects of fire and account for them in a net value change framework (Finney, 2005). Ecological effects are a function of fire regimes rather than individual fires (Clarke, 2008; Enright et al., 2014) and could not be estimated from our fire simulation approach.
The cost of prescribed burning was rarely less than the reduction in risk cost for the values considered. Only in three case studies did we identify cost-effective solutions. These case studies differed in the optimal solution but were all near significant urban interfaces. Two of the case study landscapes had only one or two cost-effective solutions and only the NSW Blue Mountains had greater options with 40 of the 49 treatments providing cheaper alternatives to the “do nothing” approach. It is impossible to determine the reasons for this with a sample size of one. Regardless, the cheapest solution in the Blue Mountains was close to the current management strategy for the area (NSW RFS unpublished data).
Protection of life and property is the primary goal of fire managers around the world (Fernandes and Botelho, 2003; Penman et al., 2011) and cost may not be the optimal way to identify preferred approaches to achieve this goal. Loss of property and life can have significant flow on effects to communities. For example, the psychological effects of wildfires have been infrequently studied but the impact is extensive (Perry and Lindell, 1978; Morrissey and Reser, 2007; Papadatou et al., 2012). It may be desirable for fire agencies to spend more money than they save in order to decrease the impacts of wildfire on areas such as mental health and other measures of community wellbeing. A range of participatory approaches are available which can allow stakeholders to weight the various values in order to enhance decision making processes (Gregory et al., 2001; Kiker et al., 2005)
Model Limitations
Inclusion of a greater variety of values would increase the general applicability of the results. Our modeling approach considered five assets covering a range of values representing populations, infrastructure and the environment. There are a range of other values that could be included should the data become available. It is necessary for a value to have a spatial representation, a loss function to link to the fire behavior model outputs and a cost for any losses to be included in the modeling approach. Without all three of these, it is not possible to include in the overall analysis.
Dollar value was used in our study for comparisons between treatments and assets, however there are a range of other values that are not well represented in dollar terms. Non-market values such as biodiversity, mental health and community cohesion are difficult to place in an economic framework (Venn and Calkin, 2011; Milne et al., 2014). Furthermore, environmental, social and cultural benefits from treatments may not be realized for many years or decades (Burgess et al., 2005; Kalies and Yocom Kent, 2016), whereas negative impacts of fire are often realized immediately, e.g., loss of houses, or in the months following the fire, e.g., debris flows (Nyman et al., 2011).
Including smoke impacts on populations is likely to have significant consequences for future studies of this kind. Smoke from prescribed burns can result in mortality and respiratory illness in local populations (Bowman and Johnston, 2005; Weisshaupt et al., 2005; Broome et al., 2016). Similarly, smoke from wildfires can also increase mortality in populations (Borchers Arriagada et al., 2020). Modeling the impact of smoke from fires requires an understanding of the rate of consumption of fuels and a three-dimensional smoke dispersal model (Wain et al., 2008) and was beyond the current study. Smoke from prescribed burning and wildfires can also impact on viticultural production with significant economic impacts expected in wine growing regions (Kennison et al., 2007, 2009). Inclusion of smoke in future modeling approaches would be prudent and an important priority.
Asset loss functions used were relatively simple but exclude actions of individuals. Loss functions were based on fire behavior and the distribution of assets, without accounting for human behavior. Individuals can significantly alter their risk to their property and the risk of life loss through their actions. The chance of a house surviving a wildfire can be increased 3–6 times if residents stay and defend (when it is safe to do so; Wilson and Ferguson, 1986; Ramsay et al., 1987; Blanchi and Leonard, 2008; Whittaker et al., 2012). These values can be enhanced by the property being well prepared for wildfire (Jakes et al., 2007; McCaffrey and Rhodes, 2009; McGee, 2011; Penman et al., 2013b, 2018). Similarly, mental preparedness for fires will reduce the risk of life loss during a fire (McGee and Russell, 2003; Paton et al., 2006; Morrissey and Reser, 2007; Eriksen and Prior, 2013; Prior and Eriksen, 2013). All these actions can affect individuals but cannot readily be included in the modeling process at this stage, as there are a wide range of factors affecting an individual's decision-making process.
Across the globe there has been significant investment in fire suppression (Loane and Gould, 1986; Calkin and Gebert, 2006; Milne et al., 2014; Thompson and Anderson, 2015), however our study did not explicitly include consideration of the role of past suppression in altering fuel loads. North American studies have found aggressive fire suppression strategies reduce the number and extent of wildfires in an area which results in an increase in landscape fuel load and subsequent wildfire size (e.g., Calkin et al., 2015). No such relationships have not been reported from Australia. In contrast, fuel responses vary according to vegetation type, productivity and climate (Thomas et al., 2014; McColl-Gausden and Penman, 2019; McColl-Gausden et al., 2019) with some systems having reduced fuel loads with increasing time since fire (Dixon et al., 2018; Zylstra, 2018) and others increasing (McCaw et al., 2002). The model used assumes an initial increase of fuels to a plateau and therefore does not assume an ongoing increase in landscape fuel hazard with an absence or reduction in fire.
We were not able to account for the interaction of prescribed burning with suppression. Prescribed burning was selected as it is one of the most widespread preventative strategy employed in Australia and elsewhere. One of the goals of prescribed burning is to reduce fire behavior in order to increase suppression effectiveness (Fernandes and Botelho, 2003; Penman et al., 2020). However, effectiveness of suppression is also influenced by the response time, fire size on arrival, number and type of resources deployed and the weather at the time of the fire (Hirsch and Martell, 1996; Hirsch et al., 1998; Finney et al., 2009; Plucinski, 2012). Coarse assessments of suppression effectiveness interactions with prescribed burning have given mixed results with some suggesting limited effects (Penman et al., 2013a) and others suggesting strong effects in ideal scenarios (Penman and Cirulis, 2020). Furthermore, few have been able to calculate the costs of suppression but acknowledge this is a significant component of fire agencies budgets (Gould, 1987; Butry et al., 2001; Calkin and Gebert, 2006; Snider et al., 2006). Therefore, it would be important to develop the capacity to include the interactive effects of suppression and prescribed burning in future cost-effectiveness studies.
Conclusion
There is no “one size fits all” solution to prescribed burning across landscapes. Environmental, human and societal challenges are likely to dictate ideal solutions. Based on current knowledge cost-effective prescribed burning solutions may be limited in scope, but this conclusion may change as some of the limitations noted above are overcome. Nonetheless, other factors such as social acceptability of treatments and wildfires may be greater determinants of treatment regions rather than cost alone.
Data Availability Statement
The datasets generated for this study are available on request to the corresponding author.
Author Contributions
All authors developed the approach through a series of workshops. BC ran the simulations and Bayesian Network analyses. TP undertook the analyses with feedback from all authors. TP wrote the manuscript and all authors contributed to the editing of the manuscript.
Funding
Funding for the salaries of BC and HC was from the Bushfire and Natural Hazards Co-operative Research Center. Salary of OP was from the NSW Rural Fire Service. No other funding was received for this work.
Conflict of Interest
The authors declare that the research was conducted in the absence of any commercial or financial relationships that could be construed as a potential conflict of interest.
Acknowledgments
This research was partly supported by the Bushfire and Natural Hazards Cooperative Research Center. Thanks are owed to the Victorian Department of Environment, Land, Water and Planning, NSW Rural Fire Service, Queensland Fire and Emergency Services, Tasmanian Fire Service and the ACT Parks, and Conservation Service for providing data for the PHOENIX simulations.
Supplementary Material
The Supplementary Material for this article can be found online at: https://www.frontiersin.org/articles/10.3389/ffgc.2020.00079/full#supplementary-material
References
Ager, A. A., Day, M. A., and Vogler, K. (2016). Production possibility frontiers and socioecological tradeoffs for restoration of fire adapted forests. J. Environ. Manage. 176, 157–168. doi: 10.1016/j.jenvman.2016.01.033
Ager, A. A., Vaillant, N. M., and Finney, M. A. (2010). A comparison of landscape fuel treatment strategies to mitigate wildland fire risk in the urban interface and preserve old forest structure. For. Ecol. Manage. 259, 1556–1570. doi: 10.1016/j.foreco.2010.01.032
Ahern, A., and Chladhil, M. (1999). How Far Do Bushfires Penetrate Urban Areas? In 'Australian Disaster Conference, Emergency Managemenent of Australia'. Canberra: ACT.
Bentley, P. D., and Penman, T. D. (2017). Is there an inherent conflict in managing fire for people and conservation? Intern. J. Wildland Fire 26, 455–468. doi: 10.1071/WF16150
Berry, A. H., Donovan, G., and Hesseln, H. (2006). Prescribed burning costs and the WUI: economic effects in the Pacific Northwest. Western J. Appl. Forestry 21, 72–78. doi: 10.1093/wjaf/21.2.72
Blanchi, R., and Leonard, J. (2008). “Property safety: judging structural safety,” in Community Bushfire Safety, eds J. Handmer and K. Haynes (Melbourne: Bushfire Co-operative Research Centre), 77–85.
Blanchi, R., Leonard, J., Haynes, K., Opie, K., James, M., Oliveira, F. D., et al. (2014). Environmental circumstances surrounding bushfire fatalities in Australia 1901–2011. Environ. Sci. Policy 37, 192–203. doi: 10.1016/j.envsci.2013.09.013
Boer, M. M., Sadler, R. J., Wittkuhn, R., McCaw, L., and Grierson, P. F. (2009). Long-term impacts of prescribed burning on regional extent and incidence of wildfires – evidence from fifty years of active fire management in SW Australian forests. For. Ecol. Manage 259, 132–142. doi: 10.1016/j.foreco.2009.10.005
Borchers Arriagada, N., Bowman, D. M. J. S., Palmer, A. J., and Johnston, F. H. (2020). “Climate change, wildfires, heatwaves and health impacts in Australia,” in Extreme Weather Events and Human Health: International Case Studies, ed R. Akhtar (Cham: Springer International Publishing), 99–116.
Bowman, D. (2018). Wildfire science is at a loss for comprehensive data. Nature 560:7. doi: 10.1038/d41586-018-05840-4
Bowman, D. M., and Johnston, F. H. (2005). Wildfire smoke, fire management, and human health. Ecohealth 2, 76–80. doi: 10.1007/s10393-004-0149-8
Bowman, D. M. J. S., Moreira-Muñoz, A., et al. (2019). Human–environmental drivers and impacts of the globally extreme 2017 Chilean fires. Ambio 48, 350–362. doi: 10.1007/s13280-018-1084-1
Bradstock, R., Penman, T., Boer, M., Price, O., and Clarke, H. (2014). Divergent responses of fire to recent warming and drying across south-eastern Australia. Glob. Chang. Biol 20, 1412–1428. doi: 10.1111/gcb.12449
Bradstock, R., Williams, R., and Gill, A. (2012). Flammable Australia: Fire Regimes, Biodiversity and Ecosystems in a Changing World. Melbourne: CSIRO Publishing.
Bradstock, R. A., Boer, M. M., Cary, G. J., Price, O. F., Williams, R. J., Barrett, D., et al. (2012a). Modelling the potential for prescribed burning to mitigate carbon emissions from wildfires in fire-prone forests of Australia. Intern. J. Wildland Fire 21, 629–639. doi: 10.1071/WF11023
Bradstock, R. A., Cary, G. J., Davies, I., Lindenmayer, D. B., Price, O. F., and Williams, R. J. (2012b). Wildfires, fuel treatment and risk mitigation in Australian eucalypt forests: insights from landscape-scale simulation. J. Environ. Manage 105, 66–75. doi: 10.1016/j.jenvman.2012.03.050
Bradstock, R. A., Cohn, J. S., Gill, A. M., Bedward, M., and Lucas, C. (2009). Prediction of the probability of large fires in the Sydney region of south-eastern Australia using fire weather. Intern. J. Wildland Fire 18, 932–943. doi: 10.1071/WF08133
Broome, R. A., Johnston, F. H., Horsley, J., and Morgan, G. G. (2016). A rapid assessment of the impact of hazard reduction burning around Sydney, May 2016. Med. J. Australia 205, 407–408. doi: 10.5694/mja16.00895
Burgess, C. P., Johnston, F. H., Bowman, D. M. J. S., and Whitehead, P. J. (2005). Healthy country: healthy people? Exploring the health benefits of indigenous natural resource management. Aust. N. Z. J. Public Health 29, 117–122. doi: 10.1111/j.1467-842X.2005.tb00060.x
Butry, D. T., Mercer, E. D., Prestemon, J. P., Pye, J. M., and Holmes, T. P. (2001). What is the price of catastrophic wildfire? J. Forestry 99, 9–17. doi: 10.1093/jof/99.11.9
Byram, G. M. (1959). “Combustion of forest fuels,” in Forest Fire: Control and Use, ed K. P. Davis (New York, NY: McGraw-Hill), 61–89.
Calkin, D., and Gebert, K. (2006). Modeling fuel treatment costs on forest service lands in the Western United States. Western J. Appl. Forestry 21, 217–221. doi: 10.1093/wjaf/21.4.217
Calkin, D. E., Cohen, J. D., Finney, M. A., and Thompson, M. P. (2014). How risk management can prevent future wildfire disasters in the wildland-urban interface. Proc. Natl. Acad. Sci. U.S.A. 111:746. doi: 10.1073/pnas.1315088111
Calkin, D. E., Thompson, M. P., and Finney, M. A. (2015). Negative consequences of positive feedbacks in US wildfire management. Forest Ecosyst. 2:9. doi: 10.1186/s40663-015-0033-8
Chen, S. H., and Pollino, C. A. (2012). Good practice in Bayesian network modelling. Environ. Modell. Softw. 37, 134–145. doi: 10.1016/j.envsoft.2012.03.012
Cheney, N. P., Gould, J. S., and Catchpole, W. R. (1998). Prediction of fire spread in Grasslands. Int. J. Wildland Fire 8, 1–13. doi: 10.1071/WF9980001
Cirulis, B., Clarke, H., Boer, M., Penman, T., Price, O., and Bradstock, R. (2020). Quantification of inter-regional differences in risk mitigation from prescribed burning across multiple management values. Intern. J. Wildland Fire. 29, 414–426. doi: 10.1071/WF18135
Clarke, H., Gibson, R., Cirulis, B., Bradstock, R. A., and Penman, T. D. (2019). Developing and testing models of the drivers of anthropogenic and lightning-caused wildfire ignitions in south-eastern Australia. J. Environ. Manage 235, 34–41. doi: 10.1016/j.jenvman.2019.01.055
Clarke, M. F. (2008). Catering for the needs of fauna in fire management: science or just wishful thinking? Wildlife Res. 35, 385–394. doi: 10.1071/WR07137
Cohen, J. D., and Stratton, R. D. (2008). Home Destruction Examination, Grass Valley Fire, Lake Arrowhead, California. Missoula, MT: US Department of Agriculture.
Collins, K. M., Penman, T. D., and Price, O. F. (2016). Some wildfire ignition causes pose more risk of destroying houses than others. PLoS ONE 11:e0162083. doi: 10.1371/journal.pone.0162083
Collins, L., Bradstock, R. A., and Penman, T. D. (2013). Can precipitation influence landscape controls on wildfire severity? A case study within temperate eucalypt forests of south-eastern Australia. Intern. J. Wildland Fire 23, 9–90. doi: 10.1071/WF12184
Cunningham, C. J. (1984). Recurring natural fire hazards - a case-study of the Blue Mountains, New-South-Wales, Australia. Appl. Geogr. 4, 5–27. doi: 10.1016/0143-6228(84)90002-X
Dixon, K. M., Cary, G. J., Worboys, G. L., Seddon, J., and Gibbons, P. (2018). A comparison of fuel hazard in recently burned and long-unburned forests and woodlands. Intern. J. Wildland Fire 27, 609–622. doi: 10.1071/WF18037
Driscoll, D. A., Bode, M., Bradstock, R. A., Keith, D. A., Penman, T. D., and Price, O. F. (2016). Resolving future fire management conflicts using multi-criteria decision making. Conserv. Biol. 30, 196–205. doi: 10.1111/cobi.12580
Enright, N. J., Fontaine, J. B., Lamont, B. B., Miller, B. P., and Westcott, V. C. (2014). Resistance and resilience to changing climate and fire regime depend on plant functional traits. J. Ecol. 102, 1572–1581. doi: 10.1111/1365-2745.12306
Eriksen, C., and Prior, T. (2013). Defining the importance of mental preparedness for risk communication and residents well-prepared for wildfire. Intern. J. Disaster Risk Reduction 6, 87–97. doi: 10.1016/j.ijdrr.2013.09.006
Fernandes, P. M., and Botelho, H. S. (2003). A review of prescribed burning effectiveness in fire hazard reduction. Intern. J. Wildland Fire 12, 117–128. doi: 10.1071/WF02042
Finney, M., Grenfell, I. C., and McHugh, C. W. (2009). Modeling containment of large wildfires using generalized linear mixed-model analysis. Forest Science 55, 249–255. doi: 10.1093/forestscience/55.3.249
Finney, M. A. (2005). The challenge of quantitative risk analysis for wildland fire. For. Ecol. Manage 211, 97–108. doi: 10.1016/j.foreco.2005.02.010
Florec, V., Burton, M., Pannell, D., Kelso, J., and Milne, G. (2019). Where to prescribe burn: the costs and benefits of prescribed burning close to houses. Intern. J. Wildland Fire. 29, 440–458. doi: 10.1071/WF18192
Fromm, O. (2000). Ecological structure and functions of biodiversity as elements of its total economic value. Environ. Res. Econ. 16, 303–328. doi: 10.1023/A:1008359022814
Gibbons, P., van Bommel, L., Malcolm Gill, A., Cary, G. J., Driscoll, D. A., et al. (2012). Land management practices associated with house loss in wildfires. PLoS ONE 7:e29212. doi: 10.1371/journal.pone.0029212
Gill, A. M., Stephens, S. L., and Cary, G. J. (2013). The worldwide “wildfire” problem. Ecol. Appl. 23, 438–454. doi: 10.1890/10-2213.1
Gosper, C. R., Prober, S. M., and Yates, C. J. (2013). Estimating fire interval bounds using vital attributes: implications of uncertainty and among-population variability. Ecol. Appl. 23, 924–935. doi: 10.1890/12-0621.1
Gould, J. S. (1987). “The cost-effectiveness of airtankers in the south-east region of South Australia and the south-west region of Victoria,” in Commonwealth Scientific and Industrial Research Organization, Division of Forest Research, Vol. 9. (Canberra: Australian Capital Territory).
Gregory, R., McDaniels, T., and Fields, D. (2001). Decision aiding, not dispute resolution: creating insights through structured environmental decisions. J. Policy Analysis Managem. 20, 415–432. doi: 10.1002/pam.1001
Gundale, M. J., DeLuca, T. H., Fiedler, C. E., Ramsey, P. W., Harrington, M. G., and Gannon, J. E. (2005). Restoration treatments in a Montana ponderosa pine forest: effects on soil physical, chemical and biological properties. For. Ecol. Manage 213, 25–38. doi: 10.1016/j.foreco.2005.03.015
Harris, S., Anderson, W., Kilinc, M., and Fogarty, L. (2012). The relationship between fire behaviour measures and community loss: an exploratory analysis for developing a bushfire severity scale. Natural Hazards 63, 391–415. doi: 10.1007/s11069-012-0156-y
Hilton, J. E., Miller, C., Sullivan, A. L., and Rucinski, C. (2015). Effects of spatial and temporal variation in environmental conditions on simulation of wildfire spread. Environ. Modell. Softw. 67, 118–127. doi: 10.1016/j.envsoft.2015.01.015
Hirsch, K. G., Corey, P. N., and Martell, D. L. (1998). Using expert judgement to model initial attack fire crew effectiveness. Forest Sci. 44, 539–549.
Hirsch, K. G., and Martell, D. L. (1996). A review of initial attack fire crew productivity and effectiveness. Intern. J. Wildland Fire 6, 199–215. doi: 10.1071/WF9960199
Hughes, R., and Mercer, D. (2009). Planning to reduce risk: the wildfire management overlay in Victoria, Australia. Geograph. Res. 47, 124–141. doi: 10.1111/j.1745-5871.2008.00556.x
Hunt, C. (2008). Economy and ecology of emerging markets and credits for bio-sequestered carbon on private land in tropical Australia. Ecol. Econo. 66, 309–318. doi: 10.1016/j.ecolecon.2007.09.012
Jakes, P., Kruger, L., Monroe, M. C., Nelson, K. C., and Sturtevant, V. (2007). Improving wildfire preparedness: lessons from communities across the U.S. Human Ecol. Rev. 14, 188–197. Available online at: https://www.jstor.org/stable/24707705
Johnson, S., Mengersen, K., de Waal, A., Marnewick, K., Cilliers, D., Houser, A. M., et al. (2010). Modelling cheetah relocation success in Southern Africa using an iterative bayesian network development cycle. Ecol. Modell. 221, 641–651. doi: 10.1016/j.ecolmodel.2009.11.012
Kalies, E. L., and Yocom Kent, L. L. (2016). Tamm review: are fuel treatments effective at achieving ecological and social objectives? A systematic review. For. Ecol. Manage 375, 84–95. doi: 10.1016/j.foreco.2016.05.021
Kelly, R. A., Jakeman, A. J., Barreteau, O., Borsuk, M. E., ElSawah, S., Hamilton, S. H., et al. (2013). Selecting among five common modelling approaches for integrated environmental assessment and management. Environ. Modell. Softw. 47, 159–181. doi: 10.1016/j.envsoft.2013.05.005
Kennison, K. R., Wilkinson, K. L., Pollnitz, A. P., Williams, H. G., and Gibberd, M. R. (2009). Effect of timing and duration of grapevine exposure to smoke on the composition and sensory properties of wine. Aust. J. Grape Wine Res 15, 228–237. doi: 10.1111/j.1755-0238.2009.00056.x
Kennison, K. R., Wilkinson, K. L., Williams, H. G., Smith, J. H., and Gibberd, M. R. (2007). Smoke-derived taint in wine: effect of postharvest smoke exposure of grapes on the chemical composition and sensory characteristics of wine. J. Agric. Food Chem 55, 10897–10901. doi: 10.1021/jf072509k
Kenny, B., Sutherland, E., Tasker, E., and Bradstock, R. (2004). Guidelines for Ecologically Sustainable Fire Management. Hurstville: NSW National Parks and Wildlife Service.
Kiker, G. A., Bridges, T. S., Varghese, A., Seager, T. P., and Linkov, I. (2005). Application of multicriteria decision analysis in environmental decision making. Integr. Environ. Assess. Manag 1, 95–108. doi: 10.1897/IEAM_2004a-015.1
Knight, I., and Coleman, J. (1993). A fire perimeter expansion algorithm based on Huygen's wavelet propagation. Int. J. Wildland Fire 3, 73–84. doi: 10.1071/WF9930073
Korb, K. B., and Nicholson, A. E. (2011). Bayesian Artificial Intelligence. Computer Science and Data Analysis 2nd Edn. Boca Raton, FL: CRC/Chapman Hall.
Langhans, C., Smith, H. G., Chong, D. M. O., Nyman, P., Lane, P. N. J., and Sheridan, G. J. (2016). A model for assessing water quality risk in catchments prone to wildfire. J. Hydrol. 534, 407–426. doi: 10.1016/j.jhydrol.2015.12.048
Loane, I. T., and Gould, J. S. (1986). Aerial Suppression of Bushfires: Cost-Benefit Study for Victoria.' Canberra: CSIRO Publishing.
Loehle, C. (2004). Applying landscape principles to fire hazard reduction. For. Ecol. Manage 198, 261–267. doi: 10.1016/j.foreco.2004.04.010
Marcot, B. G., Holthausen, R. S., Raphael, M. G., Rowland, M. M., and Wisdom, M. J. (2001). Using Bayesian belief networks to evaluate fish and wildlife population viability under land management alternatives from an environmental impact statement. For. Ecol. Manag. 153, 29–42. doi: 10.1016/S0378-1127(01)00452-2
Marcot, B. G., Steventon, J. D., Sutherland, G. D., and McCann, R. K. (2006). Guidelines for developing and updating Bayesian belief networks applied to ecological modeling and conservation. Can. J. For. Res. 36, 3063–3074.
McArthur, A. G. (1967). Fire Behaviour in Eucalypt Forests. Leaflet No. 107. Canberra, ACT: Forest Research Institute, Forestry and Timber Bureau.
McCaffrey, S., and Rhodes, A. (2009). Public response to wildfire: is the australian “stay and defend or leave early” approach an option for wildfire management in the United States? J. Forestry 107, 9–15. doi: 10.1093/jof/107.1.9
McCaw, W. L., Neal, J. E., and Smith, R. H. (2002). Stand characteristics and fuel accumulation in a sequence of even-aged Karri (Eucalyptus diversicolor) stands in south-west Western Australia. For. Ecol. Manage 158, 263–271. doi: 10.1016/S0378-1127(00)00719-2
McColl-Gausden, S. C., Bennett, L. T., Duff, T. J., Cawson, J. G., and Penman, T. D. (2019). Climatic and edaphic gradients predict variation in wildland fuel hazard in south-eastern Australia. Ecography. 232, 243–253. doi: 10.1111/ecog.04714
McColl-Gausden, S. C., and Penman, T. D. (2019). Pathways of change: predicting the effects of fire on flammability. J. Environ. Manage 232, 243–253. doi: 10.1016/j.jenvman.2018.11.063
McFayden, C. B., Boychuk, D., Woolford, D. G., Wheatley, M. J., and Johnston, L. (2019). Impacts of wildland fire effects on resources and assets through expert elicitation to support fire response decisions. Intern. J. Wildland Fire 28, 885–900. doi: 10.1071/WF18189
McGee, T. K. (2011). Public engagement in neighbourhood level wildfire mitigation and preparedness: case studies from Canada, the US and Australia. J. Environ. Manage 92, 2524–2532. doi: 10.1016/j.jenvman.2011.05.017
McGee, T. K., and Russell, S. (2003). “It's just a natural way of life…” an investigation of wildfire preparedness in rural Australia. Global Environ. Change Part B 5, 1–12. doi: 10.1016/j.hazards.2003.04.001
Milne, M., Clayton, H., Dovers, S., and Cary, G. J. (2014). Evaluating benefits and costs of wildland fires: critical review and future applications. Environ. Hazards 13, 114–132. doi: 10.1080/17477891.2014.888987
Morrissey, S. A., and Reser, J. P. (2007). Natural disasters, climate change and mental health considerations for rural Australia. Austr. J. Rural Health 15, 120–125. doi: 10.1111/j.1440-1584.2007.00865.x
Murphy, B. P., Bradstock, R. A., Boer, M. M., Carter, J., Cary, G. J., Cochrane, M. A., et al. (2013). Fire regimes of Australia: a pyrogeographic model system. J. Biogeogr. 40, 1048–1058. doi: 10.1111/jbi.12065
Noble, I. R., Bary, G. A. V., and Gill, A. M. (1980). Mcarthurs fire danger meters expressed as equations. Austral. J. Ecol. 5, 201–204. doi: 10.1111/j.1442-9993.1980.tb01243.x
Nyman, P., Sheridan, G. J., Smith, H. G., and Lane, P. N. J. (2011). Evidence of debris flow occurrence after wildfire in upland catchments of south-east Australia. Geomorphology 125, 383–401. doi: 10.1016/j.geomorph.2010.10.016
Papadatou, D., Giannopoulou, I., Bitsakou, P., Bellali, T., Talias, M. A., and Tselepi, K. (2012). Adolescents' reactions after a wildfire disaster in Greece. J. Trauma. Stress 25, 57–63. doi: 10.1002/jts.21656
Paterson, G., and Chong, D. (2011). “Implementing the Phoenix fire spread model for operational use,” in Surveying and Spatial Sciences Conference (Wellington), 111
Paton, D., Kelly, G., Burgelt, P. T., and Doherty, M. (2006). Preparing for bushfires: understanding intentions. Disaster. Prev. Manag 15, 566–575. doi: 10.1108/09653560610685893
Penman, S. H., Price, O. F., Penman, T. D., and Bradstock, R. A. (2018). The role of defensible space on the likelihood of house impact from wildfires in forested landscapes of south eastern Australia. Intern. J. Wildland Fire 28, 4–14. doi: 10.1071/WF18046
Penman, T. D., Bradstock, R. A., and Price, O. F. (2014). Reducing wildfire risk to urban developments: simulation of cost-effective fuel treatment solutions in south eastern Australia. Environ. Modell. Software 52, 166–175. doi: 10.1016/j.envsoft.2013.09.030
Penman, T. D., Christie, F. J., Andersen, A. N., Bradstock, R. A., Cary, G. J., Henderson, M. K., et al. (2011). Prescribed burning: how can it work to conserve the things we value? Intern. J. Wildland Fire 20, 721–733. doi: 10.1071/WF09131
Penman, T. D., and Cirulis, B. A. (2020). Cost effectiveness of fire management strategies in southern Australia. Intern. J. Wildland Fire 29, 427–439. doi: 10.1071/WF18128
Penman, T. D., Collins, L., Duff, T. J., Price, O. F., and Cary, G. J. (2020). “Scientific evidence regarding effectiveness of prescribed burning,” in Perspectives of Prescribed Burning in Landscape Management, eds A. Leavesley, M. Wouters, and R. Thornton (Bushfire: Natural Hazards CRC, AFAC), 99–111.
Penman, T. D., Collins, L., Price, O. F., Bradstock, R. A., Metcalf, S., and Chong, D. M. O. (2013a). Examining the relative effects of fire weather, suppression and fuel treatment on fire behaviour – A simulation study. J. Environ. Manage 131, 325–333. doi: 10.1016/j.jenvman.2013.10.007
Penman, T. D., Eriksen, C., Blanchi, R., Chladil, M., Gill, A. M., Haynes, K., et al. (2013b). Defining adequate means of residents to prepare property for protection from wildfire. Intern. J. Disaster Risk Reduction 6, 67–77. doi: 10.1016/j.ijdrr.2013.09.001
Penman, T. D., Kavanagh, R. P., Binns, D. L., and Melick, D. R. (2007). Patchiness of prescribed burns in dry sclerophyll eucalypt forests in South-eastern Australia. For. Ecol. Manage 252, 24–32. doi: 10.1016/j.foreco.2007.06.004
Penman, T. D., and York, A. (2010). Climate and recent fire history affect fuel loads in Eucalyptus forests: implications for fire management in a changing climate. For. Ecol. Manage 260, 1791–1797. doi: 10.1016/j.foreco.2010.08.023
Perry, R. W., and Lindell, M. K. (1978). Psychological consequences of natural disaster - review of research on American communities. Mass Emergen. 3, 105–115.
Plucinski, M. P. (2012). Factors affecting containment area and time of Australian forest fires featuring aerial suppression. Forest Sci. 58, 390–398. doi: 10.5849/forsci.10-096
Pollino, C. A., Woodberry, O., Nicholson, A., Korb, K., and Hart, B. T. (2007). Parameterisation and evaluation of a Bayesian network for use in an ecological risk assessment. Environ. Modell. Softw. 22, 1140–1152. doi: 10.1016/j.envsoft.2006.03.006
Price, O. F., and Bradstock, R. A. (2012). The efficacy of fuel treatment in mitigating property loss during wildfires: insights from analysis of the severity of the catastrophic fires in 2009 in Victoria, Australia. J. Environ. Manage 113, 146–157. doi: 10.1016/j.jenvman.2012.08.041
Price, O. F., Penman, T. D., Bradstock, R. A., Boer, M. M., and Clarke, H. (2015). Biogeographical variation in the potential effectiveness of prescribed fire in south-eastern Australia. J. Biogeogr 42, 2234–2245. doi: 10.1111/jbi.12579
Prior, T., and Eriksen, C. (2013). Wildfire preparedness, community cohesion and social–ecological systems. Global Environ. Change 23, 1575–1586. doi: 10.1016/j.gloenvcha.2013.09.016
Radeloff, V. C., Hammer, R. B., Stewart, S. I., Fried, J. S., Holcomb, S. S., and McKeefry, J. F. (2005). The wildland-urban interface in the United States. Ecol. Applic. 15, 799–805. doi: 10.1890/04-1413
Ramsay, G. C., McArthur, N. A., and Dowling, V. P. (1987). Preliminary results from an examination of house survival in the 16 February 1983 Bushfires in Australia. Fire Mater. 11, 49–51. doi: 10.1002/fam.810110105
Roxburgh, S. H., Wood, S. W., Mackey, B. G., Woldendorp, G., and Gibbons, P. (2006). Assessing the carbon sequestration potential of managed forests: a case study from temperate Australia. J. Appl. Ecol. 43, 1149–1159. doi: 10.1111/j.1365-2664.2006.01221.x
Russell-Smith, J. (2016). Fire management business in Australia's tropical savannas: lighting the way for a new ecosystem services model for the north? Ecol. Managem. Restoration 17, 4–7. doi: 10.1111/emr.12201
Safford, H. D., Schmidt, D. A., and Carlson, C. H. (2009). Effects of fuel treatments on fire severity in an area of wildland-urban interface, Angora Fire, Lake Tahoe Basin, California. For. Ecol. Manage 258, 773–787. doi: 10.1016/j.foreco.2009.05.024
Scott, J. H., Thompson, M. P., and Gilbertson-Day, J. W. (2016). Examining alternative fuel management strategies and the relative contribution of National Forest System land to wildfire risk to adjacent homes – A pilot assessment on the Sierra National Forest, California, USA. For. Ecol. Manage 362, 29–37. doi: 10.1016/j.foreco.2015.11.038
Sierra, L. A., Yepes, V., García-Segura, T., and Pellicer, E. (2018). Bayesian network method for decision-making about the social sustainability of infrastructure projects. J. Clean. Prod. 176, 521–534. doi: 10.1016/j.jclepro.2017.12.140
Smith, H. G., Sheridan, G. J., Lane, P. N., Nyman, P., and Haydon, S. (2011). Wildfire effects on water quality in forest catchments: a review with implications for water supply. J. Hydrol. 396, 170–192. doi: 10.1016/j.jhydrol.2010.10.043
Snider, G., Daugherty, P. J., and Wood, D. (2006). The irrationality of continued fire suppression: an avoided cost analysis of fire hazard reduction treatments versus no treatment. J. Forestry 104, 431–437. doi: 10.1093/jof/104.8.431
Stephenson, C. (2010). A Literature Review on the Economic, Social and Environmental Impacts of Severe Bushfires in South-Eastern Australia. Victoria: Victorian Government Department of Sustainability and Environment.
Stockmann, K., Burchfield, J., Calkin, D., and Venn, T. (2010). Guiding preventative wildland fire mitigation policy and decisions with an economic modeling system. Forest Policy Econ. 12, 147–154. doi: 10.1016/j.forpol.2009.09.009
Syphard, A. D., and Keeley, J. E. (2019). Factors Associated with Structure Loss in the 2013–2018 California Wildfires. Fire 2:49. doi: 10.3390/fire2030049
Thomas, P. B., Watson, P. J., Bradstock, R. A., Penman, T. D., and Price, O. F. (2014). Modelling surface fine fuel dynamics across climate gradients in eucalypt forests of south-eastern Australia. Ecography 37, 827–837. doi: 10.1111/ecog.00445
Thompson, M., and Anderson, N. (2015). Modeling fuel treatment impacts on fire suppression cost savings: a review. Califor. Agricul. 69, 164–170. doi: 10.3733/ca.v069n03p164
Thompson, M. P., Riley, K. L., Loeffler, D., and Haas, J. R. (2017). Modeling fuel treatment leverage: encounter rates, risk reduction, and suppression cost impacts. Forests 8:469. doi: 10.3390/f8120469
Thompson, M. P., Scott, J., Helmbrecht, D., and Calkin, D. E. (2013). Integrated wildfire risk assessment: framework development and application on the Lewis and Clark National Forest in Montana, USA. Integr. Environ. Assess. Manag. 9, 329–342. doi: 10.1002/ieam.1365
Tolhurst, K., Shields, B., and Chong, D. (2008). Phoenix: development and application of a bushfire risk management tool. Austral. J. Emerg. Managem. 23, 47–54.
Tolhurst, K. G., and Chong, D. M. O. (2011). “Assessing potential house losses using PHOENIX rapidfire,” in Bushfire CRC & AFAC 2011 Conference Science Day, ed R. P. Thornton (Sydney: Bushfire CRC), 74–86.
Tolhurst, K. G., and McCarthy, G. (2016). Effect of prescribed burning on wildfire severity: a landscape-scale case study from the 2003 fires in Victoria. Austral. Forestry 79, 1–14. doi: 10.1080/00049158.2015.1127197
Venn, T. J., and Calkin, D. E. (2011). Accommodating non-market values in evaluation of wildfire management in the United States: challenges and opportunities. Intern. J. Wildland Fire 20, 327–339. doi: 10.1071/WF09095
Victorian DELWP (2015). “Strategic bushfire risk management plan - barwon otway,” in Department of Environment, Water and Planning (Melbourne), 42.
Wain, A., Mills, G., McCaw, L., and Brown, T. (2008). “Chapter 23 managing smoke from wildfires and prescribed burning in Southern Australia,” in Developments in Environmental Science, Vol. 8, eds A. Bytnerowicz, M. J. Arbaugh, A. R. Riebau, and C. Andersen (New York, NY: Elsevier), 535–550.
Weisshaupt, B. R., Carroll, M. S., Blatner, K. A., Robinson, W. D., and Jakes, P. J. (2005). Acceptability of smoke from prescribed forest burning in the northern inland west: a focus group approach. J. Forestry 103, 189–193. doi: 10.1093/jof/103.4.189
Whittaker, J., Handmer, J., and Mercer, D. (2012). Vulnerability to bushfires in rural Australia: a case study from East Gippsland, Victoria. J. Rural Stud 28, 161–173. doi: 10.1016/j.jrurstud.2011.11.002
Wilson, A. A. G., and Ferguson, I. S. (1986). Predicting the probability of house survival during bushfires. J. Environ. Manage 23, 259–270.
Keywords: Bayes network (BN), fire simulation, wildfire (bushfire), risk, trade off analysis, cost-benefit
Citation: Penman TD, Clarke H, Cirulis B, Boer MM, Price OF and Bradstock RA (2020) Cost-Effective Prescribed Burning Solutions Vary Between Landscapes in Eastern Australia. Front. For. Glob. Change 3:79. doi: 10.3389/ffgc.2020.00079
Received: 23 December 2019; Accepted: 04 June 2020;
Published: 10 July 2020.
Edited by:
Michele Salis, National Research Council (CNR), ItalyReviewed by:
Paulo M. Fernandes, University of Trás-os-Montes and Alto Douro, PortugalKarin Lynn Riley, United States Forest Service (USDA), United States
Copyright © 2020 Penman, Clarke, Cirulis, Boer, Price and Bradstock. This is an open-access article distributed under the terms of the Creative Commons Attribution License (CC BY). The use, distribution or reproduction in other forums is permitted, provided the original author(s) and the copyright owner(s) are credited and that the original publication in this journal is cited, in accordance with accepted academic practice. No use, distribution or reproduction is permitted which does not comply with these terms.
*Correspondence: Trent D. Penman, dHJlbnQucGVubWFuQHVuaW1lbGIuZWR1LmF1