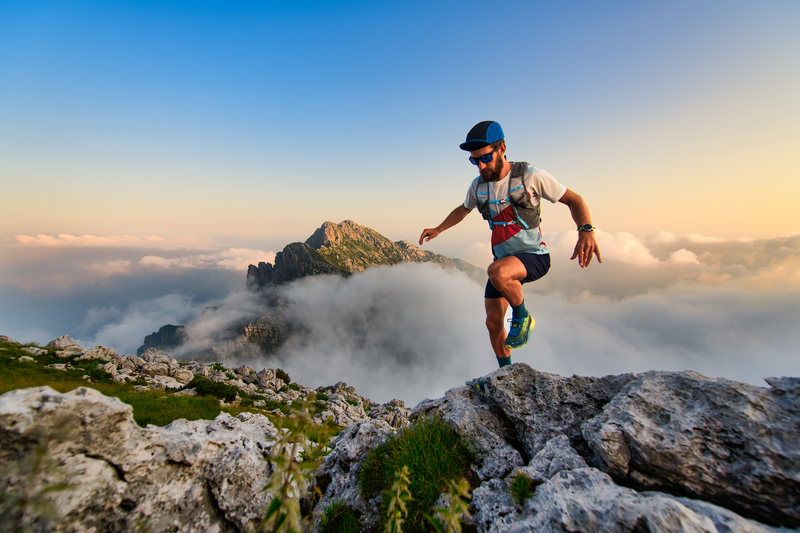
95% of researchers rate our articles as excellent or good
Learn more about the work of our research integrity team to safeguard the quality of each article we publish.
Find out more
ORIGINAL RESEARCH article
Front. Food. Sci. Technol. , 12 December 2024
Sec. Food Modeling
Volume 4 - 2024 | https://doi.org/10.3389/frfst.2024.1491396
This article is part of the Research Topic Advancing Food Processing: Novel Technologies and Modeling Techniques View all 4 articles
Granular materials are widely encountered in food processing, but understanding their behavior and movement mechanisms remains in the early stages of research. In this paper, we present our recent modeling and simulation work on chute granular flow using both the discrete element method (DEM) and continuum method. Based on the simulation data, we apply machine learning techniques such as Random Forest, Linear Regression, and Ridge Regression to evaluate the effectiveness of these models in predicting granular flow patterns. The granular materials in our study consist of soft-sphere particles with a 1 mm diameter, driven by gravity as they flow down a chute inclined relative to the horizontal plane. Our DEM and continuum simulation results show good agreement in modeling the chute flow, and the machine learning approach demonstrates promising potential for predicting flow patterns. The results of this chute flow study can provide a benchmark solution for more complex flow problems involving factors such as particle shape, size, interparticle interactions, and external obstacles.
Granular materials are among the most commonly encountered media in natural and industrial processes, perhaps second only to water. Examples of natural granular material processes include landslides, avalanches, sediment transport, volcanic pyroclastic flows and lava, soil creep and erosion, debris flows, sand dune and glacial movements. Understanding these processes is critical for predicting natural events and mitigating their impacts from hazards. In industry, granular material processes are widely applied across various sectors: from ore crushing, grinding, and sorting in mining, powder mixing, tablet formation, and coating in pharmaceutical, black mass (e.g., lithium, nickel, cobalt) extracting and classifying from end-of-life (EoL) electric vehicle (EV) batteries in recycling, to mixing, packaging, and quality control of granular food products. Optimizing the handling, processing, control, and transport of particulate materials is essential for improving efficiency, productivity, quality, safety, and substantiality in industrial processes. The growing interest in granular material processes underscores the importance of granular flow studies for gaining a fundamental understanding of these complex systems and addressing real-world problems.
Granular flow studies focus on the behavior and movement of granular materials, ranging in size from fine powders to large rocks while exhibiting complex behaviors distinct from both solid and fluid states. Despite its significance, understanding granular flow remains one of the most challenging scientific knowledge gaps today (Forterre and Pouliquen, 2008; Guo and Curtis, 2015; Gray, 2018; Kamrin et al., 2024; Zhao et al., 2023). Accurately modeling and simulating these systems is still difficult due to the complex nature arising from interactions between particle-particle, particle-boundary, and particle-environment, as well as intrinsic particle properties associated with size, shape, friction, and cohesion. The complexity is further compounded by the transitions between different flow regimes. For example, a regime of moving particles can exhibit a mixed type of gas-liquid-solid behavior during flow, with the formation of granular shocks or particle-free regions (Cui, 2021; Cui et al., 2022; Tregaskis et al., 2022).
These complexities are particularly evident in the food industry, where granular food ingredients (e.g., rice, barley, or malt) are exposed to unique conditions due to irregular shapes, varying sizes, moisture content, and particle degradation or breakage during processing. Addressing these challenges requires accurate models, rigorous simulations, and sophisticated experimental and validation techniques to achieve reliable, robust, and predictive insights into these systems (Muntán et al., 2009; Vakis et al., 2018; Fries, 2021; Dhiman and Prabhakar, 2021).
In recent years, machine learning (ML) and artificial intelligence (AI) are increasingly being explored to analyze vast amounts of data generated from simulations and experiments in fluid mechanics (Brunton et al., 2020; Molinaro et al., 2021). By incorporating machine learning algorithms into data analytics, patterns and relationships that traditional methods might miss can be identified (Breiman, 2001; Choi and Kumar, 2024), and the models can be trained to predict complex behaviors of granular materials (Raissi et al., 2020; Mao et al., 2020). On the other hand, machine learning can optimize simulation parameters, reducing computational costs and time, for example, by establishing a surrogate model between the parameter space and output domain (Sen et al., 2018; Ma et al., 2022; Choi and Kumar, 2024).
In this paper, we will utilize both the Discrete Element Method (DEM) and continuum modeling to simulate granular flow driven by gravity down an inclined plane, usually referred as chute flow. DEM offers microstructural details into particle dynamics by capturing inter-particle and particle-wall interactions associated with collisions, friction, and cohesion, which are crucial when the behavior of individual particles significantly impacts the overall system. On the other hand, continuum simulations provide a macroscopic insight on bulk flow behavior by treating granular materials as a continuous medium, where parameters such as flow thickness, density, velocity, rheological laws and stresses can be incorporated into the governing equations of fluid mechanics. By examining both the micro-scale interactions and macro-scale dynamics of granular materials during their flow, we aim to achieve a more comprehensive and unified understanding of their behaviors.
Since the formulation of the discrete element method (DEM) by Cundall and Strack (1979), DEM-based simulations have gained significant popularity in recent years. This is largely due to their ability to provide a direct understanding of the granular material interactions and behaviors at microstructural level (e.g., (Guo and Curtis, 2015; Kieckhefen et al., 2020; Kamrin et al., 2024)).
Moreover, coupling the computational fluid dynamics (CFD) calculations of the fluid phase with the DEM computations of the granular phase has provided an enhanced platform to understand mixed solid-liquid-gas behavior under more realistic conditions such as granular materials immersed in an ambient fluid e.g., (Boyer et al., 2011; Amarsid et al., 2017; Cui et al., 2020). For the collapse of a granular column, for example, its run-out may behave very differently, depending on whether the use of coarse or fine grains are immersed partially in water (Si et al., 2018; He et al., 2022). On the other hand, the coupled CFD-DEM simulation has the adoption of much larger time steps in simulation by incorporating the concept of a “parcel” to represent a cloud of particles with similar properties (Bérard et al., 2020). In this approach, the discrete element method models the granular particle system, while the volume-averaged Navier-Stokes equations solve the fluid flow through a multiphase-Lagrangian framework (Crowe et al., 2012; Vijayan et al., 2021; Li et al., 2020; Cui et al., 2022).
Our DEM simulation adopts a soft-sphere approach, allowing particles to deform slightly upon contact. The translational and angular motions of each individual particle can be modelled in Equations 1, 2, respectively, as follows,
where
Figure 1. Schematic of the chute flow set-up. The Cartesian coordinate
The CFD model for fluid flow adopts the volume-averaged Navier-Stokes equations, where the volume occupied by the fluid within each cell depends on the volume taken by the solid particles. Let
and the momentum Equation 4 is
where
The continuum simulation of chute flow is based on solving a depth-averaged granular flow model of the shallow water type (Stoker, 1957; Eglit and Shahinpoor, 1983; Savage and Hutter, 1989). By averaging the properties of the granular flow across the depth of the flow, this model reduces the computational complexity associated with simulating three-dimensional simulations. With the normal velocity component
where
where
Using the conservative variables
where
To align with the dimensional DEM simulations, the following scaling is used: the length scale is set to
Without considering the complexity of the flow released from the hopper and gate, we focus our simulation on a region of the flow that is sufficiently developed along the slope of the chute. As shown in Figure 1, a typical chute flow set-up consists of a hopper, a release gate, and a chute with a bed and sidewalls. The chute has a downslope length
Following the selection of the simulation domain, the DEM simulation is conducted in a three-dimensional region where
The governing Equation 8 is solved numerically using a finite-difference method (Cui, 2014; Cui, 2021) on a uniform H-grid covering the domain defined by
Since DEM and continuum simulations are based on fundamentally different modeling frameworks in both spatial and temporal domains, it is particularly effective to first investigate the time-dependent development of the simulations. This approach provides an overall perspective, as well as a qualitative comparison, between the two methods. To achieve this, we simulate spherical particles with a diameter of 1 mm flowing down a 150 mm slope inclined at
As shown in Figure 2, the DEM solutions on the left-hand side generally agree well with the continuum solutions on the right-hand side, particularly at later stages of development, say, from
Figure 2. DEM and continuum simulations of time-dependent development in granular flow down a 150 mm by 20 mm slope.
Figure 3 provides a more quantitative comparison for the spatial-temporal averaged downslope velocity <u>. This velocity is calculated during the simulation in a form of
Figure 3. Time-dependent development of downslope velocity over a 150 mm slope: the solid line shows the depth-averaged continuum model result; the dash-dot line indicates the normalized DEM simulation velocity. The dash-dot-dot, dotted, and dashed lines represent the normalized velocities at the free surface, mid-depth, and basal surface, respectively.
A key advantage of continuum simulations over DEM simulations is their significantly lower computational cost. In our case, under similar computing conditions, the continuum simulation required only 42 s on a
Further details of velocities obtained from the DEM steady-state solution are shown in Figure 4. In this figure, the velocities have been averaged in the cross-slope
Figure 4. Velocity profiles between the DEM and continuum simulations: (A) velocity field in the
Accurately capturing the velocity profile is crucial for modelling the rheological behaviour of granular flows, particularly in continuum simulations (Pouliquen, 1999; GDR, 2004; Franci and Cremonesi, 2019; Tregaskis et al., 2022), while also serving as a metric for assessing the robustness and effectiveness of DEM simulations. As particle characteristics become more complex–such as in shape, size, moisture content, attrition, breakage, and mixing–modelling inter-particle contact and interaction becomes increasingly challenging (Guo and Curtis, 2015; Zhong et al., 2016; Vakis et al., 2018). However, detailed insights into particle velocity profiles are vital for uncovering critical physical insights into particle dynamics and behaviors. Since spherical particles represent the most typical shape, our results could offer a benchmark solution for further studies, including the application of machine learning.
With the rapid advancement of computing technologies, artificial intelligence and machine learning are increasingly being applied to analyze fluid mechanics problems (Brunton et al., 2020; Molinaro et al., 2021). This section explores the use of machine learning techniques to analyze and interpret the granular flow dataset generated from the DEM simulations discussed in Section 3.1.
Using the Random Forest method as an example, we briefly explain how machine learning can be applied to granular flow data analysis (Breiman, 2001; Zaki and Meira, 2014; Clarke et al., 2009) from training, optimization, to prediction.
Let
Let
where
Consequently, a loss function, e.g., the Mean Squared Error (MSE), as given in Equation 11 as follows
can be used to measure the discrepancy between the true labels
Without repeating further details of the training process (Breiman, 2001), the model is evaluated using a test dataset
Figure 5. The
The trained RandomForest model is then applied to predict outputs for new, unseen data points
When training a machine learning model, it is essential to split the dataset
In Figure 5, three machine learning models–RandomForest, LinearRegression, and RidgeRegression–are used to train the granular flow dataset with different numbers of feature vectors at
Figure 6 shows the predicted output,
Figure 6. Random Forest, Ridge Regression and Linear Regression machine learning applied to the granular flow dataset, with a comparison to the “true” flow pattern in the test data. (A, B) Test data (C) Random forest (D) Random forest (E) Ridge regression (F) Ridge regression (G) Linear regression (H) Linear regression
Even with the RandomForest model, the predicted result shows a significant discrepancy from the true solution, highlighting the challenges of applying machine learning to accurately predict even relatively simple granular flow patterns. One possible reason for this disagreement is that the original training dataset was selected from the initial injection phase of the flow through to its steady state. This may have complicated (deliberately) the training process due to the continuously evolving flow patterns, as seen in Figure 2. Nevertheless, there is ongoing development in machine learning techniques to address such complexities, with methods like physics-informed neural networks (PINNs) (Karniadakis et al., 2021; Wang et al., 2021; Mao et al., 2020) and graph neural networks (GNNs) (Choi and Kumar, 2024) being tested for tackling complex fluid mechanics problems.
In this study, we investigated the dynamics of granular flow using both Discrete Element Method (DEM) and continuum modeling, providing an insight to the micro-scale interactions and macro-scale behavior of granular materials. The comparative analysis revealed that although both methods produced generally consistent results, discrepancies, particularly in early-stage flow development, underscore the complexity of accurately simulating granular materials. Furthermore, we explored the application of machine learning techniques, including Random Forest, Ridge Regression, and Linear Regression models, to predict particle positions within the granular flow. While the Random Forest model outperformed the linear models, the overall prediction accuracy highlighted the inherent challenges in using machine learning for complex granular systems.
While the use of spherical particle shapes provides robustness in DEM simulations, actual particle conditions are often more complex, especially in food processing applications.These factors may impose significant complexity to understanding granular particle behaviors. As illustrate in Figure 7, particle size and shape, even for spheres and capsules, can substantially influence particle packing condition, measured by the volume fraction. Within the moving flow mass, particles tend to segregate noticeably based on volume fraction. On the other hand, food particles frequently exhibit varying properties across seasons and regions, making it further challenging to accurately model and simulate their behavior due to the limited knowledge of their complex compositions (Vakis et al., 2018; Fries, 2021). Further exploration in the field of contact mechanics, fracture mechanics, and tribology is therefore essential for more realistically modeling particle interactions at the microscopic scale. Similarly, improvements in granular process rheology are needed to more accurately capture macroscopic flow behaviors (Dunatunga and Kamrin, 2015; Krishnaraj and Nott, 2015). These remain some of the most demanding tasks in the modeling and simulation of granular food processes.
Figure 7. Size and shape on the volume fraction (with error bars) of granular particles, and
Using the rice milling process as an example, it involves several mechanical processes that exert various forces on the grain, significantly influencing the extent of breakage (Einav, 2007). Particle breakage under impact conditions is often modeled by considering energy dissipation, material resistance, and energy efficiency (Vogel and Peukert, 2003; Shitanda et al., 2001), and DEM-PBM (particle balance method) simulations have been tested for this purpose (Han et al., 2016; Metta et al., 2018; Cabiscol et al., 2021; Nakamura et al., 2022). Additionally, the Material Point Method (MPM) has been introduced in continuum modeling to allow granular materials to transition through various phases during the flow process (Dunatunga and Kamrin, 2015; Haeri and Skonieczny, 2022).
Given the challenges faced by traditional machine learning models in predicting granular flow patterns, future efforts should explore advanced techniques, such as physics-informed neural networks (PINNs) and graph neural networks (GNNs) (Cai et al., 2020; Choi and Kumar, 2024). These methods, which integrate physical laws and complex network structures, could offer more accurate predictions by better capturing the inherent dynamics of granular materials.
Finally, continuous validation of simulation results against experimental data is crucial for ensuring the reliability of both DEM and continuum models. Establishing benchmark cases, particularly for spherical particles, as presented in this study, can provide a valuable reference for future research, enabling the comparison and improvement of various modeling approaches.
The original contributions presented in the study are included in the article/supplementary material, further inquiries can be directed to the corresponding author.
XC: Conceptualization, Methodology, Supervision, Writing–original draft, Writing–review and editing. DA: Data curation, Investigation, Software, Writing–original draft, Writing–review and editing. HZ: Conceptualization, Project administration, Resources, Writing–review and editing. MH: Conceptualization, Project administration, Resources, Writing–review and editing. AA: Conceptualization, Project administration, Resources, Writing–review and editing. TO: Investigation, Writing–review and editing. KS: Investigation, Writing–review and editing. SF: Investigation, Writing–review and editing.
The author(s) declare that financial support was received for the research, authorship, and/or publication of this article. The authors would like to acknowledge the support of the Innovate United Kingdom grant BB/S020993, EPSRC Grant EP/V521140/1, the Royal Society grant SIF/R2/212009, and Innovate United Kingdom Grant 10075111 for conducting this research.
Xinjun Cui is a Royal Society Short Industry Fellow. For the purpose of open access, the authors have applied a Creative Commons Attribution (CC BY) licence to any Author Accepted Manuscript version arising from this submission.
Author AA was employed by Koolmill Systems Ltd.
The remaining authors declare that the research was conducted in the absence of any commercial or financial relationships that could be construed as a potential conflict of interest.
All claims expressed in this article are solely those of the authors and do not necessarily represent those of their affiliated organizations, or those of the publisher, the editors and the reviewers. Any product that may be evaluated in this article, or claim that may be made by its manufacturer, is not guaranteed or endorsed by the publisher.
Amarsid, L., Delenne, J. Y., Mutabaruka, P., Monerie, Y., Perales, F., and Radjai, F. (2017). Viscoinertial regime of immersed granular flows. Phys. Rev. E 96, 012901. doi:10.1103/PhysRevE.96.012901
Bérard, A., Patience, G. S., and Blais, B. (2020). Experimental methods in chemical engineering: unresolved CFD-DEM. Can. J. Chem. Eng. 98, 424–440. doi:10.1002/cjce.23686
Boyer, F., Guazzelli, É., and Pouliquen, O. (2011). Unifying suspension and granular rheology. Phys. Rev. Lett. 107, 188301. doi:10.1103/PhysRevLett.107.188301
Brunton, S. L., Noack, B. R., and Koumoustakos, P. (2020). Machine learning for fluid mechanics. Annu. Rev. Fluid Mech. 52, 477–508. doi:10.1146/annurev-fluid-010719-060214
Cabiscol, R., Finke, J. H., and Kwade, A. (2021). A bi-directional DEM-PBM coupling to evaluate chipping and abrasion of pharmaceutical tablets. Adv. Powder Technol. 32, 2839–2855. doi:10.1016/j.apt.2021.06.002
Cai, S., Mao, Z., Wang, Z., Yin, M., and Karniadakis, G. E. (2020). Physics-informed neural network (PINNs) for fluid mechanics: a review. Acta Mech. Sinca 37, 7127–1738. doi:10.48550/arXiv.2105.09506
Choi, Y., and Kumar, K. (2024). Graph neural network-based surrogate model for granular flows. Comput. Geotech. 166, 106015. doi:10.1016/j.compgeo.2023.106015
Clarke, B., Fokoué, E., and Zhang, H. (2009). Principles and theory for data mining and machine learning. Springer.
Crowe, C. T., Sommerfeld, M., and Tsuji, Y. (2012). Multiphase flows with droplets and particles, 2nd Ed. Boca Raton, (CRC Press)
Cui, K. F. E., Zhou, G. D., Jing, L., Chen, X., and Song, D. (2020). Generalized friction and dilatancy laws for immersed granular flows consisting of large and small particles. Phys. Fluids 32, 113312. doi:10.1063/5.0024762
Cui, X. (2014). Computational and experimental studies of rapid free-surface granular flows around obstacles. Comput. & Fluids 89, 179–190. doi:10.1016/j.compfluid.2013.10.036
Cui, X. (2021). Strong oblique shock waves in granular free-surface flows. Phys. Fluids 33, 0833024. doi:10.1063/5.0057700
Cui, X., and Gray, J. M. N. T. (2013). Gravity-driven granular free-surface flow around a circular cylinder. J. Fluid Mech. 720, 314–337. doi:10.1017/jfm.2013.42
Cui, X., Harris, M., Howarth, M., Zealey, D., Brown, R., and Shepherd, J. (2022). Granular flow around a cylindrical obstacle in an inclined chute. Phys. Fluids 34, 093308. doi:10.1063/5.0101694
Cundall, P. A., and Strack, O. D. L. (1979). A discrete numerical model for granular assemblies. Geotechnique 29 (1), 47–65. doi:10.1680/geot.1979.29.1.47
Dhiman, A., and Prabhakar, P. K. (2021). Micronization in food processing: a comprehensive review of mechanistic approach, physicochemical, functional properties and self-stability of micronized food materials. J. Food Eng. 292, 110248. doi:10.1016/j.jfoodeng.2020.110248
Dunatunga, S., and Kamrin, K. (2015). Continuum modelling and simulation of granular flows through their many phases. J. Fluid Mech. 779, 483–513. doi:10.1017/jfm.2015.383
Eglit, M. E. (1983). “Some mathematical models of snow avalanches,”. Advances in mechanics and the flow of granular materials. Editor M. Shahinpoor (Clausthal-Zellerfeld: Gulf Publishing Company), 2, 577–588.
Einav, I. (2007). Breakage mechanics-Part II: modelling granular materials. J. Mech. Phys. 55 (6), 1298–1320. doi:10.1016/j.jmps.2006.11.004
Forterre, Y., and Pouliquen, O. (2008). Flows of dense granular media. Annu. Rev. Fluid Mech. 40, 1–24. doi:10.1146/annurev.fluid.40.111406.102142
Franci, A., and Cremonesi, M. (2019). 3D regularized (I) rheology for granular flows simulation. J. Comput. Phys. 378, 257–277. doi:10.1016/j.jcp.2018.11.011
Fries, L. (2021). Modeling food particle systems: a review of current progress and challenges. Annu. Rev. Chem. Biomol. Eng. 12, 97–113. doi:10.1146/annurev-chembioeng-121820-081524
Gdr, M. (2004). On dense granular flows. Eur. Phys. J. E 14, 341–365. doi:10.1140/epje/i2003-10153-0
Gray, J. M. N. T. (2018). Particle segregation in dense granular flows. Annu. Rev. Fluid Mech. 50, 407–433. doi:10.1146/annurev-fluid-122316-045201
Gray, J. M. N. T., Wieland, M., and Hutter, K. (1999). Gravity-driven free surface flow of granular avalanches over complex basal topography. Proc. Roy. Soc. A 455, 1841–1874. doi:10.1098/rspa.1999.0383
Guo, Y., and Curtis, J. S. (2015). Discrete element method simulations for complex granular flows. Annu. Rev. Fluid Mech. 47, 21–46. doi:10.1146/annurev-fluid-010814-014644
Haeri, A., and Skonieczny, K. (2022). Three-dimensionsal granular flow continuum modeling via material point method with hyperelastic nonlocal granular fluidity. Comput. Methods Appl. Mech. Engrg. 394, 114904. doi:10.1016/j.cma.2022.114904
Han, Y., Jia, F., Zeng, Y., Jiang, L., Zhang, Y., and Cao, B. (2016). Effects of rotation speed and outlet opening on particle flow in a vertical rice mill. Powder Technol. 297, 153–164. doi:10.1016/j.powtec.2016.04.022
He, K., Shi, H., and Yu, X. (2022). Effects of interstitial water on collapses of partially immersed granular columns. Phys. Fluids 34, 023306. doi:10.1063/5.0079468
Kamrin, K., Hill, K. M., Goldman, D. I., and Andrade, J. E. (2024). Advances in modeling dense granular media. Annu. Rev. Fluid Mech. 56, 215–240. doi:10.1146/annurev-fluid-121021-022045
Karniadakis, G. E., Kevrekidis, I. G., Lu, L., Perdikaris, P., Wang, S., and Yang, L. (2021). Physics-informed machine learning. Nat. Rev. Phys. 3, 422–440. doi:10.1038/s42254-021-00314-5
Kieckhefen, P., Pietsch, S., Dosta, M., and Heinrich, S. (2020). Possibilities and limits of computational fluid dynamics - discrete element method simulations in process engineering: a review of recent advancements and future trends. Annu. Rev. Chem. Biomol. Eng. 11, 397–422. doi:10.1146/annurev-chembioeng-110519-075414
Krishnaraj, K. P., and Nott, P. R. (2015). A dilation-driven vortex flow in sheared granular materials explains a rheometric anomaly. Nat. comms 7, 10630. doi:10.1038/ncomms10630
Li, T., Zhang, H., Kuang, S., Yan, H., Diao, X., Huang, Z., et al. (2020). Experimental and numerical study of coarse particle conveying in the small absorber sphere system: overview and some recent CFD-DEM simulations. Nucl. Eng. Des. 357, 110420. doi:10.1016/j.nucengdes.2019.110420
Ma, G., Guan, S., Wang, Q., Feng, Y. T., and Zhou, W. (2022). A predictive deep learning framework for path-dependent mechanical behavior of granular materials. Acta Geotech. 17, 3463–3478. doi:10.1007/s11440-021-01419-y
Mao, Z., Jagtap, A. D., and Karniadakis, G. E. (2020). Physics-informed neural networks for high-speed flows. Comput. Methods Appl. Mech. Engrg. 360, 112789. doi:10.1016/j.cma.2019.112789
Metta, N., Ierapetritou, M., and Ramachandran, R. (2018). A multiscale DEM-PBM approach for a continuous comilling process using a mechanistically developed breakage kernel. Chem. Eng. Sci. 178, 211–221. doi:10.1016/j.ces.2017.12.016
Molinaro, R., Singh, J., Catsoulis, S., Narayanan, C., and Lakehal, D. (2021). Embedding data analytics and CFD into the digital twin concept. Comput. Fluids 214, 104759. doi:10.1016/j.compfluid.2020.104759
Muntán, E., García, C., Oller, P., Martí, G., García, A., and Gutiérrez, E. (2009). Reconstructing snow avalanche in the southeastern pyrenees. Nat. Hazard Earth Syst. Sci. 9, 159901612. doi:10.5194/nhess-9-1599-2009
Nakamura, H., Baba, T., Ohsaki, S., Watano, S., Takehara, K., and Higuchi, T. (2022). Numerical simulation of wet granulation using the DEM-PBM coupling method with a deterministically calculated agglomeration kernel. Chem. Eng. J. 450, 138298. doi:10.1016/j.cej.2022.138298
Nessyahu, H., and Tadmor, E. (1990). Non-oscillatory central differencing for hyperbolic conservation laws. J. Comput. Phys. 87, 408–463. doi:10.1016/0021-9991(90)90260-8
Pouliquen, O. (1999). Scaling laws in granular flows down rough inclined planes. Phys. Fluids 11 (3), 542–548. doi:10.1063/1.869928
Raissi, M., Yazdani, A., and Karniadakis, G. E. (2020). Hidden fluid mechanics: learning velocity and pressure fields from flow visualizations. Science 367, 1026–1030. doi:10.1126/science.aaw4741
Renzo, A. D., and Maio, F. P. D. (2004). Comparison of contact-force models for the simulation of collisions in DEM-based granular flow codes. Chem. Eng. Sci. 59, 525–541. doi:10.1016/j.ces.2003.09.037
Savage, S. B., and Hutter, K. (1989). The motion of a finite mass of granular material down a rough incline. J. Fluid Mech. 199, 177–215. doi:10.1017/s0022112089000340
Sen, O., Gaul, N. J., Choi, K. K., Jacobs, G., and Udaykumar, H. S. (2018). Evaluation of multifidelity surrogate modeling techniques to construct closure laws for drag in shock–particle interactions. J. Comput. Phys. 371, 434–451. doi:10.1016/j.jcp.2018.05.039
Shitanda, D., Nishiyama, Y., and Koide, S. (2001). Performance analysis of impeller and rubber roll husker using different variaties of rice. Agri. Eng. Int. CIGR J. 19.
Si, P., Shi, H., and Yu, X. (2018). Development of a mathematical model for submarine granular flows. Phys. Fluids 30, 083302. doi:10.1063/1.5030349
Tregaskis, C., Johnshon, C. G., Cui, X., and Gray, J. M. N. T. (2022). Subscritical and supercritical granular flow around an obstacle on a rough inclined plane. J. Fluid Mech. 933, A25-1–A25-41. doi:10.1017/jfm.2021.1074
Vakis, A. I., Yastrebov, V. S., Scheibert, J., Nicola, L., Dini, D., Ciavarella, M., et al. (2018). Modelling and simulation in tribology across scales: an overview. Tribol. Int. 125, 169–199. doi:10.1016/j.triboint.2018.02.005
Vijayan, A., Baju, J., and Kumar, A. R. (2021). Fluid flow assisted mixing of binary granular beds using CFD-DEM. Powder Technol. 383, 183–197. doi:10.1016/j.powtec.2021.01.040
Vogel, L., and Peukert, W. (2003). Breakage behaviour of different materials-construction of a mastercurve for the breakage probability. Powder Technol. 129, 101–110. doi:10.1016/s0032-5910(02)00217-6
Wang, K., Che, Y., Mehana, M., Lubbers, N., Bennet, K. C., Kang, Q., et al. (2021). A physics-informed and hierarchically regularized data-drive model for predicting fluid flow through porous media. J. Comput. Phys. 445, 110526. doi:10.1016/j.jcp.2021.110526
Zaki, M. J., and Meira, W. (2014). Data mining and analysis: fundamental concepts and algorithms. Cambridge University Press.
Zhao, J., Zhao, S., and Luding, S. (2023). The role of particle shape in computational modelling of granular matter. Nat. Rev. Phys. 5, 505–525. doi:10.1038/s42254-023-00617-9
Keywords: granular flows, DEM, continuum, machine learning, random forest, food processing PACS
Citation: Cui X, Adebayo D, Zhang H, Howarth M, Anderson A, Olopade T, Salami K and Farooq S (2024) Simulation of granular flows and machine learning in food processing. Front. Food. Sci. Technol. 4:1491396. doi: 10.3389/frfst.2024.1491396
Received: 04 September 2024; Accepted: 14 November 2024;
Published: 12 December 2024.
Edited by:
Nikolai I. Lebovka, National Academy of Sciences of Ukraine, UkraineReviewed by:
Roberto Arevalo, Research Centre For Energy Resources And Consumption, SpainCopyright © 2024 Cui, Adebayo, Zhang, Howarth, Anderson, Olopade, Salami and Farooq. This is an open-access article distributed under the terms of the Creative Commons Attribution License (CC BY). The use, distribution or reproduction in other forums is permitted, provided the original author(s) and the copyright owner(s) are credited and that the original publication in this journal is cited, in accordance with accepted academic practice. No use, distribution or reproduction is permitted which does not comply with these terms.
*Correspondence: X. Cui, eC5jdWlAdGVlcy5hYy51aw==
†ORCID: X. Cui, orcid.org/0000-0003-0581-3468
Disclaimer: All claims expressed in this article are solely those of the authors and do not necessarily represent those of their affiliated organizations, or those of the publisher, the editors and the reviewers. Any product that may be evaluated in this article or claim that may be made by its manufacturer is not guaranteed or endorsed by the publisher.
Research integrity at Frontiers
Learn more about the work of our research integrity team to safeguard the quality of each article we publish.