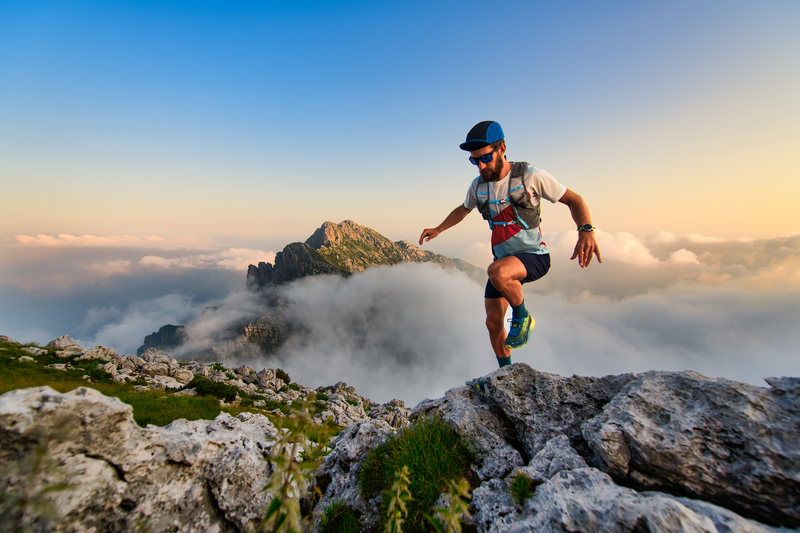
94% of researchers rate our articles as excellent or good
Learn more about the work of our research integrity team to safeguard the quality of each article we publish.
Find out more
ORIGINAL RESEARCH article
Front. Environ. Sci.
Sec. Big Data, AI, and the Environment
Volume 13 - 2025 | doi: 10.3389/fenvs.2025.1561794
This article is part of the Research Topic Formation Mechanisms of Ozone Pollution View all articles
The final, formatted version of the article will be published soon.
You have multiple emails registered with Frontiers:
Please enter your email address:
If you already have an account, please login
You don't have a Frontiers account ? You can register here
High ground-level ozone (O₃) concentrations significantly degrade urban air quality and pose threats to human health, highlighting the critical need for accurate and effective prediction of ozone levels to support environmental monitoring and policy formulation. Considering the lagged chemical interactions between ozone (O₃) and nitrogen oxides, this study integrates the historical concentrations of ozone and nitrogen dioxide (NO₂) over the past three hours as lagged feature variables. A Lagged Feature Prediction Model (LFPM) is proposed and evaluated using nine machine learning algorithms, including XGBoost. First, by combining XGBoost with SHAP (Shapley Additive Explanations) for feature selection analysis, 11 critical feature variables are identified. This approach improves computational efficiency by 30% compared to the original feature set while preserving model prediction accuracy. Subsequently, ozone concentration predictions are conducted using six meteorological variables. Results indicate that LSTM-based methods, particularly ED-LSTM, achieve the best performance among meteorological-only models (R² = 0.479). However, predictions relying solely on meteorological variables exhibit limited accuracy. The inclusion of five pollutant variables significantly enhances the predictive performance across all machine learning methods. XGBoost demonstrates the highest accuracy (R² = 0.767, RMSE = 11.35μg/m³), representing a 125% relative improvement in R² compared to predictions using meteorological variables alone. Further implementation of the LFPM model boosts prediction accuracy for all nine machine learning methods, with XGBoost maintaining the optimal performance (R² = 0.873, RMSE = 8.17μg/m³). These findings conclusively demonstrate that integrating lagged feature variables substantially improves ozone prediction accuracy.
Keywords: Ozone, Meteorological variables, Pollutant Variables, machine learning, prediction
Received: 16 Jan 2025; Accepted: 05 Mar 2025.
Copyright: © 2025 Zhu, Liu, Yuan, Cao, Cao, Liu, Xu and Zhang. This is an open-access article distributed under the terms of the Creative Commons Attribution License (CC BY). The use, distribution or reproduction in other forums is permitted, provided the original author(s) or licensor are credited and that the original publication in this journal is cited, in accordance with accepted academic practice. No use, distribution or reproduction is permitted which does not comply with these terms.
* Correspondence:
Zitao Liu, College of Marine Sciences, Shanghai Ocean University, Shanghai, China
Disclaimer: All claims expressed in this article are solely those of the authors and do not necessarily represent those of their affiliated organizations, or those of the publisher, the editors and the reviewers. Any product that may be evaluated in this article or claim that may be made by its manufacturer is not guaranteed or endorsed by the publisher.
Research integrity at Frontiers
Learn more about the work of our research integrity team to safeguard the quality of each article we publish.